the Creative Commons Attribution 4.0 License.
the Creative Commons Attribution 4.0 License.
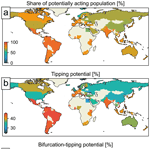
A global threshold model of enabling conditions for social tipping in pro-environmental behaviours – the role of sea level rise anticipation and climate change concern
Marc Wiedermann
Jonathan F. Donges
Jobst Heitzig
Ricarda Winkelmann
Effective climate change mitigation necessitates swift societal transformations. Positive social tipping processes, where small triggers initiate qualitative systemic shifts, are potential key mechanisms towards instigating the desired emissions mitigation. A necessary foundation for societal tipping processes is the creation of enabling conditions. Here, we assess future sea level rise estimates and social survey data within the framework of a network-based threshold model to exemplify the enabling conditions for tipping processes. We find that in many countries, the level of climate change concern is already sufficient, suggesting the enabling conditions and opportunities for social activation already exist. Further, drawing upon the interrelation between climate change concern and anticipation of future sea level rise, we report three qualitative classes of tipping potential that are regionally clustered, with the greatest potential for tipping in western Pacific Rim and East Asian countries. These findings propose a transformative pathway where climate change concern increases the social tipping potential, while extended anticipation time horizons can trigger the system towards an alternative trajectory of larger social activation for climate change mitigation.
- Article
(3724 KB) - Full-text XML
-
Supplement
(1044 KB) - BibTeX
- EndNote
With increasing greenhouse gas emissions and resultant global warming, the Earth's climate system is becoming increasingly vulnerable to irreversible and abrupt changes (Steffen et al., 2018; Armstrong McKay et al., 2022). The urgency of projected climate impacts is accentuated by interacting tipping elements in the Earth system that, once initiated, carry the potential for cascading “domino effects” (Lenton and Williams, 2013; Rocha et al., 2018; Wunderling et al., 2021). Rapid societal transformations are necessary to reduce greenhouse gas emissions and stabilize the Earth's climate system (Otto et al., 2020a; Winkelmann et al., 2022).
Contemporary societies are faced with a milieu of increased threats posed by climate change (e.g. increased risks for floods, wildfires, hurricanes, droughts). Of these threats, sea level rise (SLR) presents one of the greatest potential risks of climate change – as the impact of SLR is comparatively longer-lasting. The impact of floods, wildfires, and hurricanes can be similarly devastating to communities, yet the event itself lasts relatively shorter durations (magnitude of days), and infrastructure can be rebuilt, while for SLR, the impacts are likely irreversible (DeConto et al., 2021; Golledge, 2020), persisting at the magnitude of millennia.
With approximately 40 % of the world's population residing within 100 km of the coastline, SLR poses a global threat to coastal cities, infrastructure, and cultural heritage sites (Nicholls and Cazenave, 2010; Hinkel et al., 2014; Cazenave and Cozannet, 2014; Marzeion and Levermann, 2014). The impacts of SLR are already manifesting today (e.g. increased storm surges, flooding, groundwater salination, and harm to marine ecosystems), and the expected future impacts vary by region (Nicholls et al., 2021; Barragán and de Andrés, 2015). For example, densely populated urban centres in Japan, India, and China are among those potentially most affected by future SLR (Barragán and de Andrés, 2015) (Fig. 1b, c). Depending on the Representative Concentration Pathway (RCP) emission scenario, future SLR estimates range between 1 and 5 m (Nauels et al., 2017a) by the year 2300 (Fig. 1a), where up to 15 m of SLR by 2300 cannot be ruled out under high-emission scenarios (Masson-Delmotte et al., 2021). SLR impacts are further projected to amplify due to the large inertia and positive feedbacks (Garbe et al., 2020), locking in long-term commitments to potentially irreversible SLR (Levermann et al., 2013).
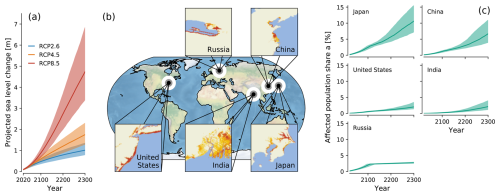
Figure 1Projected global mean sea level rise and affected world regions. (a) Projected SLR in response to greenhouse gas emissions under different RCP scenarios with low (blue), medium (orange), and high emissions (red) (estimates by MAGICC v2.0). Thick lines indicate the median projected SLR, and shaded areas indicate the range that includes 66 % of all ensemble runs. (b) Regions affected by future SLR in the five countries with the largest share of global greenhouse gas emissions. Lines indicate the areas that are directly affected by future global mean SLR within one (red), four (orange), or seven generations (yellow). (c) Average shares of population for the largest national emitters – China, United States of America, India, Russia, and Japan – that is estimated to be directly affected by projected SLR. Shaded areas again indicate the 66 % range.
The problem of future sea level rise presents a unique social–ecological dilemma: a severe clash of timescales. The most serious potential direct impacts of SLR will likely manifest on the order of centuries or more (Garbe et al., 2020; Levermann et al., 2013), but mitigation of these impacts necessitates rapid countering societal actions within the next few years to decades (Clark et al., 2016). However, future problems are often discounted (Frederick et al., 2002), and human forethought for the future becomes limited beyond the order of decades (Tonn et al., 2006; Winkelmann et al., 2022). People who do not consider distant future consequences are more likely to have climate-sceptical views (Većkalov et al., 2021) and are less likely to engage in mitigating behaviours (Milfont et al., 2012; Corral-Verdugo et al., 2017). Indeed, the dilemma of climate change presents not only a classic tragedy of the commons, but also a tragedy of the horizon (Hurlstone et al., 2020).
1.1 Social tipping as a transformative mechanism for pro-environmental behaviours
Social tipping has been proposed as a mechanism for bringing about necessary sustainability transformations and mitigating climate impacts (Milkoreit et al., 2018; Moser and Dilling, 2007; Otto et al., 2020a; Farmer et al., 2019; Tàbara et al., 2018; Nyborg et al., 2016; Lenton, 2020; Winkelmann et al., 2022). Social tipping is exemplified by qualitative systemic changes resulting from comparatively small changes within the social system or the broader environment in which the system is embedded (Winkelmann et al., 2022). Similar to the notion of climate tipping processes (Lenton et al., 2008; Armstrong McKay et al., 2022), social tipping dynamics are internally self-amplifying via positive feedback mechanisms such that rapid movements from one qualitative state into another become possible, resulting in sudden large-scale structural changes (Otto et al., 2020a; Milkoreit et al., 2018; Van Ginkel et al., 2020). Recent work has explored potential “positive tipping elements” (candidate systems for tipping) within the energy, mobility, food, and financial systems, for example (see Lenton et al., 2023, for and overview). Further, some studies have identified systems which they claim may be close to tipping or have already tipped, such as electric vehicle adoption in Norway (Lenton et al., 2022).
Social tipping processes are deeply related to other similar transformation mechanisms, where the “theory of change” initiates at the micro-level, which are eventually exhibited in structural-level changes (Geels, 2002; Olsson et al., 2014; Olsson and Moore, 2024). This is in contrast to change that initiates from the “top down” (e.g. policy change). That is, we explore how these individual changes can enact system regime changes, which themselves co-evolve to reshape the individual actions nested within these regimes. This approach should naturally be interpreted as complementary to (and not competing with) broader mechanisms towards how such rapid shifts could occur.
Within social tipping conceptualizations, two interrelated mechanisms have been proposed which shape the likelihood of the initiation of a transformation. The first is the intervention, or triggering element, which can come from a myriad of forces initiated in both natural and social systems (Winkelmann et al., 2022), as well as those emerging from diverse individual-level changes (Otto et al., 2020a; Milkoreit et al., 2018; Lenton et al., 2022). The second necessary element for social tipping processes is the development of “enabling conditions” (Tàbara et al., 2018; Lenton et al., 2022; Olsson and Moore, 2024). Here, these enabling conditions refer more to the structure of the system itself and how favourable it is to change processes. If the enabling conditions are rather “low”, a large intervention (e.g. shock, intervention) would be needed to initiate transformation processes. But, if the conditions are comparatively “high”, a wider array of even potentially unintended interventions could initiate the transformation process. Yet, how enabling conditions shape the likelihood of social tipping processes remains comparatively rather underexplored. Accordingly, we focus on how two factors, levels of climate change concern and exposure to sea level rise, can shape enabling conditions.
1.2 Climate change concern and pro-environmental behaviours
Within social systems, extensive empirical studies have focused on identifying drivers of engagement (van Valkengoed and Steg, 2019; Bergquist et al., 2022; Cologna and Siegrist, 2020) in what we will term “pro-environmental behaviours”. The idea of pro-environmental behaviours draws upon previous definitions from the broader environmental literature (Stern, 2000; Steg and Vlek, 2009) to designate an intentional action that is taken towards reducing carbon emissions. This could entail, for example, changes in individual consumption patterns, political behaviours, or even activism.
Climate change concern has been demonstrated as providing a likely necessary foundation for individual-level engagement (van Valkengoed and Steg, 2019; Hoffmann et al., 2022) in pro-environmental behaviours. Most simply, if a person is presumed to adopt a pro-environmental behaviour to mitigate the likelihood of individual or societal exposure to a climate-induced risk, they must likely first be concerned about this risk to act. Yet, concern is often insufficient, on its own, to ultimately serve as the triggering mechanism in a shift toward pro-environmental behaviour, as the role of concern is often moderated by other characteristics (Kollmuss and Agyeman, 2002), such as increased costs (Diekmann and Preisendörfer, 2003), perceptions of individual efficacy (Mayer and Smith, 2019), behavioural plasticity, (Nielsen et al., 2024), and trust (Cologna and Siegrist, 2020; Smith and Mayer, 2018). Rather, there likely needs to be an interaction with some other intervention in order to enact latent climate change concern as pro-environmental behaviour.
1.3 Anticipation of SLR can activate pro-environmental behaviours
Within social–ecological systems, experienced climate impacts (Demski et al., 2017; Konisky et al., 2016), e.g. floods and heat waves (Ricke and Caldeira, 2014), have the potential to shift attitudes and behaviours toward climate change and instigate social tipping processes (Müller et al., 2021). Behavioural changes are more likely if extreme weather events elicit an emotional response, increase the salience of climate change, or are directly attributed to climate change (Sisco, 2021).
In the case of SLR, immediate pro-environmental behaviours for mitigation are required, as even 5-year delays in reaching peak greenhouse gas (GHG) emissions can affect a future commitment of m in SLR by 2300 (Mengel et al., 2018). Yet, the most severe immediate impacts of SLR are unlikely to be experienced within current lifetimes, likely between +0.3–1.0 m by 2100 (Oppenheimer et al., 2019). Accordingly, direct experiences of SLR impacts are unlikely to trigger necessary societal changes on timescales required for mitigation (Pahl et al., 2014) within the remaining “intervention time horizon” (the period within which societal actions can influence whether a natural system tips) (Winkelmann et al., 2022).
Rather, the anticipation of the impacts of SLR presents a crucial potential pathway towards bridging the intergenerational gap. On one side, several studies have noted that sea level rise is not particularly well understood by the public (in terms of scientific accuracy; e.g. Thomas et al., 2015; Priestley et al., 2021), is seen as occurring quite far into the future (Covi and Kain, 2016), and may not receive frequent media attention (e.g. Akerlof et al., 2019). On the other side, communicating the risks posed by SLR can increase climate change attitudes – even amongst more “hard to reach” audiences such as Republicans in the United States (Bolsen et al., 2018; Smith et al., 2022). Concern about future SLR can activate desires to leave a positive legacy for the descendants of one's homeland, facilitating engagement in pro-environmental behaviours (Hurlstone et al., 2020). Recent empirical evidence suggests that concerns about future SLR constitute a unique form of societal climate risk perceptions, increasing support for climate change policies and willingness to engage in pro-climate behaviours by ∼ 15 %–30 % (Smith et al., 2020, 2022). Simulation modelling approaches have further noted the importance of anticipation of future climate impacts, where extended time horizons can foster social tipping dynamics towards stabilizing the environment and potentially preventing social–ecological collapse (Müller et al., 2021).
In this case, we suggest that anticipation of SLR can act as an instigating trigger of pro-environmental behaviour. When the enabling conditions of a system are such that social tipping processes are increasingly likely, anticipation of climate impacts may be a sufficient trigger, bridging the temporal gap between risk exposure and impacts.
