the Creative Commons Attribution 4.0 License.
the Creative Commons Attribution 4.0 License.
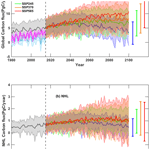
Global and northern-high-latitude net ecosystem production in the 21st century from CMIP6 experiments
Dalei Hao
Yelu Zeng
Xuesong Zhang
Climate warming is accelerating the changes in the global terrestrial ecosystems and particularly those in the northern high latitudes (NHLs; poleward of 50∘ N) and rendering the land–atmosphere carbon exchange highly uncertain. The Coupled Model Intercomparison Project Phase 6 (CMIP6) employs the most updated climate models to estimate terrestrial ecosystem carbon dynamics driven by a new set of socioeconomic and climate change pathways. By analyzing the future (2015–2100) carbon fluxes estimated by 10 CMIP6 models, we quantitatively evaluated the projected magnitudes, trends, and uncertainties in the global and NHL carbon fluxes under four scenarios plus the role of NHLs in the global terrestrial ecosystem carbon dynamics. Overall, the models suggest that the global and NHL terrestrial ecosystems will be consistent carbon sinks in the future, and the magnitude of the carbon sinks is projected to be larger under scenarios with higher radiative forcing. By the end of this century, the models on average estimate the NHL net ecosystem productivity (NEP) as 0.54 ± 0.77, 1.01 ± 0.98, 0.97 ± 1.62, and 1.05 ± 1.83 Pg C yr−1 under SSP126, SSP245, SSP370, and SSP585, respectively. The uncertainties are not substantially reduced compared with earlier results, e.g., the Coupled Climate–Carbon Cycle Model Intercomparison Project (C4MIP). Although NHLs contribute a small fraction of the global carbon sink (∼ 13 %), the relative uncertainties in NHL NEP are much larger than the global level. Our results provide insights into future carbon flux evolutions under future scenarios and highlight the urgent need to constrain the large uncertainties associated with model projections for making better climate mitigation strategies.
- Article
(4382 KB) - Full-text XML
-
Supplement
(1790 KB) - BibTeX
- EndNote
The global terrestrial biosphere is considered to be a major carbon pool and a key player in the global carbon cycle. In the last decade (2011–2020), the terrestrial biosphere has absorbed CO2 from the atmosphere at a rate of about 120 Pg C yr−1 by vegetation photosynthesis and released a similar amount of carbon back to the atmosphere through respirations from plant metabolism and microbial activities (i.e., autotrophic and heterotrophic respirations) in response to climate oscillations and disturbance-induced emissions, resulting in a land carbon sink of about 3.4 Pg C yr−1 with an additional 1.6 Pg C yr−1 loss due to land use change (Friedlingstein et al., 2020). However, these numbers of land–atmosphere carbon fluxes, especially the photosynthesis and respiration components, change over time in response to climate change and are associated with large uncertainties. For example, using trace gas measurements, Campbell et al. (2017) estimated a large increase in global terrestrial biosphere photosynthetic carbon uptake of 31 % over the 20th century accompanied by rapidly rising CO2 concentration and warming climate. This estimate however did not agree with many carbon–climate models. The global soil respiration carbon flux has also been found to increase in the past several decades, according to the analysis of a global soil respiration database, but the degree to which climate change affects the changes in heterotrophic respiration is highly uncertain (Bond-Lamberty et al., 2018). Besides the scientific importance of understanding the long-term feedbacks between the terrestrial biosphere and the climate system, it is also critical to track the changes in the global land carbon budget for making manageable climate mitigation policies as it is a key component of the global carbon budget and has been considered to be an important approach to achieving carbon neutrality.
Particularly, as the host of most of the Earth's permafrost soils, Arctic ecosystems store twice the amount of carbon as in the atmosphere and play an important role in the global carbon budget (Schuur et al., 2015; Tarnocai et al., 2009; Zimov et al., 2006). During the last few decades, the temperature in northern-high-latitude (NHL; poleward of 50∘ N) regions has been rising particularly fast. The Arctic Circle (66.5–90∘ N) has warmed more than 0.7 ∘C per decade since 1979, almost 4 times faster than the globe (Rantanen et al., 2022). Previously stored soil carbon is potentially labialized by permafrost thawing and enhanced decomposition of soil organic carbon due to a warmer climate (Belshe et al., 2012; Koven et al., 2011; Natali et al., 2014; Schaefer et al., 2011; Schuur et al., 2015; Schuur and Abbott, 2011). This shapes a positive climate feedback since the excessive carbon release would in turn stimulate climate warming (Koven et al., 2011; Schuur and Abbott, 2011; Zimov et al., 2006). On the other hand, CO2 fertilization combined with other favorable conditions could enrich plant growth and drive the expansion of vegetation, e.g., Arctic tundra and boreal forest, in the Arctic region, which may enhance plant carbon uptake and photosynthesis productivity (Berner et al., 2020; Liang et al., 2018; Mekonnen et al., 2019; Myers-Smith et al., 2020; Sistla et al., 2013). Despite the prevailing greening signal observed in the NHLs, regional browning or a negative normalized difference vegetation index (NDVI) trend was also observed (Lara et al., 2018; Phoenix and Bjerke, 2016). Disturbances such as fire are also increasing in frequency and duration in response to the warming climate change and exerting impacts on vegetation dynamics, including canopy structure and functioning, which in turn affects photosynthesis and ecosystem respirations (Hu et al., 2015; Mekonnen et al., 2019; Whitman et al., 2018). These evolving and counteracting processes complicate the determinations of whether the NHL ecosystem functions as a carbon source or sink and how this will be projected in the future. Great uncertainties are revealed from evaluating results of multiple Earth system models (ESMs) in the NHL region, with some ESMs showing NHL ecosystems as a carbon sink and others indicating an opposite sign (Fisher et al., 2014; Friedlingstein et al., 2014; Qian et al., 2010). Moreover, inconsistent model structure and diversified process representations as well as uncertainties in data, external variables, and parameterizations further compromise the confidence in predictions of ESMs (Bradford et al., 2016; Luo et al., 2016; Todd-Brown et al., 2013).
The Coupled Model Intercomparison Project (CMIP) coordinated a series of comprehensive comparisons among a handful of climate models and has become an essential element of international climate research (Eyring et al., 2016; Taylor et al., 2012). Building on the previous Atmospheric Model Intercomparison Project (AMIP), CMIPs have broadened its purposes and contributions to a wide range of disciplines to foster understanding of evolutions and changes in climate and its impacts on societal sectors from history, in the present and future (Eyring et al., 2016). Yet, great uncertainties were revealed from previous CMIPs' results, and the spread of the model responses to climate sensitivity remains large (Collins et al., 2013). A primary scientific gap of previous CMIP experiments is how the radiative forcing pathways, resulting from anthropogenic activities or natural emissions, could be optimally estimated (Stouffer et al., 2017). More recently, the CMIP Phase 6 (CMIP6) employed a number of the most updated global climate models and endorsed 21 individually designed MIPs to address various scientific questions (Eyring et al., 2016). Guided by the goals to facilitate integrated research on the impact of future scenarios over natural and human systems and to help quantify uncertainties in future projections based on multi-model simulations, the Scenario Model Intercomparison Project (ScenarioMIP; O'Neill et al., 2016) incorporates a broad range of future scenarios with various combinations of Representative Concentration Pathways (RCPs) which were initially adopted in CMIP5 and newer Shared Socioeconomic Pathways (SSPs). These integrations allow a comprehensive assessment of plausible future climate conditions covering a wide span of mitigation and adaptation options (Riahi et al., 2017; van Vuuren et al., 2014) and represent the most updated understanding of climate change and the carbon cycle in the next few decades (Eyring et al., 2016; O'Neill et al., 2016). The CMIP6 ScenarioMIP takes advantage of previous CMIP resources and makes advancements in two major updates: first, the climate models employed are more updated, with better representations of underlying physical processes, and second, the models are driven by a new set of emission pathways and land use scenarios, i.e., SSPs generated by updated versions of integrated assessment models (IAMs) with new conceptual designs of future societal development and evolution with different assumptions on the challenges to mitigation and adaptation to the climate change (O'Neill et al., 2016). The variety of SSP and RCP combinations also covers a broader range of air pollutant emissions which are supposed to bridge the gap of relatively narrow aerosol scenarios adopted in CMIP5 (Stouffer et al., 2017).
The goal of this study is thus to answer the following questions based on the CMIP6 ScenarioMIP results: (a) what is the future trajectory (spatial and temporal patterns) of global and NHL terrestrial carbon fluxes, in particular the net flux between the photosynthetic and respirational carbon fluxes, i.e., the net ecosystem productivity (NEP); (b) what is the relative role of NHLs in global terrestrial ecosystem NEP; and (c) what is the magnitude of the model uncertainties related to the answers to the first two questions?
We used NEP at both global and NHL (poleward of 50∘ N) scales from existing CMIP6 outputs in this study. For diagnosing purposes, we also analyzed the net primary productivity (NPP) and heterotrophic respiration (Rh), since they represent the two primary components of NEP: net plant carbon uptake and respirational carbon loss due to microbial decomposition, as NEP = NPP − Rh. These model outputs were obtained from the Earth System Grid Federation (ESGF) (https://esgf-node.llnl.gov/search/cmip6/, accessed on 1 October 2021), which unified the standardization to provide data access to various model outputs. Each model in CMIP6 was conducted with an ensemble of simulations with different initial conditions, which were categorized and labeled with four variant indices: the realization index (r), the initialization index (i), the physics index (p), and the forcing index (f) (Eyring et al., 2016; Petrie et al., 2021). To uniformly control the model conditions in case of unexpected uncertainties, we confined the selection of model outputs to experiments with all variant indices labeled with “1”, i.e., “r1i1p1f1”, for consistency. In particular, the ScenarioMIP experiments endorsed a set of future global change scenarios, i.e., the combinations of SSPs and RCPs, to represent the alternative evolutions of societal development, emissions, and concentrations (O'Neill et al., 2016). The RCPs are a set of four future greenhouse gas emission pathways in which the end-of-century radiative forcing approaches four target levels (2.6, 4.5, 6.0, and 8.5 W m−2), i.e., RCP2.6, RCP4.5, RCP3.7, and RCP8.5 (van Vuuren et al., 2011). The four target forcing levels are set to be realized by altering future greenhouse gas emissions and by changing underlying socioeconomic projections. The SSPs were developed to describe a set of five future global socioeconomic development scenarios (SSP1 to SSP5). Four future scenarios with different SSP and RCP combinations, including SSP1+RCP2.6 (SSP126), SSP2+RCP4.5 (SSP245), SSP3+RCP7.0 (SSP370), and SSP5+RCP 8.5 (SSP585), were considered in this study to cover a variety of future climate change projections. Overall, 10 models were selected in this study, i.e., the ACCESS-ESM1-5 (Ziehn et al., 2020), BCC_CSM2-MR (Wu et al., 2019), CanESM5 (Swart et al., 2019a), NorESM2-LM (Seland et al., 2020), NorESM2-MM (Seland et al., 2020), CESM2-WACCM (Gettelman et al., 2019; Lawrence et al., 2019), CMCC-CM2-SR5 (Cherchi et al., 2019), EC-Earth3-Veg (Wyser et al., 2020), IPSL-CM6A-LR (Dufresne et al., 2013), and MPI-ESM1-2-LR (Mauritsen et al., 2019; Reick et al., 2013). The NorESM2-LM and NorESM2-MM share the same horizontal resolution of ocean and sea ice but differ in the horizontal resolution of land and atmosphere and vary in some parameter settings in the atmosphere component. The detailed information with land and atmosphere components and spatial resolutions, as well as key relevant model features, is listed in Table 1.
