the Creative Commons Attribution 4.0 License.
the Creative Commons Attribution 4.0 License.
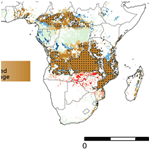
Multi-fold increase in rainforest tipping risk beyond 1.5–2 °C warming
Chandrakant Singh
Ruud van der Ent
Ingo Fetzer
Lan Wang-Erlandsson
Tropical rainforests rely on their root systems to access moisture stored in soil during wet periods for use during dry periods. When this root zone soil moisture is inadequate to sustain a forest ecosystem, they transition to a savanna-like state, losing their native structure and functions. Yet the influence of climate change on ecosystem's root zone soil moisture storage and the impact on rainforest ecosystems remain uncertain. This study assesses the future state of rainforests and the risk of forest-to-savanna transitions in South America and Africa under four Shared Socioeconomic Pathways (SSP1-2.6, SSP2-4.5, SSP3-7.0, and SSP5-8.5). Using a mass-balance-based empirical understanding of root zone storage capacity (Sr), defined as the maximum volume of root zone soil moisture per unit area accessible to vegetation's roots for transpiration, we project how rainforest ecosystems will respond to future climate changes. We find that under the end-of-the-21st-century climate, nearly one-third of the total forest area will be influenced by climate change. As the climate warms, forests will require a larger Sr than they do under the current climate to sustain their ecosystem structure and functions, making them more susceptible to water limitations. Furthermore, warming beyond 1.5–2 °C will significantly elevate the risk of a forest–savanna transition. In the Amazon, the forest area at risk of such a transition grows by about 1.7–5.8 times in size compared to the immediate lower-warming scenario (e.g. SSP2-4.5 compared to SSP1-2.6). In contrast, the risk growth in the Congo is less substantial, ranging from 0.7–1.7 times. These insights underscore the urgent need to limit the rise in global surface temperature below the Paris Agreement to conserve rainforest ecosystems and associated ecosystem services.
- Article
(6647 KB) - Full-text XML
-
Supplement
(16263 KB) - BibTeX
- EndNote
Tropical rainforests in the Amazon and Congo basins are critical to the Earth system since they store and sequester a large amount of carbon, host vast biodiversity, and regulate the global water cycle (Malhi et al., 2014). However, these forests are under severe pressure from climate and land-use changes (Davidson et al., 2012; Lewis et al., 2015; Malhi et al., 2008). These changes result in decreased precipitation, increased seasonality, and higher atmospheric water demand (Malhi et al., 2014), leading to soil moisture deficits that inhibit plant growth (Singh et al., 2020; Wang-Erlandsson et al., 2022). Furthermore, projected increases in drought frequency, severity, and duration under future climate change (Dai, 2011; Liu et al., 2018) pose imminent threats to the capacity of rainforests to maintain their native ecological structure and functions (i.e. forest resilience) (Bauman et al., 2022; Grimm et al., 2013; Jones et al., 2009).
Under water-deficit conditions, rainforests adapt by investing in their root systems to gain better access to soil moisture necessary to maintain their structure and functions (Singh et al., 2020, 2022). At the same time, the availability of surplus moisture at shallow depths minimises the need for ecosystems to invest in extensive (deeper and lateral) root systems (Bruno et al., 2006). Furthermore, forest ecosystems adapt to climate change by optimising water distribution through mechanisms such as hydraulic redistribution (Liu et al., 2020; Oliveira et al., 2005), enhancing water-use efficiency by regulating stomatal conductance, and even shedding leaves (Wolfe et al., 2016) to minimise moisture loss (Barros et al., 2019; Brum et al., 2019; Lammertsma et al., 2011). Despite their critical role, the dynamic influence of climate change on vegetation's rooting structure and subsoil moisture is challenging to measure at the ecosystem scale (Fan et al., 2017). Thus, understanding how moisture from wet periods is stored, transmitted, and lost from the soil, as well as how it is accessed by vegetation during dry periods, is critical to the ecohydrology and resilience of terrestrial ecosystems under climate change.
However, such ecohydrological dynamics remain challenging to incorporate in Earth system models (ESMs) (Lenton, 2011; Maslin and Austin, 2012; Valdes, 2011), which have complex mathematical representations of Earth system processes and interactions across different biospheres. This limits the capacity of ESMs to simulate tipping points as an emergent property of the system (i.e. properties that emerge due to multiple interactions between several system components and are not the property of an individual component) (Hirota et al., 2021; Reyer et al., 2015; Singh et al., 2023). This constraint is mainly due to our poor understanding of complex mechanisms governing the ecosystem, which are not well represented in ESMs. This includes a limited understanding of vegetation–climate feedbacks (Boulton et al., 2013, 2017; Chai et al., 2021), subsoil moisture availability (Cheng et al., 2017), ecosystem adaptation dynamics (Yuan et al., 2022), the response time of forest ecosystems to climate change perturbations, and assumptions about future (i.e. prescribed) land-use change (Hurtt et al., 2020) in the ESMs. Furthermore, in the Earth system, some interactions still remain largely unknown, thereby making the prediction of the (abrupt) forest-to-savanna transition (referring to changes in the dense-canopy structure of forests to one that mimics an open-canopy structure similar to savanna; hereafter referred to as the forest–savanna transition) challenging (Drijfhout et al., 2015; Hall et al., 2019; Koch et al., 2021).
To understand the extent of rainforest tipping risks, there is a need to assess and contrast the forest resilience consequences of the low-emission scenario and current commitment trajectories with the more commonly used high-emission scenario (Jehn et al., 2022). However, the risk of forest–savanna transitions under various possible climate future scenarios is relatively under-investigated. As a result of the conflicting findings and scenario-dependent uncertainties, the Intergovernmental Panel on Climate Change (IPCC) has only low confidence about the possible tipping of the Amazon rainforest by the end of the 21st century (Canadell et al., 2023). However, with mounting empirical evidence on how climate change influences rainforest ecosystems (Boulton et al., 2022; Küçük et al., 2022; Singh et al., 2020, 2022), the research on rainforest resilience loss has accelerated considerably in the recent decade (Ahlström et al., 2017; Huntingford et al., 2013). Yet, forest resilience is often assessed based on changes in forest carbon stocks (Huntingford et al., 2013; Parry et al., 2022) or precipitation (Hirota et al., 2011; Staal et al., 2020; Zemp et al., 2017) and rarely on the subsoil moisture availability of the ecosystem (Singh et al., 2022).
This study aims to assess the state of rainforests and the risk of a forest–savanna transition under the end-of-the-21st-century climate based on an empirical understanding of ecosystems' root zone storage dynamics. For this, we use mass-balance-derived root zone storage capacity (Sr) – representing the maximum amount of soil moisture vegetation can access for transpiration (Gao et al., 2014; Singh et al., 2020; Wang-Erlandsson et al., 2016). Our use of Sr is grounded in its effectiveness in representing ecosystems' access to soil moisture and their ability to modify above-ground structures accordingly (de Boer-Euser et al., 2016; Singh et al., 2020; Stocker et al., 2023; Wang-Erlandsson et al., 2016). It should be noted that we refer to rainforest tipping as a forest–savanna transition risk since the timing of such transitions depends on the stochastic fluctuations in other environmental factors, beyond just hydroclimate (e.g. fire, human influence, and species composition) (Cole et al., 2014; Cooper et al., 2020; Higgins and Scheiter, 2012; Poorter et al., 2016). Therefore, to project if an ecosystem is a forest or has tipped to savanna in the future, we assume the hydroclimate projected by the end of the 21st century (i.e. 2086–2100) and ecosystem are in equilibrium. However, we do not account for the time required for ecosystems to reach their (long-term) equilibrium state, which previous studies suggest can take between 50–200 years after crossing the tipping point (Armstrong McKay et al., 2022).
2.1 Study area
This study focuses on forest ecosystems (i.e. excluding savanna/grassland and vegetation in human-influenced ecosystems) extending between 15° N–35° S for South America and Africa.
2.2 Data
This analysis uses both empirical and ESM-simulated datasets of precipitation and evaporation. Empirical datasets include remotely sensed and observation-corrected precipitation and evaporation time series. Empirical precipitation estimates at daily time steps are obtained from the Climate Hazards Group InfraRed Precipitation with Station data (CHIRPS; 0.25° resolution) (Funk et al., 2015). Furthermore, empirical evaporation is derived using an equally weighted ensemble of three different datasets – (i) the Breathing Earth System Simulator (BESS; 0.5° resolution) (Jiang and Ryu, 2016), (ii) Penman–Monteith–Leuning (PML; 0.5° resolution) (Zhang et al., 2016), and (iii) FLUXCOM-RS (0.083° resolution) (Jung et al., 2019) – at monthly time steps. Here, evaporation represents the sum of all evaporated moisture from the soil, open water, and vegetation, including interception and transpiration. We only selected evaporation datasets free from biome-dependent parameterisation (such as plant function types, stomatal conductance, and maximum root allocation depth) and soil layer depth (represents maximum depth of moisture uptake). Ultimately, all evaporation datasets are bilinearly interpolated to 0.25° resolution and downscaled to daily time step using ERA5 evaporation (0.25° resolution) estimates (Hersbach et al., 2020). All empirical datasets are obtained for 2001–2012.
We also obtained precipitation and evaporation estimates from 33 ESMs (from 22 different institutes), which includes CMIP6 historical and four Shared Socioeconomic Pathway (SSP) scenario simulations (SSP1-2.6 leads to approx. 1.3–2.4 °C warming; SSP2-4.5 corresponds to 2.1–3.5 °C warming and is closest to the current trajectory, according to the nationally determined contributions (SSP3-7.0 around 2.8–4.6 °C warming; and SSP5-8.5 represents 3.3–5.7 °C warming; “°C warming” represents an increase in mean global surface temperature change by the end of the 21st century relative to 1850–1900) (IPCC, 2023) (Fig. 1; Tables S1 and S2). The historical estimates are obtained at a monthly time step for 2000–2014, and the estimates under different SSPs are obtained for 2086–2100. Though obtained estimates from different ESMs are at different spatial resolutions, we bilinearly interpolated them to 0.25° for this analysis.
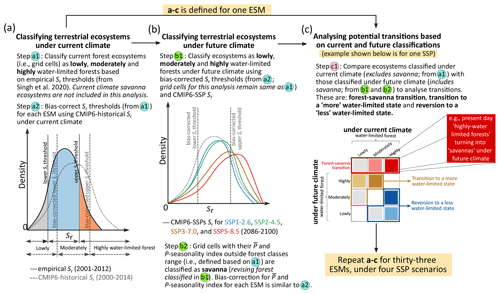
Figure 1Methodological framework for analysing the potential transitions in tropical terrestrial ecosystems using empirical and CMIP6 Earth system model (ESM) hydroclimate estimates. (a) We use root zone storage capacity (Sr)-based classification thresholds (obtained from Singh et al., 2020) – calculated using empirical precipitation (P) and evaporation (E) estimates (Fig. S1; see Sect. 2 and Appendix A1) – to classify terrestrial ecosystems under the current climate. Savanna ecosystems under the current climate are excluded from this analysis. We bias-corrected these Sr thresholds for all ESMs using the histogram equivalence method (Piani et al., 2010) (Table S1). (b) We then use these bias-corrected Sr thresholds to classify ecosystems under future climate conditions (Figs. S2 and S3). Furthermore, we use mean annual precipitation () and P seasonality index range (Sr-based forest classes from panel a) – as a proxy for ecosystem state – to revise our classification under future climate conditions (Appendix A3 and Fig. S4). (c) We then analyse the potential transitions by comparing ecosystems classified under the current climate (analysed in panel a) with those classified under future climate (analysed in panel b) individually for all ESMs (Figs. S5 and S6). The transition analysis assumes that the hydroclimate and the ecosystem are in equilibrium and does not account for the time required for transitions to occur. A detailed description is provided in the Sect. 2. An exemplification of this methodological framework is shown in Fig. S7.
Finally, to minimise the influence of human activity and non-forest land cover on the natural water cycle, we utilised land cover data to remove pixels with such features from our analysis. We began by removing human-influenced and non-forest land cover, such as savanna, grasslands, and waterbodies, from GlobCover, a global land cover classification dataset by the European Space Agency (ESA) at 300 m resolution (GlobCover land-use map, 2022). We then performed majority interpolation to convert the dataset to a 0.25° resolution and to mask grid cells with less than 50 % forest cover. This step ensured that only grid cells with over 50 % forest cover were classified as forests for further analysis.
2.3 Root zone storage-capacity-based framework for projecting forest transitions
Vegetation uptakes soil moisture from its roots; thus, the availability of root zone moisture is a key element that mediates the interaction between vegetation and climate (Brooks et al., 2015; Küçük et al., 2022; Rosas et al., 2019; Wang-Erlandsson et al., 2022). However, measuring soil (such as texture and porosity) and root characteristics (such as vertical and lateral extent and soil moisture uptake profiles) that influence access to subsoil moisture are challenging to measure at ecosystem scales (Bruno et al., 2006). Furthermore, land system models tend to oversimplify the transfer and storage of water in the root zone due to insufficient knowledge about soil–vegetation–climate interactions (Albasha et al., 2015; Hildebrandt et al., 2016; Wang et al., 2004). In such cases, the mass-balance-approach-based Sr provides a tangible and comprehensive understanding of ecosystem access to moisture stored in the soil (de Boer-Euser et al., 2016; Gao et al., 2014; McCormick et al., 2021; Stocker et al., 2023).
2.3.1 Estimating mass-balance-derived root zone storage capacity (Sr)
Derived using the mass balance approach,Sr represents the maximum amount of soil moisture accessed by vegetation for transpiration (Singh et al., 2020; Wang-Erlandsson et al., 2016). This methodology calculates the maximum extent of soil moisture within the reach of plant roots, assuming that ecosystems do not invest in expanding their root zone storage beyond what is necessary to bridge the maximum (accumulated) water deficit experienced by the vegetation during dry periods (i.e. periods in which evaporation is greater than rainfall, irrespective of the seasons). This maximum annual accumulated water deficit (Da,y) experienced by the ecosystem is calculated using daily precipitation and evaporation estimates (Appendix A1 and Fig. A1). Subsoil moisture beyond the reach of plant roots is primarily controlled by gravity-induced gradients (de Boer-Euser et al., 2016) and is not available for transpiration. The rationale is that any extensive investment (i.e. more than necessary) in root expansion would require carbon allocation and, thus, is inefficient from the perspective of the plants (Gao et al., 2014; Schenk, 2008). Since this approach does not rely on prior information about vegetation, soil, or land cover, using empirical (observation-based) datasets (Appendix A1 and Fig. A1), we capture the dynamics of actual soil moisture available for the ecosystems (Wang-Erlandsson et al., 2016). The detailed methodology for calculating Sr using precipitation and evaporation estimates is outlined in Appendix A1.
