the Creative Commons Attribution 4.0 License.
the Creative Commons Attribution 4.0 License.
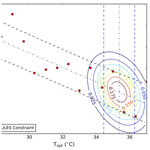
Combining local model calibration with the emergent constraint approach to reduce uncertainty in the tropical land carbon cycle feedback
Tim Jupp
Ben Booth
Peter Cox
The role of the land carbon cycle in climate change remains highly uncertain. A key source of the projection spread is related to the assumed response of photosynthesis to warming, especially in the tropics. The optimum temperature for photosynthesis determines whether warming positively or negatively impacts photosynthesis, thereby amplifying or suppressing CO2 fertilisation of photosynthesis under CO2-induced global warming. Land carbon cycle models have been extensively calibrated against local eddy flux measurements, but this has not previously been clearly translated into a reduced uncertainty in terms of how the tropical land carbon sink will respond to warming. Using a previous parameter perturbation ensemble carried out with version 3 of the Hadley Centre coupled climate–carbon cycle model (HadCM3C), we identify an emergent relationship between the optimal temperature for photosynthesis, which is especially relevant in tropical forests, and the projected amount of atmospheric CO2 at the end of the century. We combine this with a constraint on the optimum temperature for photosynthesis, derived from eddy covariance measurements using the adjoint of the Joint UK Land Environment Simulator (JULES) land surface model. Taken together, the emergent relationship from the coupled model and the constraint on the optimum temperature for photosynthesis define an emergent constraint on future atmospheric CO2 in the HadCM3C coupled climate–carbon cycle under a common emissions scenario (A1B). The emergent constraint sharpens the probability density of simulated CO2 change (2100–1900) and moves its peak to a lower value of 497 ± 91 compared to 607 ± 128 ppmv (parts per million by volume) when using the equal-weight prior. Although this result is likely to be model and scenario dependent, it demonstrates the potential of combining the large-scale emergent constraint approach with a parameter estimation using detailed local measurements.
- Article
(2378 KB) - Full-text XML
- BibTeX
- EndNote
One of the key sources of uncertainty in future climate projections is the evolution of the land carbon sink (Friedlingstein et al., 2006; Cox et al., 2000; Arora et al., 2020; Canadell et al., 2021). As climate change elevates global temperatures and CO2 conditions, the rate and efficiency of vegetation photosynthesis and respiration changes, influencing the capacity of the land to act as a repository for anthropogenic CO2 (Medlyn et al., 1999; Cox et al., 2000; Friedlingstein et al., 2006). The structure and distribution of vegetation may also change in response to the associated climate change, such as changes in precipitation patterns (Trenberth, 2011). These responses provide feedback on the initial climate change signal, potentially leading to key transitions and tipping points in the land biosphere. Notable examples include a global carbon sink-to-source transition, Amazon rainforest dieback (Cox et al., 2004), shifting of the boreal forests (Chapin et al., 2004), and greening of the Sahel (Claussen et al., 2002).
Despite the increasing complexity of the climate–carbon cycle models developed for the latest IPCC (International Panel on Climate Change) Assessment Report (AR6), there is still a significant spread in the projections of vegetation and soil carbon under common trajectories of atmospheric greenhouse gases and aerosols (Canadell et al., 2021). This spread arises partly from different climate projections within the host climate model and partly from uncertainties in the land surface models themselves. Indeed, for the Joint UK Land Environment Simulator (JULES) land surface model (Clark et al., 2011; Best et al., 2011) under one of the IPCC Special Report on Emissions Scenarios (SRES – A1B; Nakicenovic et al., 2000), the atmospheric CO2 change by the end of the century (ΔCO2) was found to range from 373.8 to 845.7 ppmv (parts per million by volume; Booth et al., 2012). This range was achieved simply by perturbing some of the model parameters related to the sensitivities of plant photosynthesis and soil respiration to temperature, stomatal conduction, soil water availability and surface evaporation, and plant competition. The key source of projection spread was found to be related to the assumed response of photosynthesis to warming, especially in the tropics (Kattge and Knorr, 2007; Booth et al., 2012; Cox et al., 2013; Mercado et al., 2018). Indeed, the optimum temperature for photosynthesis (Topt) is a common parameter in land surface models that determines whether warming has a positive or negative impact on photosynthesis, thereby either amplifying or suppressing the CO2 fertilisation of photosynthesis under CO2-induced global warming (Friedlingstein et al., 2006; Arora et al., 2020).
There is an urgent need to reduce such parametric uncertainties to make reliable and believable climate projections. Usually, to reduce uncertainty in model simulations, models are confronted with observations. However, although there is now an unprecedented volume of in situ and Earth observation (EO) data with which to confront the models, the relatively shorter timescales mean that these cannot be directly used to create constraints on changes in the Earth system over the next century. Furthermore, it is extremely computationally expensive to run complex land carbon cycle models (also known as land surface models or LSMs), within Earth system models (ESMs) to produce multiple climate–carbon cycle projections. Instead, computationally efficient ways to translate short-term constraints into reductions in the long-term projection uncertainty need to be developed.
Emergent constraints are used to bridge the gap between short-term contemporary observations and long-term future predictions (Cox et al., 2013; Wenzel et al., 2014, 2016; Hall et al., 2019; Williamson et al., 2021). Using the constraints provided by the observations and physical understanding available today, emergent constraints can be used to assess the relative likelihood of different long-term trends (Allen and Ingram, 2002). Emergent constraints identified in the carbon cycle include the sensitivity of the annual growth rate of atmospheric CO2 to tropical temperature anomalies (Cox et al., 2013) and the changing amplitude of the CO2 seasonal cycle to the projected land photosynthesis (Wenzel et al., 2016; Hall et al., 2019; Williamson et al., 2021).