Table 1The CMIP6 models analyzed in this study, the model land and atmosphere components, spatial resolutions, and key relevant model features are listed.
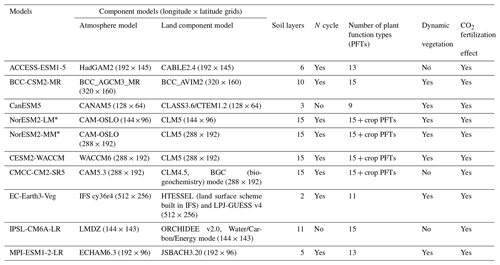
* The same models but run at different spatial resolutions.
We used monthly NEP, NPP, Rh, 2 m air temperature (TAS), and atmospheric CO2 concentration from the 10 CMIP6 models over the historical period (1980–2014) and the four future scenarios (2015–2100) in our analyses. The area-weighted sum of NEP, NPP, Rh, and NBP (net biosphere productivity), as well as area-weighted mean of TAS from different models and scenarios at global and NHL scales, was calculated. Non-land fractions of grid cells were excluded in the calculation. The bottom layer (i.e., the layer nearest to the land surface) atmospheric CO2 concentration was aggregated into global and NHL scales, too. Note that only 4 out of the 10 models have available CO2 data to date. The calculated monthly values from original outputs were further aggregated into the yearly scale for analysis. The annual model outputs with various spatial resolutions were resampled based on the model grids of BCC-CSM2-MR with a grid resolution of around 1∘ (mesh size: 320×160) for generating the spatial trend maps. The ensemble model projections and uncertainties in NEP, NPP, Rh, and TAS were evaluated by calculating the multi-model mean (μ) and standard deviation (SD, σ) of the yearly model outputs at both the global and NHL scales. Meanwhile, the contribution of model SD relative to the mean is quantified by the coefficient of variation (CV; CV ) to interpret the relative model uncertainty. We estimated the temporal trends of μ and SD using the linear least square regression method to quantitatively illustrate the ensemble model behavior against time. Additionally, the sensitivity analyses were performed by calculating the relative changes in carbon fluxes to their current levels (represented by the mean of 2010–2015) in response to the temperature rises at an increment of 1 ∘C (Pg C ∘C−1) or atmosphere CO2 concentration at an increment of 1 ppm (Pg C ppm−1) for each model at both the global and NHL scales. Finally, we evaluated trends of the NHL carbon flux changes relative to the global carbon flux changes under the future scenarios. The flux changes were calculated using the future annual carbon fluxes minus the 2015 carbon flux.
For the purpose of better understanding the uncertainties in CMIP6 future projections, we used the land carbon budget from the Global Carbon Project (GCP; Friedlingstein et al., 2020) to benchmark the CMIP6 estimates in the historical period (1980–2014), although this is not the main purpose of this study. Such comparison would be useful because it can infer the potential biases in CMIP6 projections if we consider GCP data as the most reliable estimates of the historical carbon budget. However, only 7 out of the 10 CMIP6 models output the NBP, which is the difference between NEP and disturbance-induced carbon loss (e.g., fire emissions) and land use change emissions. In addition, many models and GCP data do not provide the disturbance and land use change emissions separately, making it challenging to conduct a detailed comparison between the two data sources at a detailed level. Meanwhile, only global carbon budget was provided by GCP. Thus, we only compare NBP using the available data at the global scale.
3.1 Magnitudes of global and NHL NEP and NBP
Figure 1 shows the annual NEP in the historical (1980–2014) and future (2015–2100) periods under the 4 global change scenarios from the 10 CMIP6 models. On average, the CMIP6 models indicate a strong global terrestrial ecosystem NEP of 4.48 ± 0.54 Pg C yr−1 (annual mean ± interannual standard deviation) during the historical period, with a large spread across individual models (Fig. S1 in the Supplement). Meanwhile, the CMIP6 models suggest the global NBP of the seven available models (Fig. S2) to be 0.99 ± 0.68 Pg C yr−1. As a reference, the estimates from the GCP show the global terrestrial ecosystems as a consistent carbon sink during the historical period at 2.43 ± 0.97 Pg C yr−1, which is about half as much as the model ensemble mean NEP but higher than the NBP estimates. The models also estimate positive NHL NEPs as 0.56 ± 0.11 Pg C yr−1 during the historical period.
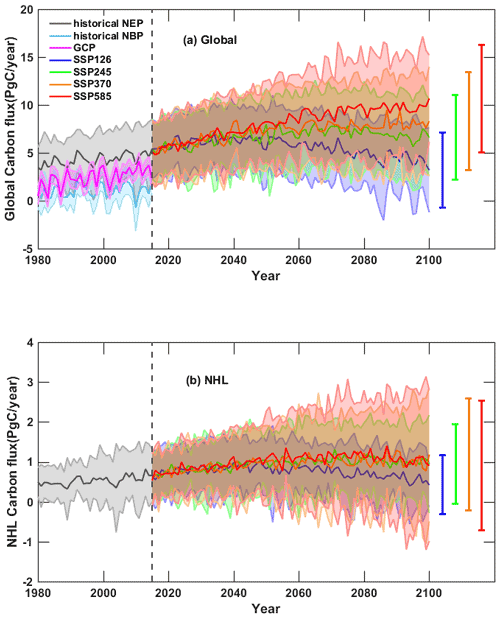
Figure 1The annual mean and SD of NEP of the 10 CMIP6 models during the historical period (1980–2014) and the future period (2015–2100) under four global change scenarios at the global (a) and northern-high-latitude (NHL) (b) scales. The shaded area indicates the SD values across the models. Error bars at the right of the panels show the mean SD of NEPs during 2095–2100 under each of the four scenarios.
Over the future years, the CMIP6 models generally suggest positive NEP over the global terrestrial ecosystems under all four scenarios (5.56 ± 0.88, 6.69 ± 0.78, 7.26 ± 0.98, and 8.13 ± 1.56 Pg C yr−1 for SSP126, SSP245, SSP370, and SSP585, respectively, according to the mean of the 10 models). For NHLs, the NEP is estimated as 0.79 ± 0.59, 0.95 ± 0.14, 0.94 ± 0.16, and 1.01 ± 0.18 Pg C yr−1 for SSP126, SSP245, SSP370, and SSP585, respectively. However, a few models indeed suggest the global terrestrial ecosystems with negative NEP at the end of the 21st century under SSP126, such as CanESM5 and EC-Earth3-Veg. In the NHLs, while most models suggest a positive NEP, BCC-CSM2-MR estimates a carbon source even though it shows the global ecosystem with a positive NEP, irrespective of the model scenarios.
3.2 Trends of global and NHL carbon fluxes in the 21st century
Relative to the average condition in 2015–2020, the CMIP6 models on average suggest that the global mean TAS will increase by 1.16, 2.45, 4.05, and 5.25 ∘C by the end of the 21st century (2095–2100) under SSP126, SSP245, SSP370, and SSP585, respectively. The growth of TAS in NHLs is projected to increase by 2.36, 4.41, 7.08, and 9.36 ∘C by the end of this century under the four scenarios, respectively, which are exclusively higher than the global levels (Fig. S3 and Table 2). The atmospheric CO2 concentrations are projected to increase at similar rates during 2015–2100 at global and NHL scales at 0.52, 2.36, 5.43, and 8.51 ppm yr−1 under SSP126, SSP245, SSP370, and SSP585, respectively (Fig. S6).
Table 2Future trends and percent changes relative to 2010–2014 for the multi-model mean NEP, NPP, Rh, and TAS as well as their uncertainties (SD across models) of the 10 CMIP6 models.
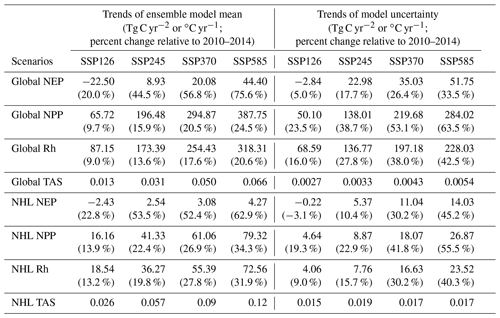
In response to the elevating temperature, NPP and Rh from the CMIP6 models (Figs. S4 and S5) show positive trends under all four scenarios, and the trends are larger under the warmer scenarios at both global and NHL scales. Global NPP will increase at rates of 65.72, 196.48, 294.87, and 387.75 Tg C yr−2 under SSP126, SSP245, SSP370, and SSP585, respectively. NHL NPP is projected to grow at rates of 16.16, 41.33, 61.06, and 79.32 Tg C yr−2 accordingly. Except SSP126, similarly positive but generally smaller trends were found for Rh at global scales (Fig. S5, Table 2) with rates of 87.15, 173.39, 254.43, and 318.31 Tg C yr−2 under the four scenarios. The NHL Rh trends are 18.64, 36.27, 55.39, and 72.56 Tg C yr−2. Normalized by the area, the growth rates are 0.44, 1.33, 1.99, and 2.62 g C yr−2 for global NPP over the four scenarios, respectively. The area-normalized growth rates in the NHL NPP are 0.54, 1.37, 2.03, and 2.63 g C yr−2, respectively. Area-normalized global Rh growth rates are 0.59, 1.17, 1.72, and 2.15 g C yr−2, while the area-normalized NHL Rh growth rates are 0.62, 1.20, 1.84, and 2.41 g C yr−2 under the four scenarios, respectively. These results indicate that the average NPP and Rh grow faster in the NHLs than at the global scale. The fast-growing Rh cancels a large part of the NPP growth and resulted in slowly growing NEPs.