In this mass balance approach, Sr only represents a hydrological buffer essential for maintaining the ecosystem's structure and functions (Gao et al., 2014; Wang-Erlandsson et al., 2016). However, other biotic and abiotic factors, such as root morphology, soil depth, and geological formations, can physically restrict Sr by limiting rooting depth, rooting structure, and the soil's water-holding capacity (Canadell et al., 1996; Jackson et al., 1996; Schenk and Jackson, 2002) (Appendix A2). Additionally, soil properties like porosity or field capacity could necessitate a deeper rooting strategy in different soil types (e.g. between sandy and clayey soil) to achieve a comparable level of Sr to sustain the ecosystem under future climate (Kukal and Irmak, 2023). However, this study assesses the impact of future climate change on the ecosystem's hydrological regime, focusing on the changes to the ecosystem's equilibrium state. Therefore, the direct influence of soil and root characteristics under future climate change on Sr (Appendix A2) and forest transitions falls outside our current scope.
2.3.2 Determining root zone storage capacity thresholds for forest transitions
A recent study by Singh et al. (2020) demonstrated that Sr can effectively represent an ecosystem's above-ground state (i.e. whether it is a forest or savanna) and its level of water stress, based on root zone moisture availability. In this study, we refine their terminology from “water-stressed state” to “water-limited state” to more precisely describe the effects of changes in hydroclimatic conditions on forest and savanna ecosystems. They classified these terrestrial ecosystem responses into four distinct categories based on the relationship between tree cover density and root zone storage capacity (Sr) (for a more detailed description, see Singh et al., 2020) as follows.
- i.
Lowly water-limited forest. Dense forests (> 70 % tree cover) that receive ample rainfall (with daily precipitation exceeding evaporation year-round; Singh et al., 2020) result in a very low Da,y (Appendix A1). In such an environment, the top layer of the soil remains consistently damp, allowing for efficient soil moisture uptake through shallow roots (< 1 m; Sr and maximum rooting depth comparison in Singh et al., 2020), as vegetation typically utilises the shortest available pathway for moisture uptake (Bruno et al., 2006). Consequently, these forest ecosystems can sustain themselves with a low Sr (< 100 mm) (Singh et al., 2020).
- ii.
Moderately water-limited forest. Although these forests retain a dense structure (> 65 % tree cover), the increased precipitation seasonality (evaporation rates remain the same as before; Singh et al., 2020) leads to a relatively higher Da,y (Appendix A1). This necessitates greater investment in their rooting systems to access subsoil moisture for dry periods, with Sr for these ecosystems ranging between 100–400 mm in South America and 100–350 mm in Africa (Singh et al., 2020). Notably, this enhanced below-ground investment does not compromise the above-ground ecosystem structure, as evidenced by the changes in ecosystem rooting structure relative to tree cover (Singh et al., 2020).
- iii.
Highly water-limited forest. With further increases in precipitation seasonality (even negligible precipitation during dry seasons) and the duration of the dry period, forests need to maximise their Sr to sustain their structure (see Figs. S2 and S3 in Singh et al., 2020). Maximum rooting depths of these ecosystems can typically range between 15–20 m (Singh et al., 2020). Maintaining ecosystems under these conditions is costly from a subsoil investment perspective (Schenk, 2008), with regions in South America and Africa showing Sr values as high as 750 and 450 mm, respectively (Singh et al., 2020). Consequently, these values represent the upper limits beyond which forest ecosystems cannot further enhance their Sr (Singh et al., 2020).
Possible mechanisms suggest that these trees adapt by shedding leaves to minimise moisture loss (Wolfe et al., 2016). However, this adaptation can reduce photosynthetic activity, leading to declines in root growth, and heighten the risk of mortality from hydraulic failures due to the unavailability of soil moisture at accessible depths (Guswa, 2008). Furthermore, the accumulation of dry leaves also perpetuates forest fires, thinning the ecosystem even further (tree cover can drop as low as 30 %) (Nepstad et al., 1999; Singh et al., 2020). Although increased tree mortality reduces competition for water, enabling some trees to survive, the heightened risk of hydraulic failures and forest fires makes these ecosystems highly susceptible to transitioning to savanna (Anderegg et al., 2016; Oliveras and Malhi, 2016; Sperry and Love, 2015).
- iv.
Savanna–grassland regime (hereafter referred to as savanna). These ecosystems, typically characterised by an open grass-dominated structure (tree cover < 40 %), have a lower water availability and demand (both precipitation and evaporation are lower than in forest ecosystems) (Ratnam et al., 2011; Singh et al., 2020). Thus, they require a lower hydrological buffer to sustain their structure and functions. For these ecosystems, Sr values can be as low as 100 mm (Singh et al., 2020). Although tree species in this ecosystem can develop deep roots (extending up to 20 m; see Figs. 2 and 3 in Singh et al., 2020), the majority of the root biomass is concentrated in the shallow soil layers (top 30–50 cm; shallow-water uptake profile) (February and Higgins, 2010; Schenk, 2008). This strategy allows for competitive moisture uptake between trees and grass species (Nippert and Holdo, 2015). This also suggests that, for savanna, deeper roots do not always necessitate a high Sr (Singh et al., 2020).
The difference in Sr thresholds between both continents is due to the presence of water-use-efficient C4 grasses in Africa (Still et al., 2003), which reduces the competitiveness for moisture uptake between tree species and grasses – leading to a lesser need for extensive Sr in the African forest ecosystem (Singh et al., 2020). Furthermore, these adaptation dynamics align with the alternative stable state theory (i.e. the forest's stabilising feedback under hydroclimatic changes and tipping risk beyond certain hydroclimatic extremes) (Hirota et al., 2011), which makes Sr more representative of the transient state of the ecosystem than precipitation (Singh et al., 2022). We, thus, use these mass-balance-derived Sr thresholds to project rainforest transitions and tipping risk under future climate change. A detailed description of how previous studies have projected rainforest tipping (Table S3) and how the Sr-based framework builds upon their shortcomings is mentioned in the Supplement.
2.3.3 Projecting forest transitions under future climate change
Due to the lack of appropriate metrics for vegetation structure (e.g. tree cover density, tree height, and floristic patterns) and the reliance on assumptions about future land-use change (i.e. prescribed rather than biophysically simulated) in ESMs (Hurtt et al., 2020), we use hydroclimate from ESMs as a proxy to project forest transitions under future climate conditions. Using this proxy, we assume that the hydroclimate projected for the end of the 21st century and the ecosystem are in equilibrium (Staal et al., 2020). We start by classifying forests under the current climate, following the approach by Singh et al. (2020), which uses the (empirical) daily estimates of CHIRPS precipitation and ensemble evaporation (2001–2012) (Appendix A1 and Sect. 2.3.2) (Fig. 1a). Since we are only interested in forest transitions, the ecosystems classified as savanna under the current climate are excluded from this analysis.
Next, for classifying ecosystems under future climate scenarios (Fig. 1b), we follow the same mass balance approach (Appendix A1). However, since precipitation and evaporation estimates from ESMs do not align with empirical estimates (Baker et al., 2021; McFarlane, 2011), we employ a bias correction method. Specifically, we use a histogram equivalence method (Piani et al., 2010) to adjust empirical Sr thresholds to comparable CMIP6 Sr thresholds for various ESMs (Table S1). This involves, first, calculating Sr using CMIP6 historical precipitation and evaporation estimates between 2000–2014 (Appendix A1 and Fig. S8). We then determine percentile-equivalent Sr thresholds for each of the 33 CMIP6 ESMs under the current climate. For example, if an empirical Sr of 100 mm corresponds to the 10th percentile (n=20 % of total pixels), we find the 10th percentile in the CMIP6 historical Sr, which may be higher or lower than 100 mm for each ESM (Fig. 1 and Table S1). These percentile-equivalent Sr thresholds are then used to classify ecosystems both under current (CMIP6 historical; 2000–2014) and future climate (CMIP6 SSPs; 2086–2100) (Fig. 1b).
Ultimately, we evaluate potential transitions by comparing ecosystems classified under current climate conditions (this excludes savanna) with those under future climate conditions (this includes savanna) (Sect. 2.3.2). These transitions are divided into three distinct categories (Figs. 1c and A2):
- i.
Forest–savanna transition. This refers to current climate forest ecosystems that risk transitioning to a savanna under future climate change. To classify savanna under future climate conditions, we assume the ecosystem is in equilibrium with the projected climate (see detailed steps in Appendix A3).
- ii.
Transition to a more water-limited state. This includes ecosystems that shift to a higher water-limited state in the future. For example, if a forest currently classified as lowly water-limited transitions to either a moderately or highly water-limited state in the future, it would fall under this category.
- iii.
Reversion to a less water-limited state. This includes ecosystems that shift to a lower water-limited state in the future.
To aggregate the results from all ESMs, grid cells with > 50 % convergence are referred to as “moderate–high model agreement”, 20 %–50 % as “moderate model agreement”, and ≤ 20 % as “low model agreement”. In the Sect. 3, we primarily discuss estimates from scenarios with > 20 % and > 50 % model convergence. While a threshold of > 20 % may seem low given the total number of ESMs analysed, it is important to recognise the variable and often limited capabilities of these ESMs, particularly in simulating biophysical interaction and emerging properties due to our limited understanding of the Earth system (Lenton et al., 2019; Stevens and Bony, 2013). Opting for a majority-based consensus in ESMs could overlook critical tipping risks identified by a minority of models, which might provide insights as valid as those from more widely agreeing models (Arora et al., 2023; Reyer et al., 2015).
2.4 Sensitivity analyses
Our methodology operates under two key assumptions, namely (i) the empirically derived Sr thresholds remain valid in the future, and (ii) the hydroclimatic estimates projected by ESMs accurately represent the actual climate, even though these models have prescribed land cover (Hurtt et al., 2020). To address the uncertainties related to the first assumption, we conduct four sensitivity analyses to assess the robustness of our analysis: (a) assuming that the regions exceeding the 99th percentile Sr are prone to a forest–savanna transition, as high-Sr investment could be unrealistic from the perspective of plants under future climate change; (b) evaluating forest transitions using three different evaporation datasets; (c) assessing forest transitions under 10- and 40-year drought return periods; and (d) adjusting the forest–savanna transition thresholds.
Regarding the second assumption, we explicitly apply this methodology across a wide range of available ESMs under four SSP scenarios to identify consistencies and discrepancies in the results. Additionally, the discrepancies between the prescribed land use and the forest transitions derived from our methodology, as well as the implications of these assumptions, are detailed in the Sect. 4.
We find that under future climate conditions (2086–2100), considering > 50 % model agreement, about one-quarter of the forests in both South America and Africa are projected to transition (Fig. 2b–g). With the > 20 % model agreement, these transitions are projected to occur for about three-quarters of the forests for both continents. Considering a lower threshold for model agreement causes double or triple counting of some transitions (Fig. 2b–g). To minimise this in further analyses, we only consider > 50 % model agreement for forests that transition to a more and less water-limited state. Furthermore, because (abrupt) forest–savanna transitions are under-represented in ESMs (Drijfhout et al., 2015; Lenton, 2011; Maslin and Austin, 2012; Valdes, 2011), we consider > 20 % model agreement for them. Considering this, we not only reduce the overlap to < 0.4 % of the total forest area (Fig. S9), but we also maximise highlighting forest–savanna transition risk for both continents.
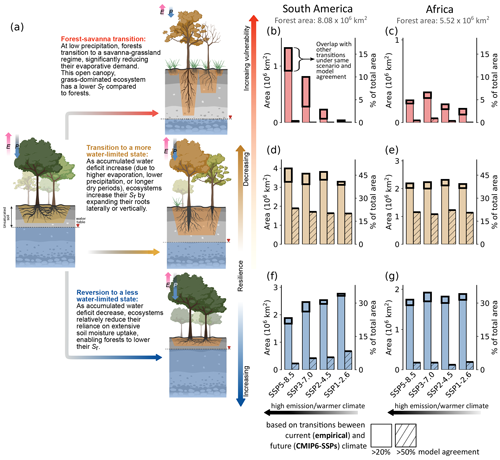
Figure 2Comparing the potential transitions under different SSP scenarios. (a) The state of the ecosystem, both above- and below-ground (post-transition), under future climate, quantifying (b, c) forest–savanna transition, (d, e) forests that transition to a more water-limited state, and (f, g) forests that revert to a less water-limited state for South America and Africa (present forest area mentioned at the top of panels b and c), respectively. For the analysis above, transitions are calculated for grid cells with model agreement > 20 % (plain bar plot) and > 50 % (hatched bar plot). These quantifications show changes in the forest area based on ecosystem transitions under (empirical) current climate conditions (2001–2012) and future climate conditions (2086–2100). For all of these transitions, we assume that the hydroclimate and the ecosystem are in equilibrium. Analyses comparing ecosystem transitions based on CMIP6 historical (2000–2014) and future (2086–2100) climate conditions are shown in Figs. S10 and S11. For each transition, the total area of spatial overlap with other transitions under the same SSP scenario and model agreement is highlighted with thick black bars. The P and E arrows in panel (a) describe the relative magnitude of precipitation and evaporation fluxes. The illustration in panel (a) is adapted from Singh et al. (2020) and created with https://BioRender.com, last access: 15 May 2024.
We find that the risk of forest–savanna transitions mainly occurs in the Guiana Shield of South America and the southern and south-eastern regions of Africa (Fig. 3). Compared to Africa, forest–savanna transitions are more prominent in South America under warmer climates (i.e. higher SSPs; Figs. 2b and 3). Our analysis reveals that the extent of forest–savanna transitions in South America decreases from almost 1.32 × 106 km2 (16.3 % of the total forest area in South America) under the highest-emission scenario to 0.04 × 106 km2 (0.5 %) under the lowest-emission scenario (Fig. 2b). Interestingly, for Africa, the extent of forest–savanna transition did not change much for different SSPs, i.e. (median) 0.25 × 106 km2, with a maximum deviation of ± 0.11 × 106 km2 (minimum and maximum extent of transition between 3 %–6.6 % of total forest area in Africa) (Fig. 2c).
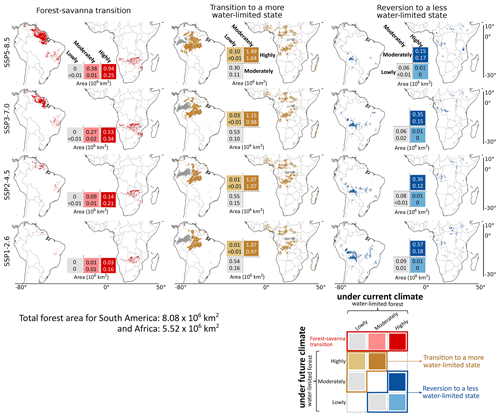
Figure 3Spatial extent of potential transitions with respect to their current state under different SSP scenarios. We analysed transitions, explicitly focusing on the forest–savanna transition, the transition to a more water-limited state, and a reversion to a less water-limited state by comparing different ecosystem classes under current (empirical; 2001–2012) and future (SSPs; 2086–2100) climate conditions (as defined in Fig. 2). All transitions shown above are analysed for moderate–high (> 50 %) model agreement, except forest–savanna transition, for which moderate (> 20 %) model agreement is considered. Values overlaying the legends correspond to the total area of transition for South America (top values) and Africa (bottom values).