Here, we explore this interrelated role of anticipation of SLR and concern for climate change in triggering social tipping processes towards pro-environmental behaviour via adoption of a network-based threshold model for social tipping. We combine projections of future SLR and cross-national social survey data on climate change concern to estimate likelihoods of social tipping processes (Ashwin et al., 2012) resulting from varying anticipation time horizons and levels of concern in 66 countries. We thereby investigate mechanisms which close the temporal gap between causes and effects of climate impacts, exploring the potential for societal transformations toward pro-environmental behaviour and planetary stewardship.
1.4 Network-based threshold model for social tipping
Models of complex social behavioural contagions (Lehmann and Ahn, 2018; Jusup et al., 2022) have explored thresholds for individual action, whereby a Pareto effect of ∼ 25 % of the population becoming activated can result in social-tipping-like processes of cascading behaviours (Centola et al., 2018). In such cases, changes in individual preference factors (Nyborg et al., 2016) and network structures (Centola and Baronchelli, 2015) can trigger rapid shifts in social norms and behaviours. Complex contagion is commonly simulated using threshold models (Dodds and Watts, 2004), social learning and diffusion models, adaptive network models (Gross and Sayama, 2009; Chu et al., 2021), or agent-based voter models (De Marchi and Page, 2014).
Recent advancements have further modelled social tipping dynamics (Müller et al., 2021) – notably, behavioural economic experimental approaches have investigated the difficulty in overcoming perceived costs associated with adopting new norms (Andreoni et al., 2021), agent-based models have identified conditions for rapid adoption of environmental behaviours (Kaaronen and Strelkovskii, 2020), and coupled social–climate models have explored emissions reduction pathways (Moore et al., 2022). Yet, modelling social tipping dynamics, and environmental behavioural change more broadly, remains challenging, as the drivers of human behaviour and preference formation are non-deterministic and are rather the product of an interrelated web of factors (e.g. risk perceptions, costs, social norms, perceived efficacy, trust, political and cultural tastes). Such modelling endeavours are further complicated within cross-cultural settings, as the drivers of climate attitudes and behaviours can vary greatly even between cultural and geographically similar locales (Smith and Mayer, 2019; Marquart-Pyatt et al., 2019).
Given these uncertainties and heterogeneities, we adopt a low-dimensional approach to modelling the interrelated role of concern and anticipation of SLR impacts that is theoretically based and driven by empirically derived parameters. We extend a recently developed refinement of Granovetter's threshold model (Granovetter, 1978) for social tipping processes (Wiedermann et al., 2020) that explores engagement in pro-environmental behaviours from cascading contagious dynamics on social networks (Watts, 2002). Pro-climate change behaviours are those taken with the intention of mitigating – or adapting to – anticipated, perceived, or experienced climate impacts (Stern, 2000). These can encompass a broad range of individual or social behaviours, such as changing consumption patterns, participating in environmental collective actions, and supporting climate-change-focused policies and political actors (Tobler et al., 2012).
This approach divides populations into three distinct groups (McCarthy and Zald, 1977; Jenkins, 1983): (I) certainly active instigators of pro-environmental behaviours (e.g. opinion shifts, social movements, or adoption of new technologies), (II) contingently active individuals whose characteristics (e.g. norms, beliefs, social identity) broadly align with those of the certainly active population but who have yet to join these pro-environmental behaviours, and (III) certainly inactive individuals who are unlikely to ever join pro-environmental behaviours (e.g. those with norms, values, or identities in opposition or those who lack the capacity to change). Specifically, we adopt a one-dimensional macroscopic approximation of an emergent threshold function which incorporates microscopic network dynamics accounting for the interrelation of behavioural contagion and network structures (see Sect. 3 for details).
We apply the network-based threshold model for social tipping to the case of SLR, examining how national-level projections of SLR impacts at varying temporal scales (2100, 2200, 2300) and climate change concern affect the share of certainly and contingently active populations across different states worldwide (Fig. 2). For this purpose we use multiple data sources to estimate the necessary parameters and input quantities: (i) future sea level projections from the MAGICC climate model (Nauels et al., 2017a) (Fig. 1a); (ii) global high-resolution topographic (Farr et al., 2007) and population distribution (Center for International Earth Science Information Network, 2016) data; and (iii) pooled, harmonized social survey data on climate change concern from 66 different countries.
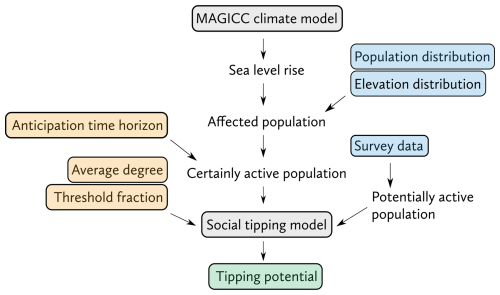
Figure 2Visualization of the modelling approach. The tipping potential in a country (green box) is estimated from sea level projections (based on climate model emulator MAGICC) and the extended Granovetter model of social tipping dynamics. Blue shading indicates external data sources (see Sect. 3). Yellow shading indicates parameters to the model. Core components of the model are marked with grey boxes.
We consider people who live in an area that is likely directly impacted by SLR within a certain anticipation time horizon to be certainly active (Group I, Fig. 1c). A country's potentially active population (the sum of Groups I and II) with share p is estimated from cross-national survey data on climate change concern (see Tables S1–S3 in the Supplement), assuming that higher levels of concern correspond to larger population shares that can potentially be mobilized for collective pro-environmental behaviour. Based on both group sizes, our model simulates the population share that ultimately participates in an action (see Fig. 3).
The more parsimonious design incorporates a similar level of qualified complexity across all model components and analytical interpretations, with the goal of minimizing sources of error originating from increased assumptions regarding additional parameters which remain unknown or are potentially even unknowable. Similar approaches have further adopted survey data and low-dimensional modelling designs to explore cross-national social tipping dynamics, particularly in the case of groundwater management (Castilla-Rho et al., 2017). Accordingly, the modelling goal is to provide grounded, interpretable, qualitative assessments of the cross-national role of concern and anticipation of SLR, providing a foundation for further exploratory studies in the emerging research on social tipping dynamics.
For every simulation, we expect to find the population share that ultimately engages in pro-environmental behaviour in one of three regimes: (i) an uncritical regime where only the untipped state exists and a large share of the population remains passive; (ii) a critical bi-stable regime where event-induced tipping (Ashwin et al., 2012) can move the system into an alternative state (i.e. potentially active population can be either active or passive, depending on initial conditions); and (iii) the tipped regime that is reached via bifurcation-induced tipping (Ashwin et al., 2012), i.e. where a large share of the potentially active population is active (see Fig. 3). By using a Monte Carlo approach, we compute the likelihoods of entering one of the three regimes and denote those as the respective per-country tipping potentials. To ensure the robustness of our results, all respective quantities are computed as averages over an ensemble of simulations for random choices of unknown parameters that govern the specific structure of the social tipping model (see Fig. 2 and Sect. 3).
3.1 Network-based threshold model for social tipping
We adopt a recently developed framework for explaining processes and mechanisms behind social tipping through a network-based micro-foundation of Granovetter's threshold model of collective behaviour (Wiedermann et al., 2020; Granovetter, 1978). The model explains collective action from cascading contagious dynamics (Watts, 2002) of social activation in a complex social network, exploring the interrelation of individual behavioural dynamics and network structures. For parsimony, we draw upon resource mobilization theory to broadly conceptualize the population as divided into three distinct groups (Jenkins, 1983; McCarthy and Zald, 1977): (i) a small fixed population share a consisting of certainly active individuals, such as instigators of a pro-environmental behaviour, that deliberately act upon a certain issue; (ii) a fixed population share c consisting of contingently active individuals whose opinions and norms align with the behaviour of the instigators but who have not joined the pro-environmental behaviour yet; and (iii) a remaining population share consisting of certainly inactive individuals who, due to opposing opinions and values, will never join a respective pro-environmental behaviour. The first two groups taken together then form the fixed potentially active population share . Actors in those groups are connected along ties in a social network, with an average number of such ties per individual, K, commonly referred to as the “average degree” of the social network (Newman, 2018). Actors become active via cascading dynamics if at least a fixed share ρ of their neighbours is active as well (Watts, 2002). The model's dynamics can be described by a one-dimensional discrete-time difference equation that computes the share of acting individuals, r(t+1), at a given time t+1 as
Here, a denotes the certainly active population share and p the potentially active population share, i.e. those that can be mobilized via social activation. F is the cumulative distribution function of the actors' activation thresholds, referred to as the emergent macroscopic threshold function. In other words, F(r(t)) represents the fraction of the contingent population (with relative size p−a) that takes part in a given pro-environmental behaviour once that movement has reached a relative size of r(t). It was first suggested (Granovetter, 1978) and then shown (Wiedermann et al., 2020) that such a threshold distribution F with typically assumed properties, such as being broad-shaped and similar to a normal distribution, emerges from pairwise social interactions between individuals in their underlying social network. In particular, assuming a random network topology given by the Erdős–Rényi model as a first approximation for the unknown underlying social network (Erdős and Rényi, 1960) yields the following analytical expression for F (Wiedermann et al., 2020).
Note that the emergent threshold function (F(r(t))) integrates information from individual behavioural thresholds and network structures and acts as a parsimonious tool for modelling social tipping processes, but the threshold function itself is rather an outcome of these processes and should not be treated as a social construct with a causal effect in its own right. Also note that even though the approximation assumes the topology of an Erdős–Rényi random network, the emergent threshold function F also represents other less trivial network structures sufficiently well (see below for details and the Supplement for numerical simulations).
The fixed points r* of Eq. (1) can be estimated by setting
and solving for r* numerically. The model shows two saddle-node bifurcations with respect to both a and p (Wiedermann et al., 2020), which is the typical form of stability landscape for a tipping element (Fig. 3a). For sufficiently large p and small values of a, i.e. a=0 in the extreme case, the model displays an uncritical regime where no tipped state exists (Fig. 3a). Increasing a pushes the system closer to a bi-stable critical regime in which external influences, such as shocks or events, can lift the system into an alternative stable state (yellow area in Fig. 3a). Further increasing a eventually triggers the commonly studied form of social tipping, i.e. bifurcation-induced tipping, where the untipped fixed point r* vanishes and the system enters the tipped regime (green area in Fig. 3a). Notably, similar dynamics and the distinction of three qualitative regimes can be observed for increasing p from low to large values, thereby giving rise to a cusp catastrophe (Wiedermann et al., 2020). In particular, for each value of p there is a critical value of a (given by the boundary between the red- and yellow-shaded area in Fig. 3) at which the system first enters the bi-stable critical regime. In that sense, increasing values of p move the boundary between the uncritical and critical regime closer to smaller values of a.
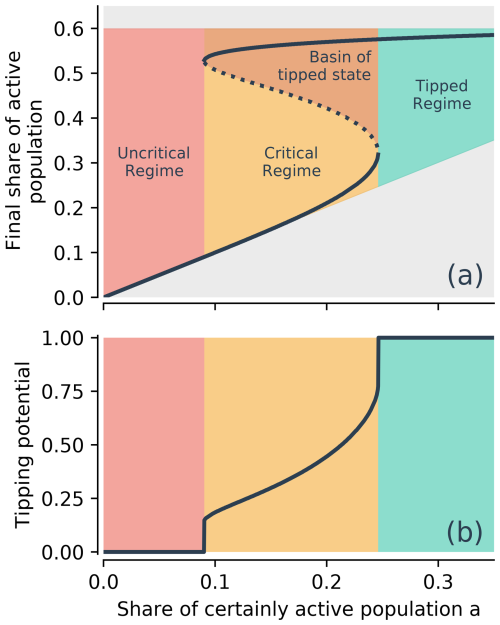
Figure 3(a) Exemplary bifurcation diagram of the social tipping model with respect to one of several influencing factors: the certainly active population share a. For low shares a of certainly active individuals the system is in the uncritical regime (red-shaded area) where no tipping is possible since only the lower branch exists. With increasing a the system enters the critical regime (yellow-shaded area) and event-induced tipping becomes possible if large enough shocks kick the steady state into the tipped state's basin of attraction (orange-shaded area). With further increasing a the model reaches its critical threshold for bifurcation-induced tipping above which only the upper branch exists (green-shaded area). (b) The tipping potential computed as the relative size of the basin of attraction of the upper branch.