CMIP6 models show a trend of NEP that first increases until the middle of the 21st century and then decreases at both NHL and global scales under SSP126. Overall, they show a slightly decreasing trend at NHL (−2.84 Tg C yr−2) and global (−22.50 Tg C yr−2) scales during 2015–2100 under SSP126. The trends are positive under SSP245 at 8.93 Tg C yr−2 at the global scale and 2.54 Tg C yr−2 for NHLs. Under SSP370 and SSP585, the positive trends become more prominent: they are 20.08 and 44.40 Tg C yr−2 at the global scale and 3.08 and 4.27 Tg C yr−2 in the NHL under SSP370 and SSP585, respectively.
3.3 Divergent carbon flux estimations among the CMIP6 models
Large uncertainties in estimated global and NHL NEP were found, measured by the standard deviation (SD) across the CMIP6 models. The average SD for global NEP over the historical period was 2.85 Pg C yr−1, and it will expand to 3.96, 4.51, 5.44, and 5.60 Pg C yr−1 by the end of the 21st century under SSP126, SSP245, SSP370, and SSP585, respectively. Specifically, the model uncertainties in global and NHL NEP are preserved under SSP126, with small shrinking trends of SD values (−2.84 and −0.22 Tg C yr−2 for global and NHL scales, respectively; Table 2). For SSP245, SSP370, and SSP585, the model uncertainties tend to expand towards the end of this century for both global and NHL scales. The model uncertainties are the largest under SSP370 and SSP585. Globally, the mean NEP values for SSP370 and SSP585 are 6.08 and 7.77 Pg C yr−1, respectively, during 2095–2100, with concomitant large SDs of 7.84 Pg C yr−1 (CV = 129 %) and 8.53 Pg C yr−1 (CV = 109.78 %). It is worth noting that the mean NEP values for SSP370 and SSP585 in NHLs are 0.77 and 0.84 Pg C yr−1, respectively, during 2095–2100, while the SDs are relatively large: 1.64 Pg C yr−1 (CV = 213.00 %) and 1.86 Pg C yr−1 (CV = 221.43 %) accordingly. Similarly, large uncertainties for NPP and Rh were identified. The average SDs for global and NHL NPP over the historical period were 14.89 and 1.51 Pg C yr−1, respectively, and they are projected to expand at rates of 50.10, 138.01, 219.68, and 284.02 Tg C yr−1 (global) and 4.64, 8.87, 18.07, and 26.87 Tg C yr−1 (NHLs) under SSP126, SSP245, SSP370, and SSP585, respectively. For Rh, the global and NHL average SDs over the historical period were 16.15 and 1.66 Pg C yr−1, respectively, and they are projected to expand at rates of 18.54, 36.27, 55.39, and 72.56 Tg C yr−1 (global) and 4.06, 7.76, 16.63, and 23.52 Tg C yr−1 (NHLs) under SSP126, SSP245, SSP370, and SSP585, respectively.
The large uncertainties in NEP are likely due to the uncertain responses of NPP and Rh to the temperature changes and CO2 fertilization effects in each model. The SDs of TAS projections by the end of the 21st century are 2.52, 2.79, 2.68, and 2.71 ∘C in the NHLs, which are much larger than those of global TAS at 0.83, 0.84, 1.04, and 1.27 ∘C, under SSP126, SSP245, SSP370, and SSP585, respectively. As shown in Fig. 2, the CMIP6-estimated annual carbon fluxes have strong linear relationships to TAS. For NPP, a 1 ∘C increase in global TAS corresponds to an increase in global annual NPP from 0.47 to 13.34 Pg C yr−1; in the NHLs, the range spans from 0.28 to 0.95 Pg C yr−1. Global annual Rh will increase at rates from 1.06 to 11.12 Pg C per 1 ∘C increase in global TAS, and the rates are between 0.28 and 1.29 Pg C yr−1 for the NHL annual Rh. All the lowest sensitivities are estimated by ACCESS-ESM-1-5, and the highest sensitivities are from CanESM5. As the residual of NPP and Rh, the sensitivities of NEP to TAS are more complicated: the global annual NEP will change at a rate between −0.59 (by ACCESS-ESM-1-5) and 2.21 Pg C yr−1 (by CanESM5) per 1 ∘C increase in global TAS, and the changing rates are between −0.37 (by BCC-CSM2-MR) and 0.23 Pg C yr−1 (by CanESM5) for the NHL annual NEP. The carbon fluxes vs. CO2 concentration and carbon fluxes vs. temperature rise demonstrate similar linear relationships, as shown in Fig. 3. The global NPP increases at a rate of 0.037 Pg C yr−1 per part per million CO2 concentration rise by IPSL-CM6A-LR to 0.064 Pg C yr−1 by BCC-CSM2-MR, and NHL NPP increases at a rate of 0.008 Pg C yr−1 by MPI-ESM1-2-LR to 0.011 Pg C yr−1 by BCC-CSM2-MR. The global Rh increases at a rate of 0.030 Pg C yr−1 per part per million CO2 concentration rise by IPSL-CM6A-LR to 0.058 Pg C yr−1 by BCC-CSM2-MR globally and from 0.007 Pg C yr−1 by IPSL-CM6A-LR to 0.015 Pg C yr−1 by BCC-CSM2-MR at the NHL scale. The NEP shows contrasting trends at the two different spatial scales relative to the CO2 concentration rise by BCC-CSM2-MR: at the global scale, NEP is positively correlated with CO2 concentration, while at the NHL scale they are negatively correlated. The other three models show slightly positive trends of NEP fluxes relative to the CO2 concentration rise at both scales. There remains a strong linear relationship between TAS and atmospheric CO2 concentrations irrespective of the model scenarios (Fig. S7), which could explain the similar trend patterns of carbon flux change in response to the TAS and CO2 concentration rise in Figs. 2 and 3.
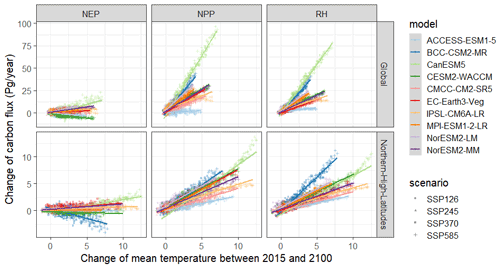
Figure 2Sensitivity of carbon flux changes in response to the TAS changes (relative to the 2015 values) at global and NHL scales for each CMIP6 model under the four future scenarios.
3.4 Latitudinal distributions of NEP
Figure 4 shows average NEP in the 10∘ latitudinal bins between 60∘ S and 90∘ N in the historical, the early (2015–2024), the middle (2050–2059), and the end (2091–2100) decades of the 21st century under the four scenarios. Overall, the global ecosystems are projected as a stronger carbon sink under SSP245, SSP370, and SSP585 than the historical period for most of the latitudes except the polar region (> 80∘ N), where the NEP remains relatively constant. Under SSP126, there is a drawdown during 2091–2100 between 20∘ S and 10∘ N. Among all the latitudinal bins, the tropical regions near the Equator act as the largest carbon sink with the highest uncertainties. However, the uncertainties at 60 and 70∘ N are exclusively larger relative to the absolute values of NEP in this region (i.e., the CV values), which are 109.44 % and 264.11 % under SSP126, 86.37 % and 173.89 % under SSP245, 106.92 % and 364.27 % under SSP370, and 119.60 % and 484.50 % under SSP585, compared with those near the Equator of 100.32 %, 58.94 %, 80.46 %, and 54.58 % for the four future scenarios, accordingly.
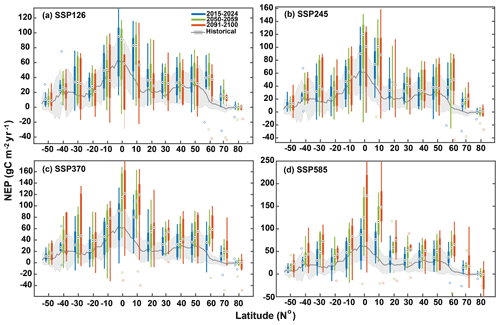
Figure 4Latitudinal distributions of NEP in the historical period and under different future scenarios. The gray lines with bands are the historical multi-model mean and uncertainties in NEP. The boxplots are the future NEP distributed in each 10∘ bin between 60∘ S and 90∘ N under (a) SSP126, (b) SSP245, (c) SSP370, and (d) SSP585, during the early (2015–2024), the middle (2050–2059), and the end (2091–2100) decades of the 21st century.
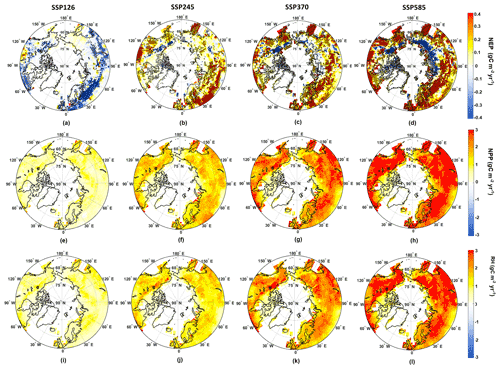
Figure 5The spatial distributions of the trends of NHL carbon fluxes under different future scenarios. The rows of the panels are NEP, NPP, and Rh from top to bottom, and the columns of the panels are SSP126, SSP245, SSP370, and SSP585 from left to right. The unit is grams of carbon per square meter per year. The black dots on the NEP maps denote significance of the regression values (p < 0.05) when fitting the carbon flux trends within each grid. Most of the model grids show significance of the regression for NPP and Rh and are not shown on the maps.
3.5 Spatial pattern of trends of NHL carbon fluxes
According to the average of CMIP6 models, Fig. 5 shows significant positive trends of NPP and Rh but mixed trends of NEP in the NHLs under all of the four scenarios. With growing radiative forcing or temperature from SSP126 to SSP585, the positive trends of NPP and Rh increase everywhere in the NHLs. The spatial pattern of NEP trends is more complicated. Under SSP126, most of the forested area in the NHLs are projected to have significantly decreasing NEP, while the other regions show no significant trends. More area starts to have significantly positive and larger NEP trends from SSP126 to SSP245 and SSP370 in response to larger radiative forcing levels. Under SSP585, which shows the highest level of radiative forcing and global warming, most of the NHL NEP, particularly areas covered by forest, is projected to have significant positive trends, while the NEP in the tundra area of northern Canada and Siberia in contrast has significant negative trends.
3.6 The role of NHLs in future global carbon flux changes
The CMIP6 models show a consistent positive contribution of the NHLs to the global carbon flux changes since 2015, measured by slopes of linear regression models between the NHL and global numbers (Fig. 6). On average, the CMIP6 models estimate that NHLs contribute 16 % of global NPP increase under SSP126 and 20 % under the other three scenarios and contribute 23 %–26 % of global Rh increase under the four scenarios. For NEP, the NHLs' contributions are between 7 % and 11 %. However, it is worth noting that some of these contributions are with high uncertainties from different models. For example, CanESM5 generally projects the largest increases in global and NHL NPP and Rh but stands out to suggest the lowest NHL contribution (i.e., the smallest slopes) to global NPP and Rh. The uncertainties (measured by the standard deviation of the slopes estimated by the 10 models) are relatively lower for NPP and Rh and scenarios with lower radiative forcing levels but become high for NEP under high-radiative-forcing scenarios. For instance, the uncertainties could be as high as 5 times the contribution estimated by the multi-model means for NEP under SSP370 and SSP585.