Data assimilation (DA) has been shown to be a useful and versatile tool to constrain the response of the carbon cycle in LSMs in the short term. DA techniques use contemporary observations to improve the performance of a model by optimising two different components, which are either the values of unknown parameters (parameter estimation) or the predictions of the model according to a given dataset (state estimation). In both cases, this is achieved by trying to find an optimal match between the model and the observations by varying the properties of the model. In numerical weather prediction, DA has predominantly been used to optimise the state whilst keeping the parameters fixed. This is because the physics are mostly known and well-understood. However, in terrestrial carbon cycle models, where most of the equations are unknown, finding the correct set of parameters is more pertinent. These models can have over a hundred internal parameters representing the environmental sensitivities of the various land surface and plant functional types. These parameters are generally chosen to represent measurable real-world quantities (e.g. surface albedo and plant root depth). This allows observationally based estimates of these parameters to be made in the early stages of the model development process. However, the detailed performance of an LSM can be very sensitive to such internal parameters, and so it is common for land surface modellers to calibrate their models against available observations. Since optimisations give the best possible values of the parameters, given the model parameterisation and structural errors, the results are more reliable than field measurements of the same parameters, which are often taken at different spatial scales than the model resolution.
In this study, we show how we can combine parameter optimisation with emergent constraint techniques to reduce uncertainty in future projections. Specifically, we derive an emergent constraint between a linear regression across the possible JULES Topt values between the change in CO2 by the end of the century (ΔCO2), and the posterior distribution of parameter Topt optimised against gross primary productivity (GPP) and latent heat (LE) in situ measurements.
2.1 A relationship between Topt and ΔCO2
In Booth et al. (2012)'s study, a large range of climate–carbon cycle feedbacks was found by perturbing the model parameters in the land surface component of the Hadley Centre coupled climate model (version 3; HadCM3C). This experiment was conducted under the common climate scenario, A1B, which describes a future world of very rapid economic growth, a global population that peaks in the mid-century and declines after that, and the rapid introduction of new and more efficient technologies, with a balance of fossil-intensive and non-fossil energy sources (Nakicenovic et al., 2000). One of the parameters perturbed in Booth et al. (2012) was Topt, which corresponds to the optimal temperature for non-light-limited photosynthesis for broadleaf forests. In JULES, non-light-limited leaf-level photosynthesis is controlled by the carboxylation rate, following the models of Collatz et al. (1991, 1992), with Topt representing the temperature at which the carboxylation rate reaches a maximum. This parameter was identified as being the most important in controlling the carbon response of the model. Indeed, a statistically highly significant (p=0.000153) relationship between Topt and net CO2 change by 2100 (ΔCO2) was found, whereas the rest of the parameters perturbed in the experiment showed little to no correlation with this change (Booth et al., 2012). Topt and ΔCO2 were shown to be anti-correlated, with higher values of Topt resulting in lower values of ΔCO2. This implies that when the optimal temperature for photosynthesis for broadleaf trees is high, more CO2 is predicted to be removed from the atmosphere through increased CO2 fertilisation. This is particularly relevant in the tropics, where in a warming world, ambient temperatures have the potential to exceed the optimal photosynthetic temperature persistently and where broadleaf trees represent large carbon stocks (Booth et al., 2012).
Using linear regression, we can exploit this relationship to calculate a probability distribution function (PDF) for the distribution of ΔCO2 given Topt; i.e. P{ΔCO2|Topt}. The contours of equal probability density around the best-fit linear regression follow a Gaussian probability density.
where f is the function describing the linear regression between ΔCO2 and Topt, and σf is the prediction error in the regression.
2.2 A constraint on Topt using local eddy flux measurements
The land surface component of HadCM3C was the Met Office Surface Exchange Scheme (MOSES; Cox et al., 1999), which became the Joint UK Land Environment Simulator (JULES). The adJULES system (Raoult et al., 2016) was developed specifically to optimise the internal parameter of the JULES land surface model using data assimilation. Data assimilation allows the integration of multiple types of data (y) in order to optimise the model parameters (x), while making allowance for associated uncertainties. It is a powerful tool which allows for objective and repeatable calibrations. A Bayesian framework is used to include prior knowledge about the parameters (xb). All errors are assumed to be Gaussian distributed (with R and B as the prior error covariance matrices for the observations and parameters, respectively). The optimisation corresponds to minimising the mismatch (J) between the model outputs and the observed data with respect to x as follows:
where M(x) is the model output vector given x. Methods for minimising the cost function range from stochastic random search algorithms to deterministic gradient-based methods.
This second class of methods was integrated into the adJULES system (Raoult et al., 2016). The adJULES system uses the adjoint of the JULES model, a computationally efficient way used to calculate the gradient of Eq. (2). The adjoint allows for efficient and repeatable optimisations utilising the gradient information. The quasi-Newton algorithm L-BFGS-B (limited memory Broyden–Fletcher–Goldfarb–Shanno algorithm with bound constraints; see Byrd et al., 1995) is used to minimise the cost function iteratively. At each iteration of the algorithm, the cost function and its gradient with respect to each parameter are evaluated. The adjoint also allows for the accurate calculation of the Hessian (second derivative of the cost function) at the optimum. The Hessian determines the posterior error covariance matrix, which is used to calculate the posterior uncertainties associated with the best-fit parameters (in the form of PDFs).