When comparing the changes in forest–savanna transition risk areas relative to their immediate lower-warming scenarios, we find considerable increases for South America. The highest relative growth of approximately 5.75 times is observed between SSP1 and SSP2, with the forest area under risk increasing from 0.04 × 106 to 0.23 × 106 km2, respectively. It increases by 3.48 times from SSP2 to SSP3 (0.23 × 106 to 0.80 × 106 km2) and by 1.65 times from SSP3 to SSP5 (0.80 × 106 to 1.32 × 106 km2). For Africa, however, the increases are more modest; the risk grows by 1.29 times from SSP1 to SSP2 (0.17 × 106 to 0.22 × 106 km2), by 1.63 times from SSP2 to SSP3 (0.22 × 106 to 0.36 × 106 km2) and is observed to decrease by 0.72 times from SSP3 to SSP5 (0.36 × 106 to 0.26 × 106 km2).
By evaluating changes to their hydroclimate, we find that under warmer climates, forest–savanna transition regions in both continents are projected to experience a decrease in precipitation. Furthermore, we observe an increase in precipitation seasonality for South America, whereas Africa shows a decrease (Fig. S12). Here, an increase in precipitation seasonality (seasonal variability in precipitation over the year) creates water-limited conditions for the ecosystem. In contrast, a decrease in seasonality and precipitation in Africa corresponds to a lower-moisture availability altogether. Nevertheless, for both these continents, this transition seems to occur for the previously highly water-limited forests under the current climate, followed by moderately water-limited forests, with the least contribution from lowly water-limited forests (Fig. 3). This highlights the looming risk on highly water-limited forests to experience a forest–savanna transition under warmer climates.
Forests that transition to a “more” water-limited state in South America are spatially aggregated towards the border between Brazil, Colombia, and Peru – covering a considerable portion of the central Amazon (Fig. 3). On the other hand, for Africa, these forests exist in moderate to small patches towards the northern and southern extent of central Congo rainforests. We observe that these transitions account for most of the projected changes to the forest states across both continents (Fig. 2d, e), with the transition to just the “highly water-limited forest” accounting for more than three-quarters of all such transitions (Fig. 3). We observe that South American forests gradually become increasingly water-limited under warmer climates, with maximum and minimum projected transition of 1.89 × 106 km2 (23.4 %) and 1.61 × 106 km2 (19.9 %) observed under the highest- and lowest-emission scenarios, respectively (Fig. 2d, e). On the other hand, for Africa, the change in the water-limited state of the forests under different SSP scenarios remains almost similar (i.e. median 1.14 (± 0.06) × 106 km2; 19.6 %–22.2 %). Analysis of their hydroclimatic changes reveals that the water limitation is induced by both a decrease in precipitation and an increase in seasonality in South America (Fig. S13). In contrast, water limitation in Africa is driven solely by an increase in seasonality. We observe that these newly water-limited forests seem to have permeated to regions that were previously (under the current climate) dominated by lowly and moderately water-limited forests (Fig. 3). Here, this shift only signifies the changes to hydroclimatic conditions, allowing forests to transition to a more water-limited state, rather than the changes to the floristic composition of terrestrial species from one location to another. Although such a shift under changing climate is not unlikely (Esquivel-Muelbert et al., 2019), they are not analysed in this study.
Forests that revert to a “less” water-limited state in South America are primarily observed in the south-eastern Amazon, with small patches observed towards eastern Brazil and the western coast of Equatorial Guinea and Gabon (Fig. 3). For Africa, the reverted forests exist in patches in the northern and southern regions of the Congo rainforest. Furthermore, for South America, we observe a gradual decrease in these reversions with an increase in warming. Here, we observe the lowest reversion of 0.23 × 106 km2 (2.8 %) under the highest-emission scenario and the highest reversion of 0.67 × 106 km2 (8.4 %) under the lowest-emission scenario (Fig. 2f, g). For Africa, these trends remain almost similar under all SSPs (i.e. median 0.18 (± 0.05) × 106 km2; 2.2 %–3.5 %). Comparing these transitions with their hydroclimatic changes reveals an overall increase in precipitation (Fig. S14). Interestingly, we observe a much higher precipitation increase for South America under high-emission scenarios than those in lower-emission scenarios. However, we find that precipitation seasonality is also higher for these ecosystems under warmer climates (Fig. S14). This suggests that increased precipitation without changes to precipitation seasonality helps decrease the water limitation of the ecosystem, compared to the ecosystems that experienced a simultaneous increase in both.
Our sensitivity analysis, detailed in Appendix B1, reveals a consistent pattern of forest transitions across various scenarios.
4.1 Asynchronous resilience risks under future climate change
Our analysis reveals the spatial extent of potential ecosystem transitions in South America and Africa and their vulnerability to future climate change (Figs. 2 and 4). For South America, we find a clear indication of a decrease in forest resilience (i.e. an increase in water-limited forests) and an increase in forest–savanna transition risk under warmer climates (Fig. 2b, d, f). In contrast, these trends are not symmetric for Africa, where transition risk shows only slight variation across the different SSPs (Fig. 2c, e, g). Similar to the results of this study, previous studies on rainforest tipping have also suggested that exceeding 1.5–2 °C will considerably increase the tipping risk (Flores et al., 2024; Jones et al., 2009; Parry et al., 2022), with the Guiana Shield in the Amazon being the most susceptible under future climate change (Cox et al., 2004; Staal et al., 2020) (Fig. 3 and Table S3). Previous studies also agree that, in contrast to the Amazon, the projected risk to Congo rainforests is not substantial (Higgins and Scheiter, 2012; Staal et al., 2020) (Fig. 2). Despite it being unclear to what extent the ESMs represent the correct carbon–water dynamics (Koch et al., 2021), our results show a further divergence between Amazon's and Congo's responses to different SSPs (Figs. 2 and S12–14). This could either be caused simply by a different response to changes in precipitation patterns over the respective regions (Kooperman et al., 2018; Li et al., 2022) or a different response to increased CO2 levels in the atmosphere (Brienen et al., 2015; Hubau et al., 2020; Trumbore et al., 2015).
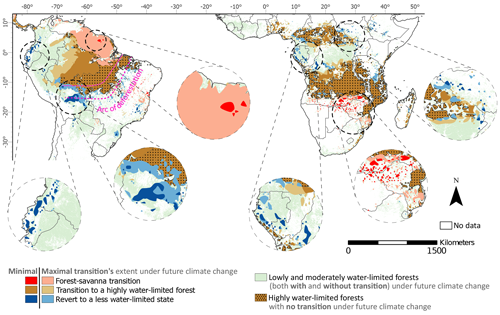
Figure 4Minimal and maximal extent of potential ecosystem transitions under future climate change in the entire study region over South America and Africa. The three transition types are forest–savanna transition, from any class to highly water-limited forests, and to a less water-limited state (see definitions in Figs. 2 and 3). For better visualisation of these transitions, in this figure, we first converted all grid cells to shape, merged them, and then smoothed them using the “polynomial approximation with exponential kernel” function (with a tolerance value of 1) in ArcGIS pro. The unsmoothed version of the transitions is shown in Fig. 3. The minimal and maximal represent the minimum and maximum possible extent of transitions (as quantified in Fig. 3) based on changes between current (empirical; 2001–2012) and future (SSPs; 2086–2100) climate conditions regardless of the SSP scenarios.
Previous empirical studies have linked these divergent responses to evolutionary and biogeographical differences between the ecosystems, which resulted in distinct species pools that uniquely influence each ecosystem's adaptability and response to climate change (Fleischer et al., 2019; Hahm et al., 2019; Hubau et al., 2020; Slik et al., 2018). These studies found that forest ecosystems in the Amazon tend to be more dynamic – grow faster due to high-CO2 levels in the atmosphere – than those in the Congo rainforests. However, these fast-growing trees also die young due to them investing substantially less in their adaptive strategies against perturbations than (less dynamic) old-growth forests (Brienen et al., 2015; Körner, 2017; Rammig, 2020). This makes the Amazon rainforest especially sensitive to CO2 emission pathways, as the positive influence of CO2-fertilisation-induced growth is counteracted by the negative impact of warming and droughts, thereby exacerbating the risk of forest mortality under high-emission scenarios (Brienen et al., 2015; Hubau et al., 2020; Yang et al., 2018). In this case, the projected changes to the future hydroclimate could be an artefact of decreased transpiration and precipitation due to forest mortality, rendering the rainforests vulnerable to tipping. In contrast, terrestrial species in Congo rainforests appear more resilient, having adapted to severe droughts during glacial periods, which makes them better equipped to handle episodic water-induced perturbations than the Amazon (Cole et al., 2014).
Nevertheless, with the compounding influence from land-use and climate-induced hydroclimatic changes (Davidson et al., 2012), these rainforests risk tipping to a savanna state. Our results highlight that by keeping the mean global surface temperature below 1.5–2 °C warming (which in this case is equivalent to SSP1-2.6 relative to the pre-industrial), we minimise forest–savanna transition risk and maximise recovery – thereby improving the resilience of rainforest ecosystems (Figs. 2, 3, and 4).
4.2 Changes in atmospheric moisture flow drive forest–savanna transition
Among all transitions, the most noticeable and catastrophic (since it is difficult to revert) is the forest–savanna transition projected in the Amazon's Guiana Shield of South America, and over the southern and south-eastern parts of Africa (Figs. 3 and 4). These transitions are associated with the shifting of the Intertropical Convergence Zone (ITCZ) (Mamalakis et al., 2021), which decreases precipitation and increases precipitation seasonality over the continents. For South America, the creation of these low-pressure bands allows the trade winds to bring in considerable moisture from the equatorial Atlantic Ocean over to Amazon by passing through the Guiana Shield and ultimately carrying it across the Río de la Plata basin via the South American low-level jet (Bovolo et al., 2018; van der Ent et al., 2010; Zemp et al., 2014). Similarly, for Africa, south-eastern trade winds bring moisture from the Indian Ocean over the centre of the African continent (Mamalakis et al., 2021).
Under a warmer climate, sea surface temperature over the equatorial Atlantic and the northern Indian Ocean is projected to increase (Pascale et al., 2019; Zilli et al., 2019), leading to a southward shift in the ITCZ over the eastern Pacific and Atlantic oceans and a northward shift over east Africa and the Indian Ocean (Mamalakis et al., 2021; Xie et al., 2010). Previous studies also acknowledge that the intense surface warming over the Sahara under future climate can also attract the ITCZ northwards in Africa (Cook and Vizy, 2012; Dunning et al., 2018; Mamalakis et al., 2021). These climate-change-induced shifts in the ITCZ can potentially both mitigate and exacerbate the effects of (accumulated) water deficit on the forest ecosystem, especially critical for highly water-limited forests, even without considering the changes to atmospheric moisture flow caused by localised deforestation (Leite-Filho et al., 2021; Schumacher et al., 2022; Staal et al., 2018; Wunderling et al., 2022). This underscores the importance of including changes in atmospheric circulation in studies that analyse the impact of future climate on the resilience of forest ecosystems (Staal et al., 2020; Zemp et al., 2017).
4.3 Discrepancy between prescribed future land use and projected transitions
The land-use information in CMIP6 ESMs is not biophysically simulated but prescribed based on simulations from integrated assessment models (IAMs) for each SSP scenario (Hurtt et al., 2020). Therefore, it is valuable to examine whether these prescribed land-use scenarios agree or conflict with the changes projected (assuming equilibrium between hydroclimate and the ecosystem) by our Sr-based ecosystem transitions (Figs. 5 and S15–17).
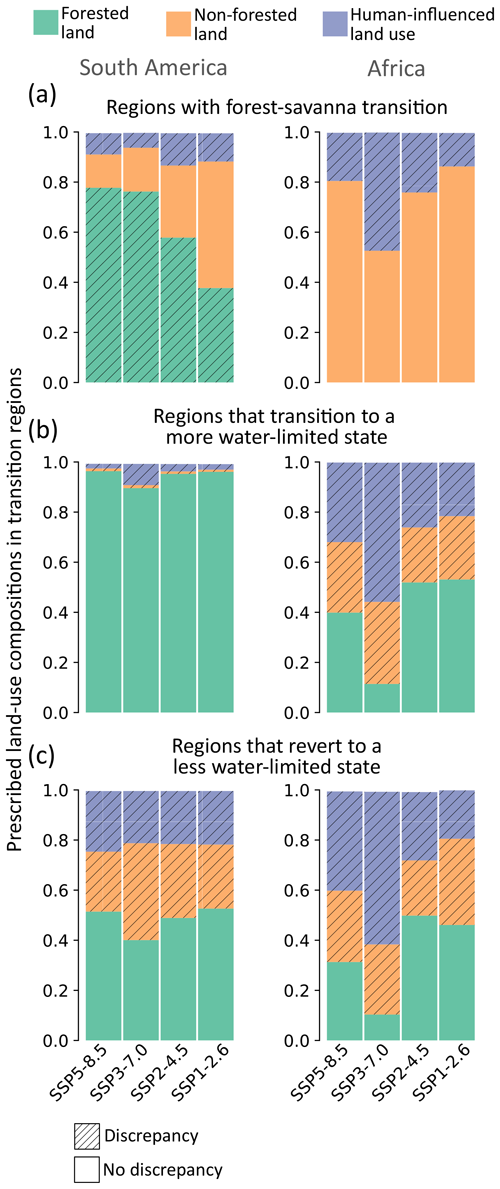
Figure 5Prescribed land-use composition for each transition region under different SSP scenarios (median 2086–2100), calculated as the ratio between the prescribed land use area and the projected transition area. Regions where IAM-prescribed land use are same as the projected transitions (from Fig. 3) are shown in plain colours (i.e. no discrepancy). Regions where IAM-prescribed land use differs from projected transitions are hatched (i.e. discrepancy).
The most noticeable discrepancies are observed in South America, where the extent of forest–savanna transitions is underestimated in prescribed land-use scenarios compared to those projected in this study (i.e. prescribed land-use predicts forests in the region whose hydroclimate cannot support forest; Figs. 4 and 5a). Additionally, in South America, our analysis highlights the potential of some forests reverting to a “less water-limited state” in places where the prescribed land use in the ESMs suggests non-forest landscapes (Figs. 4 and 5c). These discrepancies arise because the prescribed land use in CMIP6 ESMs does not shift in response to hydroclimatic changes. Despite our approach assuming equilibrium and overlooking the temporal dynamics of transitions, based on broad climate change patterns (Sect. 4.2), we believe it more accurately represents the ecohydrological state of the ecosystems.
However, these prescribed land uses can introduce errors in subsequent biophysical processes simulated in ESMs (Ma et al., 2020), affecting the accuracy of projected transitions. For example, prescribing a region as a forest that would be grassland in the future will lead to the extraction of deeper subsoil moisture in ESMs, which (actual) grasslands do not have the capacity to access (Ahlström et al., 2017; Yu et al., 2022). This will result in an overestimation of the ecosystem's evaporation, potentially altering precipitation patterns downwind, leading to inaccurate water budget assessments for these ecosystems, and consequently causing erroneous projections of the ecosystem state. These discrepancies underscore the urgent need for enhancements in the land surface components of ESMs, enabling dynamic simulations of vegetation–climate feedbacks. Such improvements would provide a more accurate representation of the ecohydrology of terrestrial ecosystems and their response to changing climate conditions.