In this analysis, we present the quantification of the future magnitudes, trends, patterns, and uncertainties in terrestrial ecosystem carbon fluxes from an ensemble of 10 CMIP6 models, with a particular focus on the Arctic–boreal regions in the northern high latitudes. The CMIP6 models estimate the global terrestrial ecosystems as a strong carbon sink but with a magnitude that is 2.06 Pg yr−1, or 85 % higher than the estimates from the benchmarking global carbon project, suggesting consideration of bias corrections when using CMIP6 modeled carbon fluxes for other applications, particularly those sensitive to the magnitude of these carbon fluxes.
On average, the CMIP6 models project large increases in NPP and Rh in the global and NHL terrestrial ecosystems in the future, while the NHLs are projected to grow 1.43, 1.13, 1.31, and 1.40 times faster for NPP and 1.47, 1.46, 1.58, and 1.55 times faster for Rh under SSP126, SSP245, SSP370, and SSP585 than those at the global scale (Table 2). This is because of the faster increase in temperature, larger CO2 fertilization effect, and higher sensitivities to the warming climate (Fig. 2) in the NHLs. Such concurrently rising NPP and Rh were widely evidenced and discussed in previous literature. Jeong et al. (2018) showed that long-term measurements of CO2 revealed increasing carbon cycling rates and decreasing soil carbon residence time in the Arctic. On one hand, greening of the world was widely identified due to more favorable vegetation growth conditions promoted by a warming climate (Piao et al., 2020; Zhu et al., 2016), and warmer temperature and CO2 fertilization were revealed to enhance the terrestrial gross primary production in the NHLs (Liang et al., 2018; Myers-Smith et al., 2020; Wenzel et al., 2016). On the other hand, the increases in Rh in response to temperature rise could be attributed to two major reasons (Bond-Lamberty et al., 2018). One reason for the rising Rh could result from more active soil bacteria metabolism, and thus enhanced soil organic matter (SOM) mineralization due to rising temperature (Crowther et al., 2016; Lu et al., 2013). The second reason could be the more abundant availability of substrates for metabolism from accelerated ecosystem carbon uptake and debris production (Bond-Lamberty et al., 2018).
The terrestrial ecosystem carbon cycle is complex, and many past and ongoing ecological studies sought to understand the underlying mechanisms. Long-term measurements at FLUXNET sites have evidenced greater bioavailable carbon stock due to the faster increasing gross primary production than the concurrent rises in ecosystem respiration in response to climate change (Falge et al., 2002). However, contradictory conclusions were drawn in some regions of the world where reduced soil carbon stocks were found due to more carbon efflux than influx (Naidu and Bagchi, 2021). The case of the NHLs is even more special, partly because the biological processes such as the vegetation phenology and soil decomposition are especially sensitive to climate change due to the extremely cold environment and the relatively faster temperature change rates (McGuire et al., 2009; Richardson et al., 2018). The thawing of permafrost is changing the soil water balance and increasing the thickness of the active layer, which renders the ancient carbon under potential decomposition (Belshe et al., 2012; Schuur et al., 2015; Schuur and Abbott, 2011). Moreover, the terrestrial carbon fluxes are influenced by the evolutions of various other climate factors, such as precipitation, soil moisture, and atmospheric nitrogen deposition (Naidu and Bagchi, 2021; Sierra et al., 2015; Yue et al., 2017). Besides the disturbance-induced carbon loss, the carbon balance in the terrestrial ecosystems will be determined by the difference between rising primary productivity and the accelerated soil carbon decomposition driven by the interplay of multiple climate drivers (McKane et al., 1997; Sistla et al., 2013). These complex processes have been reflected in the results of our CMIP6 analysis. As the residual between the carbon influx (NPP) and efflux (Rh), global and NHL NEP are projected to have more complicated changing patterns. The global and NHL NEP are growingly positive in the future, but at lower rates than NPP and Rh. While global NEP is generally higher under warmer scenarios, NHL NEP will be at similar levels by the end of the 21st century under different warming levels (e.g., SSP245, SSP370, SSP585; Fig. 1). This is partially due to the varying response of different ecosystems to the warming climate, as forest-dominated area is becoming a larger carbon sink, and tundra-dominated area is likely becoming a stronger carbon source (Fig. 4).
Yet, it is important to note that there remain large uncertainties in the magnitudes and trends of the carbon balance in the global and NHL terrestrial ecosystems. The underlying carbon cycling processes are difficult to quantify and are poorly constrained in current ESMs (Bradford et al., 2016). Sensitivities of carbon fluxes in ESMs are divergent in responses to different climate change drivers (e.g., Figs. 2 and 3), such that model uncertainties are pronounced in various aspects (Bradford et al., 2016). Although different land surface models share similar carbon flux transfer mechanisms among different carbon pools, they are diversified in the pool structures (Shao et al., 2013; Yan et al., 2014) and parameterizations (Luo and Schuur, 2020). For example, CMIP6 models were found to inaccurately estimate leaf area index (LAI), an essential biophysical variable that drives the carbon cycle and many other ecological processes (Song et al., 2021). Song et al. (2021) suggested that most CMIP6 models were not able to correctly reproduce the magnitudes of short- to long-term temporal variability in LAI, although they showed improvement in estimating seasonal LAI variations compared with CMIP5 models. Moreover, they revealed that most of the CMIP6 models overestimated the LAI in non-forested vegetation areas against observations, which largely contributed to the general overestimation of the global mean LAI. While it is hard to distinguish how the improvements of LAI estimation in CMIP6 models might contribute to their performance in estimating carbon fluxes, their physical linkages are clear. Any underestimation of LAI usually leads to lower NPP estimations and possibly higher Rh due to the lower LAI-induced cooling effects on the soil and therefore may result in lower NEP. Better seasonal variation in LAI may indicate better capture of the growing season length of vegetation and the annual carbon budget (Piao et al., 2019). In addition, the categorizations of plant functional types (PFTs) are also different among the 10 ESMs (Table 1); for example, CanESM5 has 9 PFTs, while CESM2-WACCM has 15 PFTs plus additional crop types. Most models have the nitrogen cycles coupled with carbon cycles with the exception of CanESM5 and IPSL-CM6A-LR (Table 1). For compensating the effects of nutrient limitation, IPSL-CM6A-LR adopts the downregulation function to limit the maximum photosynthesis rates to account for nutrient limitations (Boucher et al., 2020), while the CanESM5 has no nutrient limitations accounted for (Swart et al., 2019a). This could be one of the reasons CanESM5 has the largest sensitivities of NPP and Rh fluxes in response to climate change (Fig. 2). Comprehensive and standard validations of multiple variables are needed to assess the model performance and uncertainties in biogeochemical simulations across CMIP6 models (Spafford and MacDougall, 2021).
In our analysis, the uncertainties in the carbon fluxes across the CMIP6 models tend to increase over time, and they grow faster under warmer scenarios. The NHL NEP has more relative uncertainties as opposed to the mean compared with global NEP, and this difference is more pronounced in scenarios with higher radiative forcing levels. By 2100, the CMIP6 models suggest the NHLs to be a carbon sink of 0.54 ± 0.77, 1.01 ± 0.98, 0.97 ± 1.62, and 1.05 ± 1.83 Pg C yr−1 under SSP126, SSP245, SSP370, and SSP585, respectively, which are exclusively larger than the previous C4MIP results under the IPCC SRES A2 scenario with a temperature rise of approximately 3.4 (2.0–5.4) ∘C by 2100 (0.3 ± 0.3 Pg C yr−1; Qian et al., 2010). The relative uncertainties (SD, mean) for the four scenarios are 143.59 %, 97.03 %, 167.01 %, and 174.29 %, which are at levels similar to or larger than the C4MIP results (100 %), indicating the uncertainty level is not reduced in the new models. Moreover, models show distinct sensitivities of carbon fluxes in response to the future temperature rise. While NPP and Rh show a uniformly positive response to temperature rise, NEP changes could be either positive or negative for different models. The uncertainties in soil carbon dynamics and various projections of soil carbon stock and changes in different CMIP5 models were broadly evaluated and discussed in previous studies (Friedlingstein et al., 2014; Todd-Brown et al., 2013, 2014; Yan et al., 2014). Recent evaluations of soil carbon stock and sequestration of CMIP6-LUMIP models also showed large differences among different CMIP6 models, which in another way indicates the possible uncertainties in soil carbon dynamics stemming from simulation of the land use impacts in different CMIP6 models (Ito et al., 2020). All the CMIP6 model results presented in this analysis do predict rising NPP and Rh in response to temperature rise in the future, but with divergent trends and patterns. Consequently, large uncertain or even irreconcilable NEP results in the NHLs are shown among different models.
The Climate Model Intercomparison Project is a major approach to quantifying and understanding the future terrestrial ecosystem carbon cycle and its interactions with the climate system. In this study, we present the trends and patterns of future projections of carbon fluxes (particularly the net ecosystem productivity) in the global and northern-high-latitude ecosystems from a set of the most up-to-date CMIP6 models. Based on the average of the CMIP6 models, our analysis showed that global and NHL ecosystems were and would continue to be carbon sinks, although large uncertainties were found for the size and trends of the carbon sinks among different CMIP6 models, which are not obviously attenuated compared with previous model intercomparison project results. Although the warming levels and sensitivity of ecosystems to the warming temperature are higher in the NHLs, the contribution of NHLs to the global NEP increase is small, however with larger relative uncertainties. The model uncertainties are pronounced in the historical simulations and are projected to expand more widely in the future under scenarios with larger radiative forcing levels. These results revealed the emergent necessity to make endeavors to bridge the knowledge gaps between process parameterization and representations of various ESMs and the real-world processes, as well as to deepen the understanding of the underlying mechanisms of the feedforward and feedback roles of the NHL ecosystem in response to climate change.