Deriving the adjoint of a model as complex as JULES is extremely costly. Fortunately, this has been done for JULES v2.2, which uses the same photosynthesis model as MOSES, allowing us to optimise the same parameters and photosynthesis model as used in HadCM3C and, therefore, in Booth et al. (2012)'s perturbation experiment. In Raoult et al. (2016), adJULES was used to improve the model performance at a wide range of broadleaf sites by optimising the key land surface parameters perturbed in Booth et al. (2012). Each parameter was assigned a wide prior distribution, allowing the parameters to take values from a large range of credible values elicited from expert opinion. The optimisation was performed using monthly in situ GPP and LE data from CO2 eddy fluxes measured at FluxNet sites (Baldocchi et al., 2001; Pastorello et al., 2020). The FluxNet database contains more than 500 locations worldwide, and all of the data are processed in a harmonised manner, using the standard methodologies including correction, gap-filling, and partitioning (Papale et al., 2006). A large number of broadleaf sites was selected from this database (27 in total; see Raoult et al., 2016, for details). The optimisation was performed in a multi-site configuration, i.e. simultaneously over all selected sites, and over both fluxes to find a single set of best-fit model parameters and their associated uncertainties. The optimisation returned best-fit parameters with posterior distributions much narrower than the prior, thereby reducing the range of viable parameter values. From these posterior distributions, we obtain an observationally constrained PDF for Topt (i.e. P(Topt)).
2.3 Calculation of the PDF for ΔCO2
We follow the method used by Cox et al. (2018) to bring these two elements together and calculate the PDF for ΔCO2. The PDF for ΔCO2 is calculated by numerically integrating over the product of two PDFs, namely P{ΔCO2|Topt} and P(Topt).
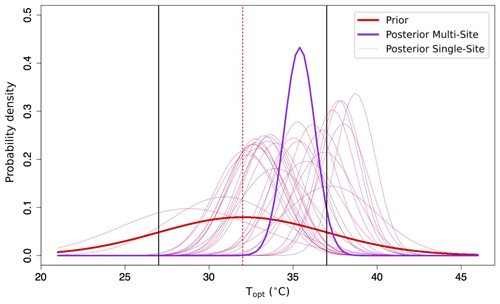
Figure 1Different PDFs of P(Topt) found when using the adJULES system to optimise the JULES land surface model against FluxNet data. The prior distribution (red) of the parameter is compared to the posterior distribution (purple) found by simultaneously calibrating over the 27 broadleaf FluxNet sites considered in Raoult et al. (2016, i.e. a multi-site optimisation) and the individual posterior distributions found by calibrating at each site separately (i.e. single-site optimisations). All distributions are modelled by a Gaussian curve. Note that the range used in the optimisation (entire x axis) is greater than the range used in Booth et al. (2012, vertical black lines). The initial value of Topt in JULES is highlighted by the dashed red line.
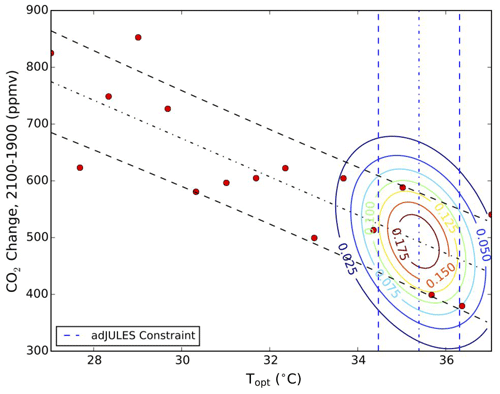
Figure 2Contours of the probability density for the linear regression adapted from Booth et al. (2012). The red dots show the relationship between different Topt values and the resulting change in CO2 by the end of the century from the parameter perturbation experiment of Booth et al. (2012, see Table A1 for these values). The thin dashed black line shows the best-fit linear regression, and the thick dashed black lines on either side show the best-fit linear regression plus and minus the prediction error (see Sect. 2). The vertical blue lines show the observational constraint on the Topt value, with the best fit shown by the thin dashed blue line, and the thick vertical dashed lines on either side showing plus and minus 1 standard error about this value. The continuous contours are the product of these two underlying PDFs. The integral of these contours across the x-axis variable leads to the Topt-constrained PDF shown in Fig. 3a.
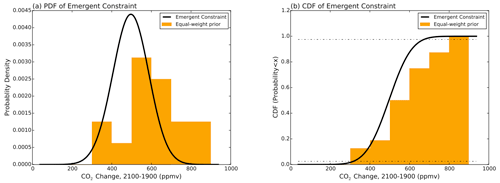
Figure 3Emergent constraint on the sensitivity of Topt to the magnitude of the future carbon cycle response. The probability density histogram for the unconstrained Topt values (orange) and the conditional PDF arising from the emergent constraint (black) are shown in panel (a) and the corresponding cumulative distribution in panel (b). The horizontal dotted–dashed line shows the 95 % confidence limits on the cumulative density function (CDF) plot. The orange histograms (both panels) show the prior distributions that arise from the equal weighting of the parameter perturbation experiment in 500 ppmv bins.
Figure 1 shows how the distribution of likely Topt values, i.e. P(Topt), changes when the JULES LSM is optimised against local measurements of photosynthesis (GPP) and latent heat (LE) using the adJULES system (Raoult et al., 2016). We can see that the posterior distribution is much more pronounced than the prior and suggests a higher parameter value than previously used. Values of Topt taken from this distribution, when used in the JULES model, will result in the best fit of the model to local measurements of photosynthesis (GPP) and latent heat (LE) and therefore improve the model's credibility.
