the Creative Commons Attribution 4.0 License.
the Creative Commons Attribution 4.0 License.
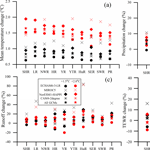
Spatial–temporal changes in runoff and terrestrial ecosystem water retention under 1.5 and 2 °C warming scenarios across China
Ran Zhai
Fulu Tao
Zhihui Xu
The Paris Agreement set a long-term temperature goal of holding the global average temperature increase to below 2.0 ∘C above pre-industrial levels, pursuing efforts to limit this to 1.5 ∘C; it is therefore important to understand the impacts of climate change under 1.5 and 2.0 ∘C warming scenarios for climate adaptation and mitigation. Here, climate scenarios from four global circulation models (GCMs) for the baseline (2006–2015), 1.5, and 2.0 ∘C warming scenarios (2106–2115) were used to drive the validated Variable Infiltration Capacity (VIC) hydrological model to investigate the impacts of global warming on runoff and terrestrial ecosystem water retention (TEWR) across China at a spatial resolution of 0.5∘. This study applied ensemble projections from multiple GCMs to provide more comprehensive and robust results. The trends in annual mean temperature, precipitation, runoff, and TEWR were analyzed at the grid and basin scale. Results showed that median change in runoff ranged from 3.61 to 13.86 %, 4.20 to 17.89 %, and median change in TEWR ranged from −0.45 to 6.71 and −3.48 to 4.40 % in the 10 main basins in China under 1.5 and 2.0 ∘C warming scenarios, respectively, across all four GCMs. The interannual variability of runoff increased notably in areas where it was projected to increase, and the interannual variability increased notably from the 1.5 to the 2.0 ∘C warming scenario. In contrast, TEWR would remain relatively stable, the median change in standard deviation (SD) of TEWR ranged from −10 to 10 % in about 90 % grids under 1.5 and 2.0 ∘C warming scenarios, across all four GCMs. Both low and high runoff would increase under the two warming scenarios in most areas across China, with high runoff increasing more. The risks of low and high runoff events would be higher under the 2.0 than under the 1.5 ∘C warming scenario in terms of both extent and intensity. Runoff was significantly positively correlated to precipitation, while increase in maximum temperature would generally cause runoff to decrease through increasing evapotranspiration. Likewise, precipitation also played a dominant role in affecting TEWR. Our results were supported by previous studies. However, there existed large uncertainties in climate scenarios from different GCMs, which led to large uncertainties in impact assessment. The differences among the four GCMs were larger than differences between the two warming scenarios. Our findings on the spatiotemporal patterns of climate impacts and their shifts from the 1.5 to the 2.0 ∘C warming scenario are useful for water resource management under different warming scenarios.
- Article
(8883 KB) - Full-text XML
-
Supplement
(7096 KB) - BibTeX
- EndNote
The global average surface temperature increased by 0.85 ∘C from 1880 to 2012, and the beginning of the 21st century has been the warmest on record (IPCC, 2013). In 2015, the Paris Agreement set a long-term temperature goal of holding the global average temperature increase to below 2.0 ∘C above pre-industrial levels, pursuing efforts to limit this to 1.5 ∘C, because the risks and impacts of climate change were thought to decrease significantly under global warming of 1.5 than 2.0 ∘C (Schleussner et al., 2016). This calls for explicitly spatial climate change impact assessment on multiple sectors under global warming of 1.5 and 2.0 ∘C. Up to now, climate impact under 1.5 and 2.0 ∘C warming scenarios has been rarely assessed but is urgently needed for climate adaptation and mitigation.
Global warming is likely to have major impacts on the hydrological cycle (Huntington, 2006; Milliman et al., 2008; Arnell and Gosling, 2013), such as changing precipitation patterns and increasing risks of extreme hydrological events (Wang et al., 2012; Zhang et al., 2016). China is vulnerable to future climate change; the impacts of climate change on water resources in China have been of key concern (Piao et al., 2010; Leng et al., 2015). Hydrological models have been routinely used to investigate the impacts of climate change on water resources, driven by climate scenarios from global circulation models (GCMs). Several previous studies had assessed climate change impacts on water resources in some river basins over China (e.g., Chen et al., 2012; Li et al., 2013; Zhang et al., 2016). For example, with the Xinanjiang model and HBV (Hydrologiska Byråns Vattenbalansavdelning) model in the Qingjiang Watershed, Chen et al. (2012) showed runoff would firstly decrease during 2011–2040 and then increase under A2 and B2 scenarios relative to the baseline period (1962–1990). Using the SIMHYD and GR4J rainfall–runoff models, driven by climate scenarios from 20 GCMs, mean runoff was projected to increase by most of the GCMs under a 1.0 ∘C increase in global average surface air temperature across the Yarlung Tsangpo River basin (Li et al., 2013). Using the Soil and Water Assessment Tool (SWAT), Zhang et al. (2016) showed that future runoff would not change much under Representative Concentration Pathways 2.6 (RCP2.6) and 4.5 (RCP4.5) scenarios but increase significantly under the RCP8.5 scenario from three different GCMs (BCC-CSM1.1, CanESM2, and NorESM1-M) in the Xin River basin of China. However, climate change impact on water resources across all of China has rarely been investigated. Using the Variable Infiltration Capacity (VIC) model, Wang et al. (2012) showed the total amount of annual runoff over China would increase by approximately 3–10 % by 2050 using the climate projections of RCM-PRECIS under A2, B2, and A1B emissions scenarios, with uneven distribution, relative to 1961–1990. Using the VIC model driven by climate scenarios from five GCMs under the RCP8.5 emission scenario, Leng et al. (2015) showed that climate change could increase water-related risks across China in the 21st century because of projected decrease in runoff and increase in interannual variability. The changes in runoff under 1.5 and 2.0 ∘C warming scenarios across China have not been investigated yet. Because of vast territory and large population, it is important to understand the explicitly spatial changes in water resources under 1.5 and 2.0 ∘C warming scenarios across China.
With the rapid growth of population and economy, ecosystem degradation and ecosystem services have increasingly become a hot topic. Terrestrial ecosystem water retention (TEWR) is one of important ecosystem services (Gong et al., 2017; Xu et al., 2017). Different methods have been used to quantify TEWR. One of popular methods is based on terrestrial ecosystem water balance; the capacity of TEWR is the difference between the amount of precipitation and the sum of runoff and evapotranspiration (Ouyang et al., 2016; Xu et al., 2017). It is of great importance to evaluate TEWR service under changing climate for ecosystem and water resource management (Tao et al., 2003).
To our knowledge, this study is the first to investigate the changes in runoff and TEWR service across China under 1.5 and 2.0 ∘C warming scenarios, as well as the differences between the two warming scenarios. The objectives of this study are (1) to investigate the characteristics of expected changes in temperature and precipitation under 1.5 and 2.0 ∘C warming scenarios; (2) to investigate the changes in runoff and TEWR across China under 1.5 and 2.0 ∘C warming scenarios at the grid scale and basin scale; and (3) to evaluate the dominant factors for changes in runoff and TEWR under warming climate.
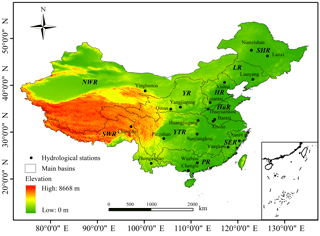
Figure 1The 10 main basins in China, as well as the 20 catchments (hydrological stations) used for calibrating and validating the VIC hydrological model (available at: http://www.hydro.washington.edu/Lettenmaier/Models/VIC/index-old.shtml, last access: 10 October 2014).
2.1 Study domain
There are 10 main basins in China (Fig. 1), including the Songhua River basin (SHR), Liao River basin (LR), northwest river basins (NWR), Hai River basin (HR), and Yellow River basin (YR) in the northern China; and the Yangtze River basin (YTR), Huai River basin (HuR), southeast river basins (SER), southwest river basins (SWR), and Pearl River basin (PR) in southern China (Leng et al., 2015; J. Y. Liu et al., 2017a). The temperature increases from north to south, and precipitation increases gradually from northwest to southeast (Xie et al., 2007). Mean annual runoff for China is around 284 mm based on synchronous runoff data for a 50-year period from 1956 to 2005 (Wang et al., 2012). However, water resource is unevenly distributed spatially and seasonally. In most areas, there is more than 70 % of total runoff in the flood season from June to October (Wang et al., 2012). Water is more abundant in southern China than in northern China (Piao et al., 2010).