The CMIP6 model results are publicly available on the ESGF website: https://doi.org/10.22033/ESGF/CMIP6.4320, Ziehn et al., 2019; https://doi.org/10.22033/ESGF/CMIP6.3028, Xin et al., 2019; https://doi.org/10.22033/ESGF/CMIP6.3683, Swart et al., 2019b; https://doi.org/10.22033/ESGF/CMIP6.8248, Seland et al., 2019; https://doi.org/10.22033/ESGF/CMIP6.8250, Bentsen et al., 2019; https://doi.org/10.22033/ESGF/CMIP6.10100, Danabasoglu, 2019; https://doi.org/10.22033/ESGF/CMIP6.3887, Lovato and Peano, 2020; https://doi.org/10.22033/ESGF/CMIP6.4876, EC-Earth Consortium, 2019; https://doi.org/10.22033/ESGF/CMIP6.5262, Boucher et al., 2019; https://doi.org/10.22033/ESGF/CMIP6.6690, Wieners et al., 2019;
The codes for processing the data and generating the figures are available at https://github.com/qhgogogo/CMIP6-carbonflux (last access: 17 May 2021; https://doi.org/10.5281/zenodo.4768532, Qiu, 2021).
The supplement related to this article is available online at: https://doi.org/10.5194/esd-14-1-2023-supplement.
HQ and MC designed the study, processed the data, and prepared the original manuscript. DH, YZ, and XZ contributed subsequent analysis and helped write and edit the manuscript.
The contact author has declared that none of the authors has any competing interests.
Publisher’s note: Copernicus Publications remains neutral with regard to jurisdictional claims in published maps and institutional affiliations.
We acknowledge the World Climate Research Programme, which, through its Working Group on Coupled Modelling, coordinated and promoted CMIP6. We thank the climate modeling groups for producing and making available their model output, the Earth System Grid Federation (ESGF) for archiving the data and providing access, and the multiple funding agencies who support CMIP6 and ESGF, as well as the efforts of all involved modeling centers.
This research has been supported by the National Aeronautics and Space Administration (grant no. NNH18ZDA001N, award no. 80HQTR19T0055).
This paper was edited by Anping Chen and reviewed by Xu Lian and one anonymous referee.
Belshe, E. F., Schuur, E. A. G., Bolker, B. M., and Bracho, R.: Incorporating spatial heterogeneity created by permafrost thaw into a landscape carbon estimate, J. Geophys. Res.-Biogeo., 117, G01026, https://doi.org/10.1029/2011JG001836, 2012.
Berner, L. T., Massey, R., Jantz, P., Forbes, B. C., Macias-Fauria, M., Myers-Smith, I., Kumpula, T., Gauthier, G., Andreu-Hayles, L., Gaglioti, B. V., Burns, P., Zetterberg, P., D'Arrigo, R., and Goetz, S. J.: Summer warming explains widespread but not uniform greening in the Arctic tundra biome, Nat. Commun., 11, 4621, https://doi.org/10.1038/s41467-020-18479-5, 2020.
Bentsen, M., Oliviè, D. J. L., Seland, Ø., Toniazzo, T., Gjermundsen, A., Graff, L. S., Debernard, J. B., Gupta, A. K., He, Y., Kirkevåg, A., Schwinger, J., Tjiputra, J., Aas, K. S., Bethke, I., Fan, Y., Griesfeller, J., Grini, A., Guo, C., Ilicak, M., Karset, I. H. H., Landgren, O. A., Liakka, J., Moseid, K. O., Nummelin, A., Spensberger, C., Tang, H., Zhang, Z., Heinze, C., Iversen, T., and Schulz, M.: NCC NorESM2-MM model output prepared for CMIP6 ScenarioMIP, Earth System Grid Federation [data set], https://doi.org/10.22033/ESGF/CMIP6.8250, 2019.
Bond-Lamberty, B., Bailey, V. L., Chen, M., Gough, C. M., and Vargas, R.: Globally rising soil heterotrophic respiration over recent decades, Nature, 560, 80–83, https://doi.org/10.1038/s41586-018-0358-x, 2018.
Boucher, O., Denvil, S., Levavasseur, G., Cozic, A., Caubel, A., Foujols, M.-A., Meurdesoif, Y., Cadule, P., Devilliers, M., Dupont, E., and Lurton, T.: IPSL IPSL-CM6A-LR model output prepared for CMIP6 ScenarioMIP, Earth System Grid Federation [data set], https://doi.org/10.22033/ESGF/CMIP6.5262, 2019.
Boucher, O., Servonnat, J., Albright, A. L., Aumont, O., Balkanski, Y., Bastrikov, V., Bekki, S., Bonnet, R., Bony, S., Bopp, L., Braconnot, P., Brockmann, P., Cadule, P., Caubel, A., Cheruy, F., Codron, F., Cozic, A., Cugnet, D., D'Andrea, F., Davini, P., Lavergne, C. de, Denvil, S., Deshayes, J., Devilliers, M., Ducharne, A., Dufresne, J.-L., Dupont, E., Éthé, C., Fairhead, L., Falletti, L., Flavoni, S., Foujols, M.-A., Gardoll, S., Gastineau, G., Ghattas, J., Grandpeix, J.-Y., Guenet, B., Guez, L., E., Guilyardi, E., Guimberteau, M., Hauglustaine, D., Hourdin, F., Idelkadi, A., Joussaume, S., Kageyama, M., Khodri, M., Krinner, G., Lebas, N., Levavasseur, G., Lévy, C., Li, L., Lott, F., Lurton, T., Luyssaert, S., Madec, G., Madeleine, J.-B., Maignan, F., Marchand, M., Marti, O., Mellul, L., Meurdesoif, Y., Mignot, J., Musat, I., Ottlé, C., Peylin, P., Planton, Y., Polcher, J., Rio, C., Rochetin, N., Rousset, C., Sepulchre, P., Sima, A., Swingedouw, D., Thiéblemont, R., Traore, A. K., Vancoppenolle, M., Vial, J., Vialard, J., Viovy, N., and Vuichard, N.: Presentation and Evaluation of the IPSL-CM6A-LR Climate Model, J. Adv. Model. Earth Sy., 12, e2019MS002010, https://doi.org/10.1029/2019MS002010, 2020.
Bradford, M. A., Wieder, W. R., Bonan, G. B., Fierer, N., Raymond, P. A., and Crowther, T. W.: Managing uncertainty in soil carbon feedbacks to climate change, Nat. Clim. Change, 6, 751–758, https://doi.org/10.1038/nclimate3071, 2016.
Campbell, J. E., Berry, J. A., Seibt, U., Smith, S. J., Montzka, S. A., Launois, T., Belviso, S., Bopp, L., and Laine, M.: Large historical growth in global terrestrial gross primary production, Nature, 544, 84–87, https://doi.org/10.1038/nature22030, 2017.
Cherchi, A., Fogli, P. G., Lovato, T., Peano, D., Iovino, D., Gualdi, S., Masina, S., Scoccimarro, E., Materia, S., Bellucci, A., and Navarra, A.: Global Mean Climate and Main Patterns of Variability in the CMCC-CM2 Coupled Model, J. Adv. Model. Earth Sy., 11, 185–209, https://doi.org/10.1029/2018MS001369, 2019.
Collins, M., Knutti, R., Gutowski, W., Brooks, H., Shindell, D., and Webb, R.: Long-term Climate Change: Projections, Commitments and Irreversibility, Geological and Atmospheric Sciences Reports, in: Climate change 2013-The physical science basis: Contribution of working group I to the fifth assessment report of the intergovernmental panel on climate change, Cambridge University Press, 1029–1136, ISBN 9781107057991, 2013.
Crowther, T. W., Todd-Brown, K. E. O., Rowe, C. W., Wieder, W. R., Carey, J. C., Machmuller, M. B., Snoek, B. L., Fang, S., Zhou, G., Allison, S. D., Blair, J. M., Bridgham, S. D., Burton, A. J., Carrillo, Y., Reich, P. B., Clark, J. S., Classen, A. T., Dijkstra, F. A., Elberling, B., Emmett, B. A., Estiarte, M., Frey, S. D., Guo, J., Harte, J., Jiang, L., Johnson, B. R., Kröel-Dulay, G., Larsen, K. S., Laudon, H., Lavallee, J. M., Luo, Y., Lupascu, M., Ma, L. N., Marhan, S., Michelsen, A., Mohan, J., Niu, S., Pendall, E., Peñuelas, J., Pfeifer-Meister, L., Poll, C., Reinsch, S., Reynolds, L. L., Schmidt, I. K., Sistla, S., Sokol, N. W., Templer, P. H., Treseder, K. K., Welker, J. M., and Bradford, M. A.: Quantifying global soil carbon losses in response to warming, Nature, 540, 104–108, https://doi.org/10.1038/nature20150, 2016.
Danabasoglu, G.: NCAR CESM2-WACCM model output prepared for CMIP6 ScenarioMIP, Earth System Grid Federation [data set], https://doi.org/10.22033/ESGF/CMIP6.10100, 2019.
Dufresne, J.-L., Foujols, M.-A., Denvil, S., Caubel, A., Marti, O., Aumont, O., Balkanski, Y., Bekki, S., Bellenger, H., Benshila, R., Bony, S., Bopp, L., Braconnot, P., Brockmann, P., Cadule, P., Cheruy, F., Codron, F., Cozic, A., Cugnet, D., de Noblet, N., Duvel, J.-P., Ethé, C., Fairhead, L., Fichefet, T., Flavoni, S., Friedlingstein, P., Grandpeix, J.-Y., Guez, L., Guilyardi, E., Hauglustaine, D., Hourdin, F., Idelkadi, A., Ghattas, J., Joussaume, S., Kageyama, M., Krinner, G., Labetoulle, S., Lahellec, A., Lefebvre, M.-P., Lefevre, F., Levy, C., Li, Z. X., Lloyd, J., Lott, F., Madec, G., Mancip, M., Marchand, M., Masson, S., Meurdesoif, Y., Mignot, J., Musat, I., Parouty, S., Polcher, J., Rio, C., Schulz, M., Swingedouw, D., Szopa, S., Talandier, C., Terray, P., Viovy, N., and Vuichard, N.: Climate change projections using the IPSL-CM5 Earth System Model: from CMIP3 to CMIP5, Clim. Dynam., 40, 2123–2165, https://doi.org/10.1007/s00382-012-1636-1, 2013.
EC-Earth Consortium (EC-Earth): EC-Earth-Consortium EC-Earth3-Veg model output prepared for CMIP6 ScenarioMIP, Earth System Grid Federation [data set], https://doi.org/10.22033/ESGF/CMIP6.4876, 2019.
Eyring, V., Bony, S., Meehl, G. A., Senior, C. A., Stevens, B., Stouffer, R. J., and Taylor, K. E.: Overview of the Coupled Model Intercomparison Project Phase 6 (CMIP6) experimental design and organization, Geosci. Model Dev., 9, 1937–1958, https://doi.org/10.5194/gmd-9-1937-2016, 2016.