Given that the social network structures within the 66 countries that are included in our simulation are likely largely heterogeneous and in many cases undetermined, we adopt a Monte Carlo approach to check for the robustness of our results by numerically estimating the potential for social tipping and resultant instantiations of emergent threshold functions by randomly sampling unknown parameter values for K and ρ. We observed that the resulting ensemble of threshold functions (F(r)) then not only resembles simple network topologies such as the Erdős–Rényi random network, but also sufficiently covers other more realistic network topologies. In particular, we perform robustness checks using an array of topologies (real-world empirical data about Facebook friendships – Barabási and Albert, 1999, Watts and Strogatz, 1998; real-world empirical data about ring topologies and random geometric networks – Dall and Christensen, 2002), finding that in most cases, the ensemble threshold function fits estimates emerging from micro-simulation models, except in cases where the certainly active nodes are heavily clustered in modular networks (see Figs. S1 and S2 in the Supplement). This is to be expected, since in networks where the certainly active population is clustered within a highly modularized network structure, it is unlikely for the network to exhibit cascading processes resulting in social tipping across a broader population, as tipping would be contained to specific clusters and not throughout the network as a whole. However, we suggest that in the case of anticipation of SLR, real-world social networks are less likely to have such highly modularized network structures, as for many countries, SLR affects broad sections of coastlines, stretching across diverse social and geographic groupings.
3.2 Estimation of certainly active population from SLR
We estimate the certainly active population share a(T) per country by the proportion of individuals that are projected to be affected by sea level rise (SLR) at a given anticipation time horizon T after 2020. Here, we assume individuals to be affected if they live at an elevation X that lies at or below projected SLR at time T.
Particularly, we use median SLR projections until 2300 obtained from the MAGICC climate model v2.0 (Nauels et al., 2017a) for the RCP8.5 scenario that provides an upper bound of the RCPs (Riahi et al., 2011) (solid red line in Fig. 1a). Generally, the MAGICC climate model emulator estimates approximately 1 m of sea level change for low-emission scenario RCP2.6, ranging up to approximately 5 m of sea level rise for high-emission scenario RCP8.5 by the year 2300 (Fig. 1a). These projections are consistent with process-based models in IPCC AR6 (Masson-Delmotte et al., 2021), which further note that even more severe SLR of more than 15 m by 2300 cannot be excluded under high-emission scenarios (IPCC, 2019).
In order to estimate the population–elevation distribution (Fig. 4) we combine country-specific gridded population data from the Socioeconomic Data and Applications Center (SEDAC) (Center for International Earth Science Information Network, 2016) with SRTM30 near-global digital elevation data (Farr et al., 2007), both provided at an angular resolution of °. Since entries in the SRTM30 data are truncated to full metres, we add uniformly distributed random noise of magnitude 1 m to the entry of each grid cell. The median SLR projections are then combined with the country-specific population–elevation distributions to obtain a time series of population shares that are affected by SLR until 2300.
3.3 Estimation of potentially active population from social survey data
We estimate the potentially active population share pC in a country C from subjects' expressed concern for climate change in six different recent cross-national social survey programmes: International Public Opinion on Climate Change (2022), International Social Survey Programme (2010, Environment III), European Social Survey (2016, Wave 8), PEW Global Attitudes Survey (spring 2015), Eurobarometer (2017, EB 87.1; 2019, EB 91.3), and Life in Transition Survey (2010, II) – see Tables S1–S3 in the Supplement.
We assume that subjects that are not concerned at all are not potentially active. In contrast, subjects with the highest level of concern are counted as surely belonging to the potentially active population share. For subjects with intermediate levels of concern, we assume that a certain share is potentially active, using the following approach to estimate this share: each survey “sp” contains an item capturing individual perceptions of climate change concern across multiple countries C. These items are given on an ordinal scale, with varying numbers of outcomes nsp. We rescale those outcomes to take integer values such that gives the relative frequency of response i in country C and survey programme “sp”. We then estimate the potentially active population share pC,sp in country C as
such that if all participants were to respond isp=0, and if all participants were to respond . In total, we compile data for 66 countries with access to the sea. Some countries, especially in the European Union, are covered by all six surveys, while other countries only appear in a single instance, with an average coverage of 2.36 survey programmes per country. In cases where multiple survey programmes are available for a particular country, we then adopt the median value of concern over all survey programmes for that country as the estimated potentially active population share pC in Fig. 5 and Fig. 6. An overview of estimated levels of concern across countries is given in Tables S2 and S3.
3.4 Preprocessing, model setup, and ensemble simulations
In alignment with earlier works (Marzeion and Levermann, 2014), we exclude countries with exceptionally low elevation profiles, i.e. the Netherlands, Azerbaijan, and Kazakhstan, that for large parts show elevation values even below the present sea level. We additionally exclude all countries that are not adjacent to any larger body of water and, thus, exclusively lie inland, e.g. Mongolia or Austria. In total, we excluded 22 countries from these analyses.
In a first step, we vary the potentially active population share p across its entire valid value range in order to obtain comprehensive statistics about the model's dynamics. We draw N=2000 random combinations of parameters and and compute r* numerically from Eq. (3) for every combination of and a(T), for . In a second analysis, we then fix the potentially active population share in each country, pC, according to the estimated levels of concern (see above).
3.5 Tipping potential and intervention sizes
The tipping potential in Figs. 5 and 6 is computed as the average basin stability (Menck et al., 2013) of the tipped fixed point (subscript “ub” for the upper branch) in Fig. 3 taken over an ensemble of N=2000 simulations with randomly drawn values of K and ρ (see above). For a single simulation s we define the tipping potential utot(s) as follows.
Here, (subscript “m” for the middle branch) is the location of the fixed point corresponding to the unstable branch of the model's bifurcation diagram (dashed line in Fig. 3a), p is the potentially active population share, and a is the certainly active population share. The total tipping potential utot is then given as follows.
The total tipping potential utot measures the combined effects of event-induced (first term on the right-hand side of Eq. 8) and bifurcation-induced tipping (second term on the right-hand side of Eq. 8). Each contribution to utot is thus either if the system is in the critical regime or utot(s)=1 if the system is in the tipped regime (Fig. 3b). When aggregated over all simulations s, the bifurcation-induced tipping potential ubif computes the share of simulations in which bifurcation tipping occurred (depicted in Fig. 5, third row) and forms a direct contribution to utot.
For all simulations s where the model's steady state falls into the critical regime (yellow-shaded area in Fig. 3), we compute the required intervention size I(s) to tip the equilibrated system from the stable untipped state (subscript “l” for the lower branch) into the stable tipped state. Specifically, we express I(s) as the minimum contingent population share required to lift the system from over the middle branch . This yields
The required intervention sizes I depicted in Fig. 5 are then computed as the respective percentiles of the set of all ensemble members s for which we obtain positive values of I(s).
4.1 Anticipation and concern foster complementary forms of social tipping
The tipping potentials are first estimated for the five countries with the largest greenhouse gas emissions (China, the US, India, Russia, and Japan) (IEA, 2020) for varying anticipation time horizons (e.g. 100, 150, or 200 years into the future) and potentially active population shares. These five countries were chosen as examples as they present cases where effective shifts towards pro-environmental behaviours might have the most substantial impact on global carbon emissions, an approach similarly adopted in other modelling work (e.g. Ricke and Caldeira, 2014). We then further assess the corresponding tipping potentials for the fixed potentially active population share according to the estimated level of climate change concern in each country (see also Sect. 3).
We find that the overall tipping potential increases strongly with greater potentially active population shares. A notable dependency of this tipping potential on the time horizon can especially be observed for countries where a larger part of the population lives at or close to sea level, as in the case of Japan or China (Fig. 5a).
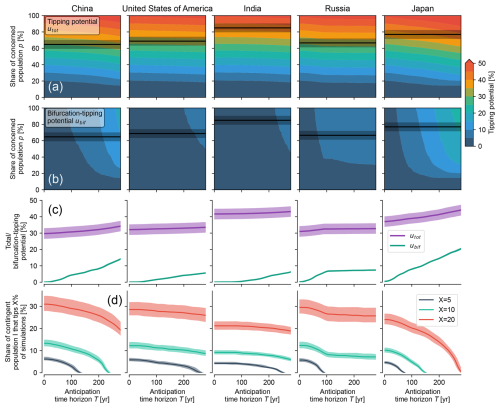
Figure 5Social tipping potential for the five highest greenhouse-gas-emitting countries: China, the USA, India, Russia, and Japan (in terms of CO2 equivalents). (a) Total tipping potential and (b) bifurcation-induced tipping potential for varying shares of concerned population p and anticipation time horizon T, from zero (blue) to more than 50 % (red). Black lines indicate the estimated country-specific climate change concern pC, with a shaded interval of ±5 % deviation indicating that variations in the level of concern do not qualitatively alter the results. (c) Total, utot, and bifurcation-induced tipping potential, ubif, for the estimated levels of country-specific climate change concern. Shaded areas indicate variations of utot and ubif within the ±5 % band of concern. (d) Size I of necessary interventions to tip a given share X of simulations once a country's social system reaches the critical regime. The temporal axis reflects the anticipation time horizon of T years prior to 2020, i.e. T=0 implies that individuals do not anticipate any future impacts, while the maximum possible value, T=280, assumes individuals to anticipate sea level impacts up to 2300, the penultimate year in the MAGICC projections (see also Fig. 1).
With extended anticipation time horizons, the bifurcation-induced tipping potential, i.e. cases where the system shifts into the tipped regime, increases most significantly if the potentially active population is larger than at least approximately 20 % (see Fig. 5b) – a level that is surpassed in all five considered countries. For the estimated values of the potentially active population, our model suggests increased bifurcation-induced tipping potential in countries with large near-sea-level population density, particularly China and Japan (Fig. 5c). This implies that while the overall tipping potential is relatively similar across contexts, bifurcation-induced tipping becomes more likely in regions which are more vulnerable to future SLR.
However, compared with the overall large potential, bifurcation-induced tipping remains comparatively unlikely (see Fig. 5a and b). This implies that even though an alternative stable state of collective pro-environmental behaviour may exist, the system is unlikely to reach this state by mere crossing of a single critical threshold or tipping point. This is because in most cases, the share of the certainly active population directly affected by SLR is not sufficient to trigger such bifurcation-induced tipping. Instead, the system is mostly found in a critical, yet not tipped, state where interventions then carry the potential to kick the system into its alternative stable state via event-induced tipping. The required intervention size, measured in terms of the contingent population share, generally decreases as the anticipation time horizon grows, moving the system closer to the tipped state (see Fig. 3). Comparatively, small intervention sizes (less than 10 %) of spontaneously activated populations can suffice to trigger transitions to the alternative state with a 5 % chance, indicating a potential for event-induced tipping (see black lines in Fig. 5d).
Additionally, our model indicates that countries with larger potentially active population shares have correspondingly lower required intervention sizes for initiating event-induced tipping (for instance, India and Japan; see Fig. 5d), as the gap is lowered between an untipped stable state and the basin of attraction of the tipped state (see the last row of Fig. 5 and Fig. 3). But, even in cases of the highest climate change concern, such as in India, our model indicates that a non-zero intervention size is needed when the anticipation time horizon is shorter, implying that at least some intervention is necessary to trigger tipping processes. Increasing the anticipation time horizon translates into substantially smaller required intervention sizes, eventually even reaching zero for the modelled cases of Japan and, in parts, China (Fig. 5d).
4.2 Three global classes of tipping processes
Drawing upon a broader comparative analysis covering 66 countries with access to the sea, we find that concern for climate change and the total tipping potential are strongly correlated (Fig. 6a and b). Accordingly, we identify three qualitative classes of tipping processes facilitating pro-environmental behaviour that vary by the country-specific values of total and bifurcation-induced tipping potentials (Table 1).