2.2 Model description
A large-scale semi-distributed hydrological model, VIC, was applied in this study. We divided China into grids with three layers of soil in this study. The grids were between 18 and 54∘ N from south to north, and between 73 and 135.5∘ E from west to east. Only the grids with the whole area located within the borders of mainland China were investigated in this research. The soil and vegetation in each grid were considered in the model. Each grid cell is described by N+1 land cover tiles; it represents N different tiles of vegetation and bare soil. The total evapotranspiration includes canopy evaporation (Ec, mm) of each vegetation tile, vegetation transpiration (Et, mm) of each vegetation tile and bare soil evaporation (E1, mm); the formulation of the total evapotranspiration is
where Cn is the vegetation coverage fraction for the nth vegetation tile, CN+1 is the bare soil fraction, and in each grid. The total runoff consists of surface runoff and base flow (Wang et al., 2012); the formulation of the total runoff is
where Qs,n (mm) and Qb,n (mm) are the surface runoff and base flow for the n land cover tile, respectively (Gao et al., 2010). The VIC model uses the variable infiltration curve to account for the spatial heterogeneity of runoff generation. It assumes that surface runoff for the upper two soil layers is generated by those areas for which precipitation exceeds the storage capacity of the soil. The ARNO method is used to describe base flow, which only happens in the third layer of soil (Todini, 1996). A routing model is used to calculate runoff in each catchment after running the VIC model (Lohmann et al., 1996). More details could be found in Liang et al. (1994, 1996), Liang and Xie (2001), Xie et al. (2003), and Gao et al. (2010).
2.3 Data
Bias-corrected climate data sets for this study were from the project “Half a degree Additional warming, Prognosis and Projected Impacts” (HAPPI). It provides climate data to assess how the climate, especially extreme weather, might be different from the present in the world under 1.5 and 2.0 ∘C warmer than pre-industrial conditions (Mitchell et al., 2017). Large ensembles of simulations for three time periods have been produced after being bias corrected using the Inter-Sectoral Impact Model Intercomparison Project (ISIMIP2b) bias-correction approach (Frieler et al., 2017), from four GCMs up to now, including ECHAM6-3-LR, MIROC5, NorESM1-HAPPI, and CAM4-2degree (Table 1) (http://portal.nersc.gov/c20c/data/ClimateAnalytics/, last access: 20 October 2017). The first time period was from 2006 to 2015 which is the most recently observed 10 years; the second time period was from 2106 to 2115 under 1.5 and 2.0 ∘C warming scenarios, respectively. Each simulation within a time period was different from the others in its initial weather state (Mitchell et al., 2017). Table 1 shows the available ensemble members in each GCM under the current period from 2006 to 2015, and 1.5 and 2.0 ∘C warming scenarios from 2106 to 2115. The input data of VIC include daily precipitation, daily maximum temperature, daily minimum temperature, and daily wind speed. In addition, we analyzed annual precipitation and annual mean temperature to show the differences between GCMs.
The observation daily weather data, including daily time series of precipitation, maximum temperature, minimum temperature, and wind speed, from 1961 to 1987 and from 2006 to 2015, were obtained from the China Meteorological Administration (CMA) and used for calibrating and validating the VIC model. The meteorological data were interpolated to each grid through linear interpolation weighted by the inverse squared distances between the meteorological stations and the center of each grid cell (Xie et al., 2007). The 1 km land cover data were from the University of Maryland (http://glcfapp.glcf.umd.edu:8080/esdi/index.jsp, last access: 8 May 2016). The 1 km soil texture data (China Soil Map Based Harmonized World Soil Database, v1.1) and 1 km digital elevation model data set were obtained from the Cold and Arid Regions Sciences Data Center at Lanzhou (http://westdc.westgis.ac.cn, last access: 30 May 2016). These data were used to build the VIC model. The NASA Shuttle Radar Topographic Mission (SRTM) 90 m digital elevation data (http://srtm.csi.cgiar.org/, last access: 7 June 2017) were used to extract each catchment. Monthly runoff observation data, obtained from the hydrological year book of China and local water resources department, were used for calibrating and validating the VIC model. A detailed description was presented in Zhai and Tao (2017).
2.4 VIC model parameters' calibration and validation
Monthly runoff data from 1961 to 1979 were used to calibrate and validate the VIC model (Wang et al., 2012; J. Y. Liu et al., 2017a). Seven parameters in the VIC model needed to be calibrated because they were difficult to obtain, including the variable infiltration curve parameter (b), the maximum velocity of base flow (Dsmax), the fraction of Dsmax where non-linear base flow begins (Ds), the fraction of maximum soil moisture where non-linear base flow occurs (Ws), and the thickness of each soil moisture layer (di, i=1, 2, 3). We divided 1961–1979 into three periods, including preheating period (1961–1962), calibration period (1963–1969), and validation period (1970–1979) in each catchment. The parameters calibrated in a catchment were further validated in other catchments located in the same basin. The VIC model was run at daily time step, and the results were aggregated to monthly time steps at each catchment for calibrating and validating parameters. The relative error (BIAS) and the Nash–Sutcliffe efficiency coefficient (NSE) were used to calibrate and validate the parameters:
-
The BIAS (%) represents the error between simulated () and observed mean monthly runoff ():
-
The NSE (Nash and Sutcliffe, 1970) represents the matching degree between the simulated and observed runoff:
where Qi,o and Qi,s are the observed monthly runoff (mm) and the simulated monthly runoff (mm) at month i, and is the mean observed monthly runoff (mm). A good simulation result will have NSE close to 1 and BIAS approaching 0.
2.5 Quantification of TEWR service
In this study, considering the input water and output water of a certain grid, we adopted the following equation to calculate the total amount of TEWR capacity (Ouyang et al., 2016; Xu et al., 2017):
where W represents TEWR (mm), P represents precipitation (mm), ET represents evapotranspiration (mm), and R represents runoff (mm).
2.6 Analysis
The climate scenarios from the GCMs of ECHAM6-3-LR, MIROC5, NorESM1-HAPPI, and CAM4-2degree were used as input to drive the VIC hydrological model. Each GCM had three climate change scenarios as output: baseline period from 2006 to 2015, 1.5 ∘C warming scenario from 2106 to 2115, and 2.0 ∘C warming scenario from 2106 to 2115. For each GCM of ECHAM6-3-LR, NorESM1-HAPPI, and CAM4-2degree, we had 200 simulations (10 years × 20 ensembles) for the baseline period, 1.5, and 2.0 ∘C warming scenarios, respectively. For the GCM of MIROC5, we had 100 simulations (10 years × 10 ensembles) for the baseline period, 1.5, and 2.0 ∘C warming scenarios, respectively. Changes in annual mean temperature and precipitation were calculated using each ensemble for the future 10-year period (2106–2115) under 1.5 and 2.0 ∘C warming scenarios relative to the corresponding ensemble for the baseline period (2006–2015). Changes in annual mean and SD (standard deviation) as a measure of interannual variability were used to analyze the impacts of climate change on runoff and TEWR across China. We computed the changes in annual mean and SD of runoff and TEWR as the relative differences between the simulations using each ensemble for the future 10-year period (2106–2115) under 1.5 and 2.0 ∘C warming scenarios relative to the simulations using the corresponding ensemble for the baseline period (2006–2015). For each warming scenario in each GCM, we adopted the median value of the changes among ensembles, which should be the most likely result avoiding abnormal value (Tao and Zhang, 2011). We calculated the median value of annual mean temperature change, precipitation change, runoff change, TEWR change, and the median value of SD change of runoff and TEWR among all 70 ensembles under the four GCMs of each grid. Then, we calculated probability density functions of runoff change and TEWR change through the median value from all 70 ensembles in every grid in the 10 main basins across China under 1.5 and 2.0 ∘C warming scenarios (2106–2115) relative to the baseline period (2006–2015). The basin mean was calculated by averaging the values for the individual grid cells within the basin for each ensemble of a GCM.