4.4 Limitations
This study assumes that the Sr-derived thresholds used to classify terrestrial ecosystems under current climate conditions remain valid under future climate change. However, forests themselves are dynamically adapting their structure and functions in response to climate change, altering their critical thresholds (Doughty et al., 2023). Thus, assuming a static critical threshold may lead to inaccuracies in estimating forests' resilience to future climate change. For instance, under the CO2 fertilisation effect, forests may become more water-use efficient (i.e. transpire less and therefore need for a lower Sr) (Xue et al., 2015), potentially delaying their tipping under warming scenarios compared to those projected in this study. Conversely, factors such as nutrient limitation (Condit et al., 2013) or extensive human influence (van Nes et al., 2016) in the ecosystem might lead to an earlier tipping than anticipated.
However, the uncertainty surrounding the effect of CO2 fertilisation, nutrient limitation, and human influence on vegetation remains a significant research frontier for enhancing our understanding of rainforest tipping under future climate change (Fleischer et al., 2019; Hofhansl et al., 2016). Additionally, factors such as precipitation variability, species composition, soil properties, and topography can contribute to varied local-scale forest responses to future climate change (Staal et al., 2020). It should also be noted that though these uncertainties may hinder our understanding of local-scale forest resilience, the influence of future hydroclimatic changes on forests still constitutes major prediction uncertainties. Therefore, in this study, regardless of how these influences are parameterised or simulated in each ESM, we assume that hydroclimatic estimates projected by the ESMs represent the actual climate.
Of course, this assumption opens us and other studies projecting forest conditions to future climate change to certain limitations. Our ability to project forest–savanna transitions (or any transition) relies on the model's capacity to simulate complex feedbacks. Some models capture complex vegetation–atmosphere interaction, simulating local- and regional-scale feedbacks across time (Ferreira et al., 2011; Jach et al., 2020); others rely on simpler parameterisation (Nof, 2008) (e.g. parameterisation of CO2 fertilisation; Koch et al., 2021). However, caution should be taken to not overgeneralise the functioning of tropical forests just from the analysis presented in this study and also to realise the current potential of ESMs to simulate them (Staal et al., 2020). We believe that by considering simulations from multiple ESMs under different SSP scenarios, we not only highlight the agreements and conflicts between potential transitions but also allow future studies to disentangle vegetation–climate feedbacks and improve the modelling of local-scale interactions (e.g. vegetation's water uptake profile, species response to CO2 fertilisation) in the ESMs.
Classifying terrestrial ecosystems based on empirical and CMIP6-ESM-derived Sr allowed us to assess the future transitions in the rainforest ecosystems. Our findings indicate that the climate projected under the lowest-emission scenarios significantly reduces the risk of rainforest tipping and maximises reversion to a less water-limited state, while the climate projected under the high-emission scenarios has the opposite effect on the forest ecosystem. Specifically, in the Amazon rainforest, the risk of forest-to-savanna transition increases considerably with incremental increases in warming. Conversely, in the Congo, the variation in the transition risk across different emission scenarios is relatively minor.
Notably, our analysis suggests a very limited tipping risk that is “unavoidable” (i.e. regions prone to a forest–savanna transition in all scenarios), and the vast majority of potential transition risks can still be avoided by steering towards a less severe climate scenario, thereby underscoring the critical window of opportunity. Moreover, regions projected to revert to a less water-limited state could potentially become more amenable to restoration and responsive to deforestation prevention efforts. This study highlights the importance of restricting global temperature change below 1.5–2 °C warming relative to the pre-industrial levels to prevent forest tipping risks and provide the best conditions for effective ecosystem stewardship.
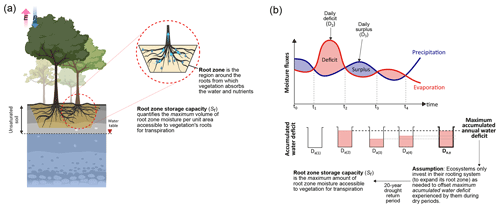
Figure A1The figure illustrates the root zone storage capacity (Sr) of the ecosystem. (a) We show the difference between the ecosystem's root zone and how that constitutes its Sr. (b) Conceptual illustration of how the ecosystem's precipitation and evaporation fluxes constitute the maximum accumulated annual water deficit (Da,y) and Sr. The figure is adopted from Singh (2023a) and Wang-Erlandsson et al. (2016).
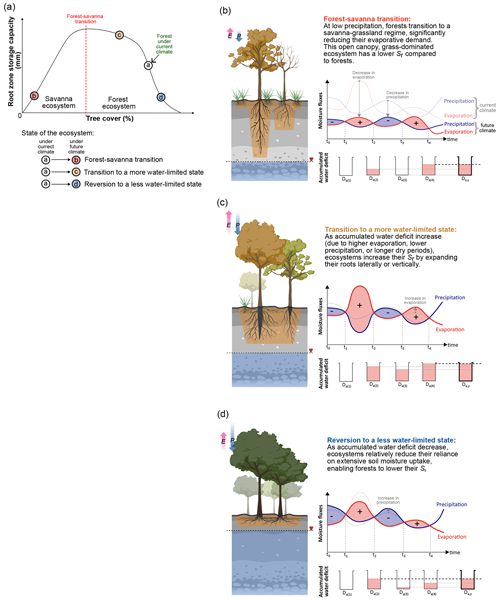
Figure A2(a) The figure compares the root zone storage capacity (Sr) with the ecosystem state (i.e. tree cover). This figure expands on the conceptual illustration from Fig. A1, showing how the ecosystem's precipitation and evaporation fluxes contribute to Sr under different forest transition scenarios, namely (b) forest–savanna transition, (c) transition to a more water-limited state, and (d) reversion to a less water-limited state.
A1 Root zone storage capacity calculation
Our method to calculate Sr is adopted from Singh et al. (2020). For estimating Sr, we first obtained the water deficit (Dt) at a daily time step from the daily estimates of precipitation (Pt) and evaporation (Et) (Fig. A1) using
Here, t denotes the day count since the start of the simulation, with a simulation for each grid starting in the month with maximum precipitation. Second, we calculated the accumulated water deficit integrated at each 1 d time step for 1 year using
where Da(t+1) is the accumulated water deficit at each time step (Fig. A1). Here, an increase in the accumulated water deficit will occur when Et > Pt and a decrease when Et < Pt. However, since this algorithm estimates a running estimate of root zone storage reservoir size, we use a maximum function to calculate the accumulated water deficit, which by definition can never be below zero. Not allowing Da(t+1) to be negative also means that excess moisture from precipitation will either contribute to deep drainage or runoff. Last, the maximum accumulated annual water deficit (Da,y) will represent the maximum storage required by the vegetation to respond to the critical dry periods (Fig. A1).
This simulation runs for a whole year, with n denoting the number of days in year y.
Different terrestrial ecosystems (e.g. forest, savanna, and grassland) adapt to different drought return periods (de Boer-Euser et al., 2016; Gao et al., 2014; Wang-Erlandsson et al., 2016). For instance, grasslands and savannas adapt to shorter drought return periods (i.e. < 10 years and 10–20 years, respectively). In contrast, forests adapt to long drought return periods (> 40 years) (Wang-Erlandsson et al., 2016). For this study, we use a uniform 20-year drought return period (following Bouaziz et al., 2020; Nijzink et al., 2016) to avoid any artificially introduced transitions between different ecosystems. Thus, this 20-year drought return period Sr refers to the maximum amount of root zone moisture accessible to vegetation for transpiration during the largest accumulated annual water deficit expected every 20 years under static climate conditions. We analyse this using the Gumbel extreme value distribution (Gumbel, 1958) and apply it to normalise all Da,y. The Gumbel distribution (F(x)) is given by
where μ and α are the location and scale parameters, respectively. We calculate this using the Python package “skextremes” (skextremes documentation),
where K is the frequency factor given by
and yt is the reduced variate given by
where T is the drought return period (i.e. 20 years used in this study), is the mean annual accumulated deficit for the years 2001–2012, and σn is the standard deviation of the sample. Also, yn is the reduced mean, and Sn is the reduced standard deviation, which for n=11 years (since we are calculating Sr in a hydrological year – and the simulation starts mid-year – we therefore lose 1 year) is equal to 0.4996 and 0.9676, respectively (Gumbel, 1958).
Since the CMIP6 (CMIP6 historical and CMIP6 SSP estimates; the time frames considered are 2000–2014 and 2086–2100, respectively) does not have daily estimates of evaporation and precipitation for all Earth system models (ESMs), we directly use the monthly estimates of precipitation and evaporation to modify Eq. (A1) as
Here, t(monthly) denotes the month count since the start of the simulation. The rest of the steps (Eqs. A2–7) remain the same for CMIP6 datasets. For CMIP6 runs, yn and Sn in Eq. (6) are calculated for n=14 years (Eq. A7) equal to 0.5100 and 1.0095, respectively. The Sr estimates derived from daily and monthly empirical estimates (from Eqs. A1 and A8) are compared in Fig. S8 to evaluate uncertainty.
A2 Abiotic and biotic factors influence soil moisture availability
In this study, Sr quantifies the hydrological buffer necessary for an ecosystem to maintain its structure and functions, reflecting the amount of root zone soil moisture available to vegetation for transpiration. Our mass-balance-based Sr methodology, while not directly distinguishing between the biotic and abiotic influences on soil moisture and root characteristics, does incorporate their critical role in shaping the ecohydrology of the ecosystem under climate change. By utilising empirical precipitation and evaporation data, our approach theoretically captures the combined impact of these biotic and abiotic factors on the actual hydrological regime (including soil moisture) of the ecosystem (Sect. 2.3.2).
We acknowledge that abiotic factors such as soil texture, structure, and depth profoundly affect soil water-holding capacity (Fayos, 1997). For instance, field studies suggest that clay- and organic-rich soils exhibit superior water retention capabilities due to their fine textures and high surface areas, which are crucial to vegetation for moisture uptake during extended dry periods (Bronick and Lal, 2005; Fayos, 1997). Additionally, the depth and porosity of soil also dictate its ability to absorb and store water in the soil, with deeper less-compacted soils providing a higher buffer against drought by allowing greater water infiltration (Indoria et al., 2020; Smith et al., 2001). By altering temperature and precipitation patterns, climate change can modify these abiotic soil properties, potentially leading to a loss in the soil water retention capacity through erosion and compaction (Dexter, 2004).
Moreover, biotic factors, including plant–root dynamics and microbial activity, also play essential roles in shaping the ecosystem (Brunner et al., 2015; Sveen et al., 2024). Deep and extensive root systems not only directly improve access to deeper soil moisture but also physically modify the soil to enhance its permeability and storage (Canadell et al., 1996; Jackson et al., 1996). Additionally, microbial processes contribute by breaking down organic matter, thereby improving the soil's structural integrity and ability to retain water (Dittert et al., 2006). These biotic interactions, coupled with changing abiotic factors under climate change, underscore the complex dynamics that govern soil moisture availability and ecosystem resilience. However, this study does not consider the direct impact of future climate change on biotic and abiotic factors, or their influence on ecosystems, beyond changes to Sr.
A3 Using precipitation to discern savanna from forests under future climate change
Under future climate change, some ecosystems will remain forests, while others may transition to savanna. In our Sr-based framework, without information about above-ground forest structure, it is difficult to discern whether an ecosystem is a forest or savanna just with Sr (for instance, an ecosystem with Sr of 200 mm can either be a moderately water-limited forest or savanna; Sect. 2.3.2). Differentiating these ecosystems is easier under the current climate, where we have several remote-sensing products capturing vegetation structure (e.g. tree cover density, tree height, and floristic patterns) (Aleman et al., 2020; Hirota et al., 2011; Xu et al., 2016). However, under future climate, we must find a proxy, since land-use information in ESMs is prescribed (i.e. not biophysically simulated) (Ma et al., 2020).
To address this, previous studies have either relied on vegetation structure proxies provided by ESMs (e.g. net primary productivity) (Boulton et al., 2013; Jones et al., 2009) or assumed that terrestrial ecosystems are in equilibrium with their climate (Staal et al., 2020) (see the Supplement). In this study, we adopted the latter approach and utilised climate variables, specifically (bias-corrected) mean annual precipitation and the precipitation seasonality index, as proxies to make this distinction (Fig. S4). The climate conditions (or range) necessary for forest ecosystems to sustain themselves are determined by comparing empirical estimates of mean annual precipitation and precipitation seasonality index with Sr. These estimates are then bias-corrected (following the same methods described in Sect. 2.3.3) before applying them to future climate scenarios. This (revised) classification of terrestrial ecosystems is then used to assess forest transitions under future climate change scenarios.
B1 Sensitivity analysis reveals robust performance of the framework
Sensitivity analysis reveals that by setting an extreme Sr threshold – signifying a forest–savanna transition for ecosystems that cannot maintain their above-ground structure at high Sr – we observe some shifts near the already projected risk regions and coastal areas (Figs. 3 and S18). However, the transition risk identified in the coastal regions may be an artefact of interpolating hydroclimate estimates to higher resolution. Additionally, since evaporation is more prevalent over oceans than land, this could result in high-Sr values, thereby projecting an elevated tipping risk in these coastal areas.
We also discover that variations in the evaporation datasets and return periods used for calculating Sr have a minimal effect on forest transitions (Figs. S19 and S20). Although the forest classification thresholds may shift with different evaporation products under current climate conditions (Singh et al., 2020), our histogram equivalence method ensures that forest classifications under future climates adjust accordingly, resulting in only minor alterations to the final outcome (Figs. 1b and S19). Furthermore, while Sr values tend to increase with increase with shorter return periods, the impact of these changes becomes less significant with longer return periods (Wang-Erlandsson et al., 2016), leading to minor variations in the end results (Fig. S20).
Moreover, lowering the forest–savanna transition thresholds can reduce the risk of forest–savanna transition since it expands the associated range of climate conditions (i.e. mean annual precipitation and seasonality) necessary for forests to sustain their structure and functions (Fig. S21). Conversely, increasing the forest–savanna transition threshold leads to an opposite trend, where the risk of transition increases (Fig. S22). Despite these sensitivity analyses, the variation in transition magnitudes is minor, and the trends across different SSP scenarios for both continents remain consistent (Figs. 2 and S18–22). Therefore, the conclusions drawn from this study remain robust, even with variations in factors that could potentially affect forest transitions.
The Python language scripts used for the analyses presented in this study are available from GitHub at https://github.com/chandrakant6492/Future-forest-transitions-CMIP6 (Singh, 2023b). The Python language code for calculating (empirical) root zone storage capacity is available from GitHub at https://github.com/chandrakant6492/Drought-coping-strategy (Singh et al., 2020).
All the data generated during this study are made publicly available on Zenodo at https://doi.org/10.5281/zenodo.7845439 (Singh, 2023c). Other datasets that support the findings of this study are publicly available at https://aims2.llnl.gov/ (last access: 7 February 2023, CMIP6; citations referred to in Table S2), https://github.com/chandrakant6492/Drought-coping-strategy (Singh et al., 2020) (root zone storage capacity; empirical), https://data.chc.ucsb.edu/products/CHIRPS-2.0/ (Funk et al., 2015) (P-CHIRPS), ftp://147.46.64.183/ (Jiang and Ryu, 2016) (E-BESS), ftp://ftp.bgc-jena.mpg.de (Jung et al., 2019) (E-FLUXCOM), https://doi.org/10.4225/08/5719A5C48DB85 (Zhang et al., 2016) (E-PML), https://doi.org/10.24381/cds.adbb2d47 (Hersbach et al., 2023) (E-ERA5), and http://due.esrin.esa.int/page_globcover.php (GlobCover land-use map, 2022). Potential transitions for each ESM based on the comparison between empirical (2001–2012) and SSP (2086–2100) scenarios are presented in the Supplement.