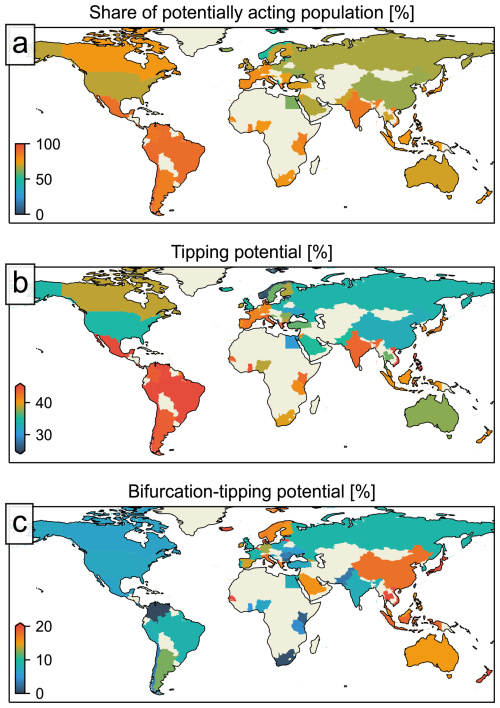
Figure 6Cross-national potential for social tipping towards increased pro-environmental behaviour. (a) Estimated country-specific potentially active population share. (Total) tipping potential (utot) (b) and bifurcation-induced tipping potential (ubif) (c) for the estimated values of climate change concern from social survey data and the largest possible anticipation time horizons. Countries are geographically clustered according to Tipping Classes I–III, i.e. countries with large total and bifurcation-induced tipping potential (Tipping Class I) in the Indo-Pacific, countries with large total and low bifurcation-induced tipping potential (Tipping Class II) in the remaining Southern Hemisphere, and low total and bifurcation-induced tipping potential (Tipping Class III) in Europe and North America.
4.2.1 Tipping Class I – large total and high bifurcation-induced tipping potential
In these countries, the emergence of social tipping processes is fostered by heightened levels of climate change concern and SLR-sensitive elevation–population profiles. In this case, an increase in the anticipation time horizon or a relatively small intervention size can trigger a transition to an alternative state of increased pro-environmental behaviour. Therefore, such social systems can be considered to already be in a rather critical state.
Examples for this class (Class I) primarily include countries along the Pacific Ocean, such as Indonesia, Japan, Vietnam, and China. Here, SLR is likely to affect large population shares due to large metropolitan areas located near the coast (Kulp and Strauss, 2019). Combined with overall heightened climate change concern in these countries (see Fig. 6a), individual events and expanded anticipation time horizons both have the potential to instigate social tipping processes in our model (Table 1).
4.2.2 Tipping Class II – large total and low bifurcation-induced tipping potential
Countries in this class are characterized by high levels of concern and low population shares likely to be affected by projected SLR. This indicates that the corresponding countries might be sufficiently close to or already in a critical state, where relatively small interventions would suffice to induce transitions towards an alternative state via event-induced tipping. However, bifurcation-induced tipping pathways resulting from expanded anticipation time horizons are comparatively unlikely due to less SLR-sensitive elevation–population profiles.
Class II mostly covers countries in South America and Africa and along the Indian Ocean. Climate change concern in those countries can often be attributed to more short-term impacts than those related to SLR, such as water scarcity, more frequent extreme weather events, or shifts in precipitation variability (Howe et al., 2019). This is also reflected within the scope of our model, as the total tipping potential (driven by climate change concern) and the bifurcation-induced potential (driven by anticipated SLR impacts) are largely uncorrelated (Fig. 6b and c). We suggest that such temporally more immediate climate impacts could potentially trigger a rapid short-term mobilization of a large population share, thereby acting as a (non-deliberate) social tipping intervention, which can effectively kick the respective social systems into an alternative state of increased pro-environmental behaviour via event-induced tipping processes.
4.2.3 Tipping Class III – small total and small bifurcation-induced tipping potential
This class is exemplified by cases where both the elevation profile and low climate change concern effectively hinder social tipping. Accordingly, only large intervention sizes potentially push the system towards an alternative state of increased pro-environmental behaviour. Further increasing concern could lower the baseline of the required intervention sizes, thereby rendering the system more critical (moving it towards Tipping Class II). However, due to the country-specific elevation profiles, increased anticipation time horizons are expected to only have minor influences on the intervention sizes required for triggering social tipping, as the projected impacts of SLR remain comparatively small.
Class III covers North America and parts of Europe. Here, immediate threats from SLR are comparatively small, since large population shares live further inland. Likewise, climate change concern is comparatively lower (see Fig. 6a), potentially due to larger perceived psychological distances to these risks (Spence et al., 2012). Such psychological distances can result from comparatively higher economic development, larger perceived preparedness for the impacts of climate change (Spence et al., 2012), and decreased vulnerability (Maiella et al., 2020). Thus, according to our model, social tipping in these counties is fostered largely through elevating climate change concern (Singh et al., 2017). Then, once concern reaches a sufficiently high level, other events or interventions would have the potential to kick the system into an alternative state of increased pro-environmental behaviour.
Notably, a fourth tipping class with low total tipping potential and a large bifurcation-induced tipping potential cannot exist since by definition the former exceeds the latter (see Sect. 3).
5.1 Summary and discussion
Here, we adopt a network-based threshold model for social tipping to explore transformative pathways towards an alternative state of increased pro-environmental behaviour resultant from climate change concern and anticipated impacts of projected sea level rise across 66 countries with access to the sea. We find that in many countries, climate change concern has reached a point where the system can be considered critical such that an alternative stable tipped state exists (where a large population share engages in pro-environmental behaviours), and sensitive interventions (e.g. policy regime changes or social movements) or stochastic events (e.g. storm surges or floods) have the potential to push the system towards that tipped state.
Our model suggests that climate change concern has reached sufficiently high levels in many countries such that social tipping processes towards increased pro-environmental behaviour could be instigated. However, concern alone is insufficient to trigger such social tipping processes. Rather, concern functions to create critical, enabling conditions under which a system becomes more susceptible to social tipping interventions (such as by political or civil society actors). Expanded anticipation time horizons can directly lower the required intervention size, thereby increasing the likelihood that social tipping dynamics will emerge.
As such, the two factors, climate change concern and anticipation time horizons, are of a complementary yet mutually beneficial nature. Increases in concern make tipping dynamics more likely to occur following external influences or interventions, while the more deterministic process largely manifests through increased anticipation time horizons. As the anticipation time horizons necessary to trigger tipping are often on a centennial timescale, event-induced tipping (Ashwin et al., 2012) via interventions presents a comparatively more likely pathway towards instigating social tipping processes in the near future.
In this way, in our model social tipping processes largely differ from those in climate systems (see also Winkelmann et al., 2022), which often focus on identifying scenarios where a (single) control parameter crosses a critical threshold, e.g. in global mean surface temperature, as the instigator of systemic transgression into an alternative stable state (Lenton et al., 2008). Instead, the notion of sensitive intervention (Farmer et al., 2019) and event-induced tipping becomes ever more important for social tipping processes, as critical thresholds of control parameters might be infeasible to reach or impossible to predict.
A key finding from our modelled results is the emergence of three qualitative classes of social tipping. Here we identify a transformation pathway towards increased potential for social tipping towards pro-environmental behaviour. While the tipping potential within a Class III state is comparatively low, it increases with climate change concern such that the possibility for tipping processes begins to exist (i.e. leading to a Class II state). Then, extended anticipation time horizons reduce the intervention sizes necessary to trigger tipping processes (Tipping Class I) such that a comparatively smaller kick within the system, or its environment, carries the potential to instigate social tipping processes.
Given the increasing social relevance of anthropogenic climate change, identifying social systems that are in more critical states can guide strategic policy entrepreneurs (Kingdon, 1995). Targeted interventions can amplify critical conditions or even provide a substantial enough kick to instigate pro-environmental behaviours. For example, climate change social movements can play a crucial role, providing a mechanism to develop new political coalitions (Weible and Sabatier, 2017), thereby increasing the salience of climate change as a political issue. Such increased salience can effectively open a policy window, allowing climate policy advocates to promote their agendas (Kingdon, 1995). Further large shifts in public opinion can punctuate sticky environmental regulatory regimes (Baumgartner and Jones, 2010) and political–institutional lock-ins (Cecere et al., 2014), resulting in the adoption of new public policies, such as carbon taxation (Lemoine and Traeger, 2016) or incentivizing development of alternative energy supplies (Patt and Lilliestam, 2018).
In contrast to common behavioural contagion modelling approaches (Dodds and Watts, 2004; Böttcher et al., 2017), our country-specific estimations of social tipping potentials are uniquely based on real-world observations (i.e. projected SLR impacts, measured elevation–population distributions, and a comprehensive review of social survey data on climate change concern). We thereby contribute a novel perspective to the growing field of social–ecological systems modelling that aims to specifically account for the dynamic interactions between biogeophysical Earth and social systems on up to planetary scales (Müller-Hansen et al., 2017; Beckage et al., 2018; Donges et al., 2020; Beckage et al., 2022; Moore et al., 2022).
Additionally, our modelling exercise expands upon previous studies of threshold dynamics and social tipping that have largely focused on social systems in isolation, either theoretically (Granovetter, 1978; Schelling, 1971), in controlled laboratory experiments (Centola et al., 2018; Centola and Baronchelli, 2015), or via network-based numerical simulations (Watts, 2002; Singh et al., 2013; Karsai et al., 2016).
Overall, we find that concern for climate change increases the criticality and likelihood for social tipping processes, and expanded anticipation time horizons and growing shares of active population move the system closer to a critical threshold, effectively reducing the required intervention size needed to ultimately kick the system into a alternative state where pro-environmental behaviour becomes the social norm. Our study highlights the potential that lies in the combination of these factors, pushing social systems closer to positive tipping points, and is thus a first step towards truly closing the loop from climate impacts to substantive societal transformation (Donges et al., 2017).
5.2 Limitations
We acknowledge that social tipping processes can be triggered by a variety of distinct external factors, such as in the aftermath of extreme events (Demski et al., 2017; Konisky et al., 2016) or in response to large-scale environmental changes (Ioris, 2020). However, our model simulates the potential for social tipping solely resulting from anticipation of projected SLR. We find that, on its own, SLR is comparatively unlikely to result in bifurcation-induced tipping. However, our approach does not yet account for other potential impacts that are directly related to SLR, such as increased flood risks, coastal erosion, or increased vulnerability to storm surges (Kulp and Strauss, 2019). These impacts are comparatively more likely to affect even larger population shares in coastal areas and are likely to occur in the coming years and decades (Muis et al., 2016; Buchanan et al., 2016; Tebaldi et al., 2012). As such, our modelling approach can be considered rather conservative in estimating potentials for tipping processes triggered by SLR-related impacts. As with any simulation, these results should be understood in line with the level of parsimony and abstraction used in this model. There is comparatively less research on how perceptions of sea level rise affect attitudes and behaviours globally compared to extreme weather impacts, like floods or droughts. Even in those cases, the effect of extreme weather on pro-environmental behaviours is mixed, with outcomes depending heavily on the design (Quoß and Rudolph, 2022). Therefore, these results should be viewed cautiously, as they are based on simplified assumptions and should not be seen as definitive.
For now, we assume a straightforward conversion of SLR into certainly active populations as a probable scenario of subjective risk assessment, in which individuals simply compare official projections with their knowledge of the elevation profile of their local region. However, SLR is projected to vary regionally, with coastal areas around the Indian Ocean and western Pacific being affected more severely than other locales (Perrette et al., 2013). Since these are also regions where climate change concern is highest, such regional SLR differences could result in even greater potential for social tipping.
Further, we acknowledge that the survey-based measure of population shares of climate change concern does not indicate the same share of awareness of risks faced by SLR in each of these countries. This is rather a limitation based upon data availability – where cross-national measures of broader concern about climate change are more widely available, here serving as a “best available data” proxy. Further, as we adopt country-level shares, we also presume that there are unaccounted for heterogeneities within countries, particularly for larger locales with varying elevation profiles. We suggest that further research explore such dynamics, likely within a given case study country. For example, within the United States, a minority of the population may be directly exposed to SLR, but larger shares of the population are concerned about climate change (exhibiting a substantial potentially active share). Particularly, recent evidence suggests that anticipation of SLR and climate change concern have a strong, positive association (r=0.76) within the United States (Smith et al., 2022), suggesting that the potentially active population does not need to be directly exposed to SLR to be conceivably motivated towards pro-environmental behaviours via networked dynamics.