Two hydrological indicators, Q90 (low runoff), which refers to the magnitude of runoff that is exceeded in 90 % of the time, and Q10 (high runoff), which refers to the magnitude of runoff that is exceeded in 10 % of the time, were used to evaluate the risks of hydrological extremes. We used all ensemble simulations in the baseline period in 2006–2015, in 2106–2115 under the 1.5 ∘C warming scenario, and in 2106–2115 under the 2.0 ∘C warming scenario to evaluate the changes in low runoff and high runoff. Therefore, there were 700 years of data (3 GCMs × 20 ensembles × 10 years + 1 GCM × 10 ensembles × 10 years = 700) for the baseline period in 2006–2015, 1.5 ∘C warming scenario in 2106–2115, and 2.0 ∘C warming scenario in 2106–2115, respectively.
The Pearson correlation coefficient (r) was used to analyze the dominant factors affecting runoff and TEWR:
where n represents sample size, including four GCMs, two warming scenarios relative to the baseline in each GCM, 20 or 10 ensembles in each warming scenario, and 10 years in each ensemble; thus, there were 1400 samples (3 GCMs × 2 warming scenarios × 20 ensembles × 10 years + 1 GCM × 2 warming scenarios × 10 ensembles × 10 years = 1400) in each grid. xi and yi are variable change values under 1.5 and 2.0 ∘C warming scenarios (2106–2115) relative to the baseline period (2006–2015) in each data set, and , are the mean change values of each variable in each grid.
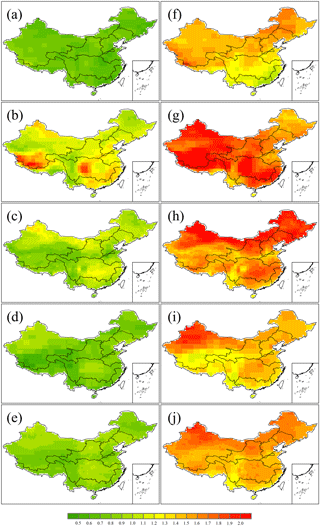
Figure 2Median values of the projected changes in annual mean temperature (∘C) in China under the 1.5 ∘C (a–e) and 2.0 ∘C (f–j) warming scenarios by ECHAM6-3-LR (a, f), MIROC5 (b, g), NorESM1-HAPPI (c, h), CAM4-2degree (d, i), and all four GCMs (e, j), relative to 2006–2015.
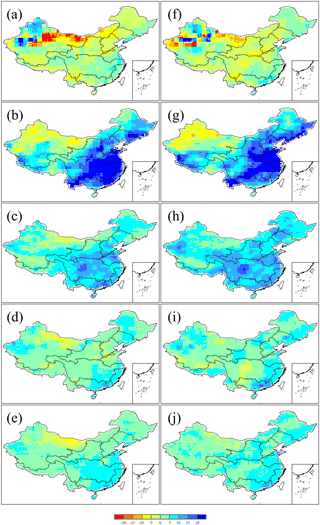
Figure 3Median values of the projected changes in annual precipitation (%) in China under the 1.5 ∘C (a–e) and 2.0 ∘C (f–j) warming scenarios by ECHAM6-3-LR (a, f), MIROC5 (b, g), NorESM1-HAPPI (c, h), CAM4-2degree (d, i), and all four GCMs (e, j), relative to 2006–2015.
Table 2Information on the 10 catchments (hydrological stations) used for calibrating and validating parameters and the performance of the VIC model for monthly runoff simulation in each catchment.
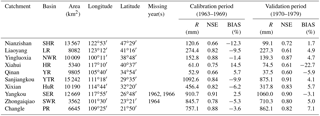
R represents annual mean runoff of each station in the calibration period or validation period.
3.1 VIC model parameters' calibration and validation
The VIC model was calibrated and validated in 10 catchments located in different main basins in China (Figs. 1 and S1 in the Supplement). Then, the calibrated parameters were applied in all the grids located in the same basin. The NSE values of monthly runoff were above 0.70 in eight catchments in the calibration period, while the NSE values were above 0.70 in seven catchments in the validation period (Table 2). Except the Xiahui catchment, the BIAS values in all catchments were between −15 and 15 %, which indicated the model-simulated monthly runoff fairly well. Generally, the VIC model performed better in catchments located in southern China where there was more precipitation and runoff compared with catchments located in northern China. The NSE values for the Sanjiangkou, Xixian, Yangkou, Zhongaiqiao, and Changle catchments in southern China were all more than 0.75, and the BIAS values were between −10 and 10 %. To make our parameter transplantation more convincing, we validated the calibrated parameters in 10 other catchments different from those used for parameters calibration. Catchment areas ranged from 11 280 to 730 036 km2. Generally, results showed that the parameters calibrated in a catchment were also validated for other catchments located in the same basin; the NSE values for these 10 catchments were all larger than 0.65, and except for the Bantai catchment, the BIAS values were all between −20 and 20 % (Table 3).
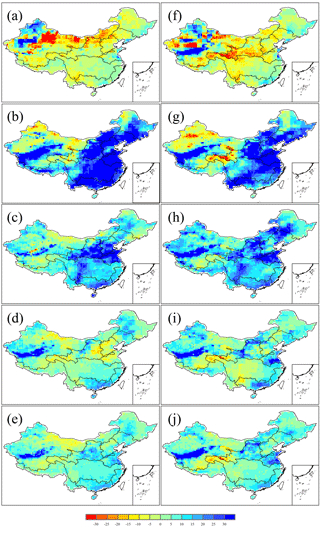
Figure 4Median values of the projected changes in annual runoff (%) in China under the 1.5 ∘C (a–e) and 2.0 ∘C (f–j) warming scenarios by ECHAM6-3-LR (a, f), MIROC5 (b, g), NorESM1-HAPPI (c, h), CAM4-2degree (d, i), and all four GCMs (e, j), relative to 2006–2015.
3.2 Climate change across China under 1.5 and 2.0 ∘C warming scenarios
The median values of the changes in annual mean temperature and annual precipitation under the two warming scenarios for each GCM and all four GCMs were shown in Figs. 2 and 3, respectively. Generally, ECHAM6-3-LR and CAM4-2degree projected a relatively small increase in annual mean temperature (Fig. 2a, d, f, i). MIROC5 projected a relatively large increase in annual mean temperature in comparison with other GCMs (Fig. 2b, g). As for annual precipitation, ECHAM6-3-LR projected a decrease in precipitation over large areas across China under the 1.5 ∘C warming scenario, and the decreasing trends reduced in northeastern China and northwestern China, and increased in the Yellow River basin, Huai River basin, Yangtze River basin, and southwest river basins under the 2.0 ∘C warming scenario (Fig. 3a, f). In contrast, MIROC5, NorESM1-HAPPI, and CAM4-2degree projected an increase in precipitation over large areas across China under both the 1.5 and 2.0 ∘C warming scenarios. In particular, MIROC5 projected the largest increase in precipitation by more than 20 % in large areas in southern China (Fig. 3b, g). Nearly all of the four GCMs projected that precipitation decreased more (or increased less) in most areas located in northwestern China than other areas in China (Fig. 3). In contrast, precipitation was projected to increase more (or decrease less) in southeastern China (Fig. 3). There were large differences among the projections by the four different GCMs, suggesting a large uncertainty from climate change projection. When taking all 70 ensembles of the four GCMs as a whole, annual mean temperature was projected to increase more in northern China and in the middle and lower reaches of the Yangtze River basin than in other areas (Fig. 2e, j). In addition, annual precipitation was projected to increase more in southeastern China under the 1.5 ∘C warming scenario, and the increasing trend was projected to narrow down in the Yangtze River basin and extend to some areas located in northern China under the 2.0 ∘C warming scenario (Fig. 3e, j).