The supplement related to this article is available online at: https://doi.org/10.5194/esd-15-1543-2024-supplement.
All authors contributed to the conceptualization and methodology development of this research. CS conducted the formal analysis, investigation, and visualization. The original draft was written and prepared by CS, with inputs from RvdE, IF, and LWE. All authors participated in reviewing and editing the final paper.
At least one of the (co-)authors is a member of the editorial board of Earth System Dynamics. The peer-review process was guided by an independent editor, and the authors also have no other competing interests to declare.
Publisher's note: Copernicus Publications remains neutral with regard to jurisdictional claims made in the text, published maps, institutional affiliations, or any other geographical representation in this paper. While Copernicus Publications makes every effort to include appropriate place names, the final responsibility lies with the authors.
This article is part of the special issue “Tipping points in the Anthropocene”. It is a result of the “Tipping Points: From Climate Crisis to Positive Transformation” international conference hosted by the Global Systems Institute (GSI) and University of Exeter (12–14 September 2022), as well as the associated creation of a Tipping Points Research Alliance by GSI and the Potsdam Institute for Climate Research, Exeter, Great Britain, 12–14 September 2022.
Chandrakant Singh, Ingo Fetzer, and Lan Wang-Erlandsson acknowledge funding support from the European Research Council (ERC) project “Earth Resilience in the Anthropocene” (project no. ERC-2016-ADG-743080). Lan Wang-Erlandsson also acknowledges funding support from the Swedish Research Council for Sustainable Development (Formas; project no. 2019-01220) and the IKEA Foundation. Ruud van der Ent acknowledges funding support from the Dutch Research Council (NWO) (project no. 016.Veni.181.015). The authors also acknowledge the computational support provided by the Microsoft Planetary Computer (https://planetarycomputer.microsoft.com, last access: 22 June 2023) for performing the analyses.
This research has been supported by the H2020 European Research Council (grant no. ERC-2016-ADG-743080), the Svenska Forskningsrådet Formas (grant no. 2019-01220), and the Nederlandse Organisatie voor Wetenschappelijk Onderzoek (grant no. 016.Veni.181.015).
The publication of this article was funded by the Swedish Research Council, Forte, Formas, and Vinnova.
This paper was edited by Anping Chen and reviewed by two anonymous referees.
Ahlström, A., Canadell, J. G., Schurgers, G., Wu, M., Berry, J. A., Guan, K., and Jackson, R. B.: Hydrologic resilience and Amazon productivity, Nat. Commun., 8, 387, https://doi.org/10.1038/s41467-017-00306-z, 2017.
Albasha, R., Mailhol, J.-C., and Cheviron, B.: Compensatory uptake functions in empirical macroscopic root water uptake models – Experimental and numerical analysis, Agr. Water Manage., 155, 22–39, https://doi.org/10.1016/j.agwat.2015.03.010, 2015.
Aleman, J. C., Fayolle, A., Favier, C., Staver, A. C., Dexter, K. G., Ryan, C. M., Azihou, A. F., Bauman, D., Beest, M. te, Chidumayo, E. N., Comiskey, J. A., Cromsigt, J. P. G. M., Dessard, H., Doucet, J.-L., Finckh, M., Gillet, J.-F., Gourlet-Fleury, S., Hempson, G. P., Holdo, R. M., Kirunda, B., Kouame, F. N., Mahy, G., Gonçalves, F. M. P., McNicol, I., Quintano, P. N., Plumptre, A. J., Pritchard, R. C., Revermann, R., Schmitt, C. B., Swemmer, A. M., Talila, H., Woollen, E., and Swaine, M. D.: Floristic evidence for alternative biome states in tropical Africa, P. Natl. Acad. Sci. USA, 117, 28183–28190, https://doi.org/10.1073/pnas.2011515117, 2020.
Anderegg, W. R. L., Klein, T., Bartlett, M., Sack, L., Pellegrini, A. F. A., Choat, B., and Jansen, S.: Meta-analysis reveals that hydraulic traits explain cross-species patterns of drought-induced tree mortality across the globe, P. Natl. Acad. Sci. USA, 113, 5024–5029, https://doi.org/10.1073/pnas.1525678113, 2016.
Armstrong McKay, D. I., Staal, A., Abrams, J. F., Winkelmann, R., Sakschewski, B., Loriani, S., Fetzer, I., Cornell, S. E., Rockström, J., and Lenton, T. M.: Exceeding 1.5 °C global warming could trigger multiple climate tipping points, Science, 377, eabn7950, https://doi.org/10.1126/science.abn7950, 2022.
Arora, V. K., Seiler, C., Wang, L., and Kou-Giesbrecht, S.: Towards an ensemble-based evaluation of land surface models in light of uncertain forcings and observations, Biogeosciences, 20, 1313–1355, https://doi.org/10.5194/bg-20-1313-2023, 2023.
Baker, J. C. A., Garcia-Carreras, L., Buermann, W., Souza, D. C. de, Marsham, J. H., Kubota, P. Y., Gloor, M., Coelho, C. A. S., and Spracklen, D. V.: Robust Amazon precipitation projections in climate models that capture realistic land–atmosphere interactions, Environ. Res. Lett., 16, 074002, https://doi.org/10.1088/1748-9326/abfb2e, 2021.
Barros, F. de V., Bittencourt, P. R. L., Brum, M., Restrepo-Coupe, N., Pereira, L., Teodoro, G. S., Saleska, S. R., Borma, L. S., Christoffersen, B. O., Penha, D., Alves, L. F., Lima, A. J. N., Carneiro, V. M. C., Gentine, P., Lee, J.-E., Aragão, L. E. O. C., Ivanov, V., Leal, L. S. M., Araujo, A. C., and Oliveira, R. S.: Hydraulic traits explain differential responses of Amazonian forests to the 2015 El Niño-induced drought, New Phytol., 223, 1253–1266, https://doi.org/10.1111/nph.15909, 2019.
Bauman, D., Fortunel, C., Delhaye, G., Malhi, Y., Cernusak, L. A., Bentley, L. P., Rifai, S. W., Aguirre-Gutiérrez, J., Menor, I. O., Phillips, O. L., McNellis, B. E., Bradford, M., Laurance, S. G. W., Hutchinson, M. F., Dempsey, R., Santos-Andrade, P. E., Ninantay-Rivera, H. R., Chambi Paucar, J. R., and McMahon, S. M.: Tropical tree mortality has increased with rising atmospheric water stress, Nature, 608, 528–533, https://doi.org/10.1038/s41586-022-04737-7, 2022.
Bouaziz, L. J. E., Steele-Dunne, S. C., Schellekens, J., Weerts, A. H., Stam, J., Sprokkereef, E., Winsemius, H. H. C., Savenije, H. H. G., and Hrachowitz, M.: Improved Understanding of the Link Between Catchment-Scale Vegetation Accessible Storage and Satellite-Derived Soil Water Index, Water Resour. Res., 56, e2019WR026365, https://doi.org/10.1029/2019WR026365, 2020.
Boulton, C. A., Good, P., and Lenton, T. M.: Early warning signals of simulated Amazon rainforest dieback, Theor. Ecol., 6, 373–384, https://doi.org/10.1007/s12080-013-0191-7, 2013.
Boulton, C. A., Booth, B. B. B., and Good, P.: Exploring uncertainty of Amazon dieback in a perturbed parameter Earth system ensemble, Glob. Change Biol., 23, 5032–5044, https://doi.org/10.1111/gcb.13733, 2017.
Boulton, C. A., Lenton, T. M., and Boers, N.: Pronounced loss of Amazon rainforest resilience since the early 2000s, Nat. Clim. Change, 12, 271–278, https://doi.org/10.1038/s41558-022-01287-8, 2022.
Bovolo, C. I., Wagner, T., Parkin, G., Hein-Griggs, D., Pereira, R., and Jones, R.: The Guiana Shield rainforests – overlooked guardians of South American climate, Environ. Res. Lett., 13, 074029, https://doi.org/10.1088/1748-9326/aacf60, 2018.
Brienen, R. J. W., Phillips, O. L., Feldpausch, T. R., Gloor, E., Baker, T. R., Lloyd, J., Lopez-Gonzalez, G., Monteagudo-Mendoza, A., Malhi, Y., Lewis, S. L., Vásquez Martinez, R., Alexiades, M., Álvarez Dávila, E., Alvarez-Loayza, P., Andrade, A., Aragão, L. E. O. C., Araujo-Murakami, A., Arets, E. J. M. M., Arroyo, L., Aymard C., G. A., Bánki, O. S., Baraloto, C., Barroso, J., Bonal, D., Boot, R. G. A., Camargo, J. L. C., Castilho, C. V., Chama, V., Chao, K. J., Chave, J., Comiskey, J. A., Cornejo Valverde, F., da Costa, L., de Oliveira, E. A., Di Fiore, A., Erwin, T. L., Fauset, S., Forsthofer, M., Galbraith, D. R., Grahame, E. S., Groot, N., Hérault, B., Higuchi, N., Honorio Coronado, E. N., Keeling, H., Killeen, T. J., Laurance, W. F., Laurance, S., Licona, J., Magnussen, W. E., Marimon, B. S., Marimon-Junior, B. H., Mendoza, C., Neill, D. A., Nogueira, E. M., Núñez, P., Pallqui Camacho, N. C., Parada, A., Pardo-Molina, G., Peacock, J., Peña-Claros, M., Pickavance, G. C., Pitman, N. C. A., Poorter, L., Prieto, A., Quesada, C. A., Ramírez, F., Ramírez-Angulo, H., Restrepo, Z., Roopsind, A., Rudas, A., Salomão, R. P., Schwarz, M., Silva, N., Silva-Espejo, J. E., Silveira, M., Stropp, J., Talbot, J., ter Steege, H., Teran-Aguilar, J., Terborgh, J., Thomas-Caesar, R., Toledo, M., Torello-Raventos, M., Umetsu, R. K., van der Heijden, G. M. F., van der Hout, P., Guimarães Vieira, I. C., Vieira, S. A., Vilanova, E., Vos, V. A., and Zagt, R. J.: Long-term decline of the Amazon carbon sink, Nature, 519, 344–348, https://doi.org/10.1038/nature14283, 2015.
Bronick, C. J. and Lal, R.: Soil structure and management: a review, Geoderma, 124, 3–22, https://doi.org/10.1016/j.geoderma.2004.03.005, 2005.
Brooks, P. D., Chorover, J., Fan, Y., Godsey, S. E., Maxwell, R. M., McNamara, J. P., and Tague, C.: Hydrological partitioning in the critical zone: Recent advances and opportunities for developing transferable understanding of water cycle dynamics, Water Resour. Res., 51, 6973–6987, https://doi.org/10.1002/2015WR017039, 2015.
Brum, M., Vadeboncoeur, M. A., Ivanov, V., Asbjornsen, H., Saleska, S., Alves, L. F., Penha, D., Dias, J. D., Aragão, L. E. O. C., Barros, F., Bittencourt, P., Pereira, L., and Oliveira, R. S.: Hydrological niche segregation defines forest structure and drought tolerance strategies in a seasonal Amazon forest, J. Ecol., 107, 318–333, https://doi.org/10.1111/1365-2745.13022, 2019.
Brunner, I., Herzog, C., Dawes, M. A., Arend, M., and Sperisen, C.: How tree roots respond to drought, Front. Plant Sci., 6, 547, https://doi.org/10.3389/fpls.2015.00547, 2015.
Bruno, R. D., da Rocha, H. R., de Freitas, H. C., Goulden, M. L., and Miller, S. D.: Soil moisture dynamics in an eastern Amazonian tropical forest, Hydrol. Process., 20, 2477–2489, https://doi.org/10.1002/hyp.6211, 2006.
Canadell, J., Jackson, R. B., Ehleringer, J. B., Mooney, H. A., Sala, O. E., and Schulze, E.-D.: Maximum rooting depth of vegetation types at the global scale, Oecologia, 108, 583–595, https://doi.org/10.1007/BF00329030, 1996.
Canadell, J. G., Monteiro, P. M. S., Costa, M. H., Cunha, L. C. D., Cox, P. M., Eliseev, A. V., Henson, S., Ishii, M., Jaccard, S., Koven, C., Lohila, A., Patra, P. K., Piao, S., Syampungani, S., Zaehle, S., Zickfeld, K., Alexandrov, G. A., Bala, G., Bopp, L., Boysen, L., Cao, L., Chandra, N., Ciais, P., Denisov, S. N., Dentener, F. J., Douville, H., Fay, A., Forster, P., Fox-Kemper, B., Friedlingstein, P., Fu, W., Fuss, S., Garçon, V., Gier, B., Gillett, N. P., Gregor, L., Haustein, K., Haverd, V., He, J., Hewitt, H. T., Hoffman, F. M., Ilyina, T., Jackson, R., Jones, C., Keller, D. P., Kwiatkowski, L., Lamboll, R. D., Lan, X., Laufkötter, C., Quéré, C. L., Lenton, A., Lewis, J., Liddicoat, S., Lorenzoni, L., Lovenduski, N., Macdougall, A. H., Mathesius, S., Matthews, D. H., Meinshausen, M., Mokhov, I. I., Naik, V., Nicholls, Z. R. J., Nurhati, I. S., O'sullivan, M., Peters, G., Pongratz, J., Poulter, B., Sallée, J.-B., Saunois, M., Schuur, E. A. G. I., Seneviratne, S., Stavert, A., Suntharalingam, P., Tachiiri, K., Terhaar, J., Thompson, R., Tian, H., Turnbull, J., Vicente-Serrano, S. M., Wang, X., Wanninkhof, R. H., Williamson, P., Brovkin, V., Feely, R. A., and Lebehot, A. D.: Global Carbon and other Biogeochemical Cycles and Feedbacks, in: Climate Change 2021: The Physical Science Basis. Contribution of Working Group I to the Sixth Assessment Report of the Intergovernmental Panel on Climate Change, edited by: Masson-Delmotte, V., Zhai, P., Pirani, A., Connors, S. L., Péan, C., Berger, S., Caud, N., Chen, Y., Goldfarb, L., Gomis, M. I., Huang, M., Leitzell, K., Lonnoy, E., Matthews, J. B. R., Maycock, T. K., Waterfield, T., Yelekçi, O., Yu, R., and Zhou, B., Cambridge University Press, Cambridge, United Kingdom and New York, NY, USA, 673–816, https://doi.org/10.1017/9781009157896.007, 2021.
Chai, Y., Martins, G., Nobre, C., von Randow, C., Chen, T., and Dolman, H.: Constraining Amazonian land surface temperature sensitivity to precipitation and the probability of forest dieback, npj Clim. Atmos. Sci., 4, 1–7, https://doi.org/10.1038/s41612-021-00162-1, 2021.
Cheng, S., Huang, J., Ji, F., and Lin, L.: Uncertainties of soil moisture in historical simulations and future projections, J. Geophys. Res.-Atmos., 122, 2239–2253, https://doi.org/10.1002/2016JD025871, 2017.