Observation-based research has noted how social contagion varies by geographic factors, such as the physical distance an actor has to travel to participate (Traag et al., 2017), individual propensity for behavioural change (State and Adamic, 2015), and the complexity of contagion spreading across social network structures (Guilbeault and Centola, 2021) and forms of social interaction (Iacopini et al., 2019). Given the potentially heterogeneous social network structures across the 66 countries, we assessed the robustness of our results using numerical Monte Carlo simulations of cascading dynamics across a diverse set of common network topologies. We found that in most cases, there is a parameterization of the emergent threshold function F that is similar to what one would expect from certain other non-trivial network topologies. In that sense, our modelling approach can be interpreted not only as an ensemble of different Erdős–Rény networks, but also an ensemble of different network topologies (see the Supplement for further detailed discussion).
Our results exclude lower-lying regions (e.g. Azerbaijan, Kazakhstan, and the Netherlands) as well as those that are situated inland (e.g. Mongolia, Austria) as these present more “extreme” cases outside the likely data distribution of the remaining sample, an approach that has also been adopted by similar modelling exercises (e.g. Marzeion and Levermann, 2014). Particularly in the case of lower-lying countries, we consider these to be more specific cases that are not likely as well explained by the model (as the level of the active population would be comparatively high, likely overpredicting the potential for tipping). Accordingly, we intend to adopt a more cautious approach, where excluding such cases from our model potentially presents more “conservative” findings with regard to the tipping potential.
We ultimately note that, while illustrative, neither the mechanism we explore here nor the underlying model results should be interpreted in an overly deterministic manner. For example, tipping can in fact occur in any of the three identified classes, as concern is large enough for most countries so that they might have already entered into a critical regime. Rather, our findings suggest that as countries transition towards Tipping Class I, the tipping potential increases and the intervention size necessary to trigger social tipping decreases correspondingly.
5.3 Outlook
In addition to natural drivers, deliberate interventions by policymakers and climate actors carry the potential to instigate social tipping processes (Lenton, 2020), for instance via financial disclosure (Farmer et al., 2019), information feedbacks (Otto et al., 2020a), or climate movements (McAdam, 2017). To assess the potential for deliberately initiated societal transformations, i.e. positive social tipping (Tàbara et al., 2018; Hinkel et al., 2020; Lenton et al., 2022; Fesenfeld et al., 2022), future research should focus on identifying processes and mechanisms that lower the intervention sizes needed to kick the system into, or increase the potential to shift the system closer to, an alternative state of increased pro-environmental behaviour. Thus, the actual type of event instigating social tipping processes can emerge from an array of sources (e.g. natural or social) across varying scales (e.g. macro or micro). For now, our model is agnostic to the specific form of the instigating event but rather aims to identify factors affecting the tipping potential of systems.
The individual likelihood to engage in pro-environmental behaviours results from an interplay of internal (i.e. concern, values, attitudes, and beliefs) and external (contextual, political, economic) factors, as well as a multitude of potential barriers (i.e. current behaviours, adaptive capacity, social norms) (Kollmuss and Agyeman, 2002). Our proposed model currently assumes that behavioural change is not associated with costs to the individual. Moreover, there are unique factors within each context, many of which may even be unobservable or unknowable, that affect the capacity for tipping processes in a given social system. Still, our idealized scenario demonstrates that due to the inherent complexity of social systems (Schill et al., 2019; Levin et al., 2013) tipping processes arise from multiple intertwined and mutually co-dependent factors which potentially lead to alternative future trajectories, for example in energy, financial, or socio-political systems (Otto et al., 2020a; Farmer et al., 2019; Lenton, 2020; Sharpe and Lenton, 2021; Tàbara et al., 2018).
Future research should factor in relevant heterogeneities within and between countries, such as different forms of agency (Otto et al., 2020b; Stadelmann-Steffen et al., 2021), shifting social norms (Nyborg et al., 2016), cultural dimensions (Hofstede and Bond, 1984), the susceptibility of the country (or region) to a broader range of climate impacts, or the ability to participate in social movements or civil society, notably focusing on the potential inequality in the likelihood of SLR impacts being experienced by populations that are less likely to have the capacity to proactively mitigate or retroactively adapt.
Similarly, important future work is necessary to identify the mechanisms shaping social tipping processes, drawing upon further modelling work (such as exploring interactions between natural and social systems; Müller-Hansen et al., 2017), or utilizing further observed or experimental data. Such work would need to be well-tailored to the individual action and setting. There is much existing work that can be drawn upon from the pro-environmental behaviour literature (largely enacted by environmental social psychologists, public policy researchers, and behavioural economists). This is being complemented by emerging literature on social tipping mechanisms, often drawing upon country-specific case studies (e.g. Boulton et al., 2023; Smith, 2023). The goal of this modelling exercise was to develop a level of abstraction necessary to allow for cross-national comparisons. As such, these findings should be seen as in complement to the rich literature exploring the more specific and diverse mechanisms of environmental change (individually, socially, and politically).
As recent research has noted, social tipping processes can present risky assumptions (Milkoreit, 2023), namely that a system has the potential to be intentionally manipulated and that this manipulation would have the desired “positive” outcome. Further, transformations framed as social tipping points can be overly reductionist and not reflective of multifaceted dynamics that ultimately shape social change (Kopp et al., 2025). We recognize the validity of these concerns and wish to emphasize them here. We also recognize that this emerging field of study is still comparatively young and that recent efforts have been made towards connecting social tipping with longer-standing literature on transformation processes (e.g. Olsson and Moore, 2024), as well as developing social-science-based methodologies (e.g. Hodbod et al., 2024). Accordingly, as previously noted, our modelling exercise should be interpreted as an exploratory scenario, parameterized using country-level factors, and should not be interpreted as either deterministic or comprehensive of the diverse set of mechanisms surrounding the instigation and ultimate direction of pro-climate behaviours shaping societal transformations.
We explore one mechanism for social tipping processes, noting a potential transformative pathway resulting from the interrelation of climate change concern and anticipation of SLR via adoption of a social activation model. Given the necessity for rapid societal transformations (Rockström et al., 2023) and the potential for social tipping processes to instigate such changes (Otto et al., 2020a; Winkelmann et al., 2022), we encourage further research in this field. Future work can explore global conditions instigating social tipping, highlight unique mechanisms within country- or region-specific case studies, explore the role of short-term shocks (such as extreme events or rapid political mobilization) as triggers (Ricke and Caldeira, 2014), and identify the distinct circumstances under which diverse social tipping elements are likely to experience transformative changes.
The SRTM30 elevation data are available from the US Geological Survey (https://dds.cr.usgs.gov/srtm/version2_1/SRTM30/, NASA Shuttle Radar Topography Mission, 2013). The Gridded Population data of the World (GPW) v2 were obtained from the Center for International Earth Science Information Network (CIESEN; https://doi.org/10.7927/H49C6VHW, CIESIN, 2018). The MAGICC v2.0 sea-level rise projections were computed using the model source code (https://doi.org/10.5281/zenodo.572395, Nauels et al., 2017b) and supplementary input data (https://doi.org/10.5281/zenodo.572398, Nauels et al., 2017c). International Social Survey Programme (2020, “Environment IV”) data are available at https://doi.org/10.4232/1.14153 (ISSP Research Group, 2023). International Public Opinion on Climate Change (2022) data are available at https://climatecommunication.yale.edu/publications/international-public-opinion-on-climate-change-2022/ (Leiserowitz et al., 2022). European Social Survey (2016, Wave 8) data are available at https://doi.org/10.21338/NSD-ESS8-2016 (ESS ERIC, 2023). Eurobarometer (2017, 87.1) data are available at https://doi.org/10.4232/1.13738 (European Commission and European Parliament, Brussels, 2021). Eurobarometer (2019, 91.3) data are available at https://doi.org/10.4232/1.13372 (European Commission, Brussels, 2019). Life in Transition Survey (2010, Wave II) data are available at https://www.ebrd.com/what-we-do/economic-research-and-data/data/lits.html (European Bank for Reconstruction and Development, 2025). Pew Global Attitudes Survey (spring 2015) data are available at https://www.pewresearch.org/global/2015/06/23/spring-2015-survey/ (Pew Research Center, 2025).
The supplement related to this article is available online at https://doi.org/10.5194/esd-16-545-2025-supplement.
RW and JFD conceived the study. EKS and MW designed the research, developed and analysed the model results, and co-wrote the manuscript. JFD, JH, and RW contributed to the model design and analysis. All authors contributed to the writing of the manuscript.
At least one of the (co-)authors is a member of the editorial board of Earth System Dynamics. The peer-review process was guided by an independent editor, and the authors also have no other competing interests to declare.
Publisher's note: Copernicus Publications remains neutral with regard to jurisdictional claims made in the text, published maps, institutional affiliations, or any other geographical representation in this paper. While Copernicus Publications makes every effort to include appropriate place names, the final responsibility lies with the authors.
This article is part of the special issue “Tipping points in the Anthropocene”. It is a result of the “Tipping Points: From Climate Crisis to Positive Transformation” international conference hosted by the Global Systems Institute (GSI) and University of Exeter (12–14 September 2022), as well as the associated creation of a Tipping Points Research Alliance by GSI and the Potsdam Institute for Climate Research, Exeter, Great Britain, 12–14 September 2022.
We thank Alexander Nauels for providing the sea level rise projections computed from the MAGICC climate model v2.0. We also thank Julia Bognar, Christina Eder, and Alexia Katsanidou for their insightful feedback and suggestions. This work was developed in the context of the COPAN collaboration at the Potsdam Institute for Climate Impact Research (PIK). Marc Wiedermann and E. Keith Smith were supported by the Leibniz Association (project DOMINOES). E. Keith Smith is supported by funding from the European Union's Horizon Europe research and innovation programme under grant agreement no. 101056891 (CAPABLE). Jonathan F. Donges is grateful for financial support by the Stordalen Foundation via the Planetary Boundary Research Network (PB.net), the Earth League's EarthDoc programme, the European Research Council advanced grant project ERA (Earth Resilience in the Anthropocene; grant ERC-2016-ADG-743080), and the German Federal Ministry for Education and Research (BMBF) via the project “PIK Change” under grant 01LS2001A. The authors gratefully acknowledge the European Regional Development Fund (ERDF), BMBF, and the Land Brandenburg for providing resources on the high-performance computing system at PIK.
This research has been supported by the Leibniz-Gemeinschaft (grant no. SAW-2017-PIK-4), the Bundesministerium für Bildung und Forschung (grant no. 01LS2001A), the European Research Council, Horizon Europe (grant no. ERC-2016-ADG-743080), the Stordalen Foundation (Planetary Boundary Research Network), and the Earth League (EarthDoc programme).
This paper was edited by Steven R. Smith and reviewed by four anonymous referees.