In addition to displaying the results found by optimising simultaneously over all of the broadleaf sites found in Raoult et al. (2016), Fig. 1 also considers distributions of Topt found when optimising at each individual broadleaf site. Though none of these gives such a narrow distribution, the majority do suggest that the optimal value for the parameter (shown by the peak of the distributions) is higher than previously used in the JULES model. This gives confidence in the posterior distribution found by calibrating over all sites. Furthermore, one of the known limitations of gradient-based methods is their tendency to become stuck in local minima (i.e. not finding the “true” global minimum). Optimisations over multiple sites have been shown to be more robust, with the additional constraints from each site acting to smooth the cost function, thus making local minima less common. As such, multi-site optimisations are more reliable for finding the true best-fit parameters and associated PDFs. For the remainder of this study, we will solely use the posterior distribution found by calibrating over all sites.
Through this multi-site calibration, we find Topt (i.e. the optimal temperature for non-limited photosynthesis for broadleaf forests) to be around 35 ∘C, with an uncertainty of approximately ± 0.9 ∘C. This value falls well within the typical 30–40 ∘C temperature range observed in most leaf-scale photosynthetic-temperature response curves (Kattge and Knorr, 2007). However, land surface models are not commonly run at the leaf scale – especially not when run within wider Earth system models to predict climate change. Furthermore, Topt at the leaf scale has been shown to differ from Topt at ecosystem level (Field et al., 1995; Huang et al., 2019), where additional processes limiting photosynthesis may be impacted by temperature changes (e.g. accelerated leaf ageing at high atmospheric temperatures). While Huang et al. (2019) showed that the global mean of Topt at the ecosystem scale was lower (23 ± 6 ∘C) than the leaf-level values, they also showed that warmer regions had higher ecosystem-scale Topt values than cooler ones. Indeed, for the tropics, these values were found to be close to the growing season air temperature and again similar to the values of Topt obtained through the adJULES calibration.
We can now translate the reduction of the uncertainty in the Topt into a reduction of the uncertainty in carbon–climate feedbacks. Instead of running computationally expensive climate models with a new set of the parameter ensembles generated from the posterior distribution, this posterior PDF in Topt can be directly translated into a PDF for atmospheric carbon change, using the carbon cycle sensitivity identified in Booth et al. (2012) as an emergent constraint. The linear relationship between Topt and CO2 change is shown in Fig. 2. The vertical blue lines included in this figure show the Topt constraint from adJULES. These lines are found at the upper end of the figure and select a narrow range of Topt values. Using this constraint, we can derive tighter bounds on the CO2 response of the model. The linear regression and Topt constraint can then be used to generate contours from the product of the two PDFs and hence the Topt-constrained PDF of CO2 change between 1900 and 2100.
Figure 3a shows this PDF. This PDF is compared to the histogram arising from the assumption that all of the Topt values in the ensemble are equally likely to be true. The emergent constraint from the Topt optimisation sharpens the PDF of CO2 change (2100–1900) and moves its peak to a lower values of 496.5 ± 91 compared to 606.6 ± 128 ppmv when using the equal-weight prior. Figure 3a shows the resulting cumulative density function (CDF), which gives the probability of CO2 change (2100–1900) taking a value lower than the value shown on the x axis. The 95 % confidence limits (shown by the horizontal black lines) range from 300 to 650 ppmv. We see that values higher than 650 ppmv become extremely unlikely. The Topt constraint, therefore, reduces the estimated probability of CO2 change values, predicting a slightly stronger carbon sink over broadleaf trees than previously suggested by the JULES climate predictions and reducing the range of possible responses by 30 % and discounting higher values of CO2 change. Although both the calibration of Topt (Raoult et al., 2016) and the parameter perturbation experiment were conducted globally (Booth et al., 2012), the latter found that the dominant cause of the spread in future CO2 was due to the tropical land and specifically due to the assumed optimum temperature for photosynthesis tropical forests.
Data assimilation and emergent constraints are two powerful techniques which can enable more precise projections of climate change. By bridging the gap between both techniques, we have shown that optimisations can be used not only to improve the current state of the model but also to constrain climate predictions. Short-scale half-hourly observations spanning only a few years can be used to inform us about expected changes in the next century. By severely reducing the uncertainty in Topt, we have reduced the uncertainty in the CO2 change predicted by JULES under HadCM3C for the end of the century under the A1B climate scenario. These results are no doubt model and scenario dependent. Nevertheless, this study highlights a new methodology to use should future models show strong emergent relationships between model parameters and future climate change.
JULES is a complex and ever-evolving land surface model, with more processes being added regularly. Newer versions JULES now exist, so an updated parameter perturbation experiment would need to be conducted to understand the new sensitivities of the model to future climate change. However, running JULES coupled with a climate–carbon model like HadGEM3 (the successor to HadCM3C) to test these sensitivities requires a lot of time and resources. Instead, we may need to rely on different tools such as the IMOGEN (Integrated Model Of Global Effects of climatic aNomalies; Huntingford et al., 2010) system, an emulator of climate change using pattern scaling. Furthermore, developing the adjoint of the newest version of JULES is complicated. Deriving the adjoint of complex models like JULES is costly and becomes quickly outdated as the model versions advance. Fortunately, newer optimisation schemes have become available (e.g. LAVENDAR; Pinnington et al., 2020), which still allow for posterior PDFs to be generated after each optimisation.