Falge, E., Baldocchi, D., Tenhunen, J., Aubinet, M., Bakwin, P., Berbigier, P., Bernhofer, C., Burba, G., Clement, R., Davis, K. J., Elbers, J. A., Goldstein, A. H., Grelle, A., Granier, A., Guðmundsson, J., Hollinger, D., Kowalski, A. S., Katul, G., Law, B. E., Malhi, Y., Meyers, T., Monson, R. K., Munger, J. W., Oechel, W., Paw U, K. T., Pilegaard, K., Rannik, Ü., Rebmann, C., Suyker, A., Valentini, R., Wilson, K., and Wofsy, S.: Seasonality of ecosystem respiration and gross primary production as derived from FLUXNET measurements, Agr. Forest Meteorol., 113, 53–74, https://doi.org/10.1016/S0168-1923(02)00102-8, 2002.
Fisher, J. B., Sikka, M., Oechel, W. C., Huntzinger, D. N., Melton, J. R., Koven, C. D., Ahlström, A., Arain, M. A., Baker, I., Chen, J. M., Ciais, P., Davidson, C., Dietze, M., El-Masri, B., Hayes, D., Huntingford, C., Jain, A. K., Levy, P. E., Lomas, M. R., Poulter, B., Price, D., Sahoo, A. K., Schaefer, K., Tian, H., Tomelleri, E., Verbeeck, H., Viovy, N., Wania, R., Zeng, N., and Miller, C. E.: Carbon cycle uncertainty in the Alaskan Arctic, Biogeosciences, 11, 4271–4288, https://doi.org/10.5194/bg-11-4271-2014, 2014.
Friedlingstein, P., Meinshausen, M., Arora, V. K., Jones, C. D., Anav, A., Liddicoat, S. K., and Knutti, R.: Uncertainties in CMIP5 Climate Projections due to Carbon Cycle Feedbacks, J. Climate, 27, 511–526, https://doi.org/10.1175/JCLI-D-12-00579.1, 2014.
Friedlingstein, P., O'Sullivan, M., Jones, M. W., Andrew, R. M., Hauck, J., Olsen, A., Peters, G. P., Peters, W., Pongratz, J., Sitch, S., Le Quéré, C., Canadell, J. G., Ciais, P., Jackson, R. B., Alin, S., Aragão, L. E. O. C., Arneth, A., Arora, V., Bates, N. R., Becker, M., Benoit-Cattin, A., Bittig, H. C., Bopp, L., Bultan, S., Chandra, N., Chevallier, F., Chini, L. P., Evans, W., Florentie, L., Forster, P. M., Gasser, T., Gehlen, M., Gilfillan, D., Gkritzalis, T., Gregor, L., Gruber, N., Harris, I., Hartung, K., Haverd, V., Houghton, R. A., Ilyina, T., Jain, A. K., Joetzjer, E., Kadono, K., Kato, E., Kitidis, V., Korsbakken, J. I., Landschützer, P., Lefèvre, N., Lenton, A., Lienert, S., Liu, Z., Lombardozzi, D., Marland, G., Metzl, N., Munro, D. R., Nabel, J. E. M. S., Nakaoka, S.-I., Niwa, Y., O'Brien, K., Ono, T., Palmer, P. I., Pierrot, D., Poulter, B., Resplandy, L., Robertson, E., Rödenbeck, C., Schwinger, J., Séférian, R., Skjelvan, I., Smith, A. J. P., Sutton, A. J., Tanhua, T., Tans, P. P., Tian, H., Tilbrook, B., van der Werf, G., Vuichard, N., Walker, A. P., Wanninkhof, R., Watson, A. J., Willis, D., Wiltshire, A. J., Yuan, W., Yue, X., and Zaehle, S.: Global Carbon Budget 2020, Earth Syst. Sci. Data, 12, 3269–3340, https://doi.org/10.5194/essd-12-3269-2020, 2020.
Gettelman, A., Mills, M. J., Kinnison, D. E., Garcia, R. R., Smith, A. K., Marsh, D. R., Tilmes, S., Vitt, F., Bardeen, C. G., McInerny, J., Liu, H.-L., Solomon, S. C., Polvani, L. M., Emmons, L. K., Lamarque, J.-F., Richter, J. H., Glanville, A. S., Bacmeister, J. T., Phillips, A. S., Neale, R. B., Simpson, I. R., DuVivier, A. K., Hodzic, A., and Randel, W. J.: The Whole Atmosphere Community Climate Model Version 6 (WACCM6), J. Geophys. Res.-Atmos., 124, 12380–12403, https://doi.org/10.1029/2019JD030943, 2019.
Hu, F. S., Higuera, P. E., Duffy, P., Chipman, M. L., Rocha, A. V., Young, A. M., Kelly, R., and Dietze, M. C.: Arctic tundra fires: natural variability and responses to climate change, Frontiers in Ecology and the Environment, 13, 369–377, https://doi.org/10.1890/150063, 2015.
Ito, A., Hajima, T., Lawrence, D. M., Brovkin, V., Delire, C., Guenet, B., Jones, C. D., Malyshev, S., Materia, S., McDermid, S. P., Peano, D., Pongratz, J., Robertson, E., Shevliakova, E., Vuichard, N., Wårlind, D., Wiltshire, A., and Ziehn, T.: Soil carbon sequestration simulated in CMIP6-LUMIP models: implications for climatic mitigation, Environ. Res. Lett., 15, 124061, https://doi.org/10.1088/1748-9326/abc912, 2020.
Jeong, S.-J., Bloom, A. A., Schimel, D., Sweeney, C., Parazoo, N. C., Medvigy, D., Schaepman-Strub, G., Zheng, C., Schwalm, C. R., Huntzinger, D. N., Michalak, A. M., and Miller, C. E.: Accelerating rates of Arctic carbon cycling revealed by long-term atmospheric CO2 measurements, Science Advances, 4, eaao1167, https://doi.org/10.1126/sciadv.aao1167, 2018.
Koven, C. D., Ringeval, B., Friedlingstein, P., Ciais, P., Cadule, P., Khvorostyanov, D., Krinner, G., and Tarnocai, C.: Permafrost carbon-climate feedbacks accelerate global warming, P. Natl. Acad. Sci. USA, 108, 14769–14774, https://doi.org/10.1073/pnas.1103910108, 2011.
Lara, M. J., Nitze, I., Grosse, G., Martin, P., and McGuire, A. D.: Reduced arctic tundra productivity linked with landform and climate change interactions, Sci. Rep.-UK, 8, 2345, https://doi.org/10.1038/s41598-018-20692-8, 2018.
Lawrence, D. M., Fisher, R. A., Koven, C. D., Oleson, K. W., Swenson, S. C., Bonan, G., Collier, N., Ghimire, B., Kampenhout, L. van, Kennedy, D., Kluzek, E., Lawrence, P. J., Li, F., Li, H., Lombardozzi, D., Riley, W. J., Sacks, W. J., Shi, M., Vertenstein, M., Wieder, W. R., Xu, C., Ali, A. A., Badger, A. M., Bisht, G., Broeke, M. van den, Brunke, M. A., Burns, S. P., Buzan, J., Clark, M., Craig, A., Dahlin, K., Drewniak, B., Fisher, J. B., Flanner, M., Fox, A. M., Gentine, P., Hoffman, F., Keppel-Aleks, G., Knox, R., Kumar, S., Lenaerts, J., Leung, L. R., Lipscomb, W. H., Lu, Y., Pandey, A., Pelletier, J. D., Perket, J., Randerson, J. T., Ricciuto, D. M., Sanderson, B. M., Slater, A., Subin, Z. M., Tang, J., Thomas, R. Q., Martin, M. V., and Zeng, X.: The Community Land Model Version 5: Description of New Features, Benchmarking, and Impact of Forcing Uncertainty, J. Adv. Model. Earth Sy., 11, 4245–4287, https://doi.org/10.1029/2018MS001583, 2019.
Liang, J., Xia, J., Shi, Z., Jiang, L., Ma, S., Lu, X., Mauritz, M., Natali, S. M., Pegoraro, E., Penton, C. R., Plaza, C., Salmon, V. G., Celis, G., Cole, J. R., Konstantinidis, K. T., Tiedje, J. M., Zhou, J., Schuur, E. A. G., and Luo, Y.: Biotic responses buffer warming-induced soil organic carbon loss in Arctic tundra, Glob. Change Biol., 24, 4946–4959, https://doi.org/10.1111/gcb.14325, 2018.
Lovato, T. and Peano, D.: CMCC CMCC-CM2-SR5 model output prepared for CMIP6 ScenarioMI, Earth System Grid Federation [data set], https://doi.org/10.22033/ESGF/CMIP6.3887, 2020.
Lu, M., Zhou, X., Yang, Q., Li, H., Luo, Y., Fang, C., Chen, J., Yang, X., and Li, B.: Responses of ecosystem carbon cycle to experimental warming: a meta-analysis, Ecology, 94, 726–738, https://doi.org/10.1890/12-0279.1, 2013.
Luo, Y. and Schuur, E. A. G.: Model parameterization to represent processes at unresolved scales and changing properties of evolving systems, Glob. Change Biol., 26, 1109–1117, https://doi.org/10.1111/gcb.14939, 2020.
Luo, Y., Ahlström, A., Allison, S. D., Batjes, N. H., Brovkin, V., Carvalhais, N., Chappell, A., Ciais, P., Davidson, E. A., Finzi, A., Georgiou, K., Guenet, B., Hararuk, O., Harden, J. W., He, Y., Hopkins, F., Jiang, L., Koven, C., Jackson, R. B., Jones, C. D., Lara, M. J., Liang, J., McGuire, A. D., Parton, W., Peng, C., Randerson, J. T., Salazar, A., Sierra, C. A., Smith, M. J., Tian, H., Todd-Brown, K. E. O., Torn, M., Groenigen, K. J. van, Wang, Y. P., West, T. O., Wei, Y., Wieder, W. R., Xia, J., Xu, X., Xu, X., and Zhou, T.: Toward more realistic projections of soil carbon dynamics by Earth system models, Global Biogeochem. Cy., 30, 40–56, https://doi.org/10.1002/2015GB005239, 2016.