Akerlof, K., Merrill, J., Yusuf, J.-E., Covi, M., and Rohring, E.: Key beliefs and attitudes for sea-level rise policy, Coast. Manage., 47, 406–428, 2019. a
Andreoni, J., Nikiforakis, N., and Siegenthaler, S.: Predicting social tipping and norm change in controlled experiments, P. Natl. Acad. Sci. USA, 118, e2014893118, https://doi.org/10.1073/pnas.2014893118, 2021. a
Armstrong McKay, D. I., Staal, A., Abrams, J. F., Winkelmann, R., Sakschewski, B., Loriani, S., Fetzer, I., Cornell, S. E., Rockström, J., and Lenton, T. M.: Exceeding 1.5 C global warming could trigger multiple climate tipping points, Science, 377, eabn7950, https://doi.org/10.1126/science.abn7950, 2022. a, b
Ashwin, P., Wieczorek, S., Vitolo, R., and Cox, P.: Tipping points in open systems: bifurcation, noise-induced and rate-dependent examples in the climate system, Philos. T. R. Soc. A, 370, 1166–1184, 2012. a, b, c, d
Barabási, A.-L. and Albert, R.: Emergence of Scaling in Random Networks, Science, 286, 509–512, https://doi.org/10.1126/science.286.5439.509, 1999. a
Barragán, J. M. and de Andrés, M.: Analysis and trends of the world's coastal cities and agglomerations, Ocean Coast. Manage., 114, 11–20, https://doi.org/10.1016/j.ocecoaman.2015.06.004, 2015. a, b
Baumgartner, F. R. and Jones, B. D.: Agendas and instability in American politics, University of Chicago Press, Chicago, ISBN 978-0-226-03949-7, 2010. a
Beckage, B., Gross, L. J., Lacasse, K., Carr, E., Metcalf, S. S., Winter, J. M., Howe, P. D., Fefferman, N., Franck, T., Zia, A., and Kinzig, A/: Linking models of human behaviour and climate alters projected climate change, Nat. Clim. Change, 8, 79–84, 2018. a
Beckage, B., Moore, F. C., and Lacasse, K.: Incorporating human behaviour into Earth system modelling, Nature Human Behaviour, 6, 1493–1502, 2022. a
Bergquist, M., Nilsson, A., Harring, N., and Jagers, S. C.: Meta-analyses of fifteen determinants of public opinion about climate change taxes and laws, Nat. Clim. Change, 12, 235–240, 2022. a
Bolsen, T., Kingsland, J., and Palm, R.: The impact of frames highlighting coastal flooding in the USA on climate change beliefs, Climatic Change, 147, 359–368, 2018. a
Böttcher, L., Nagler, J., and Herrmann, H. J.: Critical behaviors in contagion dynamics, Phys. Rev. Lett., 118, 088301, https://doi.org/10.1103/PhysRevLett.118.088301, 2017. a
Boulton, C. A., Buxton, J. E., and Lenton, T. M.: Early opportunity signals of a tipping point in the UK's second-hand electric vehicle market, EGUsphere [preprint], https://doi.org/10.5194/egusphere-2023-2234, 2023. a
Buchanan, M. K., Kopp, R. E., Oppenheimer, M., and Tebaldi, C.: Allowances for evolving coastal flood risk under uncertain local sea-level rise, Climatic Change, 137, 347–362, 2016. a
Castilla-Rho, J. C., Rojas, R., Andersen, M. S., Holley, C., and Mariethoz, G.: Social tipping points in global groundwater management, Nature Human Behaviour, 1, 640–649, 2017. a
Cazenave, A. and Cozannet, G. L.: Sea level rise and its coastal impacts, Earth's Future, 2, 15–34, https://doi.org/10.1002/2013EF000188, 2014. a
Cecere, G., Corrocher, N., Gossart, C., and Ozman, M.: Lock-in and path dependence: an evolutionary approach to eco-innovations, J. Evol. Econ., 24, 1037–1065, 2014. a
Center for International Earth Science Information Network: Gridded population of the world, version 4 (GPWv4): population density, NASA Socioeconomic Data and Applications Center (SEDAC) Palisades, NY, https://doi.org/10.7927/H49C6VHW, 2016. a, b
Center for International Earth Science Information Network (CIESIN): Columbia University, Gridded Population of the World, Version 4 (GPWv4): Population Density, Revision 11, Palisades, NY, NASA Socioeconomic Data and Applications Center (SEDAC) [data set], https://doi.org/10.7927/H49C6VHW, 2018.
Centola, D. and Baronchelli, A.: The spontaneous emergence of conventions: An experimental study of cultural evolution, P. Natl. Acad. Sci. USA, 112, 1989–1994, 2015. a, b
Centola, D., Becker, J., Brackbill, D., and Baronchelli, A.: Experimental evidence for tipping points in social convention, Science, 360, 1116–1119, 2018. a, b
Chu, O., Donges, J., Pop-Eleches, G., and Robertson, G.: The micro-dynamics of geographic polarization: a model and an application to survey data from Ukraine, P. Natl. Acad. Sci. USA, 118, e2104194, https://doi.org/10.1073/pnas.2104194118, 2021. a
Clark, P. U., Shakun, J. D., Marcott, S. A., Mix, A. C., Eby, M., Kulp, S., Levermann, A., Milne, G. A., Pfister, P. L., Santer, B. D., Schrag, D. P., Solomon, S., Stocker, T. F., Strauss, B. H., Weaver, A. J., Winkelmann, R., Archer, D., Bard, E., Goldner, A., Lambeck, K., Pierrehumbert, R. T., and Plattner, G.-K.: Consequences of twenty-first-century policy for multi-millennial climate and sea-level change, Nat. Clim. Change, 6, 360–369, https://doi.org/10.1038/nclimate2923, 2016. a
Cologna, V. and Siegrist, M.: The role of trust for climate change mitigation and adaptation behaviour: A meta-analysis, J. Environ. Psychol., 69, 101428, https://doi.org/10.1016/j.jenvp.2020.101428, 2020. a, b
Corral-Verdugo, V., Caso-Niebla, J., Tapia-Fonllem, C., and Frías-Armenta, M.: Consideration of immediate and future consequences in accepting and responding to anthropogenic climate change, Psychology, 8, 1519–1531, 2017. a
Covi, M. P. and Kain, D. J.: Sea-level rise risk communication: Public understanding, risk perception, and attitudes about information, Environ. Commun., 10, 612–633, 2016. a
Dall, J. and Christensen, M.: Random geometric graphs, Phys. Rev. E, 66, 016121, https://doi.org/10.1103/PhysRevE.66.016121, 2002. a
DeConto, R. M., Pollard, D., Alley, R. B., Velicogna, I., Gasson, E., Gomez, N., Sadai, S., Condron, A., Gilford, D. M., Ashe, E. L., and Kopp, R. E.: The Paris Climate Agreement and future sea-level rise from Antarctica, Nature, 593, 83–89, 2021. a
De Marchi, S. and Page, S. E.: Agent-based models, Annu. Rev. Polit. Sci., 17, 1–20, 2014. a
Demski, C., Capstick, S., Pidgeon, N., Sposato, R. G., and Spence, A.: Experience of extreme weather affects climate change mitigation and adaptation responses, Climatic Change, 140, 149–164, https://doi.org/10.1007/s10584-016-1837-4, 2017. a, b
Diekmann, A. and Preisendörfer, P.: Green and Greenback: The Behavioral Effects of Environmental Attitudes in Low-Cost and High-Cost Situations, Ration. Soc., 15, 441–472, https://doi.org/10.1177/1043463103154002, 2003. a
Dodds, P. S. and Watts, D. J.: Universal behavior in a generalized model of contagion, Phys. Rev. Lett., 92, 218701, https://doi.org/10.1103/PhysRevLett.92.218701, 2004. a, b
Donges, J. F., Winkelmann, R., Lucht, W., Cornell, S. E., Dyke, J. G., Rockström, J., Heitzig, J., and Schellnhuber, H. J.: Closing the loop: Reconnecting human dynamics to Earth System science, The Anthropocene Review, 4, 151–157, 2017. a
Donges, J. F., Heitzig, J., Barfuss, W., Wiedermann, M., Kassel, J. A., Kittel, T., Kolb, J. J., Kolster, T., Müller-Hansen, F., Otto, I. M., Zimmerer, K. B., and Lucht, W.: Earth system modeling with endogenous and dynamic human societies: the copan:CORE open World–Earth modeling framework, Earth Syst. Dynam., 11, 395–413, https://doi.org/10.5194/esd-11-395-2020, 2020. a
Erdős, P. and Rényi, A.: On the evolution of random graphs, Publ. Math. Inst. Hung. Acad. Sci., 5, 17–61, https://doi.org/10.1515/9781400841356.38, 1960. a
European Bank for Reconstruction and Development: https://www.ebrd.com/what-we-do/economic-research-and-data/data/lits.html, last access: 14 April 2025.
European Commission, Brussels: Eurobarometer 91.3 (2019), GESIS Data Archive, Cologne, ZA7572 Data file Version 1.0.0, [data set], https://doi.org/10.4232/1.13372, 2019.
European Commission and European Parliament, Brussels: Eurobarometer 87.1 (2017), GESIS Data Archive, Cologne, ZA6861 Data file Version 2.0.0, [data set], https://doi.org/10.4232/1.13738, 2021.
European Social Survey European Research Infrastructure (ESS ERIC): ESS round 8 – 2016, Welfare attitudes, Attitudes to climate change, Sikt – Norwegian Agency for Shared Services in Education and Research, https://doi.org/10.21338/NSD-ESS8-2016, 2023.
Farmer, J., Hepburn, C., Ives, M., Hale, T., Wetzer, T., Mealy, P., Rafaty, R., Srivastav, S., and Way, R.: Sensitive intervention points in the post-carbon transition, Science, 364, 132–134, 2019. a, b, c, d
Farr, T. G., Rosen, P. A., Caro, E., Crippen, R., Duren, R., Hensley, S., Kobrick, M., Paller, M., Rodriguez, E., Roth, L., and Seal, D.: The shuttle radar topography mission, Rev. Geophys., 45, 1–33, https://doi.org/10.1029/2005RG000183, 2007. a, b
Fesenfeld, L. P., Schmid, N., Finger, R., Mathys, A., and Schmidt, T. S.: The politics of enabling tipping points for sustainable development, One Earth, 5, 1100–1108, 2022. a
Frederick, S., Loewenstein, G., and O'Donoghue, T.: Time Discounting and Time Preference: A Critical Review, J. Econ. Lit., 40, 351–401, 2002. a
Garbe, J., Albrecht, T., Levermann, A., Donges, J. F., and Winkelmann, R.: The hysteresis of the Antarctic ice sheet, Nature, 585, 538–544, 2020. a, b
Geels, F. W.: Technological transitions as evolutionary reconfiguration processes: a multi-level perspective and a case-study, Res. Policy, 31, 1257–1274, 2002. a
Golledge, N. R.: Long-term projections of sea-level rise from ice sheets, WIRes Clim. Change, 11, e634, https://doi.org/10.1002/wcc.634, 2020. a
Granovetter, M.: Threshold models of collective behavior, Am. J. Sociol., 83, 1420–1443, 1978. a, b, c, d
Gross, T. and Sayama, H. (Eds.): Adaptive networks, Springer, https://doi.org/10.1007/978-3-642-01284-6 1, 2009. a
Guilbeault, D. and Centola, D.: Topological measures for identifying and predicting the spread of complex contagions, Nat. Commun., 12, 1–9, 2021. a
Hinkel, J., Lincke, D., Vafeidis, A. T., Perrette, M., Nicholls, R. J., Tol, R. S., Marzeion, B., Fettweis, X., Ionescu, C., and Levermann, A.: Coastal flood damage and adaptation costs under 21st century sea-level rise, P. Natl. Acad. Sci. USA, 111, 3292–3297, 2014. a
Hinkel, J., Mangalagiu, D., Bisaro, A., and Tàbara, J. D.: Transformative narratives for climate action, Climatic Change, 160, 495–506, https://doi.org/10.1007/s10584-020-02761-y, 2020. a
Hodbod, J., Milkoreit, M., Baggio, J., Mathias, J.-D., and Schoon, M.: Principles for a case study approach to social tipping points, in: Positive Tipping Points Towards Sustainability: Understanding the Conditions and Strategies for Fast Decarbonization in Regions, 79–99, Springer International Publishing Cham, https://doi.org/10.1007/978-3-031-50762-5, 2024. a
Hoffmann, R., Muttarak, R., Peisker, J., and Stanig, P.: Climate change experiences raise environmental concerns and promote Green voting, Nat. Clim. Change, 12, 148–155, 2022. a
Hofstede, G. and Bond, M. H.: Hofstede's culture dimensions: An independent validation using Rokeach's value survey, J. Cross Cult. Psychol., 15, 417–433, 1984. a
Howe, P. D., Marlon, J. R., Mildenberger, M., and Shield, B. S.: How will climate change shape climate opinion?, Environ. Res. Lett., 14, 113001, https://doi.org/10.1088/1748-9326/ab466a, 2019. a
Hurlstone, M. J., Price, A., Wang, S., Leviston, Z., and Walker, I.: Activating the legacy motive mitigates intergenerational discounting in the climate game, Global Environ. Change, 60, 102008, https://doi.org/10.1016/j.gloenvcha.2019.102008, 2020. a, b
Iacopini, I., Petri, G., Barrat, A., and Latora, V.: Simplicial models of social contagion, Nat. Commun., 10, 1–9, 2019. a
IEA (International Energy Agency): CO2 Emissions from Fuel Combustion, IEA, 2020. a
IPCC: IPCC Special Report on the Ocean and Cryosphere in a Changing Climate, edited by: Pörtner, H.-O., Roberts, D. C., Masson-Delmotte, V., Zhai, P., Tignor, M., Poloczanska, E., Mintenbeck, K., Alegría, A., Nicolai, M., Okem, A., Petzold, J., Rama, B., and Weyer, N. M.: Cambridge University Press. https://doi.org/10.1017/9781009157964, 2019. a
Ioris, A. A.: Socioecological economics of water development in the Brazilian Amazon: Elements for a critical reflection, Ecol. Econ., 173, https://doi.org/10.1016/j.ecolecon.2020.106654, 2020. a
ISSP Research Group: International Social Survey Programme: Environment IV – ISSP 2020, GESIS, Cologne, ZA7650 Data file Version 2.0.0, https://doi.org/10.4232/1.14153, 2023.