3.3 Changes in runoff across China under 1.5 and 2.0 ∘C warming scenarios
There were significant differences in projected change in runoff using the VIC model driven by the four different GCMs (Fig. 4). The projected runoff pattern was consistent with that of precipitation generally, suggesting precipitation change played a dominant role in runoff change. For example, under 1.5 and 2.0 ∘C warming scenarios by ECHAM6-3-LR, runoff was projected to decrease in most areas across China (Fig. 4a, f) due to the projected decrease in precipitation (Fig. 3a, f). In contrast, using the climate scenarios by MIROC5, runoff was projected to increase most (Fig. 4b, g) due to the projected increase in precipitation (Fig. 3b, g). In addition, increase in temperature would lead to increase in evapotranspiration generally (Fig. S2), which resulted in decrease in runoff. For example, under the 1.5 ∘C warming scenario by CAM4-2degree, precipitation would increase in most areas but the magnitude of increase was small; runoff was projected to decrease in large areas in the Hai River basin, Yellow River basin, Huai River basin, and the source regions of the Yellow River basin and Yangtze River basin (Fig. 4d). Using the climate scenarios by MIROC5 and NorESM1-HAPPI, runoff was projected to increase in most areas across China (Fig. 4b, c, g, h), suggesting the positive effects of precipitation increase should exceed the negative effects of temperature increase. For the median change across all 70 ensembles in the four GCMs, runoff was projected to increase in large areas in China, especially in the Yellow River basin, Huai River basin, and Pearl River basin (Fig. 4e, j). In contrast, runoff was projected to decrease obviously in areas located in the northwest river basins under the 1.5 ∘C warming scenario and the source regions of the Yellow River basin and the Yangtze River basin under the 2.0 ∘C warming scenario (Fig. 4e, j).
For each GCM, the median changes in SD of runoff among the ensembles under 1.5 and 2.0 ∘C warming scenarios were presented (Fig. 5). The SD was projected to increase notably in areas where the annual runoff increased notably, for all four GCMs. Furthermore, the SD of the simulated runoff increased more under the 2.0 ∘C warming scenario than that under the 1.5 ∘C warming scenario generally (Fig. 5e, j), suggesting that interannual variation of runoff would increase with climate warming.
3.4 Changes in low runoff and high runoff across China under 1.5 and 2.0 ∘C warming scenarios
Both low runoff (Q90) and high runoff (Q10) were projected to increase in large areas across China, and decrease in some areas in the northwest river basins and the source regions of the Yellow River basin and the Yangtze River basin (Fig. 6). High runoff was expected to increase more (or decrease less) than low runoff in most areas (Fig. 6). In some areas in the northwest river basins, Songhua River basin, and the source regions of the Yellow River basin and the Yangtze River basin, low runoff was projected to decrease under both the 1.5 and 2.0 ∘C warming scenarios (Fig. 6a, b). The areas with low runoff decreasing were projected to enlarge under the 2.0 ∘C warming scenario (Fig. 6a, b) around the source regions of the Yellow River basin and the Yangtze River basin, suggesting much more drought risks under the 2.0 ∘C warming scenario than under the 1.5 ∘C warming scenario. Additionally, the low runoff increased less under the 2.0 ∘C warming scenario than under the 1.5 ∘C warming scenario in most grids in China (Fig. 6a, b). The areas with high runoff increasing were projected to enlarge under the 2.0 ∘C warming scenario than under the 1.5 ∘C warming scenario (Fig. 6c, d), because the SD of annual precipitation was projected to increase more under the 2.0 ∘C warming scenario than under the 1.5 ∘C warming scenario in most areas across China (Fig. S3). The intensity of high runoff was also expected to increase under the 2.0 ∘C warming scenario than under the 1.5 ∘C warming scenario in most areas across China, especially in the Huai River basin (Fig. 6c, d), suggesting flood risks would increase under the 2.0 ∘C warming scenario. In contrast, high runoff in some areas in the source regions of the Yellow River basin and the Yangtze River basin was expected to decrease, and decreased more under the 2.0 ∘C warming scenario than under the 1.5 ∘C warming scenario (Fig. 6c, d), which was caused by increasing evapotranspiration. Generally, high runoff was expected to increase more than low runoff in most areas across China, and the risks of high runoff and low runoff were expected to increase under the 2.0 ∘C warming scenario than under the 1.5 ∘C warming scenario.
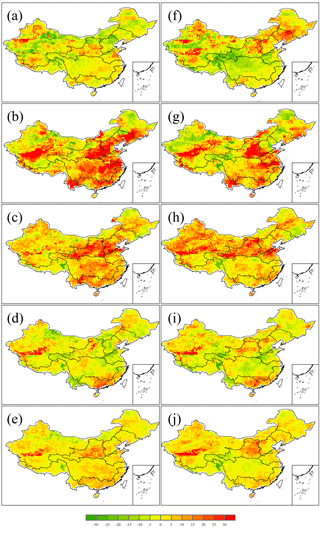
Figure 8Median values of the changes in SD of TEWR (%) in China under 1.5 ∘C (a–e) and 2.0 ∘C (f–j) warming scenarios from ECHAM6-3-LR (a, f), MIROC5 (b, g), NorESM1-HAPPI (c, h), CAM4-2degree (d, i), and all four GCMs (e, j), relative to 2006–2015.
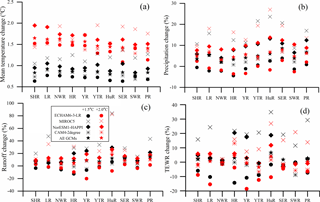
Figure 9Median values of changes in annual mean temperature (∘C) (a), annual precipitation (%) (b), annual runoff (%) (c), and annual TEWR (%) (d) in all corresponding ensembles of the four GCMs during 2106–2115 under 1.5 and 2.0 ∘C warming scenarios relative to the baseline period (2006–2015), respectively, in the 10 main basins across China.
3.5 Changes in TEWR across China under 1.5 and 2.0 ∘C warming scenarios
Changes in TEWR were consistent with changes in precipitation and runoff. With the climate scenarios by ECHAM6-3-LR, TEWR was projected to decrease in large areas in China under both the 1.5 and 2.0 ∘C warming scenarios (Fig. 7a, f), mainly due to the projected decrease in precipitation. In addition, precipitation was not the only factor for changes in TEWR. For example, precipitation was projected to increase in the source regions of the Yellow River basin and Yangtze River basin under warming scenarios by MIROC5 (Fig. 3b, g), but TEWR there was projected to decrease (Fig. 7b, g) due to increasing evapotranspiration (Fig. S2b, g). Based on the median value of all 70 ensembles from the four GCMs, TEWR was projected to be more stable than runoff (Figs. 4e, j, 7e, j); projected changes for most grids would range from −5 to 5 %. Contrary to runoff, TEWR was projected to decrease under the 2.0 ∘C warming scenario rather than under the 1.5 ∘C warming scenario relative to the baseline period in most grids (Figs. 4e, j, 7e, j).
As runoff, the SD of TEWR was projected to increase notably in areas where the TEWR increased notably for all four GCMs generally (Fig. 8). However, the SD was projected to increase in some areas where TEWR decreased, such as the Liao River basin under the 2.0 ∘C warming scenario by ECHAM6-3-LR (Figs. 7f, 8f). As for the median SD value of the 70 ensembles, it was projected to increase more across China under 1.5 ∘C (Fig. 8e) than under the 2.0 ∘C warming scenario (Fig. 8j), suggesting the interannual variability of TEWR would be larger under the 1.5 ∘C warming scenario than under the 2.0 ∘C warming scenario in most grids. The changes in SD of TEWR were not as significant as that of runoff. The median changes in SD of TEWR ranged from −10 to 10 % in about 90 % grids under 1.5 and 2.0 ∘C warming scenarios, respectively, across all four GCMs (Fig. 8e, j). In addition, the differences among the 10 main basins were not as significant as runoff.
Table 4Median values of changes in annual mean temperature (∘C), annual precipitation (%), annual runoff (%), and annual TEWR (%) in all corresponding ensembles of the four GCMs over 2106–2115 under 1.5 and 2.0 ∘C warming scenarios relative to baseline period (2006–2015), respectively, in the 10 main basins across China.
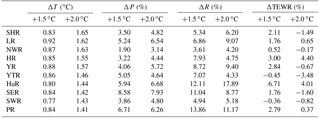
ΔT, ΔP, ΔR, and ΔTEWR represent annual mean temperature change, annual precipitation change, annual runoff change and annual TEWR change.