Cole, L. E. S., Bhagwat, S. A., and Willis, K. J.: Recovery and resilience of tropical forests after disturbance, Nat. Commun., 5, 3906, https://doi.org/10.1038/ncomms4906, 2014.
Condit, R., Engelbrecht, B. M. J., Pino, D., Pérez, R., and Turner, B. L.: Species distributions in response to individual soil nutrients and seasonal drought across a community of tropical trees, P. Natl. Acad. Sci. USA, 110, 5064–5068, https://doi.org/10.1073/pnas.1218042110, 2013.
Cook, K. H. and Vizy, E. K.: Impact of climate change on mid-twenty-first century growing seasons in Africa, Clim. Dynam., 39, 2937–2955, https://doi.org/10.1007/s00382-012-1324-1, 2012.
Cooper, G. S., Willcock, S., and Dearing, J. A.: Regime shifts occur disproportionately faster in larger ecosystems, Nat. Commun., 11, 1175, https://doi.org/10.1038/s41467-020-15029-x, 2020.
Cox, P. M., Betts, R. A., Collins, M., Harris, P. P., Huntingford, C., and Jones, C. D.: Amazonian forest dieback under climate-carbon cycle projections for the 21st century, Theor. Appl. Climatol., 78, 137–156, https://doi.org/10.1007/s00704-004-0049-4, 2004.
Dai, A.: Drought under global warming: a review, WIREs Clim. Change, 2, 45–65, https://doi.org/10.1002/wcc.81, 2011.
Davidson, E. A., de Araújo, A. C., Artaxo, P., Balch, J. K., Brown, I. F., C. Bustamante, M. M., Coe, M. T., DeFries, R. S., Keller, M., Longo, M., Munger, J. W., Schroeder, W., Soares-Filho, B. S., Souza, C. M., and Wofsy, S. C.: The Amazon basin in transition, Nature, 481, 321–328, https://doi.org/10.1038/nature10717, 2012.
de Boer-Euser, T., McMillan, H. K., Hrachowitz, M., Winsemius, H. C., and Savenije, H. H. G.: Influence of soil and climate on root zone storage capacity, Water Resour. Res., 52, 2009–2024, https://doi.org/10.1002/2015WR018115, 2016.
Dexter, A. R.: Soil physical quality: Part II. Friability, tillage, tilth and hard-setting, Geoderma, 120, 215–225, https://doi.org/10.1016/j.geoderma.2003.09.005, 2004.
Dittert, K., Wätzel, J., and Sattelmacher, B.: Responses of Alnus glutinosa to Anaerobic Conditions – Mechanisms and Rate of Oxygen Flux into the Roots, Plant Biol., 8, 212–223, https://doi.org/10.1055/s-2005-873041, 2006.
Doughty, C. E., Keany, J. M., Wiebe, B. C., Rey-Sanchez, C., Carter, K. R., Middleby, K. B., Cheesman, A. W., Goulden, M. L., da Rocha, H. R., Miller, S. D., Malhi, Y., Fauset, S., Gloor, E., Slot, M., Oliveras Menor, I., Crous, K. Y., Goldsmith, G. R., and Fisher, J. B.: Tropical forests are approaching critical temperature thresholds, Nature, 621, 105–111, https://doi.org/10.1038/s41586-023-06391-z, 2023.
Drijfhout, S., Bathiany, S., Beaulieu, C., Brovkin, V., Claussen, M., Huntingford, C., Scheffer, M., Sgubin, G., and Swingedouw, D.: Catalogue of abrupt shifts in Intergovernmental Panel on Climate Change climate models, P. Natl. Acad. Sci. USA, 112, E5777–E5786, https://doi.org/10.1073/pnas.1511451112, 2015.
Dunning, C. M., Black, E., and Allan, R. P.: Later Wet Seasons with More Intense Rainfall over Africa under Future Climate Change, J. Clim., 31, 9719–9738, 2018.
Esquivel-Muelbert, A., Baker, T. R., Dexter, K. G., Lewis, S. L., Brienen, R. J. W., Feldpausch, T. R., Lloyd, J., Monteagudo-Mendoza, A., Arroyo, L., Álvarez-Dávila, E., Higuchi, N., Marimon, B. S., Marimon-Junior, B. H., Silveira, M., Vilanova, E., Gloor, E., Malhi, Y., Chave, J., Barlow, J., Bonal, D., Cardozo, N. D., Erwin, T., Fauset, S., Hérault, B., Laurance, S., Poorter, L., Qie, L., Stahl, C., Sullivan, M. J. P., Steege, H. ter, Vos, V. A., Zuidema, P. A., Almeida, E., Oliveira, E. A. de, Andrade, A., Vieira, S. A., Aragão, L., Araujo-Murakami, A., Arets, E., C, G. A. A., Baraloto, C., Camargo, P. B., Barroso, J. G., Bongers, F., Boot, R., Camargo, J. L., Castro, W., Moscoso, V. C., Comiskey, J., Valverde, F. C., Costa, A. C. L. da, Pasquel, J. del A., Fiore, A. D., Duque, L. F., Elias, F., Engel, J., Llampazo, G. F., Galbraith, D., Fernández, R. H., Coronado, E. H., Hubau, W., Jimenez-Rojas, E., Lima, A. J. N., Umetsu, R. K., Laurance, W., Lopez-Gonzalez, G., Lovejoy, T., Cruz, O. A. M., Morandi, P. S., Neill, D., Vargas, P. N., Camacho, N. C. P., Gutierrez, A. P., Pardo, G., Peacock, J., Peña-Claros, M., Peñuela-Mora, M. C., Petronelli, P., Pickavance, G. C., Pitman, N., Prieto, A., Quesada, C., Ramírez-Angulo, H., Réjou-Méchain, M., Correa, Z. R., Roopsind, A., Rudas, A., Salomão, R., Silva, N., Espejo, J. S., Singh, J., Stropp, J., Terborgh, J., Thomas, R., Toledo, M., Torres-Lezama, A., Gamarra, L. V., van de Meer, P. J., van der Heijden, G., van der Hout, P., Vasquez Martinez, R., Vela, C., Vieira, I. C. G., and Phillips, O. L.: Compositional response of Amazon forests to climate change, Glob. Change Biol., 25, 39–56, https://doi.org/10.1111/gcb.14413, 2019.
Fan, Y., Miguez-Macho, G., Jobbágy, E. G., Jackson, R. B., and Otero-Casal, C.: Hydrologic regulation of plant rooting depth, P. Natl. Acad. Sci. USA, 114, 10572–10577, https://doi.org/10.1073/pnas.1712381114, 2017.
Fayos, C. B.: The roles of texture and structure in the water retention capacity of burnt Mediterranean soils with varying rainfall, CATENA, 31, 219–236, https://doi.org/10.1016/S0341-8162(97)00041-6, 1997.
February, E. C. and Higgins, S. I.: The distribution of tree and grass roots in savannas in relation to soil nitrogen and water, S. Afr. J. Bot., 76, 517–523, https://doi.org/10.1016/j.sajb.2010.04.001, 2010.
Ferreira, D., Marshall, J., and Rose, B.: Climate Determinism Revisited: Multiple Equilibria in a Complex Climate Model, J. Clim., 24, 992–1012, https://doi.org/10.1175/2010JCLI3580.1, 2011.
Fleischer, K., Rammig, A., De Kauwe, M. G., Walker, A. P., Domingues, T. F., Fuchslueger, L., Garcia, S., Goll, D. S., Grandis, A., Jiang, M., Haverd, V., Hofhansl, F., Holm, J. A., Kruijt, B., Leung, F., Medlyn, B. E., Mercado, L. M., Norby, R. J., Pak, B., von Randow, C., Quesada, C. A., Schaap, K. J., Valverde-Barrantes, O. J., Wang, Y.-P., Yang, X., Zaehle, S., Zhu, Q., and Lapola, D. M.: Amazon forest response to CO2 fertilization dependent on plant phosphorus acquisition, Nat. Geosci., 12, 736–741, https://doi.org/10.1038/s41561-019-0404-9, 2019.
Flores, B. M., Montoya, E., Sakschewski, B., Nascimento, N., Staal, A., Betts, R. A., Levis, C., Lapola, D. M., Esquível-Muelbert, A., Jakovac, C., Nobre, C. A., Oliveira, R. S., Borma, L. S., Nian, D., Boers, N., Hecht, S. B., ter Steege, H., Arieira, J., Lucas, I. L., Berenguer, E., Marengo, J. A., Gatti, L. V., Mattos, C. R. C., and Hirota, M.: Critical transitions in the Amazon forest system, Nature, 626, 555–564, https://doi.org/10.1038/s41586-023-06970-0, 2024.
Funk, C., Peterson, P., Landsfeld, M., Pedreros, D., Verdin, J., Shukla, S., Husak, G., Rowland, J., Harrison, L., Hoell, A., and Michaelsen, J.: The climate hazards infrared precipitation with stations – a new environmental record for monitoring extremes, Sci. Data, 2, 150066, https://doi.org/10.1038/sdata.2015.66, 2015 (data available at: https://data.chc.ucsb.edu/products/CHIRPS-2.0/, last access: 18 January 2023).
Gao, H., Hrachowitz, M., Schymanski, S. J., Fenicia, F., Sriwongsitanon, N., and Savenije, H. H. G.: Climate controls how ecosystems size the root zone storage capacity at catchment scale: Root zone storage capacity in catchments, Geophys. Res. Lett., 41, 7916–7923, https://doi.org/10.1002/2014GL061668, 2014.
GlobCover land-use map: Global Land Cover Service, http://due.esrin.esa.int/page_globcover.php, last access: 27 February 2022.
Grimm, N. B., Chapin III, F. S., Bierwagen, B., Gonzalez, P., Groffman, P. M., Luo, Y., Melton, F., Nadelhoffer, K., Pairis, A., Raymond, P. A., Schimel, J., and Williamson, C. E.: The impacts of climate change on ecosystem structure and function, Front. Ecol. Environ., 11, 474–482, https://doi.org/10.1890/120282, 2013.
Gumbel, E. J.: Statistics of extremes, Columbia University Press, New York, 1958.
Guswa, A. J.: The influence of climate on root depth: A carbon cost-benefit analysis, Water Resour. Res., 44, W02427, https://doi.org/10.1029/2007WR006384, 2008.
Hahm, W. J., Rempe, D. M., Dralle, D. N., Dawson, T. E., Lovill, S. M., Bryk, A. B., Bish, D. L., Schieber, J., and Dietrich, W. E.: Lithologically Controlled Subsurface Critical Zone Thickness and Water Storage Capacity Determine Regional Plant Community Composition, Water Resour. Res., 55, 3028–3055, https://doi.org/10.1029/2018WR023760, 2019.
Hall, A., Cox, P., Huntingford, C., and Klein, S.: Progressing emergent constraints on future climate change, Nat. Clim. Change, 9, 269–278, https://doi.org/10.1038/s41558-019-0436-6, 2019.
Hersbach, H., Bell, B., Berrisford, P., Hirahara, S., Horányi, A., Muñoz-Sabater, J., Nicolas, J., Peubey, C., Radu, R., Schepers, D., Simmons, A., Soci, C., Abdalla, S., Abellan, X., Balsamo, G., Bechtold, P., Biavati, G., Bidlot, J., Bonavita, M., Chiara, G. D., Dahlgren, P., Dee, D., Diamantakis, M., Dragani, R., Flemming, J., Forbes, R., Fuentes, M., Geer, A., Haimberger, L., Healy, S., Hogan, R. J., Hólm, E., Janisková, M., Keeley, S., Laloyaux, P., Lopez, P., Lupu, C., Radnoti, G., Rosnay, P. de, Rozum, I., Vamborg, F., Villaume, S., and Thépaut, J.-N.: The ERA5 Global Reanalysis, Q. J. Roy. Meteorol. Soc., 245, 111840, https://doi.org/10.1002/qj.3803, 2020.
Hersbach, H., Bell, B., Berrisford, P., Biavati, G., Horányi, A., Muñoz Sabater, J., Nicolas, J., Peubey, C., Radu, R., Rozum, I., Schepers, D., Simmons, A., Soci, C., Dee, D., and Thépaut, J.-N.: ERA5 hourly data on single levels from 1940 to present, Copernicus Climate Change Service (C3S) Climate Data Store (CDS) [data set], https://doi.org/10.24381/cds.adbb2d47, 2023.
Higgins, S. I. and Scheiter, S.: Atmospheric CO2 forces abrupt vegetation shifts locally, but not globally, Nature, 488, 209–212, https://doi.org/10.1038/nature11238, 2012.
Hildebrandt, A., Kleidon, A., and Bechmann, M.: A thermodynamic formulation of root water uptake, Hydrol. Earth Syst. Sci., 20, 3441–3454, https://doi.org/10.5194/hess-20-3441-2016, 2016.
Hirota, M., Holmgren, M., Van Nes, E. H., and Scheffer, M.: Global Resilience of Tropical Forest and Savanna to Critical Transitions, Science, 334, 232–235, https://doi.org/10.1126/science.1210657, 2011.
Hirota, M., Flores, B. M., Betts, R., Borma, L. S., Esquivel-Muelbert, A., Jakovac, C., Lapola, D. M., Montoya, E., Oliveira, R. S., and Sakschewski, B.: Chapter 24: Resilience of the Amazon forest to global changes: Assessing the risk of tipping points, in: Amazon Assessment Report 2021, edited by: Nobre, C., Encalada, A., Anderson, E., Roca Alcazar, F. H., Bustamante, M., Mena, C., Peña-Claros, M., Poveda, G., Rodriguez, J. P., Saleska, S., Trumbore, S. E., Val, A., Villa Nova, L., Abramovay, R., Alencar, A., Rodriguez Alzza, A. C., Armenteras, D., Artaxo, P., Athayde, S., Barretto Filho, H. T., Barlow, J., Berenguer, E., Bortolotto, F., Costa, F. de A., Costa, M. H., Cuvi, N., Fearnside, P., Ferreira, J., Flores, B. M., Frieri, S., Gatti, L. V., Guayasamin, J. M., Hecht, S., Hirota, M., Hoorn, C., Josse, C., Lapola, D. M., Larrea, C., Larrea-Alcazar, D. M., Lehm Ardaya, Z., Malhi, Y., Marengo, J. A., Melack, J., Moraes R., M., Moutinho, P., Murmis, M. R., Neves, E. G., Paez, B., Painter, L., Ramos, A., Rosero-Peña, M. C., Schmink, M., Sist, P., ter Steege, H., Val, P., van der Voort, H., Varese, M., and Zapata-Ríos, G.: Amazon Assessment Report 2021, UN Sustainable Development Solutions Network (SDSN), https://doi.org/10.55161/QPYS9758, 2021.
Hofhansl, F., Andersen, K. M., Fleischer, K., Fuchslueger, L., Rammig, A., Schaap, K. J., Valverde-Barrantes, O. J., and Lapola, D. M.: Amazon Forest Ecosystem Responses to Elevated Atmospheric CO2 and Alterations in Nutrient Availability: Filling the Gaps with Model-Experiment Integration, Front. Earth Sci., 4, 19, https://doi.org/10.3389/feart.2016.00019, 2016.