This study acts as a proof of concept – a blueprint for constraining future projections of a land surface model. We have shown that observational datasets are crucial in helping us understand and reduce uncertainty in large-scale climate feedback. With the growing volume of observational data available, both from in situ and satellite observations, there is a unique opportunity to perform multiple data stream optimisations, which increase the credibility of the posterior parameter distributions. There are many datasets we could use to constrain the carbon cycle, including the interannual variability in the leaf area index, solar-induced fluorescence, and atmospheric CO2. Furthermore, due to the strong coupling between the carbon–water–energy cycles, we could move to use other constraints to optimise the model parameters, such as soil moisture and land surface temperature. Note that unlike the more orthodox application of DA in weather forecasting, the Raoult et al. (2016) study used DA for parameter estimation to derive optimum JULES parameters to fit FluxNet observational data rather than to nudge state variables. The paper shows that the resulting constraint on the optimum temperature for photosynthesis (Topt) in turn provides an emergent constraint on the increase in atmospheric CO2 by 2100 in a coupled climate–carbon cycle model (Booth et al., 2012). Although this clear link is very likely to be model dependent, we present it here as a first example of how local model calibration and the emergent constraint technique can be used to constrain global climate–carbon cycle projections.
Table A1Results from Booth et al. (2012)'s parameter perturbation experiment.
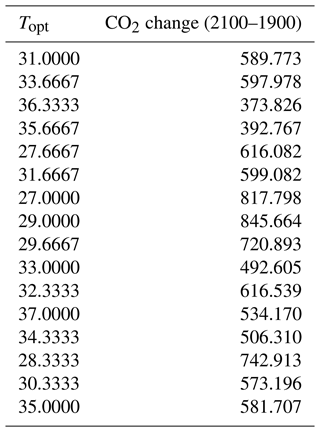
The code and data used in this paper are available at https://doi.org/10.5281/zenodo.8143078 (Raoult, 2023).
NR and PC designed the study. BB provided data from the parameter perturbation experiment. NR and TJ built the adJULES system used to constrain JULES parameters. NR and PC generated the figures. All authors contributed to writing and editing the text.
The contact author has declared that none of the authors has any competing interests.
Publisher’s note: Copernicus Publications remains neutral with regard to jurisdictional claims in published maps and institutional affiliations.
This research has been supported by the H2020 Marie Skłodowska-Curie Actions (grant no. 101020078). Peter Cox has been supported by the European Research Council project “Emergent Constraints on Climate–Land feedbacks in the Earth System” (ECCLES; grant no. 742472) and the European Union's Horizon 2020 project “Climate–Carbon Interactions in the Current Century” (4C; grant no. 821003).
This paper was edited by Anping Chen and reviewed by Mousong Wu and Yue He.
Allen, M. R. and Ingram, W. J.: Constraints on future changes in climate and the hydrologic cycle, Nature, 419, 224–232, 2002. a
Arora, V. K., Katavouta, A., Williams, R. G., Jones, C. D., Brovkin, V., Friedlingstein, P., Schwinger, J., Bopp, L., Boucher, O., Cadule, P., Chamberlain, M. A., Christian, J. R., Delire, C., Fisher, R. A., Hajima, T., Ilyina, T., Joetzjer, E., Kawamiya, M., Koven, C. D., Krasting, J. P., Law, R. M., Lawrence, D. M., Lenton, A., Lindsay, K., Pongratz, J., Raddatz, T., Séférian, R., Tachiiri, K., Tjiputra, J. F., Wiltshire, A., Wu, T., and Ziehn, T.: Carbon–concentration and carbon–climate feedbacks in CMIP6 models and their comparison to CMIP5 models, Biogeosciences, 17, 4173–4222, https://doi.org/10.5194/bg-17-4173-2020, 2020. a, b
Baldocchi, D., Falge, E., Gu, L., Olson, R., Hollinger, D., Running, S., Anthoni, P., Bernhofer, C., Davis, K., Evans, R., Fuentes, J., Goldstein, A., Katul, G., Law, B., Lee, X., Malhi, Y., Meyers, T., Munger, W., Oechel, W., Paw U, K. T., Pilegaard, K., Schmid, H. P., Valentini, R., Verma, S., Vesala, T., Wilson, K., and Wofsy, S.: FLUXNET: a new tool to study the temporal and spatial variability of ecosystem-scale carbon dioxide, water vapor, and energy flux densities, Bull. Am. Meteorol. Soc., 82, 2415–2434, 2001. a
Best, M. J., Pryor, M., Clark, D. B., Rooney, G. G., Essery, R. L. H., Ménard, C. B., Edwards, J. M., Hendry, M. A., Porson, A., Gedney, N., Mercado, L. M., Sitch, S., Blyth, E., Boucher, O., Cox, P. M., Grimmond, C. S. B., and Harding, R. J.: The Joint UK Land Environment Simulator (JULES), model description – Part 1: Energy and water fluxes, Geosci. Model Dev., 4, 677–699, https://doi.org/10.5194/gmd-4-677-2011, 2011. a