4.1 Differences in climate variables and water resources between 1.5 and 2.0 ∘C warming scenarios by each GCM at the basin scale
To evaluate climate change and its potential impact on runoff and TEWR at the basin scale, the median values of the annual mean temperature change, annual precipitation change, annual runoff change, and annual TEWR change for all 10 basins were summarized (Table 4 and Fig. 9) across all 70 ensembles from the four GCMs. The uncertainties of all 70 ensembles in each main basin of annual mean temperature change, annual precipitation change, annual runoff change, and annual TEWR change were shown in Fig. S4. In addition, the median changes in low runoff (Q90) and high runoff (Q10) in each main basin in China from each GCM were shown in Fig. S5. At the basin scale, annual mean temperature increased more in northern China (the median value ranged from 0.83 to 0.92 ∘C under the 1.5 ∘C warming scenario, and ranged from 1.55 to 1.65 ∘C under the 2.0 ∘C warming scenario) than in southern China (the median value ranged from 0.77 to 0.86 ∘C under the 1.5 ∘C warming scenario, and ranged from 1.41 to 1.46 ∘C under the 2.0 ∘C warming scenario), and the differences between northern China and southern China grew larger under the 2.0 than under the 1.5 ∘C warming scenario (Fig. 9a), while annual precipitation increased more in southern China (the median value ranged from 3.86 to 8.58 % under the 1.5 ∘C warming scenario, and ranged from 4.64 to 7.93 % under the 2.0 ∘C warming scenario) than in northern China (the median value ranged from 1.90 to 5.24 % under the 1.5 ∘C warming scenario, and ranged from 3.14 to 6.54 % under the 2.0 ∘C warming scenario) (Fig. 9b). Generally, both runoff and TEWR would change consistently with precipitation (Fig. 9b, c, d). Annual mean temperature increased less under the climate scenarios by ECHAM6-3-LR than the other three GCMs in most basins. Annual precipitation was projected to decrease slightly in most basins under the climate scenarios by ECHAM6-3-LR. However, precipitation was projected to increase in all basins under the climate scenarios by the other three GCMs (Fig. 9b). Runoff was projected to increase under the climate scenarios by the four GCMs, except some basins under the climate scenarios by ECHAM6-3-LR and CAM4-2degree (Fig. 9c). According to the median value across all ensembles in the four GCMs, runoff was projected to increase in all basins (the median value ranged from 3.61 to 13.86 % under the 1.5 ∘C warming scenario, and ranged from 4.20 to 17.89 % under the 2.0 ∘C warming scenario), but TEWR was projected to decrease or increase less than runoff (Fig. 9c, d) (the median value ranged from −0.45 to 6.71 % under the 1.5 ∘C warming scenario, and ranged from −3.48 to 4.40 % under the 2.0 ∘C warming scenario). In addition, our results showed that the differences of runoff and TEWR between GCMs were larger than those between warming scenarios by a certain GCM. This finding was supported by many other research studies (Chen et al., 2011; Ouyang et al., 2015). In addition, we found that the changes in runoff and TEWR were more pronounced than those of precipitation. Precipitation changes ranged from −4.42 to 27.02 %; however, projected changes in runoff and TEWR would range from −20.12 to 84.02 % and from −18.57 to 34.84 % of each GCM in every basin, respectively.
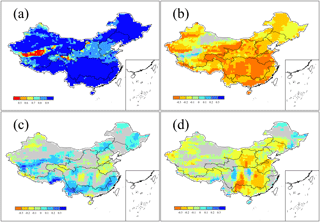
Figure 10Spatial patterns of the Pearson correlation coefficient (r) between data series of runoff changes and four key impact factors changes (a: runoff and precipitation, b: runoff and maximum temperature, c: runoff and minimum temperature, and d: runoff and wind speed) under 1.5 and 2.0 ∘C warming scenarios during 2106–2115, relative to the baseline period (2006–2015). Only grids with significant correlation (p<0.05) were shown.
The variations of runoff under different GCMs and warming scenarios were larger in the Huai River basin than in other basins (Fig. S4) and extremely large under the climate scenario by MIROC5 (Fig. 9c), suggesting there could be larger uncertainty in the Huai River basin than in other basins. The projected median annual mean precipitation and median annual mean runoff were, respectively, about 919 and 204 mm during 2006 to 2015 by MIROC5 in the Huai River basin. However, precipitation and temperature increase did not lead to a significant increase in evapotranspiration (Fig. S2b, g); more than 20 % increase in precipitation would lead to a large percentage increase in runoff because the base value in the baseline period was small.
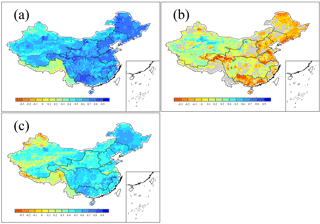
Figure 11Spatial patterns of the Pearson correlation coefficient (r) between data series of TEWR changes and three key impact factors changes (a: TEWR and precipitation; b: TEWR and evapotranspiration; c: TEWR and runoff) under 1.5 and 2.0 ∘C warming scenarios during 2106–2115, relative to the baseline period (2006–2015). Only grids with significant correlation (p<0.05) were shown.
Probability density functions of median changes of runoff and TEWR across all ensembles in the four GCMs for all the grids in each basin were presented in Figs. S6 and S7, respectively. Runoff was projected to increase with higher probability under the 2.0 than under the 1.5 ∘C warming scenario in most basins across China (Fig. S6), because precipitation was projected to increase more under the 2.0 than under the 1.5 ∘C warming scenario in most basins across China (Fig. 9b and Table 4). TEWR was projected to change less than runoff under both the 1.5 and 2.0 ∘C warming scenarios (2106–2115) compared to the baseline period (2006–2015). TEWR change was projected to decrease with higher probability under the 2.0 than under the 1.5 ∘C warming scenario compared with baseline period in most basins across China (Fig. S7). Probability density functions of changes of low runoff and high runoff under 1.5 and 2.0 ∘C warming scenarios across all ensembles of the four GCMs for all the grids in each basin were presented in Figs. S8 and S9, respectively. Most grids under the two warming scenarios showed increasing low runoff and high runoff across China. High runoff was projected to increase more in most basins across China than low runoff under the 2.0 ∘C warming scenario than under the 1.5 ∘C warming scenario (Figs. S8 and S9). In addition, this, consistent with the increase in SD of runoff under the 2.0 ∘C warming scenario (Fig. 5), may imply more flood and drought risks under the 2.0 ∘C warming scenario than under the 1.5 ∘C warming scenario.
4.2 Major factors controlling changes in runoff and TEWR
The VIC model has four input climate variables, including precipitation, maximum temperature, minimum temperature, and wind speed; the Pearson correlation coefficient was calculated between the projected changes in annual runoff and the changes in climate variables, including annual precipitation (Fig. 10a), annual mean maximum temperature (Fig. 10b), annual mean minimum temperature (Fig. 10c), and annual mean wind speed (Fig. 10d). Only significant correlations were shown (p<0.05). Generally, the correlations between precipitation change and runoff change were much more significant than the other three variables in China (Fig. 10). This finding was supported by some previous studies (Dan et al., 2012; Wang et al., 2016; Huang et al., 2016; J. Y. Liu et al., 2017a). The correlations were smaller in the Yellow River basin, Hai River basin, and Huai River basin than in other basins – even less than 0.5 for some grids in the northwest river basins and the source regions of the Yellow River basin (Fig. 10a). This may be caused by complex topography and land type, as well as the arid conditions, which prevented the amount of water needed to form runoff (D. Liu et al., 2017). There were significant negative correlations between annual runoff change and annual mean maximum temperature change in most areas across China. Increasing annual maximum temperature would lead to runoff decrease in most areas because of increasing evapotranspiration (Huang et al., 2016), especially in southern China (Fig. 10b). The correlations between annual runoff change and annual mean minimum temperature change were not significant in nearly half of the studied grids in China, which were negative in most areas in the Hai River basin, Huai River basin, the source regions of the Yellow River basin and Yangtze River basin, and some areas in the Yellow River basin, Yangtze River basin, Pearl River basin, southwest river basins, and northwest river basins, and positive at other areas. Increase in annual mean minimum temperature would increase melting of snow or ice in the Tibetan Plateau and high-latitude areas with cold weather regime, resulting in an increase in water supply to runoff (D. Liu et al., 2017). So correlations between precipitation and runoff were smaller in some areas in the Tibetan Plateau. Since increase in minimum temperature was accompanied by an increase in precipitation in most of the climate scenarios, there were positive correlations between minimum temperature change and runoff change in some areas. However, in other areas (e.g., Huai River basin, Hai River basin), increase in minimum temperature change would lead to decrease in runoff change, mainly caused by increasing evapotranspiration. There were significantly negative correlations between runoff change and wind speed change in most areas. Decrease in wind speed would lead to less evapotranspiration (She et al., 2017) and consequently more runoff.