Hubau, W., Lewis, S. L., Phillips, O. L., Affum-Baffoe, K., Beeckman, H., Cuní-Sanchez, A., Daniels, A. K., Ewango, C. E. N., Fauset, S., Mukinzi, J. M., Sheil, D., Sonké, B., Sullivan, M. J. P., Sunderland, T. C. H., Taedoumg, H., Thomas, S. C., White, L. J. T., Abernethy, K. A., Adu-Bredu, S., Amani, C. A., Baker, T. R., Banin, L. F., Baya, F., Begne, S. K., Bennett, A. C., Benedet, F., Bitariho, R., Bocko, Y. E., Boeckx, P., Boundja, P., Brienen, R. J. W., Brncic, T., Chezeaux, E., Chuyong, G. B., Clark, C. J., Collins, M., Comiskey, J. A., Coomes, D. A., Dargie, G. C., de Haulleville, T., Kamdem, M. N. D., Doucet, J.-L., Esquivel-Muelbert, A., Feldpausch, T. R., Fofanah, A., Foli, E. G., Gilpin, M., Gloor, E., Gonmadje, C., Gourlet-Fleury, S., Hall, J. S., Hamilton, A. C., Harris, D. J., Hart, T. B., Hockemba, M. B. N., Hladik, A., Ifo, S. A., Jeffery, K. J., Jucker, T., Yakusu, E. K., Kearsley, E., Kenfack, D., Koch, A., Leal, M. E., Levesley, A., Lindsell, J. A., Lisingo, J., Lopez-Gonzalez, G., Lovett, J. C., Makana, J.-R., Malhi, Y., Marshall, A. R., Martin, J., Martin, E. H., Mbayu, F. M., Medjibe, V. P., Mihindou, V., Mitchard, E. T. A., Moore, S., Munishi, P. K. T., Bengone, N. N., Ojo, L., Ondo, F. E., Peh, K. S.-H., Pickavance, G. C., Poulsen, A. D., Poulsen, J. R., Qie, L., Reitsma, J., Rovero, F., Swaine, M. D., Talbot, J., Taplin, J., Taylor, D. M., Thomas, D. W., Toirambe, B., Mukendi, J. T., Tuagben, D., Umunay, P. M., van der Heijden, G. M. F., Verbeeck, H., Vleminckx, J., Willcock, S., Wöll, H., Woods, J. T., and Zemagho, L.: Asynchronous carbon sink saturation in African and Amazonian tropical forests, Nature, 579, 80–87, https://doi.org/10.1038/s41586-020-2035-0, 2020.
Huntingford, C., Zelazowski, P., Galbraith, D., Mercado, L. M., Sitch, S., Fisher, R., Lomas, M., Walker, A. P., Jones, C. D., Booth, B. B. B., Malhi, Y., Hemming, D., Kay, G., Good, P., Lewis, S. L., Phillips, O. L., Atkin, O. K., Lloyd, J., Gloor, E., Zaragoza-Castells, J., Meir, P., Betts, R., Harris, P. P., Nobre, C., Marengo, J., and Cox, P. M.: Simulated resilience of tropical rainforests to CO2-induced climate change, Nat. Geosci., 6, 268–273, https://doi.org/10.1038/ngeo1741, 2013.
Hurtt, G. C., Chini, L., Sahajpal, R., Frolking, S., Bodirsky, B. L., Calvin, K., Doelman, J. C., Fisk, J., Fujimori, S., Klein Goldewijk, K., Hasegawa, T., Havlik, P., Heinimann, A., Humpenöder, F., Jungclaus, J., Kaplan, J. O., Kennedy, J., Krisztin, T., Lawrence, D., Lawrence, P., Ma, L., Mertz, O., Pongratz, J., Popp, A., Poulter, B., Riahi, K., Shevliakova, E., Stehfest, E., Thornton, P., Tubiello, F. N., van Vuuren, D. P., and Zhang, X.: Harmonization of global land use change and management for the period 850–2100 (LUH2) for CMIP6, Geosci. Model Dev., 13, 5425–5464, https://doi.org/10.5194/gmd-13-5425-2020, 2020.
Indoria, A. K., Sharma, K. L., and Reddy, K. S.: Chapter 18 - Hydraulic properties of soil under warming climate, in: Climate Change and Soil Interactions, edited by: Prasad, M. N. V. and Pietrzykowski, M., Elsevier, 473–508, https://doi.org/10.1016/B978-0-12-818032-7.00018-7, 2020.
IPCC: Climate Change 2021 – The Physical Science Basis: Working Group I Contribution to the Sixth Assessment Report of the Intergovernmental Panel on Climate Change, 1st ed., Cambridge University Press, https://doi.org/10.1017/9781009157896, 2023.
Jach, L., Warrach-Sagi, K., Ingwersen, J., Kaas, E., and Wulfmeyer, V.: Land Cover Impacts on Land-Atmosphere Coupling Strength in Climate Simulations With WRF Over Europe, J. Geophys. Res.-Atmos., 125, e2019JD031989, https://doi.org/10.1029/2019JD031989, 2020.
Jackson, R. B., Canadell, J., Ehleringer, J. R., Mooney, H. A., Sala, O. E., and Schulze, E. D.: A global analysis of root distributions for terrestrial biomes, Oecologia, 108, 389–411, https://doi.org/10.1007/BF00333714, 1996.
Jehn, F. U., Kemp, L., Ilin, E., Funk, C., Wang, J. R., and Breuer, L.: Focus of the IPCC Assessment Reports Has Shifted to Lower Temperatures, Earth's Future, 10, e2022EF002876, https://doi.org/10.1029/2022EF002876, 2022.
Jiang, C. and Ryu, Y.: Multi-scale evaluation of global gross primary productivity and evapotranspiration products derived from Breathing Earth System Simulator (BESS), Remote Sens. Environ., 186, 528–547, https://doi.org/10.1016/j.rse.2016.08.030, 2016 (data available at: ftp://147.46.64.183/, last access: 18 January 2023).
Jones, C., Lowe, J., Liddicoat, S., and Betts, R.: Committed terrestrial ecosystem changes due to climate change, Nat. Geosci., 2, 484–487, https://doi.org/10.1038/ngeo555, 2009.
Jung, M., Koirala, S., Weber, U., Ichii, K., Gans, F., Camps-Valls, G., Papale, D., Schwalm, C., Tramontana, G., and Reichstein, M.: The FLUXCOM ensemble of global land-atmosphere energy fluxes, Sci. Data, 6, 74, https://doi.org/10.1038/s41597-019-0076-8, 2019 (data available at: ftp://ftp.bgc-jena.mpg.de, last access: 18 January 2023).
Koch, A., Hubau, W., and Lewis, S. L.: Earth System Models Are Not Capturing Present-Day Tropical Forest Carbon Dynamics, Earth's Future, 9, e2020EF001874, https://doi.org/10.1029/2020EF001874, 2021.
Kooperman, G. J., Chen, Y., Hoffman, F. M., Koven, C. D., Lindsay, K., Pritchard, M. S., Swann, A. L. S., and Randerson, J. T.: Forest response to rising CO2 drives zonally asymmetric rainfall change over tropical land, Nat. Clim. Change, 8, 434–440, https://doi.org/10.1038/s41558-018-0144-7, 2018.
Körner, C.: A matter of tree longevity, Science, 355, 130–131, https://doi.org/10.1126/science.aal2449, 2017.
Küçük, Ç., Koirala, S., Carvalhais, N., Miralles, D. G., Reichstein, M., and Jung, M.: Characterizing the Response of Vegetation Cover to Water Limitation in Africa Using Geostationary Satellites, J. Adv. Model. Earth Sy., 14, e2021MS002730, https://doi.org/10.1029/2021MS002730, 2022.
Kukal, M. S. and Irmak, S.: Can limits of plant available water be inferred from soil moisture distributions?, Agr. Environ. Lett., 8, e20113, https://doi.org/10.1002/ael2.20113, 2023.
Lammertsma, E. I., Boer, H. J. de, Dekker, S. C., Dilcher, D. L., Lotter, A. F., and Wagner-Cremer, F.: Global CO2 rise leads to reduced maximum stomatal conductance in Florida vegetation, P. Natl. Acad. Sci. USA, 108, 4035–4040, https://doi.org/10.1073/pnas.1100371108, 2011.
Leite-Filho, A. T., Soares-Filho, B. S., Davis, J. L., Abrahão, G. M., and Börner, J.: Deforestation reduces rainfall and agricultural revenues in the Brazilian Amazon, Nat. Commun., 12, 2591, https://doi.org/10.1038/s41467-021-22840-7, 2021.
Lenton, T. M.: Early warning of climate tipping points, Nat. Clim. Change, 1, 201–209, https://doi.org/10.1038/nclimate1143, 2011.
Lenton, T. M., Rockström, J., Gaffney, O., Rahmstorf, S., Richardson, K., Steffen, W., and Schellnhuber, H. J.: Climate tipping points – too risky to bet against, Nature, 575, 592–595, https://doi.org/10.1038/d41586-019-03595-0, 2019.
Lewis, S. L., Edwards, D. P., and Galbraith, D.: Increasing human dominance of tropical forests, Science, 349, 827–832, https://doi.org/10.1126/science.aaa9932, 2015.
Li, Y., Brando, P. M., Morton, D. C., Lawrence, D. M., Yang, H., and Randerson, J. T.: Deforestation-induced climate change reduces carbon storage in remaining tropical forests, Nat. Commun., 13, 1964, https://doi.org/10.1038/s41467-022-29601-0, 2022.
Liu, W., Sun, F., Lim, W. H., Zhang, J., Wang, H., Shiogama, H., and Zhang, Y.: Global drought and severe drought-affected populations in 1.5 and 2 °C warmer worlds, Earth Syst. Dynam., 9, 267–283, https://doi.org/10.5194/esd-9-267-2018, 2018.
Liu, Y., Kumar, M., Katul, G. G., Feng, X., and Konings, A. G.: Plant hydraulics accentuates the effect of atmospheric moisture stress on transpiration, Nat. Clim. Change, 10, 691–695, https://doi.org/10.1038/s41558-020-0781-5, 2020.
Ma, L., Hurtt, G. C., Chini, L. P., Sahajpal, R., Pongratz, J., Frolking, S., Stehfest, E., Klein Goldewijk, K., O'Leary, D., and Doelman, J. C.: Global rules for translating land-use change (LUH2) to land-cover change for CMIP6 using GLM2, Geosci. Model Dev., 13, 3203–3220, https://doi.org/10.5194/gmd-13-3203-2020, 2020.
Malhi, Y., Roberts, J. T., Betts, R. A., Killeen, T. J., Li, W., and Nobre, C. A.: Climate Change, Deforestation, and the Fate of the Amazon, Science, 319, 169–172, https://doi.org/10.1126/science.1146961, 2008.
Malhi, Y., Gardner, T. A., Goldsmith, G. R., Silman, M. R., and Zelazowski, P.: Tropical Forests in the Anthropocene, Annu. Rev. Env. Resour., 39, 125–159, https://doi.org/10.1146/annurev-environ-030713-155141, 2014.
Mamalakis, A., Randerson, J. T., Yu, J.-Y., Pritchard, M. S., Magnusdottir, G., Smyth, P., Levine, P. A., Yu, S., and Foufoula-Georgiou, E.: Zonally contrasting shifts of the tropical rain belt in response to climate change, Nat. Clim. Change, 11, 143–151, https://doi.org/10.1038/s41558-020-00963-x, 2021.
Maslin, M. and Austin, P.: Climate models at their limit?, Nature, 486, 183–184, https://doi.org/10.1038/486183a, 2012.
McCormick, E. L., Dralle, D. N., Hahm, W. J., Tune, A. K., Schmidt, L. M., Chadwick, K. D., and Rempe, D. M.: Widespread woody plant use of water stored in bedrock, Nature, 597, 225–229, https://doi.org/10.1038/s41586-021-03761-3, 2021.
McFarlane, N.: Parameterizations: representing key processes in climate models without resolving them, WIREs Clim. Change, 2, 482–497, https://doi.org/10.1002/wcc.122, 2011.
Nepstad, D. C., Verssimo, A., Alencar, A., Nobre, C., Lima, E., Lefebvre, P., Schlesinger, P., Potter, C., Moutinho, P., Mendoza, E., Cochrane, M., and Brooks, V.: Large-scale impoverishment of Amazonian forests by logging and fire, Nature, 398, 505–508, https://doi.org/10.1038/19066, 1999.
Nijzink, R., Hutton, C., Pechlivanidis, I., Capell, R., Arheimer, B., Freer, J., Han, D., Wagener, T., McGuire, K., Savenije, H., and Hrachowitz, M.: The evolution of root-zone moisture capacities after deforestation: a step towards hydrological predictions under change?, Hydrol. Earth Sys. Sci., 20, 4775–4799, https://doi.org/10.5194/hess-20-4775-2016, 2016.
Nippert, J. B. and Holdo, R. M.: Challenging the maximum rooting depth paradigm in grasslands and savannas, Funct. Ecol., 29, 739–745, https://doi.org/10.1111/1365-2435.12390, 2015.
Nof, D.: Simple Versus Complex Climate Modeling, Eos, Trans. Am. Geophys. Union, 89, 544–545, https://doi.org/10.1029/2008EO520006, 2008.
Oliveira, R. S., Dawson, T. E., Burgess, S. S. O., and Nepstad, D. C.: Hydraulic redistribution in three Amazonian trees, Oecologia, 145, 354–363, https://doi.org/10.1007/s00442-005-0108-2, 2005.
Oliveras, I. and Malhi, Y.: Many shades of green: the dynamic tropical forest–savannah transition zones, Philos. T. R. Soc. B, 371, 20150308, https://doi.org/10.1098/rstb.2015.0308, 2016.
Parry, I. M., Ritchie, P. D. L., and Cox, P. M.: Evidence of localised Amazon rainforest dieback in CMIP6 models, Earth Syst. Dynam., 13, 1667–1675, https://doi.org/10.5194/esd-13-1667-2022, 2022.
Pascale, S., Carvalho, L. M. V., Adams, D. K., Castro, C. L., and Cavalcanti, I. F. A.: Current and Future Variations of the Monsoons of the Americas in a Warming Climate, Curr. Clim. Chang. Rep., 5, 125–144, https://doi.org/10.1007/s40641-019-00135-w, 2019.
Piani, C., Weedon, G. P., Best, M., Gomes, S. M., Viterbo, P., Hagemann, S., and Haerter, J. O.: Statistical bias correction of global simulated daily precipitation and temperature for the application of hydrological models, J. Hydrol., 395, 199–215, https://doi.org/10.1016/j.jhydrol.2010.10.024, 2010.