Mauritsen, T., Bader, J., Becker, T., Behrens, J., Bittner, M., Brokopf, R., Brovkin, V., Claussen, M., Crueger, T., Esch, M., Fast, I., Fiedler, S., Fläschner, D., Gayler, V., Giorgetta, M., Goll, D. S., Haak, H., Hagemann, S., Hedemann, C., Hohenegger, C., Ilyina, T., Jahns, T., Jimenéz-de-la-Cuesta, D., Jungclaus, J., Kleinen, T., Kloster, S., Kracher, D., Kinne, S., Kleberg, D., Lasslop, G., Kornblueh, L., Marotzke, J., Matei, D., Meraner, K., Mikolajewicz, U., Modali, K., Möbis, B., Müller, W. A., Nabel, J. E. M. S., Nam, C. C. W., Notz, D., Nyawira, S.-S., Paulsen, H., Peters, K., Pincus, R., Pohlmann, H., Pongratz, J., Popp, M., Raddatz, T. J., Rast, S., Redler, R., Reick, C. H., Rohrschneider, T., Schemann, V., Schmidt, H., Schnur, R., Schulzweida, U., Six, K. D., Stein, L., Stemmler, I., Stevens, B., Storch, J.-S. von, Tian, F., Voigt, A., Vrese, P., Wieners, K.-H., Wilkenskjeld, S., Winkler, A., and Roeckner, E.: Developments in the MPI-M Earth System Model version 1.2 (MPI-ESM1.2) and Its Response to Increasing CO2, J. Adv. Model. Earth Sy., 11, 998–1038, https://doi.org/10.1029/2018MS001400, 2019.
McGuire, A. D., Anderson, L. G., Christensen, T. R., Dallimore, S., Guo, L., Hayes, D. J., Heimann, M., Lorenson, T. D., Macdonald, R. W., and Roulet, N.: Sensitivity of the carbon cycle in the Arctic to climate change, Ecol. Monogr., 79, 523–555, https://doi.org/10.1890/08-2025.1, 2009.
McKane, R. B., Rastetter, E. B., Shaver, G. R., Nadelhoffer, K. J., Giblin, A. E., Laundre, J. A., and Chapin, F. S.: Climatic Effects on Tundra Carbon Storage Inferred from Experimental Data and a Model, Ecology, 78, 1170–1187, https://doi.org/10.1890/0012-9658(1997)078[1170:CEOTCS]2.0.CO;2, 1997.
Mekonnen, Z. A., Riley, W. J., Randerson, J. T., Grant, R. F., and Rogers, B. M.: Expansion of high-latitude deciduous forests driven by interactions between climate warming and fire, Nat. Plants, 5, 952–958, https://doi.org/10.1038/s41477-019-0495-8, 2019.
Myers-Smith, I. H., Kerby, J. T., Phoenix, G. K., Bjerke, J. W., Epstein, H. E., Assmann, J. J., John, C., Andreu-Hayles, L., Angers-Blondin, S., Beck, P. S. A., Berner, L. T., Bhatt, U. S., Bjorkman, A. D., Blok, D., Bryn, A., Christiansen, C. T., Cornelissen, J. H. C., Cunliffe, A. M., Elmendorf, S. C., Forbes, B. C., Goetz, S. J., Hollister, R. D., de Jong, R., Loranty, M. M., Macias-Fauria, M., Maseyk, K., Normand, S., Olofsson, J., Parker, T. C., Parmentier, F.-J. W., Post, E., Schaepman-Strub, G., Stordal, F., Sullivan, P. F., Thomas, H. J. D., Tømmervik, H., Treharne, R., Tweedie, C. E., Walker, D. A., Wilmking, M., and Wipf, S.: Complexity revealed in the greening of the Arctic, Nat. Clim. Change, 10, 106–117, https://doi.org/10.1038/s41558-019-0688-1, 2020.
Naidu, D. G. T. and Bagchi, S.: Greening of the earth does not compensate for rising soil heterotrophic respiration under climate change, Glob. Change Biol., 27, 2029–2038, https://doi.org/10.1111/gcb.15531, 2021.
Natali, S. M., Schuur, E. A. G., Webb, E. E., Pries, C. E. H., and Crummer, K. G.: Permafrost degradation stimulates carbon loss from experimentally warmed tundra, Ecology, 95, 602–608, https://doi.org/10.1890/13-0602.1, 2014.
O'Neill, B. C., Tebaldi, C., van Vuuren, D. P., Eyring, V., Friedlingstein, P., Hurtt, G., Knutti, R., Kriegler, E., Lamarque, J.-F., Lowe, J., Meehl, G. A., Moss, R., Riahi, K., and Sanderson, B. M.: The Scenario Model Intercomparison Project (ScenarioMIP) for CMIP6, Geosci. Model Dev., 9, 3461–3482, https://doi.org/10.5194/gmd-9-3461-2016, 2016.
Petrie, R., Denvil, S., Ames, S., Levavasseur, G., Fiore, S., Allen, C., Antonio, F., Berger, K., Bretonnière, P.-A., Cinquini, L., Dart, E., Dwarakanath, P., Druken, K., Evans, B., Franchistéguy, L., Gardoll, S., Gerbier, E., Greenslade, M., Hassell, D., Iwi, A., Juckes, M., Kindermann, S., Lacinski, L., Mirto, M., Nasser, A. B., Nassisi, P., Nienhouse, E., Nikonov, S., Nuzzo, A., Richards, C., Ridzwan, S., Rixen, M., Serradell, K., Snow, K., Stephens, A., Stockhause, M., Vahlenkamp, H., and Wagner, R.: Coordinating an operational data distribution network for CMIP6 data, Geosci. Model Dev., 14, 629–644, https://doi.org/10.5194/gmd-14-629-2021, 2021.
Phoenix, G. K. and Bjerke, J. W.: Arctic browning: extreme events and trends reversing arctic greening, Glob. Change Biol., 22, 2960–2962, https://doi.org/10.1111/gcb.13261, 2016.
Piao, S., Liu, Q., Chen, A., Janssens, I. A., Fu, Y., Dai, J., Liu, L., Lian, X., Shen, M., and Zhu, X.: Plant phenology and global climate change: Current progresses and challenges, Glob. Change Biol., 25, 1922–1940, https://doi.org/10.1111/gcb.14619, 2019.
Piao, S., Wang, X., Park, T., Chen, C., Lian, X., He, Y., Bjerke, J. W., Chen, A., Ciais, P., Tømmervik, H., Nemani, R. R., and Myneni, R. B.: Characteristics, drivers and feedbacks of global greening, Nature Reviews Earth & Environment, 1, 14–27, https://doi.org/10.1038/s43017-019-0001-x, 2020.
Qiu, H.: CMIP6-carbonflux, Zenodo [code], https://doi.org/10.5281/zenodo.4768532, 2021.
Qian, H., Joseph, R., and Zeng, N.: Enhanced terrestrial carbon uptake in the Northern High Latitudes in the 21st century from the Coupled Carbon Cycle Climate Model Intercomparison Project model projections, Glob. Change Biol., 16, 641–656, https://doi.org/10.1111/j.1365-2486.2009.01989.x, 2010.
Rantanen, M., Karpechko, A. Y., Lipponen, A., Nordling, K., Hyvärinen, O., Ruosteenoja, K., Vihma, T., and Laaksonen, A.: The Arctic has warmed nearly four times faster than the globe since 1979, Commun. Earth Environ., 3, 1–10, https://doi.org/10.1038/s43247-022-00498-3, 2022.
Reick, C. H., Raddatz, T., Brovkin, V., and Gayler, V.: Representation of natural and anthropogenic land cover change in MPI-ESM, J. Adv. Model. Earth Sy., 5, 459–482, https://doi.org/10.1002/jame.20022, 2013.
Riahi, K., van Vuuren, D. P., Kriegler, E., Edmonds, J., O'Neill, B. C., Fujimori, S., Bauer, N., Calvin, K., Dellink, R., Fricko, O., Lutz, W., Popp, A., Cuaresma, J. C., Kc, S., Leimbach, M., Jiang, L., Kram, T., Rao, S., Emmerling, J., Ebi, K., Hasegawa, T., Havlik, P., Humpenöder, F., Da Silva, L. A., Smith, S., Stehfest, E., Bosetti, V., Eom, J., Gernaat, D., Masui, T., Rogelj, J., Strefler, J., Drouet, L., Krey, V., Luderer, G., Harmsen, M., Takahashi, K., Baumstark, L., Doelman, J. C., Kainuma, M., Klimont, Z., Marangoni, G., Lotze-Campen, H., Obersteiner, M., Tabeau, A., and Tavoni, M.: The Shared Socioeconomic Pathways and their energy, land use, and greenhouse gas emissions implications: An overview, Global Environ. Chang., 42, 153–168, https://doi.org/10.1016/j.gloenvcha.2016.05.009, 2017.
Richardson, A. D., Hufkens, K., Milliman, T., Aubrecht, D. M., Furze, M. E., Seyednasrollah, B., Krassovski, M. B., Latimer, J. M., Nettles, W. R., Heiderman, R. R., Warren, J. M., and Hanson, P. J.: Ecosystem warming extends vegetation activity but heightens vulnerability to cold temperatures, Nature, 560, 368–371, https://doi.org/10.1038/s41586-018-0399-1, 2018.
Schaefer, K., Zhang, T., Bruhwiler, L., and Barrett, A. P.: Amount and timing of permafrost carbon release in response to climate warming, Tellus B, 63, 165–180, https://doi.org/10.1111/j.1600-0889.2011.00527.x, 2011.
Schuur, E. A. G. and Abbott, B.: High risk of permafrost thaw, Nature, 480, 32–33, https://doi.org/10.1038/480032a, 2011.
Schuur, E. A. G., McGuire, A. D., Schädel, C., Grosse, G., Harden, J. W., Hayes, D. J., Hugelius, G., Koven, C. D., Kuhry, P., Lawrence, D. M., Natali, S. M., Olefeldt, D., Romanovsky, V. E., Schaefer, K., Turetsky, M. R., Treat, C. C., and Vonk, J. E.: Climate change and the permafrost carbon feedback, Nature, 520, 171–179, https://doi.org/10.1038/nature14338, 2015.
Seland, Ø., Bentsen, M., Oliviè, D. J. L., Toniazzo, T., Gjermundsen, A., Graff, L. S., Debernard, J. B., Gupta, A. K., He, Y., Kirkevåg, A., Schwinger, J., Tjiputra, J., Aas, K. S., Bethke, I., Fan, Y., Griesfeller, J., Grini, A., Guo, C., Ilicak, M., Karset, I. H. H., Landgren, O. A., Liakka, J., Moseid, K. O., Nummelin, A., Spensberger, C., Tang, H., Zhang, Z., Heinze, C., Iversen, T., and Schulz, M.: NCC NorESM2-LM model output prepared for CMIP6 ScenarioMIP, Earth System Grid Federation [data set], https://doi.org/10.22033/ESGF/CMIP6.8248, 2019.
Seland, Ø., Bentsen, M., Olivié, D., Toniazzo, T., Gjermundsen, A., Graff, L. S., Debernard, J. B., Gupta, A. K., He, Y.-C., Kirkevåg, A., Schwinger, J., Tjiputra, J., Aas, K. S., Bethke, I., Fan, Y., Griesfeller, J., Grini, A., Guo, C., Ilicak, M., Karset, I. H. H., Landgren, O., Liakka, J., Moseid, K. O., Nummelin, A., Spensberger, C., Tang, H., Zhang, Z., Heinze, C., Iversen, T., and Schulz, M.: Overview of the Norwegian Earth System Model (NorESM2) and key climate response of CMIP6 DECK, historical, and scenario simulations, Geosci. Model Dev., 13, 6165–6200, https://doi.org/10.5194/gmd-13-6165-2020, 2020.