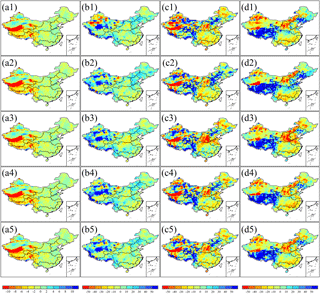
Figure 12Differences between the HAPPI data and the observed data including annual mean temperature (∘C) (a1–a5) and annual precipitation (%) (b1–b5), as well as the differences between the projected annual runoff (%) (c1–c5) and annual TEWR (%) (d1–d5) by the HAPPI data and by the observed data, for 2006–2015, based on ECHAM6-3-LR (a1, b1, c1, d1), MIROC5 (a2, b2, c2, d2), NorESM1-HAPPI (a3, b3, c3, d3), CAM4-2degree (a4, b4, c4, d4), and all four GCMs (a5, b5, c5, d5), respectively.
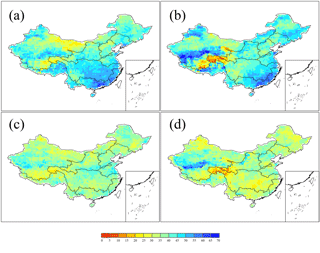
Figure 13Ensemble numbers out of 70 ensembles showing an increase in runoff (a, b) and TEWR (c, d) change under 1.5 ∘C (a, c) and 2.0 ∘C warming scenarios (b, d).
The TEWR was calculated through three variables, including precipitation, evapotranspiration, and runoff; we analyzed the correlations between TEWR and the three variables. Like runoff, the correlation coefficients were also more significant between annual TEWR change and annual precipitation change (Fig. 11a), which suggested that increase in precipitation change would lead to increase in TEWR change but not as significantly as the correlations between precipitation change and runoff change (Fig. 10a). The Pearson correlation coefficients were smaller in the southwest river basins than those in other basins. The correlations were nearly the same but smaller between TEWR change and runoff change than those between TEWR change and precipitation change (Fig. 11a, c), because runoff change and precipitation change had a strong correlation (Fig. 10a). There were negative correlations between TEWR change and evapotranspiration change in the Songhua River basin, Liao River basin, Hai River basin, Huai River basin, southeast river basins, Pearl River basin, and the source regions of the Yellow River basin and Yangtze River basin (Fig. 11b); increase in evapotranspiration change with increasing temperature change would lead to decrease in TEWR change. However, increase in evapotranspiration with increasing temperature would usually accompany increasing precipitation, which led to a positive correlation between the evapotranspiration change and TEWR change in some basins.
4.3 Uncertainty analysis
Although previous research has not investigated the changes in runoff under 1.5 and 2.0 ∘C warming scenarios, runoff changes under the RCP2.6 scenario were assessed. Since the end-of-century anthropogenic radiative forcing conditions used for 1.5 ∘C warming scenario are the same as those for the RCP2.6 scenario, the warming levels of the two scenarios are comparable (Mitchell et al., 2017). Our findings are supported by previous studies using the RCP2.6 scenario. For example, under the RCP2.6 scenario, the ensemble-averaged precipitation was expected to increase throughout China between 2015 and 2099 by 0.48 % per decade relative to 1986–2005 (J. Liu et al., 2017) among seven GCMs from the Coupled Model Intercomparison Project phase 5 (CMIP5). Precipitation would increase across most regions in China under RCP2.6 among 12 GCMs in 2070–2099 compared with 1960–1979, which is the main reason for runoff change in China (J. Y. Liu et al., 2017b). Runoff was projected to decrease in the source regions of the Yellow River basin under the RCP2.6 scenario among 12 GCMs in 2070–2099 compared with 1960–1979 (Zhang et al., 2017).
The HAPPI annual mean temperature and precipitation data were compared with the observed data, and the runoff and TEWR results driven by the HAPPI data and observation data were also compared in the baseline period (2006–2015) (Fig. 12). Median values from all ensembles in every GCM and all 70 ensembles of annual mean temperature, annual precipitation, annual runoff, and annual TEWR were used to represent HAPPI data in each grid. The differences between projected and observed temperature were generally between −2 and 2 ∘C in around 65 % of the areas in China; nevertheless, the grids located in western China showed large differences between HAPPI data and observed data (Fig. 12a1–a5), because the number of meteorological stations was sparse in western China compared to other areas. The differences in annual precipitation between the HAPPI data and the observed data ranged from −20 to 20 % in more than 75 % areas in China. The differences were smaller in southern China than those in northern China and western China (Fig. 12b1–b5). The differences between the projected runoff and TEWR using the HAPPI data and observed data ranged from −20 to 20 % in about 50 % of the grids (Fig. 12c1–c5, d1–d5).
The ensemble numbers from the 70 ensembles of the four GCMs showing an increase trend in annual runoff and annual TEWR under 1.5 and 2.0 ∘C warming scenarios were presented in Fig. 13. Runoff showed a consistent increase trend in most areas, especially in southern China (Fig. 13a, b); however, a consistent decrease trend existed in the source regions of the Yellow River basin and Yangtze River basin under the 2.0 ∘C warming scenario (Fig. 13b). Unlike runoff, TEWR was projected to change inconsistently in most areas (Fig. 13c, d). The projected changes in runoff and TEWR had large uncertainties due to uncertainties in GCMs. It is hard to determine which GCM is better than others. Therefore, this study applied ensemble projections from multiple GCMs to provide more comprehensive and robust results.
Human activities also have unavoidable impacts on water resources; more and more evidence showed that the influence of human activities on water resources is significantly enhanced (Jiang and Wang, 2016; Yuan et al., 2016; J. Y. Liu et al., 2017a; Zhai and Tao, 2017). Human activities such as land use/cover changes and the increase in water withdrawal will affect runoff in the future, which is not taken into account in this study, because changing catchment characteristics may also generate larger uncertainties in simulation (J. Y. Liu et al., 2017b). Although increase in runoff was projected in most areas across China, the runoff may experience a decrease trend with the influence of human activities such as water withdrawal for life, industry, and agriculture. Therefore, the impacts of human activities should be elaborated on in further studies.