Jenkins, J. C.: Resource mobilization theory and the study of social movements, Annu. Rev. Sociol., 9, 527–553, 1983. a, b
Jusup, M., Holme, P., Kanazawa, K., Takayasu, M., Romić, I., Wang, Z., Geček, S., Lipić, T., Podobnik, B., Wang, L., and Luo, W.: Social physics, Phys. Rep., 948,1–148, https://doi.org/10.1016/j.physrep.2021.10.005, 2022. a
Kaaronen, R. O. and Strelkovskii, N.: Cultural evolution of sustainable behaviors: Pro-environmental tipping points in an agent-based model, One Earth, 2, 85–97, 2020. a
Karsai, M., Iñiguez, G., Kikas, R., Kaski, K., and Kertész, J.: Local cascades induced global contagion: How heterogeneous thresholds, exogenous effects, and unconcerned behaviour govern online adoption spreading, Sci. Rep., 6, 1–10, 2016. a
Kingdon, J. W.: Agendas, alternatives, and public policies, Little, Brown, Boston, ISBN 978-0316493918, 1995. a, b
Kollmuss, A. and Agyeman, J.: Mind the Gap: Why do people act environmentally and what are the barriers to pro-environmental behavior?, Environ. Educ. Res., 8, 239–260, https://doi.org/10.1080/13504620220145401, 2002. a, b
Konisky, D. M., Hughes, L., and Kaylor, C. H.: Extreme weather events and climate change concern, Climatic Change, 134, 533–547, https://doi.org/10.1007/s10584-015-1555-3, 2016. a, b
Kopp, R. E., Gilmore, E. A., Shwom, R. L., Adams, H., Adler, C., Oppenheimer, M., Patwardhan, A., Russill, C., Schmidt, D. N., and York, R.: “Tipping points” confuse and can distract from urgent climate action, Nat. Clim. Change, 15, 29–36, 2025. a
Kulp, S. A. and Strauss, B. H.: New elevation data triple estimates of global vulnerability to sea-level rise and coastal flooding, Nat. Commun., 10, 1–12, 2019. a, b
Lehmann, S. and Ahn, Y.-Y.: Complex spreading phenomena in social systems, Springer, https://doi.org/10.1007/978-3-319-77332-2, 2018. a
Leiserowitz, A., Carman, J., Buttermore, N., Neyens, L., Rosenthal, S., Marlon, J., Schneider, J., and Mulcahy, K.: International Public Opinion on Climate Change, 2022, New Haven, CT: Yale Program on Climate Change Communication and Data for Good at Meta, https://climatecommunication.yale.edu/publications/international-public-opinion-on-climate-change-2022/ (last access: 14 April 2025), 2022.
Lemoine, D. and Traeger, C. P.: Economics of tipping the climate dominoes, Nat. Clim. Change, 6, 514–519, 2016. a
Lenton, T. M.: Tipping positive change, Philos. T. R. Soc. B, 375, 20190123, https://doi.org/10.1098/rstb.2019.0123, 2020. a, b, c
Lenton, T. M. and Williams, H. T.: On the origin of planetary-scale tipping points, Trend. Ecol. Evol., 28, 380–382, 2013. a
Lenton, T. M., Held, H., Kriegler, E., Hall, J. W., Lucht, W., Rahmstorf, S., and Schellnhuber, H. J.: Tipping elements in the Earth's climate system, P. Natl. Acad. Sci. USA, 105, 1786–1793, 2008. a, b
Lenton, T. M., Benson, S., Smith, T., Ewer, T., Lanel, V., Petykowski, E., Powell, T. W., Abrams, J. F., Blomsma, F., and Sharpe, S.: Operationalising positive tipping points towards global sustainability, Global Sustainability, 5, e1, https://doi.org/10.1017/sus.2021.30, 2022. a, b, c, d
Lenton, T. M., Armstrong McKay, D. I., Loriani, S., Abrams, J. F., Lade, S. J., Donges, J. F., Milkoreit, M., Powell, T., Smith, S. R., Zimm, C., Buxton, J. E., Laybourn, L., Ghadiali, A., and Dyke, J. G.: The Global Tipping Points Report 2023, University of Exeter, Exeter, UK, https://report-2023.global-tipping-points.org/ (last access: 9 April 2025), 2023. a
Levermann, A., Clark, P. U., Marzeion, B., Milne, G. A., Pollard, D., Radic, V., and Robinson, A.: The multimillennial sea-level commitment of global warming, P. Natl. Acad. Sci. USA, 110, 13745–13750, 2013. a, b
Levin, S., Xepapadeas, T., Crépin, A.-S., Norberg, J., De Zeeuw, A., Folke, C., Hughes, T., Arrow, K., Barrett, S., Daily, G., and Ehrlich, P.: Social-ecological systems as complex adaptive systems: modeling and policy implications, Environ. Dev. Econ., 18, 111–132, 2013. a
Maiella, R., La Malva, P., Marchetti, D., Pomarico, E., Di Crosta, A., Palumbo, R., Cetara, L., Di Domenico, A., and Verrocchio, M. C.: The psychological distance and climate change: A systematic review on the mitigation and adaptation behaviors, Front. Psychol., 11, 2459, https://doi.org/10.3389/fpsyg.2020.568899, 2020. a
Marquart-Pyatt, S. T., Qian, H., Houser, M. K., and McCright, A. M.: Climate change views, energy policy preferences, and intended actions across welfare state regimes: Evidence from the European Social Survey, Int. J. Sociol., 49, 1–26, 2019. a
Marzeion, B. and Levermann, A.: Loss of cultural world heritage and currently inhabited places to sea-level rise, Environ. Res. Lett., 9, 034001, https://doi.org/10.1088/1748-9326/9/3/034001, 2014. a, b, c
Masson-Delmotte, V., Zhai, P., Pirani, A., Connors, S., Péan, C., Berger, S., Caud, N., Chen, Y., Goldfarb, L., Gomis, M., Huang, M., Leitzell, K., Lonnoy, E., Matthews, J., Maycock, T., Waterfield, T., Yelekçi, O., Yu, R., and Zhou, B.: IPCC, 2021: Summary for Policymakers, in: Climate Change 2021: The Physical Science Basis. Contribution of Working Group I to the Sixth Assessment Report of the Intergovernmental Panel on Climate Change, Cambridge University Press, https://doi.org/10.1017/9781009157896.001, 2021. a, b
Mayer, A. and Smith, E. K.: Unstoppable climate change? The influence of fatalistic beliefs about climate change on behavioural change and willingness to pay cross-nationally, Clim. Policy, 19, 511–523, 2019. a
McAdam, D.: Social Movement Theory and the Prospects for Climate Change Activism in the United States, Annu. Rev. Polit. Sci., 20, 189–208, https://doi.org/10.1146/annurev-polisci-052615-025801, 2017. a
McCarthy, J. D. and Zald, M. N.: Resource mobilization and social movements: A partial theory, Am. J. Sociol., 82, 1212–1241, 1977. a, b
Menck, P. J., Heitzig, J., Marwan, N., and Kurths, J.: How basin stability complements the linear-stability paradigm, Nat. Phys., 9, 89–92, 2013. a
Mengel, M., Nauels, A., Rogelj, J., and Schleussner, C.-F.: Committed sea-level rise under the Paris Agreement and the legacy of delayed mitigation action, Nat. Commun., 9, 601, https://doi.org/10.1038/s41467-018-02985-8, 2018. a
Milfont, T. L., Wilson, J., and Diniz, P.: Time perspective and environmental engagement: A meta-analysis, Int. J. Psychol., 47, 325–334, 2012. a
Milkoreit, M.: Social tipping points everywhere? – Patterns and risks of overuse, WIRes Clim. Change, 14, e813, https://doi.org/10.1002/wcc.813, 2023. a
Milkoreit, M., Hodbod, J., Baggio, J., Benessaiah, K., Calderón-Contreras, R., Donges, J. F., Mathias, J.-D., Rocha, J. C., Schoon, M., and Werners, S. E.: Defining tipping points for social-ecological systems scholarship – an interdisciplinary literature review, Environ. Res. Lett., 13, 033005, https://doi.org/10.1088/1748-9326/aaaa75, 2018. a, b, c
Moore, F. C., Lacasse, K., Mach, K. J., Shin, Y. A., Gross, L. J., and Beckage, B.: Determinants of emissions pathways in the coupled climate–social system, Nature, 603, 103–111, 2022. a, b
Moser, S. C. and Dilling, L.: Toward the social tipping point: creating a climate for change, 491–516, Cambridge University Press, https://doi.org/10.1017/CBO9780511535871.035, 2007. a
Muis, S., Verlaan, M., Winsemius, H. C., Aerts, J. C., and Ward, P. J.: A global reanalysis of storm surges and extreme sea levels, Nat. Commun., 7, 1–12, 2016. a
Müller, P. M., Heitzig, J., Kurths, J., Lüdge, K., and Wiedermann, M.: Anticipation-induced social tipping: can the environment be stabilised by social dynamics?, Eur. Phys. J.-Spec. Top., 230, 3189–3199, https://doi.org/10.1140/epjs/s11734-021-00011-5, 2021. a, b, c
Müller-Hansen, F., Schlüter, M., Mäs, M., Donges, J. F., Kolb, J. J., Thonicke, K., and Heitzig, J.: Towards representing human behavior and decision making in Earth system models – an overview of techniques and approaches, Earth Syst. Dynam., 8, 977–1007, https://doi.org/10.5194/esd-8-977-2017, 2017. a, b
NASA Shuttle Radar Topography Mission (SRTM): Shuttle Radar Topography Mission (SRTM) Global, Distributed by OpenTopography, https://dds.cr.usgs.gov/srtm/version2_1/SRTM30/ (last access: 14 April 2025), 2013.