Shao, P., Zeng, X., Sakaguchi, K., Monson, R. K., and Zeng, X.: Terrestrial Carbon Cycle: Climate Relations in Eight CMIP5 Earth System Models, J. Climate, 26, 8744–8764, https://doi.org/10.1175/JCLI-D-12-00831.1, 2013.
Sierra, C. A., Trumbore, S. E., Davidson, E. A., Vicca, S., and Janssens, I.: Sensitivity of decomposition rates of soil organic matter with respect to simultaneous changes in temperature and moisture, J. Adv. Model. Earth Sy., 7, 335–356, https://doi.org/10.1002/2014MS000358, 2015.
Sistla, S. A., Moore, J. C., Simpson, R. T., Gough, L., Shaver, G. R., and Schimel, J. P.: Long-term warming restructures Arctic tundra without changing net soil carbon storage, Nature, 497, 615–618, https://doi.org/10.1038/nature12129, 2013.
Song, X., Wang, D.-Y., Li, F., and Zeng, X.-D.: Evaluating the performance of CMIP6 Earth system models in simulating global vegetation structure and distribution, Advances in Climate Change Research, 12, 584–595, https://doi.org/10.1016/j.accre.2021.06.008, 2021.
Spafford, L. and MacDougall, A. H.: Validation of terrestrial biogeochemistry in CMIP6 Earth system models: a review, Geosci. Model Dev., 14, 5863–5889, https://doi.org/10.5194/gmd-14-5863-2021, 2021.
Stouffer, R. J., Eyring, V., Meehl, G. A., Bony, S., Senior, C., Stevens, B., and Taylor, K. E.: CMIP5 Scientific Gaps and Recommendations for CMIP6, B. Am. Meteorol. Soc., 98, 95–105, https://doi.org/10.1175/BAMS-D-15-00013.1, 2017.
Swart, N. C., Cole, J. N. S., Kharin, V. V., Lazare, M., Scinocca, J. F., Gillett, N. P., Anstey, J., Arora, V., Christian, J. R., Hanna, S., Jiao, Y., Lee, W. G., Majaess, F., Saenko, O. A., Seiler, C., Seinen, C., Shao, A., Sigmond, M., Solheim, L., von Salzen, K., Yang, D., and Winter, B.: The Canadian Earth System Model version 5 (CanESM5.0.3), Geosci. Model Dev., 12, 4823–4873, https://doi.org/10.5194/gmd-12-4823-2019, 2019a.
Swart, N. C., Cole, J. N. S., Kharin, V. V., Lazare, M., Scinocca, J. F., Gillett, N. P., Anstey, J., Arora, V., Christian, J. R., Jiao, Y., Lee, W. G., Majaess, F., Saenko, O. A., Seiler, C., Seinen, C., Shao, A., Solheim, L., von Salzen, K., Yang, D., Winter, B., and Sigmond, M.: CCCma CanESM5 model output prepared for CMIP6 ScenarioMIP, Earth System Grid Federation [data set], https://doi.org/10.22033/ESGF/CMIP6.3683, 2019b.
Tarnocai, C., Canadell, J. G., Schuur, E. a. G., Kuhry, P., Mazhitova, G., and Zimov, S.: Soil organic carbon pools in the northern circumpolar permafrost region, Global Biogeochem. Cy., 23, GB2023, https://doi.org/10.1029/2008GB003327, 2009.
Taylor, K. E., Stouffer, R. J., and Meehl, G. A.: An Overview of CMIP5 and the Experiment Design, B. Am. Meteorol. Soc., 93, 485–498, https://doi.org/10.1175/BAMS-D-11-00094.1, 2012.
Todd-Brown, K. E. O., Randerson, J. T., Post, W. M., Hoffman, F. M., Tarnocai, C., Schuur, E. A. G., and Allison, S. D.: Causes of variation in soil carbon simulations from CMIP5 Earth system models and comparison with observations, Biogeosciences, 10, 1717–1736, https://doi.org/10.5194/bg-10-1717-2013, 2013.
Todd-Brown, K. E. O., Randerson, J. T., Hopkins, F., Arora, V., Hajima, T., Jones, C., Shevliakova, E., Tjiputra, J., Volodin, E., Wu, T., Zhang, Q., and Allison, S. D.: Changes in soil organic carbon storage predicted by Earth system models during the 21st century, Biogeosciences, 11, 2341–2356, https://doi.org/10.5194/bg-11-2341-2014, 2014.
van Vuuren, D. P., Edmonds, J., Kainuma, M., Riahi, K., Thomson, A., Hibbard, K., Hurtt, G. C., Kram, T., Krey, V., Lamarque, J.-F., Masui, T., Meinshausen, M., Nakicenovic, N., Smith, S. J., and Rose, S. K.: The representative concentration pathways: an overview, Climatic Change, 109, 5, https://doi.org/10.1007/s10584-011-0148-z, 2011.
van Vuuren, D. P., Kriegler, E., O'Neill, B. C., Ebi, K. L., Riahi, K., Carter, T. R., Edmonds, J., Hallegatte, S., Kram, T., Mathur, R., and Winkler, H.: A new scenario framework for Climate Change Research: scenario matrix architecture, Climatic Change, 122, 373–386, https://doi.org/10.1007/s10584-013-0906-1, 2014.
Wenzel, S., Cox, P. M., Eyring, V., and Friedlingstein, P.: Projected land photosynthesis constrained by changes in the seasonal cycle of atmospheric CO2, Nature, 538, 499–501, https://doi.org/10.1038/nature19772, 2016.
Whitman, E., Parisien, M.-A., Thompson, D. K., Hall, R. J., Skakun, R. S., and Flannigan, M. D.: Variability and drivers of burn severity in the northwestern Canadian boreal forest, Ecosphere, 9, e02128, https://doi.org/10.1002/ecs2.2128, 2018.
Wieners, K.-H., Giorgetta, M., Jungclaus, J., Reick, C., Esch, M., Bittner, M., Gayler, V., Haak, H., de Vrese, P., Raddatz, T., Mauritsen, T., von Storch, J.-S., Behrens, J., Brovkin, V., Claussen, M., Crueger, T., Fast, I., Fiedler, S., Hagemann, S., Hohenegger, C., Jahns, T., Kloster, S., Kinne, S., Lasslop, G., Kornblueh, L., Marotzke, J., Matei, D., Meraner, K., Mikolajewicz, U., Modali, K., Müller, W., Nabel, J., Notz, D., Peters-von Gehlen, K., Pincus, R., Pohlmann, H., Pongratz, J., Rast, S., Schmidt, H., Schnur, R., Schulzweida, U., Six, K., Stevens, B., Voigt, A., and Roeckner, E.: MPI-M MPI-ESM1.2-LR model output prepared for CMIP6 ScenarioMIP, Earth System Grid Federation [data set], https://doi.org/10.22033/ESGF/CMIP6.6690, 2019.
Wu, T., Lu, Y., Fang, Y., Xin, X., Li, L., Li, W., Jie, W., Zhang, J., Liu, Y., Zhang, L., Zhang, F., Zhang, Y., Wu, F., Li, J., Chu, M., Wang, Z., Shi, X., Liu, X., Wei, M., Huang, A., Zhang, Y., and Liu, X.: The Beijing Climate Center Climate System Model (BCC-CSM): the main progress from CMIP5 to CMIP6, Geosci. Model Dev., 12, 1573–1600, https://doi.org/10.5194/gmd-12-1573-2019, 2019.
Wyser, K., Kjellström, E., Koenigk, T., Martins, H., and Döscher, R.: Warmer climate projections in EC-Earth3-Veg: the role of changes in the greenhouse gas concentrations from CMIP5 to CMIP6, Environ. Res. Lett., 15, 054020, https://doi.org/10.1088/1748-9326/ab81c2, 2020.
Xin, X., Wu, T., Shi, X., Zhang, F., Li, J., Chu, M., Liu, Q., Yan, J., Ma, Q., and Wei, M.: BCC BCC-CSM2MR model output prepared for CMIP6 ScenarioMIP, Earth System Grid Federation [data set], https://doi.org/10.22033/ESGF/CMIP6.3028, 2019.
Yan, Y., Luo, Y., Zhou, X., and Chen, J.: Sources of variation in simulated ecosystem carbon storage capacity from the 5th Climate Model Intercomparison Project (CMIP5), Tellus B, 66, 22568, https://doi.org/10.3402/tellusb.v66.22568, 2014.
Yue, K., Fornara, D. A., Yang, W., Peng, Y., Peng, C., Liu, Z., and Wu, F.: Influence of multiple global change drivers on terrestrial carbon storage: additive effects are common, Ecol. Lett., 20, 663–672, https://doi.org/10.1111/ele.12767, 2017.
Zhu, Z., Piao, S., Myneni, R. B., Huang, M., Zeng, Z., Canadell, J. G., Ciais, P., Sitch, S., Friedlingstein, P., Arneth, A., Cao, C., Cheng, L., Kato, E., Koven, C., Li, Y., Lian, X., Liu, Y., Liu, R., Mao, J., Pan, Y., Peng, S., Peñuelas, J., Poulter, B., Pugh, T. A. M., Stocker, B. D., Viovy, N., Wang, X., Wang, Y., Xiao, Z., Yang, H., Zaehle, S., and Zeng, N.: Greening of the Earth and its drivers, Nat. Clim. Change, 6, 791–795, https://doi.org/10.1038/nclimate3004, 2016.
Ziehn, T., Chamberlain, M., Lenton, A., Law, R., Bodman, R., Dix, M., Wang, Y., Dobrohotoff, P., Srbinovsky, J., Stevens, L., Vohralik, P., Mackallah, C., Sullivan, A., O’Farrell, S., and Druken, K.: CSIRO ACCESS-ESM1.5 model output prepared for CMIP6 ScenarioMIP, Earth System Grid Federation [data set], https://doi.org/10.22033/ESGF/CMIP6.4320, 2019.
Ziehn, T., Chamberlain, M. A., Law, R. M., Lenton, A., Bodman, R. W., Dix, M., Stevens, L., Wang, Y.-P., and Srbinovsky, J.: The Australian Earth System Model: ACCESS-ESM1.5, Journal of Southern Hemisphere Earth Systems Science, 70, 193–214, https://doi.org/10.1071/ES19035, 2020.
Zimov, S. A., Schuur, E. A. G., and Chapin, F. S.: Permafrost and the Global Carbon Budget, Science, 312, 1612–1613, https://doi.org/10.1126/science.1128908, 2006.