Poorter, L., Bongers, F., Aide, T. M., Almeyda Zambrano, A. M., Balvanera, P., Becknell, J. M., Boukili, V., Brancalion, P. H. S., Broadbent, E. N., Chazdon, R. L., Craven, D., de Almeida-Cortez, J. S., Cabral, G. A. L., de Jong, B. H. J., Denslow, J. S., Dent, D. H., DeWalt, S. J., Dupuy, J. M., Durán, S. M., Espírito-Santo, M. M., Fandino, M. C., César, R. G., Hall, J. S., Hernandez-Stefanoni, J. L., Jakovac, C. C., Junqueira, A. B., Kennard, D., Letcher, S. G., Licona, J.-C., Lohbeck, M., Marín-Spiotta, E., Martínez-Ramos, M., Massoca, P., Meave, J. A., Mesquita, R., Mora, F., Muñoz, R., Muscarella, R., Nunes, Y. R. F., Ochoa-Gaona, S., de Oliveira, A. A., Orihuela-Belmonte, E., Peña-Claros, M., Pérez-García, E. A., Piotto, D., Powers, J. S., Rodríguez-Velázquez, J., Romero-Pérez, I. E., Ruíz, J., Saldarriaga, J. G., Sanchez-Azofeifa, A., Schwartz, N. B., Steininger, M. K., Swenson, N. G., Toledo, M., Uriarte, M., van Breugel, M., van der Wal, H., Veloso, M. D. M., Vester, H. F. M., Vicentini, A., Vieira, I. C. G., Bentos, T. V., Williamson, G. B., and Rozendaal, D. M. A.: Biomass resilience of Neotropical secondary forests, Nature, 530, 211–214, https://doi.org/10.1038/nature16512, 2016.
Rammig, A.: Tropical carbon sinks are saturating at different times on different continents, Nature, 579, 38–39, https://doi.org/10.1038/d41586-020-00423-8, 2020.
Ratnam, J., Bond, W. J., Fensham, R. J., Hoffmann, W. A., Archibald, S., Lehmann, C. E. R., Anderson, M. T., Higgins, S. I., and Sankaran, M.: When is a “forest” a savanna, and why does it matter?, Glob. Ecol. Biogeogr., 20, 653–660, https://doi.org/10.1111/j.1466-8238.2010.00634.x, 2011.
Reyer, C. P. O., Brouwers, N., Rammig, A., Brook, B. W., Epila, J., Grant, R. F., Holmgren, M., Langerwisch, F., Leuzinger, S., Lucht, W., Medlyn, B., Pfeifer, M., Steinkamp, J., Vanderwel, M. C., Verbeeck, H., and Villela, D. M.: Forest resilience and tipping points at different spatio-temporal scales: approaches and challenges, J. Ecol., 103, 5–15, https://doi.org/10.1111/1365-2745.12337, 2015.
Rosas, T., Mencuccini, M., Barba, J., Cochard, H., Saura-Mas, S., and Martínez-Vilalta, J.: Adjustments and coordination of hydraulic, leaf and stem traits along a water availability gradient, New Phytol., 223, 632–646, https://doi.org/10.1111/nph.15684, 2019.
Schenk, H. J.: Soil depth, plant rooting strategies and species' niches, New Phytol., 178, 223–225, https://doi.org/10.1111/j.1469-8137.2008.02427.x, 2008.
Schenk, H. J. and Jackson, R. B.: The Global Biogeography of Roots, Ecol. Monogr., 72, 311–328, https://doi.org/10.1890/0012-9615(2002)072[0311:TGBOR]2.0.CO;2, 2002.
Schumacher, D. L., Keune, J., Dirmeyer, P., and Miralles, D. G.: Drought self-propagation in drylands due to land–atmosphere feedbacks, Nat. Geosci., 15, 262–268, https://doi.org/10.1038/s41561-022-00912-7, 2022.
Singh, C.: Rooting for forest resilience: Implications of climate and land-use change on the tropical rainforests, thesis, ISBN 978-91-8014-120-8, 2023a.
Singh, C.: Future-forest-transitions-CMIP6, GitHub [code], https://github.com/chandrakant6492/Future-forest-transitions-CMIP6 (last access: 2 December 2024), 2023b.
Singh, C.: Tropical rainforest resilience under CMIP6-SSP scenarios (Version 1), Zenodo [data set], https://doi.org/10.5281/zenodo.7845439, 2023c.
Singh, C., Wang-Erlandsson, L., Fetzer, I., Rockström, J., and van der Ent, R.: Rootzone storage capacity reveals drought coping strategies along rainforest-savanna transitions, Environ. Res. Lett., 15, 124021, https://doi.org/10.1088/1748-9326/abc377, 2020 (code available at: https://github.com/chandrakant6492/Drought-coping-strategy, last access: 18 January 2023).
Singh, C., van der Ent, R., Wang-Erlandsson, L., and Fetzer, I.: Hydroclimatic adaptation critical to the resilience of tropical forests, Glob. Change Biol., 28, 2930–2939, https://doi.org/10.1111/gcb.16115, 2022.
Singh, V., Karan, S. K., Singh, C., and Samadder, S. R.: Assessment of the capability of SWAT model to predict surface runoff in open cast coal mining areas, Environ. Sci. Pollut. Res., 30, 40073–40083, https://doi.org/10.1007/s11356-022-25032-y, 2023.
Slik, J. W. F., Franklin, J., Arroyo-Rodríguez, V., Field, R., Aguilar, S., Aguirre, N., Ahumada, J., Aiba, S.-I., Alves, L. F., K, A., Avella, A., Mora, F., Aymard C., G. A., Báez, S., Balvanera, P., Bastian, M. L., Bastin, J.-F., Bellingham, P. J., van den Berg, E., da Conceição Bispo, P., Boeckx, P., Boehning-Gaese, K., Bongers, F., Boyle, B., Brambach, F., Brearley, F. Q., Brown, S., Chai, S.-L., Chazdon, R. L., Chen, S., Chhang, P., Chuyong, G., Ewango, C., Coronado, I. M., Cristóbal-Azkarate, J., Culmsee, H., Damas, K., Dattaraja, H. S., Davidar, P., DeWalt, S. J., Din, H., Drake, D. R., Duque, A., Durigan, G., Eichhorn, K., Eler, E. S., Enoki, T., Ensslin, A., Fandohan, A. B., Farwig, N., Feeley, K. J., Fischer, M., Forshed, O., Garcia, Q. S., Garkoti, S. C., Gillespie, T. W., Gillet, J.-F., Gonmadje, C., Granzow-de la Cerda, I., Griffith, D. M., Grogan, J., Hakeem, K. R., Harris, D. J., Harrison, R. D., Hector, A., Hemp, A., Homeier, J., Hussain, M. S., Ibarra-Manríquez, G., Hanum, I. F., Imai, N., Jansen, P. A., Joly, C. A., Joseph, S., Kartawinata, K., Kearsley, E., Kelly, D. L., Kessler, M., Killeen, T. J., Kooyman, R. M., Laumonier, Y., Laurance, S. G., Laurance, W. F., Lawes, M. J., Letcher, S. G., Lindsell, J., Lovett, J., Lozada, J., Lu, X., Lykke, A. M., Mahmud, K. B., Mahayani, N. P. D., Mansor, A., Marshall, A. R., Martin, E. H., Calderado Leal Matos, D., Meave, J. A., Melo, F. P. L., Mendoza, Z. H. A., et al.: Phylogenetic classification of the world's tropical forests, P. Natl. Acad. Sci. USA, 115, 1837–1842, https://doi.org/10.1073/pnas.1714977115, 2018.
Smith, C. W., Johnston, M. A., and Lorentz, S. A.: The effect of soil compaction on the water retention characteristics of soils in forest plantations, S. Afr. J. Plant Soil, 18, 87–97, https://doi.org/10.1080/02571862.2001.10634410, 2001.
Sperry, J. S. and Love, D. M.: What plant hydraulics can tell us about responses to climate-change droughts, New Phytol., 207, 14–27, https://doi.org/10.1111/nph.13354, 2015.
Staal, A., Tuinenburg, O. A., Bosmans, J. H. C., Holmgren, M., van Nes, E. H., Scheffer, M., Zemp, D. C., and Dekker, S. C.: Forest-rainfall cascades buffer against drought across the Amazon, Nat. Clim. Change, 8, 539–543, https://doi.org/10.1038/s41558-018-0177-y, 2018.
Staal, A., Fetzer, I., Wang-Erlandsson, L., Bosmans, J. H. C., Dekker, S. C., van Nes, E. H., Rockström, J., and Tuinenburg, O. A.: Hysteresis of tropical forests in the 21st century, Nat. Commun., 11, 4978, https://doi.org/10.1038/s41467-020-18728-7, 2020.
Stevens, B. and Bony, S.: What Are Climate Models Missing?, Science, 340, 1053–1054, https://doi.org/10.1126/science.1237554, 2013.
Still, C. J., Berry, J. A., Collatz, G. J., and DeFries, R. S.: Global distribution of C3 and C4 vegetation: Carbon cycle implications, Global Biogeochem. Cy., 17, 1006, https://doi.org/10.1029/2001GB001807, 2003.
Stocker, B. D., Tumber-Dávila, S. J., Konings, A. G., Anderson, M. C., Hain, C., and Jackson, R. B.: Global patterns of water storage in the rooting zones of vegetation, Nat. Geosci., 16, 250–256, https://doi.org/10.1038/s41561-023-01125-2, 2023.
Sveen, T. R., Hannula, S. E., and Bahram, M.: Microbial regulation of feedbacks to ecosystem change, Trend. Microbiol., 32, 68–78, https://doi.org/10.1016/j.tim.2023.06.006, 2024.
Trumbore, S., Brando, P., and Hartmann, H.: Forest health and global change, Science, 349, 814–818, https://doi.org/10.1126/science.aac6759, 2015.
Valdes, P.: Built for stability, Nat. Geosci., 4, 414–416, https://doi.org/10.1038/ngeo1200, 2011.
van der Ent, R. J., Savenije, H. H. G., Schaefli, B., and Steele-Dunne, S. C.: Origin and fate of atmospheric moisture over continents, Water Resour. Res., 46, W09525, https://doi.org/10.1029/2010WR009127, 2010.
van Nes, E. H., Arani, B. M. S., Staal, A., van der Bolt, B., Flores, B. M., Bathiany, S., and Scheffer, M.: What Do You Mean, `Tipping Point'?, Trend. Ecol. Evol., 31, 902–904, https://doi.org/10.1016/j.tree.2016.09.011, 2016.
Wang, E., Smith, C. J., Wang, E., and Smith, C. J.: Modelling the growth and water uptake function of plant root systems: a review, Aust. J. Agric. Res., 55, 501–523, https://doi.org/10.1071/AR03201, 2004.
Wang-Erlandsson, L., Bastiaanssen, W. G. M., Gao, H., Jägermeyr, J., Senay, G. B., van Dijk, A. I. J. M., Guerschman, J. P., Keys, P. W., Gordon, L. J., and Savenije, H. H. G.: Global root zone storage capacity from satellite-based evaporation, Hydrol. Earth Syst. Sci., 20, 1459–1481, https://doi.org/10.5194/hess-20-1459-2016, 2016.
Wang-Erlandsson, L., Tobian, A., van der Ent, R. J., Fetzer, I., te Wierik, S., Porkka, M., Staal, A., Jaramillo, F., Dahlmann, H., Singh, C., Greve, P., Gerten, D., Keys, P. W., Gleeson, T., Cornell, S. E., Steffen, W., Bai, X., and Rockström, J.: A planetary boundary for green water, Nat. Rev. Earth Environ., 3, 380–392, https://doi.org/10.1038/s43017-022-00287-8, 2022.
Wolfe, B. T., Sperry, J. S., and Kursar, T. A.: Does leaf shedding protect stems from cavitation during seasonal droughts? A test of the hydraulic fuse hypothesis, New Phytol., 212, 1007–1018, https://doi.org/10.1111/nph.14087, 2016.
Wunderling, N., Staal, A., Sakschewski, B., Hirota, M., Tuinenburg, O. A., Donges, J. F., Barbosa, H. M. J., and Winkelmann, R.: Recurrent droughts increase risk of cascading tipping events by outpacing adaptive capacities in the Amazon rainforest, P. Natl. Acad. Sci. USA, 119, e2120777119, https://doi.org/10.1073/pnas.2120777119, 2022.
Xie, S.-P., Deser, C., Vecchi, G. A., Ma, J., Teng, H., and Wittenberg, A. T.: Global Warming Pattern Formation: Sea Surface Temperature and Rainfall, J. Clim., 23, 966–986, https://doi.org/10.1175/2009JCLI3329.1, 2010.
Xu, C., Hantson, S., Holmgren, M., van Nes, E. H., Staal, A., and Scheffer, M.: Remotely sensed canopy height reveals three pantropical ecosystem states, Ecology, 97, 2518–2521, https://doi.org/10.1002/ecy.1470, 2016.
Xue, B.-L., Guo, Q., Otto, A., Xiao, J., Tao, S., and Li, L.: Global patterns, trends, and drivers of water use efficiency from 2000 to 2013, Ecosphere, 6, art174, https://doi.org/10.1890/ES14-00416.1, 2015.
Yang, Y., Saatchi, S. S., Xu, L., Yu, Y., Choi, S., Phillips, N., Kennedy, R., Keller, M., Knyazikhin, Y., and Myneni, R. B.: Post-drought decline of the Amazon carbon sink, Nat. Commun., 9, 3172, https://doi.org/10.1038/s41467-018-05668-6, 2018.
Yu, Z., Chen, X., Zhou, G., Agathokleous, E., Li, L., Liu, Z., Wu, J., Zhou, P., Xue, M., Chen, Y., Yan, W., Liu, L., Shi, T., and Zhao, X.: Natural forest growth and human induced ecosystem disturbance influence water yield in forests, Commun. Earth Environ., 3, 148, https://doi.org/10.1038/s43247-022-00483-w, 2022.
Yuan, K., Zhu, Q., Riley, W. J., Li, F., and Wu, H.: Understanding and reducing the uncertainties of land surface energy flux partitioning within CMIP6 land models, Agr. Forest Meteorol., 319, 108920, https://doi.org/10.1016/j.agrformet.2022.108920, 2022.
Zhang, Y., Pena Arancibia, J., McVicar, T., Chiew, F., Vaze, J., Zheng, H., and Wang, Y. P.: Monthly global observation-driven Penman-Monteith-Leuning (PML) evapotranspiration and components, v2, CSIRO [data set], https://doi.org/10.4225/08/5719A5C48DB85, 2016.
Zemp, D. C., Schleussner, C.-F., Barbosa, H. M. J., van der Ent, R. J., Donges, J. F., Heinke, J., Sampaio, G., and Rammig, A.: On the importance of cascading moisture recycling in South America, Atmos. Chem. Phys., 14, 13337–13359, https://doi.org/10.5194/acp-14-13337-2014, 2014.
Zemp, D. C., Schleussner, C.-F., Barbosa, H. M. J., Hirota, M., Montade, V., Sampaio, G., Staal, A., Wang-Erlandsson, L., and Rammig, A.: Self-amplified Amazon forest loss due to vegetation-atmosphere feedbacks, Nat. Commun., 8, 14681, https://doi.org/10.1038/ncomms14681, 2017.
Zhang, Y., Peña-Arancibia, J. L., McVicar, T. R., Chiew, F. H. S., Vaze, J., Liu, C., Lu, X., Zheng, H., Wang, Y., Liu, Y. Y., Miralles, D. G., and Pan, M.: Multi-decadal trends in global terrestrial evapotranspiration and its components, Sci. Rep., 6, 19124, https://doi.org/10.1038/srep19124, 2016.
Zilli, M. T., Carvalho, L. M. V., and Lintner, B. R.: The poleward shift of South Atlantic Convergence Zone in recent decades, Clim. Dynam., 52, 2545–2563, https://doi.org/10.1007/s00382-018-4277-1, 2019.