Nauels, A., Meinshausen, M., Mengel, M., Lorbacher, K., and Wigley, T. M. L.: Synthesizing long-term sea level rise projections – the MAGICC sea level model v2.0, Geosci. Model Dev., 10, 2495–2524, https://doi.org/10.5194/gmd-10-2495-2017, 2017a. a, b, c
Nauels, A., Meinshausen, M., Mengel, M., Lorbacher, K., and Wigley, T. M. L.: Source code for “Synthesizing long-term sea level rise projections – the MAGICC sea level model v2.0”, Zenodo [code], https://doi.org/10.5281/zenodo.572395, 2017b. a
Nauels, A., Meinshausen, M., Mengel, M., Lorbacher, K., and Wigley, T. M. L.: Supporting data for “Synthesizing long-term sea level rise projections – the MAGICC sea level model v2.0”, Zenodo [data set], https://doi.org/10.5281/zenodo.572398, 2017c. a
Newman, M.: Networks, Oxford university press, https://doi.org/10.1093/oso/9780198805090.001.0001, 2018. a
Nicholls, R. J. and Cazenave, A.: Sea-Level Rise and Its Impact on Coastal Zones, Science, 328, 1517–1520, https://doi.org/10.1126/science.1185782, 2010. a
Nicholls, R. J., Lincke, D., Hinkel, J., Brown, S., Vafeidis, A. T., Meyssignac, B., Hanson, S. E., Merkens, J.-L., and Fang, J.: A global analysis of subsidence, relative sea-level change and coastal flood exposure, Nat. Clim. Change, 11, 338–342, https://doi.org/10.1038/s41558-021-00993-z, 2021. a
Nielsen, K. S., Cologna, V., Bauer, J. M., Berger, S., Brick, C., Dietz, T., Hahnel, U. J., Henn, L., Lange, F., Stern, P. C., et al.: Realizing the full potential of behavioural science for climate change mitigation, Nat. Clim. Change, 14, 322–330, https://doi.org/10.1038/s41558-024-01951-1, 2024. a
Nyborg, K., Anderies, J. M., Dannenberg, A., Lindahl, T., Schill, C., Schlüter, M., Adger, W. N., Arrow, K. J., Barrett, S., Carpenter, S., Chapin, F. S., III, Crépin, A.-S., Daily, G., Ehrlich, P., Folke, C., Jager, W., Kautsky, N., Levin, S. A., Madsen, O. J., Polasky, S., Scheffer, M., Walker, B., Weber, E. U., Wilen, J., Xepapadeas, A., and de Zeeuw, A.: Social norms as solutions, Science, 354, 42–43, 2016. a, b, c
Olsson, P. and Moore, M.-L.: Transformations, agency and positive tipping points: A resilience-based approach, in: Positive Tipping Points Towards Sustainability: Understanding the Conditions and Strategies for Fast Decarbonization in Regions, 59–77, Springer International Publishing Cham, https://doi.org/10.1007/978-3-031-50762-5_4, 2024. a, b, c
Olsson, P., Galaz, V., and Boonstra, W. J.: Sustainability transformations: a resilience perspective, Ecol. Soc., 19, https://doi.org/10.5751/ES-06799-190401, 2014. a
Oppenheimer, M., Glavovic, B., Hinkel, J., van de Wal, R., Magnan, A. K., Abd-Elgawad, A., Cai, R., Cifuentes-Jara, M., Deconto, R. M., Ghosh, T., Hay, J., Isla, F., Marzeion, B., Meyssignac, B., and Sebesvari, Z.: Sea level rise and implications for low lying islands, coasts and communities, 321–445, Cambridge University Press, https://doi.org/10.1017/9781009157964.006, 2019. a
Otto, I. M., Donges, J. F., Cremades, R., Bhowmik, A., Hewitt, R. J., Lucht, W., Rockström, J., Allerberger, F., McCaffrey, M., Doe, S. S., Lenferna, A., Morán, N., van Vuuren, D. P., and Schellnhuber, H. J.: Social tipping dynamics for stabilizing Earth's climate by 2050, P. Natl. Acad. Sci. USA, 117, 2354–2365, 2020a. a, b, c, d, e, f, g
Otto, I. M., Wiedermann, M., Cremades, R., Donges, J. F., Auer, C., and Lucht, W.: Human agency in the Anthropocene, Ecol. Econ., 167, 106463, https://doi.org/10.1016/j.ecolecon.2019.106463, 2020b. a
Pahl, S., Sheppard, S., Boomsma, C., and Groves, C.: Perceptions of time in relation to climate change, WIRes Clim. Change, 5, 375–388, 2014. a
Patt, A. and Lilliestam, J.: The case against carbon prices, Joule, 2, 2494–2498, 2018. a
Perrette, M., Landerer, F., Riva, R., Frieler, K., and Meinshausen, M.: A scaling approach to project regional sea level rise and its uncertainties, Earth Syst. Dynam., 4, 11–29, https://doi.org/10.5194/esd-4-11-2013, 2013. a
Pew Research Center: https://www.pewresearch.org/global/2015/06/23/spring-2015-survey/, last access: 14 April 2025.
Priestley, R. K., Heine, Z., and Milfont, T. L.: Public understanding of climate change-related sea-level rise, PLoS One, 16, e0254348, https://doi.org/10.1371/journal.pone.0254348, 2021. a
Quoß, F. and Rudolph, L.: Operationalisation matters: Weather extremes as noisy natural experiment show no influence on political attitudes, OSF [preprint], https://doi.org/10.1007/s10584-024-03842-y, 2022. a
Riahi, K., Krey, V., Rao, S., Chirkov, V., Fischer, G., Kolp, P., Kindermann, G., Nakicenovic, N., and Rafai, P.: RCP-8.5: Exploring the consequence of high emission trajectories, Climatic Change, 10, 33–57, https://doi.org/10.1007/s10584-011-0149-y, 2011. a
Ricke, K. L. and Caldeira, K.: Natural climate variability and future climate policy, Nat. Clim. Change, 4, 333–338, 2014. a, b, c
Rocha, J. C., Peterson, G., Bodin, Ö., and Levin, S.: Cascading regime shifts within and across scales, Science, 362, 1379–1383, 2018. a
Rockström, J., Gupta, J., Qin, D., Lade, S. J., Abrams, J. F., Andersen, L. S., McKay, D. I. A., Bai, X., Bala, G., Bunn, S. E., Ciobanu, D., DeClerck, F., Ebi, K., Gifford, L., Gordon, C., Hasan, S., Kanie, N., Lenton, T. M., Loriani, S., Liverman, D. M., Mohamed, A., Nakicenovic, N., Obura, D., Ospina, D., Prodani, K., Rammelt, C., Sakschewski, B., Scholtens, J., Stewart-Koster, B., Tharammal, T., van Vuuren, D., Verburg, P. H., Winkelmann, R., Zimm, C., Bennett, E. M., Bringezu, S., Broadgate, W., Green, P. A., Huang, L., Jacobson, L., Ndehedehe, C., Pedde, S., Rocha, J., Scheffer, M., Schulte-Uebbing, L., de Vries, W., Xiao, C., Xu, C., Xu, X., Zafra-Calvo, N., and Zhang, X.: Safe and just Earth system boundaries, Nature, 619, 102–111, https://doi.org/10.1038/s41586-023-06083-8, 2023. a
Schelling, T. C.: Dynamic models of segregation, J. Math. Sociol., 1, 143–186, 1971. a
Schill, C., Anderies, J. M., Lindahl, T., Folke, C., Polasky, S., Cárdenas, J. C., Crépin, A.-S., Janssen, M. A., Norberg, J., and Schlüter, M.: A more dynamic understanding of human behaviour for the Anthropocene, Nature Sustainability, 2, 1075–1082, 2019. a
Sharpe, S. and Lenton, T. M.: Upward-scaling tipping cascades to meet climate goals: plausible grounds for hope, Clim. Policy, 21, 421–433, https://doi.org/10.1080/14693062.2020.1870097, 2021. a
Singh, A. S., Zwickle, A., Bruskotter, J. T., and Wilson, R.: The perceived psychological distance of climate change impacts and its influence on support for adaptation policy, Environ. Sci. Policy, 73, 93–99, 2017. a
Singh, P., Sreenivasan, S., Szymanski, B. K., and Korniss, G.: Threshold-limited spreading in social networks with multiple initiators, Sci. Rep., 3, 1–7, 2013. a
Sisco, M. R.: The effects of weather experiences on climate change attitudes and behaviors, Curr. Opin. Env. Sust., 52, 111–117, 2021. a
Smith, E. K. and Mayer, A.: A social trap for the climate? Collective action, trust and climate change risk perception in 35 countries, Global Environ. Change, 49, 140–153, 2018. a
Smith, E. K. and Mayer, A.: Anomalous Anglophones? Contours of free market ideology, political polarization, and climate change attitudes in English-speaking countries, Western European and post-Communist states, Climatic Change, 152, 17–34, 2019. a
Smith, E. K., Eder, C., and Katsanidou, A.: On thinning ice: understanding the knowledge, concerns and behaviors towards polar ice loss in Germany, Polar Geography, 43, 243–258, https://doi.org/10.1080/1088937X.2020.1755904, 2020. a
Smith, E. K., Eder, C., Donges, J., Heitzig, J., Katsanidou, A., Wiedermann, M., and Winkelmann, R.: Domino Effects in the Earth System – The role of wanted social tipping points, OSF [preprint], https://doi.org/10.31219/osf.io/d8scb, 2022. a, b, c
Smith, S. R.: Enabling a political tipping point for rapid decarbonisation in the United Kingdom, EGUsphere [preprint], https://doi.org/10.5194/egusphere-2023-1674, 2023. a
Spence, A., Poortinga, W., and Pidgeon, N.: The Psychological Distance of Climate Change, Risk Anal., 32, 957–972, https://doi.org/10.1111/j.1539-6924.2011.01695.x, 2012. a, b
Stadelmann-Steffen, I., Eder, C., Harring, N., Spilker, G., and Katsanidou, A.: A framework for social tipping in climate change mitigation: What we can learn about social tipping dynamics from the chlorofluorocarbons phase-out, Energy Research & Social Science, 82, 102307, https://doi.org/10.1016/j.erss.2021.102307, 2021. a
State, B. and Adamic, L.: The diffusion of support in an online social movement: Evidence from the adoption of equal-sign profile pictures, in: Proceedings of the 18th ACM Conference on Computer Supported Cooperative Work & Social Computing, 1741–1750, https://doi.org/10.1145/2675133.2675290, 2015. a
Steffen, W., Rockström, J., Richardson, K., Lenton, T. M., Folke, C., Liverman, D., Summerhayes, C. P., Barnosky, A. D., Cornell, S. E., Crucifix, M., Donges, J. F., Fetzer, I., Lade, S. J., Scheffer, M., Winkelmann, R., and Schellnhuber, H. J.: Trajectories of the Earth System in the Anthropocene, P. Natl. Acad. Sci. USA, 115, 8252–8259, 2018. a
Steg, L. and Vlek, C.: Encouraging pro-environmental behaviour: An integrative review and research agenda, J. Environ. Psychol., 29, 309–317, 2009. a
Stern, P.: Toward a coherent theory of environmentally significant behavior, J. Soc. Issues, 56, 407–424, 2000. a, b
Tàbara, J. D., Frantzeskaki, N., Hölscher, K., Pedde, S., Kok, K., Lamperti, F., Christensen, J. H., Jäger, J., and Berry, P.: Positive tipping points in a rapidly warming world, Curr. Opin. Env. Sust., 31, 120–129, 2018. a, b, c, d
Tebaldi, C., Strauss, B. H., and Zervas, C. E.: Modelling sea level rise impacts on storm surges along US coasts, Environ. Res. Lett., 7, 014032, https://doi.org/10.1088/1748-9326/7/1/014032, 2012. a
Thomas, M., Pidgeon, N., Whitmarsh, L., and Ballinger, R.: Mental models of sea-level change: A mixed methods analysis on the Severn Estuary, UK, Global Environ. Change, 33, 71–82, 2015. a
Tobler, C., Visschers, V. H., and Siegrist, M.: Addressing climate change: Determinants of consumers' willingness to act and to support policy measures, J. Environ. Psychol., 32, 197–207, 2012. a
Tonn, B., Hemrick, A., and Conrad, F.: Cognitive representations of the future: Survey results, Futures, 38, 810–829, 2006. a
Traag, V. A., Quax, R., and Sloot, P. M.: Modelling the distance impedance of protest attendance, Physica A, 468, 171–182, 2017. a
Van Ginkel, K. C., Botzen, W. W., Haasnoot, M., Bachner, G., Steininger, K. W., Hinkel, J., Watkiss, P., Boere, E., Jeuken, A., De Murieta, E. S., and and Bosello, F.: Climate change induced socio-economic tipping points: review and stakeholder consultation for policy relevant research, Environ. Res. Lett., 15, 023001, https://doi.org/10.1088/1748-9326/ab6395, 2020. a
van Valkengoed, A. M. and Steg, L.: Meta-analyses of factors motivating climate change adaptation behaviour, Nat. Clim. Change, 9, 158–163, 2019. a, b
Većkalov, B., Zarzeczna, N., Niehoff, E., McPhetres, J., and Rutjens, B. T.: A matter of time… consideration of future consequences and temporal distance contribute to the ideology gap in climate change scepticism, J. Environ. Psychol., 78, 101703, https://doi.org/10.1016/j.jenvp.2021.101703, 2021. a
Watts, D. J.: A simple model of global cascades on random networks, P. Natl. Acad. Sci. USA, 99, 5766–5771, 2002. a, b, c, d
Watts, D. J. and Strogatz, S. H.: Collective dynamics of “small-world” networks, Nature, 393, 440–442, https://doi.org/10.1038/30918, 1998. a
Weible, C. and Sabatier, P. A.: Theories of the Policy Process, Westview Press, New York, https://doi.org/10.4324/9780429494284, 2017. a
Wiedermann, M., Smith, E. K., Heitzig, J., and Donges, J. F.: A network-based microfoundation of Granovetter's threshold model for social tipping, Sci. Rep., 10, 1–10, 2020. a, b, c, d, e, f
Winkelmann, R., Donges, J. F., Smith, E. K., Milkoreit, M., Eder, C., Heitzig, J., Katsanidou, A., Wiedermann, M., Wunderling, N., and Lenton, T. M.: Social tipping processes towards climate action: a conceptual framework, Ecol. Econ., 192, 107242, https://doi.org/10.1016/j.ecolecon.2021.107242, 2022. a, b, c, d, e, f, g, h
Wunderling, N., Donges, J. F., Kurths, J., and Winkelmann, R.: Interacting tipping elements increase risk of climate domino effects under global warming, Earth Syst. Dynam., 12, 601–619, https://doi.org/10.5194/esd-12-601-2021, 2021 a