Booth, B. B., Jones, C. D., Collins, M., Totterdell, I. J., Cox, P. M., Sitch, S., Huntingford, C., Betts, R. A., Harris, G. R., and Lloyd, J.: High sensitivity of future global warming to land carbon cycle processes, Environ. Res. Lett., 7, 024002, https://doi.org/10.1088/1748-9326/7/2/024002, 2012. a, b, c, d, e, f, g, h, i, j, k, l, m, n, o
Byrd, R. H., Lu, P., Nocedal, J., and Zhu, C.: A limited memory algorithm for bound constrained optimization, SIAM J. Sci. Comput., 16, 1190–1208, https://doi.org/10.1137/0916069, 1995. a
Canadell, J. G., Monteiro, P. M. S., Costa, M. H., Cunha, L. C. D., Cox, P. M., Eliseev, A. V., Henson, S., Ishii, M., Jaccard, S., Koven, C., Lohila, A., Patra, P. K., Piao, S., Syampungani, S., Zaehle, S., Zickfeld, K., Alexandrov, G. A., Bala, G., Bopp, L., Boysen, L., Cao, L., Chandra, N., Ciais, P., Denisov, S. N., Dentener, F. J., Douville, H., Fay, A., Forster, P., Fox-Kemper, B., Friedlingstein, P., Fu, W., Fuss, S., Garçon, V., Gier, B., Gillett, N. P., Gregor, L., Haustein, K., Haverd, V., He, J., Hewitt, H. T., Hoffman, F. M., Ilyina, T., Jackson, R., Jones, C., Keller, D. P., Kwiatkowski, L., Lamboll, R. D., Lan, X., Laufkötter, C., Le Quéré, C., Lenton, A., Lewis, J., Liddicoat, S., Lorenzoni, L., Lovenduski, N., Macdougall, A. H., Mathesius, S., Matthews, D. H., Meinshausen, M., Mokhov, I. I., Naik, V., Nicholls, Z. R. J., Nurhati, I. S., O’Sullivan, M., Peters, G., Pongratz, J., Poulter, B., Sallée, J.-B., Saunois, M., Schuur, E. A. G., Seneviratne, S. I., Stavert, A., Suntharalingam, P., Tachiiri, K., Terhaar, J., Thompson, R., Tian, H., Turnbull, J., Vicente-Serrano, S. M., Wang, X., Wanninkhof, R. H., Williamson, P., Brovkin, V., Feely, R. A., and Lebehot, A. D.: Global carbon and other biogeochemical cycles and feedbacks, IPCC AR6 WGI, Final Government Distribution, chapter 5, hal-03336145, 2021. a, b
Chapin, F. S., Callaghan, T. V., Bergeron, Y., Fukuda, M., Johnstone, J., Juday, G., and Zimov, S.: Global change and the boreal forest: thresholds, shifting states or gradual change?, AMBIO, 33, 361–365, 2004. a
Clark, D. B., Mercado, L. M., Sitch, S., Jones, C. D., Gedney, N., Best, M. J., Pryor, M., Rooney, G. G., Essery, R. L. H., Blyth, E., Boucher, O., Harding, R. J., Huntingford, C., and Cox, P. M.: The Joint UK Land Environment Simulator (JULES), model description – Part 2: Carbon fluxes and vegetation dynamics, Geosci. Model Dev., 4, 701–722, https://doi.org/10.5194/gmd-4-701-2011, 2011. a
Claussen, M., Brovkin, V., and Ganopolski, A.: Africa: Greening of the Sahara, in: Challenges of a Changing Earth. Global Change – The IGBP Series, edited by: Steffen, W., Jäger, J., Carson, D. J., and Bradshaw, C., Springer, Berlin, Heidelberg, https://doi.org/10.1007/978-3-642-19016-2_23, 2002. a
Collatz, G. J., Ball, J. T., Grivet, C., and Berry, J. A.: Physiological and environmental regulation of stomatal conductance, photosynthesis and transpiration: a model that includes a laminar boundary layer, Agr. Forest Meteorol., 54, 107–136, 1991. a
Collatz, G. J., Ribas-Carbo, M., and Berry, J. A.: Coupled photosynthesis-stomatal conductance model for leaves of C4 plants, Funct. Plant Biol., 19, 519–538, 1992. a
Cox, P., Betts, R., Bunton, C., Essery, R., Rowntree, P., and Smith, J.: The impact of new land surface physics on the GCM simulation of climate and climate sensitivity, Clim. Dynam., 15, 183–203, 1999. a
Cox, P. M., Betts, R. A., Jones, C. D., Spall, S. A., and Totterdell, I. J.: Acceleration of global warming due to carbon-cycle feedbacks in a coupled climate model, Nature, 408, 184–187, 2000. a, b
Cox, P. M., Betts, R., Collins, M., Harris, P. P., Huntingford, C., and Jones, C.: Amazonian forest dieback under climate-carbon cycle projections for the 21st century, Theor. Appl. Climatol., 78, 137–156, 2004. a
Cox, P. M., Pearson, D., Booth, B. B., Friedlingstein, P., Huntingford, C., Jones, C. D., and Luke, C. M.: Sensitivity of tropical carbon to climate change constrained by carbon dioxide variability, Nature, 494, 341–344, https://doi.org/10.1038/nature11882, 2013. a, b, c
Cox, P. M., Huntingford, C., and Williamson, M. S.: Emergent constraint on equilibrium climate sensitivity from global temperature variability, Nature, 553, 319–322, 2018. a