The validated VIC model was applied to simulate future hydrological processes driven by climate scenarios by four GCMs. In general, annual mean temperature increased more in northern China than in southern China. At the basin scale, the median value of annual mean temperature change increased from 0.83 to 0.92 ∘C and from 1.55 to 1.65 ∘C in northern China; it increased from 0.77 to 0.86 ∘C and from 1.41 to 1.46 ∘C in southern China under 1.5 and 2.0 ∘C warming scenarios, respectively. On the contrary, annual precipitation increased more in southern China than northern China. At the basin scale, the median value of annual precipitation change ranged from 1.90 to 5.24 % and from 3.14 to 6.54 % in northern China; it ranged from 3.86 to 8.58 % and from 4.64 to 7.93 % in southern China under 1.5 and 2.0 ∘C warming scenarios, respectively. The projected changes in runoff and TEWR were generally consistent with the projected changes in precipitation, which were different for different GCMs under 1.5 and 2.0 ∘C warming scenarios. Annual runoff was projected to increase in most areas in China using climate scenarios by most of the four GCMs. The interannual variations of runoff were projected to increase notably in areas where annual runoff increased notably, leading to more extreme risks. The interannual variations would enlarge under the 2.0 ∘C warming scenario compared with the 1.5 ∘C warming scenario. Furthermore, the high runoff increased more than the low runoff generally, and the risks of high runoff and low runoff would be enlarged under the 2.0 ∘C warming scenario in comparison with the 1.5 ∘C warming scenario. Annual TEWR was projected to change less than annual runoff. The interannual variations of TEWR were more stable than those of runoff. At the basin scale, the median value of annual runoff change ranged from 3.61 to 13.86 % and from 4.20 to 17.89 % under 1.5 and 2.0 ∘C warming scenarios. Additionally, the median value of annual TEWR change ranged from −0.45 to 6.71 % and from −3.48 to 4.40 % under 1.5 and 2.0 ∘C warming scenarios. Multi-ensemble simulation results showed that precipitation change was the dominant factor for changes in runoff and TEWR. Maximum temperature had a negative correlation with runoff in most areas across China because it would increase evapotranspiration. A large uncertainty originated from different GCMs, so in this research we used a large ensemble simulation to provide more comprehensive and convincing results. The changing trends of runoff were consistent in most grids among the 70 ensembles. In addition, our results were also supported by previous studies which used the RCP2.6 data. The impacts of human activities should be elaborated on in further studies.
All the data are available upon request. Please contact Fulu Tao at taofl@igsnrr.ac.cn.
The supplement related to this article is available online at: https://doi.org/10.5194/esd-9-717-2018-supplement.
The authors declare that they have no conflict of interest.
This article is part of the special issue “The Earth system at a global warming of 1.5 ∘C and 2.0 ∘C”. It is not associated with a conference.
This work was supported by the National Key Research and Development Program
of China (no. 2017YFA0604703) and the National Science Foundation of China
(nos. 41571088, 41571493, and 31561143003). We acknowledge the HAPPI core team
and NERSC for data storage.
Edited by: Ning Zeng
Reviewed by: two anonymous referees
Arnell, N. W. and Gosling, S. N.: The impacts of climate change on river flow regimes at the global scale, J. Hydrol., 486, 351–364, https://doi.org/10.1016/j.jhydrol.2013.02.010, 2013.
Chen, H., Xiang, T. T., Zhou, X., and Xu, C. Y.: Impacts of climate change on the Qingjiang Watershed's runoff change trend in China, Stoch. Env. Res. Risk A., 26, 847–858, https://doi.org/10.1007/s00477-011-0524-2, 2012.
Chen, J., Brissette, F. P., Poulin, A., and Leconte, R.: Overall uncertainty study of the hydrological impacts of climate change for a Canadian watershed, Water Resour. Res., 47, https://doi.org/10.1029/2011wr010602, 2011.
Dan, L., Ji, J. J., Xie, Z. H., Chen, F., Wen, G., and Richey, J. E.: Hydrological projections of climate change scenarios over the 3H region of China: A VIC model assessment, J. Geophys. Res.-Atmos., 117, D11102, https://doi.org/10.1029/2011jd017131, 2012.
Frieler, K., Lange, S., Piontek, F., Reyer, C. P. O., Schewe, J., Warszawski, L., Zhao, F., Chini, L., Denvil, S., Emanuel, K., Geiger, T., Halladay, K., Hurtt, G., Mengel, M., Murakami, D., Ostberg, S., Popp, A., Riva, R., Stevanovic, M., Suzuki, T., Volkholz, J., Burke, E., Ciais, P., Ebi, K., Eddy, T. D., Elliott, J., Galbraith, E., Gosling, S. N., Hattermann, F., Hickler, T., Hinkel, J., Hof, C., Huber, V., J~́agermeyr, J., Krysanova, V., Marcé, R., Müller Schmied, H., Mouratiadou, I., Pierson, D., Tittensor, D. P., Vautard, R., van Vliet, M., Biber, M. F., Betts, R. A., Bodirsky, B. L., Deryng, D., Frolking, S., Jones, C. D., Lotze, H. K., Lotze-Campen, H., Sahajpal, R., Thonicke, K., Tian, H., and Yamagata, Y.: Assessing the impacts of 1.5 ∘C global warming – simulation protocol of the Inter-Sectoral Impact Model Intercomparison Project (ISIMIP2b), Geosci. Model Dev., 10, 4321–4345, https://doi.org/10.5194/gmd-10-4321-2017, 2017.
Gao, H., Tang, Q., Shi, X., Zhu, C., Bohn, T., Su, F., Sheffield, J., Pan, M., Lettenmaier, D., and Wood, E.: Water Budget Record from Variable Infiltration Capacity (VIC) Model, Algorithm Theoretical Basis Document for Terrestrial Water Cycle Data Records, Dept. of Civil and Environmental Engineering, University of Washington, Seattle, WA, 120–173, 2010.
Gong, S. H., Xiao, Y., Xiao, Y., Zhang, L., and Ouyang, Z. Y.: Driving forces and their effects on water conservation services in forest ecosystems in China, Chinese Geogr. Sci., 27, 216–228, https://doi.org/10.1007/s11769-017-0860-3, 2017.
Huang, Z., Yang, H., and Yang, D.: Dominant climatic factors driving annual runoff changes at the catchment scale across China, Hydrol. Earth Syst. Sci., 20, 2573–2587, https://doi.org/10.5194/hess-20-2573-2016, 2016.
Huntington, T. G.: Evidence for intensification of the global water cycle: Review and synthesis, J. Hydrol., 319, 83–95, https://doi.org/10.1016/j.jhydrol.2005.07.003, 2006.
IPCC: Climate Change 2013: The Physical Science Basis. Contribution of Working Group I to the Fifth Assessment Report of the Intergovernmental Panel on Climate Change, edited by: Stocker, T. F., Qin, D., Plattner, G.-K., Tignor, M., Allen, S. K., Boschung, J., Nauels, A., Xia, Y., Bex, V., and Midgley, P. M., Cambridge University Press, Cambridge, United Kingdom and New York, NY, USA, 1535 pp., 2013.
Jiang, C. and Wang, F.: Temporal changes of streamflow and its causes in the Liao River Basin over the period of 1953–2011, northeastern China, Catena, 145, 227–238, https://doi.org/10.1016/j.catena.2016.06.015, 2016.
Leng, G. Y., Tang, Q. H., Huang, M. Y., Hong, Y., and Ruby, L. L.: Projected changes in mean and interannual variability of surface water over continental China, Sci. China Earth Sci., 58, 739–754, https://doi.org/10.1007/s11430-014-4987-0, 2015.
Li, F. P., Zhang, Y. Q., Xu, Z. X., Teng, J., Liu, C. M., Liu, W. F., and Mpelasoka, F.: The impact of climate change on runoff in the southeastern Tibetan Plateau, J. Hydrol., 505, 188–201, https://doi.org/10.1016/j.jhydrol.2013.09.052, 2013.
Liang, X. and Xie, Z. H.: A new surface runoff parameterization with subgrid-scale soil heterogeneity for land surface models, Adv. Water Resour., 24, 1173–1193, https://doi.org/10.1016/s0309-1708(01)00032-x, 2001.
Liang, X., Lettenmaier, D. P., Wood, E. F., and Burges, S. J.: A simple hydrologically based model of land surface water and energy fluxes for general circulation models, J. Geophys. Res.-Atmos., 99, 14415–14428, https://doi.org/10.1029/94jd00483, 1994.
Liang, X., Lettenmaier, D. P., and Wood, E. F.: One-dimensional statistical dynamic representation of subgrid spatial variability of precipitation in the two-layer variable infiltration capacity model, J. Geophys. Res.-Atmos., 101, 21403–21422, https://doi.org/10.1029/96jd01448, 1996.
Liu, D., Mishra, A. K., and Zhang, K.: Runoff sensitivity over Asia: Role of climate variables and initial soil conditions, J. Geophys. Res.-Atmos., 122, 2218–2238, https://doi.org/10.1002/2016jd025694, 2017.
Liu, J., Du, H. B., Wu, Z. F., He, H. S., Wang, L., and Zong, S. W.: Recent and future changes in the combination of annual temperature and precipitation throughout China, Int. J. Climatol., 37, 821–833, https://doi.org/10.1002/joc.4742, 2017.
Liu, J. Y., Zhang, Q., Singh, V. P., and Shi, P. J.: Contribution of multiple climatic variables and human activities to streamflow changes across China, J. Hydrol., 545, 145–162, https://doi.org/10.1016/j.hydrol.2016.12.016, 2017a.
Liu, J. Y., Zhang, Q., Zhang, Y. Q., Chen, X., Li, J. F., and Aryal, S. K.: Deducing Climatic Elasticity to Assess Projected Climate Change Impacts on Streamflow Change across China, J. Geophys. Res.-Atmos., 122, 10197–10214, https://doi.org/10.1002/2017jd026701, 2017b.
Lohmann, D., NolteHolube, R., and Raschke, E.: A large-scale horizontal routing model to be coupled to land surface parametrization schemes, Tellus A, 48, 708–721, https://doi.org/10.1034/j.1600-0870.1996.t01-3-00009.x, 1996.
Milliman, J. D., Farnsworth, K. L., Jones, P. D., Xu, K. H., and Smith, L. C.: Climatic and anthropogenic factors affecting river discharge to the global ocean, 1951–2000, Global Planet. Change, 62, 187–194, https://doi.org/10.1016/j.gloplacha.2008.03.001, 2008.
Mitchell, D., AchutaRao, K., Allen, M., Bethke, I., Beyerle, U., Ciavarella, A., Forster, P. M., Fuglestvedt, J., Gillett, N., Haustein, K., Ingram, W., Iversen, T., Kharin, V., Klingaman, N., Massey, N., Fischer, E., Schleussner, C.-F., Scinocca, J., Seland, Ø., Shiogama, H., Shuckburgh, E., Sparrow, S., Stone, D., Uhe, P., Wallom, D., Wehner, M., and Zaaboul, R.: Half a degree additional warming, prognosis and projected impacts (HAPPI): background and experimental design, Geosci. Model Dev., 10, 571–583, https://doi.org/10.5194/gmd-10-571-2017, 2017.
Nash, J. E. and Sutcliffe, J. V.: River flow forecasting through conceptual models part I – A discussion of principles, J. Hydrol., 10, 282–290, https://doi.org/10.1016/0022-1694(70)90255-6, 1970.
Ouyang, F., Zhu, Y. H., Fu, G. B., Lu, H. S., Zhang, A. J., Yu, Z. B., and Chen, X.: Impacts of climate change under CMIP5 RCP scenarios on streamflow in the Huangnizhuang catchment, Stoch. Env. Res. Risk A., 29, 1781–1795, https://doi.org/10.1007/s00477-014-1018-9, 2015.
Ouyang, Z., Zheng, H., Xiao, Y., Polasky, S., Liu, J., Xu, W., Wang, Q., Zhang, L., Xiao, Y., Rao, E. M., Jiang, L., Lu, F., Wang, X. K., Yang, G. B., Gong, S. H., Wu, B. F., Zeng, Y., Yang, W., and Daily, G. C.: Improvements in ecosystem services from investments in natural capital, Science, 352, 1455–1459, https://doi.org/10.1126/science.aaf2295, 2016.
Piao, S. L., Ciais, P., Huang, Y., Shen, Z. H., Peng, S. S., Li, J. S., Zhou, L. P., Liu, H. Y., Ma, Y. C., Ding, Y. H., Friedlingstein, P., Liu, C. Z., Tan, K., Yu, Y. Q., Zhang, T. Y., and Fang, J. Y.: The impacts of climate change on water resources and agriculture in China, Nature, 467, 43–51, https://doi.org/10.1038/nature09364, 2010.
Schleussner, C. F., Rogelj, J., Schaeffer, M., Lissner, T., Licker, R., Fischer, E. M., Knutti, R., Levermann, A., Frieler, K., and Hare, W.: Science and policy characteristics of the Paris Agreement temperature goal, Nat. Clim. Change, 6, 827–835, https://doi.org/10.1038/nclimate3096, 2016.
She, D. X., Xia, J., Shao, Q. X., Taylor, J. A., Zhang, L. P., Zhang, X., Zhang, Y. J., and Gu, H. H.: Advanced investigation on the change in the streamflow into the water source of the middle route of China's water diversion project, J. Geophys. Res.-Atmos., 122, 6950–6961, https://doi.org/10.1002/2016jd025702, 2017.
Tao, F. L. and Zhang, Z.: Impacts of climate change as a function of global mean temperature: maize productivity and water use in China, Clim. Change, 105, 409–432, https://doi.org/10.1007/s10584-010-9883-9, 2011.
Tao, F. L., Yokozawa, M., Hayashi, Y., and Lin, E.: Terrestrial water cycle and the impact of climate change, Ambio, 32, 295–301, https://doi.org/10.1639/0044-7447(2003)032[0295:twcati]2.0.co;2, 2003.
Todini, E.: The ARNO rainfall-runoff model, J. Hydrol., 175, 339–382, https://doi.org/10.1016/s0022-1694(96)80016-3, 1996.
Wang, G. Q., Zhang, J. Y., Jin, J. L., Pagano, T. C., Calow, R., Bao, Z. X., Liu, C. S., Liu, Y. L., and Yan, X. L.: Assessing water resources in China using PRECIS projections and a VIC model, Hydrol. Earth Syst. Sci., 16, 231–240, https://doi.org/10.5194/hess-16-231-2012, 2012.
Wang, G. Q., Zhang, J. Y., Pagano, T. C., Xu, Y. P., Bao, Z. X., Liu, Y. L., Jin, J. L., Liu, C. S., Song, X. M., and Wan, S. C.: Simulating the hydrological responses to climate change of the Xiang River basin, China, Theor. Appl. Climatol., 124, 769–779, https://doi.org/10.1007/s00704-015-1467-1, 2016.
Xie, Z. H., Su, F. G., Liang, X., Zeng, Q. C., Hao, Z. C., and Guo, Y. F.: Applications of a surface runoff model with Horton and Dunne runoff for VIC, Adv. Atmos. Sci., 20, 165–172, 2003.
Xie, Z. H., Yuan, F., Duan, Q. Y., Zheng, J., Liang, M. L., and Chen, F.: Regional parameter estimation of the VIC land surface model: methodology and application to river basins in China, J. Hydrometeorol., 8, 447–468, https://doi.org/10.1175/jhm568.1, 2007.
Xu, W. H., Xiao, Y., Zhang, J. J., Yang, W., Zhang, L., Hull, V., Wang, Z., Zheng, H., Liu, J. G., Polasky, S., Jiang, L., Xiao, Y., Shi, X. W., Rao, E. M., Lu, F., Wang, X. K., Daily, G. C., and Ouyang, Z. Y.: Strengthening protected areas for biodiversity and ecosystem services in China, P. Natl. Acad. Sci. USA, 114, 1601–1606, https://doi.org/10.1073/pnas.1620503114, 2017.
Yuan, Y. J., Zhang, C., Zeng, G. M., Liang, J., Guo, S. L., Huang, L., Wu, H. P., and Hua, S. S.: Quantitative assessment of the contribution of climate variability and human activity to streamflow alteration in Dongting Lake, China, Hydrol. Process., 30, 1929–1939, https://doi.org/10.1002/hyp.10768, 2016.
Zhai, R. and Tao, F.: Contributions of climate change and human activities to runoff change in seven typical catchments across China, Sci. Total Environ., 605, 219–229, https://doi.org/10.1016/j.scitotenv.2017.06.210, 2017.
Zhang, Q., Liu, J. Y., Singh, V. P., Shi, P. J., and Sun, P.: Hydrological responses to climatic changes in the Yellow River basin, China: Climatic elasticity and streamflow prediction, J. Hydrol., 554, 635–645, https://doi.org/10.1016/j.jhydrol.2017.09.040, 2017.
Zhang, Y. Q., You, Q. L., Chen, C. C., and Ge, J.: Impacts of climate change on streamflows under RCP scenarios: A case study in Xin River Basin, China, Atmos. Res., 178, 521–534, https://doi.org/10.1016/j.atmosres.2016.04.018, 2016.