Field, C. B., Randerson, J. T., and Malmström, C. M.: Global net primary production: combining ecology and remote sensing, Remote Sens. Environ., 51, 74–88, 1995. a
Friedlingstein, P., Cox, P., Betts, R., Bopp, L., von Bloh, W., Brovkin, V., Cadule, P., Doney, S., Eby, M., Fung, I., Bala, G., John, J., Jones, C., Joos, F., Kato, T., Kawamiya, M., Knorr, W., Lindsay, K., Matthews, H. D., Raddatz, T., Rayner, P., Reick, C., Roeckner, E., Schnitzler, K.-G., Schnur, R., Strassmann, K., Weaver, A. J., Yoshikawa, C., and Zeng, N.: Climate–carbon cycle feedback analysis: results from the C4MIP model intercomparison, J. Clim., 19, 3337–3353, 2006. a, b, c
Hall, A., Cox, P., Huntingford, C., and Klein, S.: Progressing emergent constraints on future climate change, Nat. Clim. Change, 9, 269–278, 2019. a, b
Huang, M., Piao, S., Ciais, P., Peñuelas, J., Wang, X., Keenan, T. F., Peng, S., Berry, J. A., Wang, K., Mao, J., Alkama, R., Cescatti, A., Cuntz, M., De Deurwaerder, H., Gao, M., He, Y., Liu, Y., Luo, Y., Myneni, R. B., Niu, S., Shi, X., Yuan, W., Verbeeck, H., Wang, T., Wu, J., and Janssens, I. A.: Air temperature optima of vegetation productivity across global biomes, Nat. Ecol. Evol., 3, 772–779, 2019. a, b
Huntingford, C., Booth, B. B. B., Sitch, S., Gedney, N., Lowe, J. A., Liddicoat, S. K., Mercado, L. M., Best, M. J., Weedon, G. P., Fisher, R. A., Lomas, M. R., Good, P., Zelazowski, P., Everitt, A. C., Spessa, A. C., and Jones, C. D.: IMOGEN: an intermediate complexity model to evaluate terrestrial impacts of a changing climate, Geosci. Model Dev., 3, 679–687, https://doi.org/10.5194/gmd-3-679-2010, 2010. a
Kattge, J. and Knorr, W.: Temperature acclimation in a biochemical model of photosynthesis: a reanalysis of data from 36 species, Plant Cell Environ., 30, 1176–1190, 2007. a, b
Medlyn, B. E., Badeck, F. W., De Pury, D. G. G., Barton, C. V. M., Broadmeadow, M., Ceulemans, R., De Angelis, P., Forstreuter, M., Jach, M. E., Kellomäki, S., Laitat, E., Marek, M., Philippot, S., Rey. A., Strassemeyer, J., Laitinen, K., Liozon, R., Portier, B., Roberntz, P., Wang, K., and Jarvis, P. G.: Effects of elevated [CO2] on photosynthesis in European forest species: a meta-analysis of model parameters, Plant Cell Environ., 22, 1475–1495, 1999. a
Mercado, L. M., Medlyn, B. E., Huntingford, C., Oliver, R. J., Clark, D. B., Sitch, S., Zelazowski, P., Kattge, J., Harper, A. B., and Cox, P. M.: Large sensitivity in land carbon storage due to geographical and temporal variation in the thermal response of photosynthetic capacity, New Phytol., 218, 1462–1477, 2018. a
Nakicenovic, N., Alcamo, J., Grubler, A., Riahi, K., Roehrl, R., Rogner, H.-H., and Victor, N.: Special Report on Emissions Scenarios (SRES), A Special Report of Working Group III of the Intergovernmental Panel on Climate Change, Cambridge University Press, ISBN 0-521-80493-0, 2000. a, b
Papale, D., Reichstein, M., Aubinet, M., Canfora, E., Bernhofer, C., Kutsch, W., Longdoz, B., Rambal, S., Valentini, R., Vesala, T., and Yakir, D.: Towards a standardized processing of Net Ecosystem Exchange measured with eddy covariance technique: algorithms and uncertainty estimation, Biogeosciences, 3, 571–583, https://doi.org/10.5194/bg-3-571-2006, 2006. a
Pastorello, G., Trotta, C., Canfora, E., Chu, H., Christianson, D., Cheah, Y.-W., Poindexter, C., Chen, J., Elbashandy, A., Humphrey, M., Isaac, P., Polidori, D., Reichstein, M., Ribeca, A., van Ingen, C., Vuichard, N., Zhang, L., Amiro, B., Ammann, C., Arain, M. A., Ardö, J., Arkebauer, T., Arndt, S. K., Arriga, N., Aubinet, M., Aurela, M., Baldocchi, D., Barr, A., Beamesderfer, E., Marchesini, L. B., Bergeron, O., Beringer, J., Bernhofer, C., Berveiller, D., Billesbach, D., Black, T. A., Blanken, P. D., Bohrer, G., Boike, J., Bolstad, P. V., Bonal, D., Bonnefond, J.-M., Bowling, D. R., Bracho, R., Brodeur, J., Brümmer, C., Buchmann, N., Burban, B., Burns, S. P., Buysse, P., Cale, P., Cavagna, M., Cellier, P., Chen, S., Chini, I., Christensen, T. R., Cleverly, J., Collalti, A., Consalvo, C., Cook, B. D., Cook, D., Coursolle, C., Cremonese, E., Curtis, P. S., D’Andrea, E., da Rocha, H., Dai, X., Davis, K. J., De Cinti, B., de Grandcourt, A., De Ligne, A., De Oliveira, R. C., Delpierre, N., Desai, A. R., Di Bella, C. M., di Tommasi, P., Dolman, H., Domingo, F., Dong, G., Dore, S., Duce, P., Dufrêne, E., Dunn, A., Dušek, J., Eamus, D., Eichelmann, U., ElKhidir, H. A. M., Eugster, W., Ewenz, C. M., Ewers, B., Famulari, D., Fares, S., Feigenwinter, I., Feitz, A., Fensholt, R., Filippa, G., Fischer, M., Frank, J., Galvagno, M., Gharun, M., Gianelle, D., Gielen, B., Gioli, B., Gitelson, A., Goded, I., Goeckede, M., Goldstein, A. H., Gough, C. M., Goulden, M. L., Graf, A., Griebel, A., Gruening, C., Grünwald, T., Hammerle, A., Han, S., Han, X., Hansen, B. U., Hanson, C., Hatakka, J., He, Y., Hehn, M., Heinesch, B., Hinko-Najera, N., Hörtnagl, L., Hutley, L., Ibrom, A., Ikawa, H., Jackowicz-Korczynski, M., Janouš, D., Jans, W., Jassal, R., Jiang, S., Kato, T., Khomik, M., Klatt, J., Knohl, A., Knox, S., Kobayashi, H., Koerber, G., Kolle, O., Kosugi, Y., Kotani, A., Kowalski, A., Kruijt, B., Kurbatova, J., Kutsch, W. L., Kwon, H., Launiainen, S., Laurila, T., Law, B., Leuning, R., Li, Y., Liddell, M., Limousin, J.-M., Lion, M., Liska, A. J., Lohila, A., López-Ballesteros, A., López-Blanco, E., Loubet, B., Loustau, D., Lucas-Moffat, A., Lüers, J., Ma, S., Macfarlane, C., Magliulo, V., Maier, R., Mammarella, I., Manca, G., Marcolla, B., Margolis, H. A., Marras, S., Massman, W., Mastepanov, M., Matamala, R., Matthes, J. H., Mazzenga, F., McCaughey, H., McHugh, I., McMillan, A. M. S., Merbold, L., Meyer, W., Meyers, T., Miller, S. D., Minerbi, S., Moderow, U., Monson, R. K., Montagnani, L., Moore, C. E., Moors, E., Moreaux, V., Moureaux, C., Munger, J. W., Nakai, T., Neirynck, J., Nesic, Z., Nicolini, G., Noormets, A., Northwood, M., Nosetto, M., Nouvellon, Y., Novick, K., Oechel, W., Olesen, J. E., Ourcival, J.-M., Papuga, S. A., Parmentier, F.-J., Paul-Limoges, E., Pavelka, M., Peichl, M., Pendall, E., Phillips, R. P., Pilegaard, K., Pirk, N., Posse, G., Powell, T., Prasse, H., Prober, S. M., Rambal, S., Rannik, Ü., Raz-Yaseef, N., Rebmann, C., Reed, D., Resco de Dios, V., Restrepo-Coupe, N., Reverter, B. R., Roland, M., Sabbatini, S., Sachs, T., Saleska, S. R., Sánchez-Cañete, E. P., Sanchez-Mejia, Z. M., Schmid, H. P., Schmidt, M., Schneider, K., Schrader, F., Schroder, I., Scott, R. L., Sedlák, P., Serrano-Ortíz, P., Shao, C., Shi, P., Shironya, I., Siebicke, L., Šigut, L., Silberstein, R., Sirca, C., Spano, D., Steinbrecher, R., Stevens, R. M., Sturtevant, C., Suyker, A., Tagesson, T., Takanashi, S., Tang, Y., Tapper, N., Thom, J., Tomassucci, M., Tuovinen, J.-P., Urbanski, S., Valentini, R., van der Molen, M., van Gorsel, E., van Huissteden, Varlagin, A., Verfaillie, J., Vesala, T., Vincke, C., Vitale, D., Vygodskaya, N., Walker, J. P., Walter-Shea, E., Wang, H., Weber, R., Westermann, S., Wille, C., Wofsy, S., Wohlfahrt, G., Wolf, S., Woodgate, W., Li, Y., Zampedri, R., Zhang, J., Zhou, G., Zona, D., Agarwal, D., Biraud, S., Torn, M., and Papale, D.: The FLUXNET2015 dataset and the ONEFlux processing pipeline for eddy covariance data, Sci. Data, 7, 1–27, 2020. a
Pinnington, E., Quaife, T., Lawless, A., Williams, K., Arkebauer, T., and Scoby, D.: The Land Variational Ensemble Data Assimilation Framework: LAVENDAR v1.0.0, Geosci. Model Dev., 13, 55–69, https://doi.org/10.5194/gmd-13-55-2020, 2020. a
Raoult, N.: NRaoult/adJULES: adJULES with Emergent Constraints (v1.1_adJULES_with_Emergent_Constraints), Zenodo [code, data set], https://doi.org/10.5281/zenodo.8143078, 2023. a
Raoult, N. M., Jupp, T. E., Cox, P. M., and Luke, C. M.: Land-surface parameter optimisation using data assimilation techniques: the adJULES system V1.0, Geosci. Model Dev., 9, 2833–2852, https://doi.org/10.5194/gmd-9-2833-2016, 2016. a, b, c, d, e, f, g, h, i
Trenberth, K. E.: Changes in precipitation with climate change, Clim. Res., 47, 123–138, 2011. a
Wenzel, S., Cox, P. M., Eyring, V., and Friedlingstein, P.: Emergent constraints on climate-carbon cycle feedbacks in the CMIP5 Earth system models, J. Geophys. Res.-Biogeo., 119, 794–807, 2014. a
Wenzel, S., Cox, P. M., Eyring, V., and Friedlingstein, P.: Projected land photosynthesis constrained by changes in the seasonal cycle of atmospheric CO2, Nature, 538, 499–501, 2016. a, b
Williamson, M. S., Thackeray, C. W., Cox, P. M., Hall, A., Huntingford, C., and Nijsse, F. J.: Emergent constraints on climate sensitivities, Rev. Modern Phys., 93, 025004, https://doi.org/10.1103/RevModPhys.93.025004, 2021. a, b