ESD Ideas: Global climate response scenarios for IPCC assessments
IPCC Working Group I has long employed socioeconomic scenarios, based on discrete storylines, to sample the uncertainty in future forcing of the climate system, but analogous scenarios to sample the uncertainty in the global climate response have not been employed. Here, we argue that to enable development of robust climate policies this gap should be addressed, and we propose a simple methodology.
The Working Group I (WGI) contribution to the Sixth Assessment Report (AR6) of the Intergovernmental Panel on Climate Change (IPCC) is in preparation for publication in 2021. One of the requirements is to provide assessed projections of global climate. Such projections depend on future forcing of the climate system and on the response to this forcing (Hawkins and Sutton, 2009). Traditionally, uncertainty in future forcing has been explored in WGI using a discrete set of socioeconomic scenarios, whereas uncertainty in the climate response has been characterised by a likely range (66 % probability) for future climate under each socioeconomic scenario. The likely ranges have been derived from multi-model projections produced by the WCRP Coupled Model Intercomparison Project (CMIP)1. However, a focus on the likely range for future climate is ill-suited to the needs of policy makers faced by problems of risk assessment (Sutton, 2018, 2019). In risk assessment, there is special interest in high-impact scenarios, even if their likelihood is considered low (King et al., 2015).
To address the needs of risk assessment, Sutton (2019) proposed that IPCC WGI should employ a discrete set of scenarios to sample uncertainty in the global climate response2, analogous to the socioeconomic scenarios used to sample forcing uncertainty. This idea can also address the challenge for AR6 (and other IPCC assessments) to present global climate projections that are consistent with the assessment of key parameters such as ECS3. Here, we present a simple demonstration of how this could be done for projections of global mean surface air temperature (GSAT), exploiting the CMIP6 projections and estimates of ECS for each model.
For each of the chosen socioeconomic scenarios (Shared Socioeconomic Pathways; SSPs), we regress the simulated mean GSAT change onto ECS from each CMIP6 model in each overlapping 20-year period (with central years 2025–2090, examples in Fig. 1a and b). The slope of this regression defines the climate response scenario for each SSP and time period (Fig. 1c) and can be used to produce GSAT projections as a function of ECS (climate response scenario) and SSP (emissions scenario). Figure 1c shows projections and climate response scenarios for GSAT change under SSP1-2.6 and SSP5-8.5. In each case, the 5 %–95 % range spanned by the currently available CMIP6 models is shaded, and three response scenarios are shown corresponding to ECS values of 2, 4 and 5 ∘C. No quantitative likelihood is attached to each scenario and there is no “best estimate” – they are merely chosen to illustrate a range of possibilities relevant to risk assessment. For the purposes of discussion, we imagine that the AR6 assessment is that ECS is likely in the range of 2.5 to 4.0 ∘C and very likely in the range of 2.0 to 5.0 ∘C. Thus, the 4 ∘C ECS scenario corresponds to the upper end of our likely range. As impacts and risks have been assessed to increase rapidly with GSAT (e.g. the “burning embers” figure; Field et al., 2014), it could be used to estimate the highest impacts consistent with the assessed likely range. The 5 ∘C ECS scenario may be considered a “physically plausible high-impact scenario”, in line with the definition of Sutton (2018). It corresponds to a highly sensitive climate system leading to rapid warming and rapidly increasing risks and associated costs of adaptation and/or mitigation. Under the 2 ∘C ECS scenario, the direct impacts and costs of climate change would be less severe, or delayed. However, it might still be considered high impact from a policy point of view, as it could imply that the costs of adaptation and mitigation would be lower than previously anticipated.
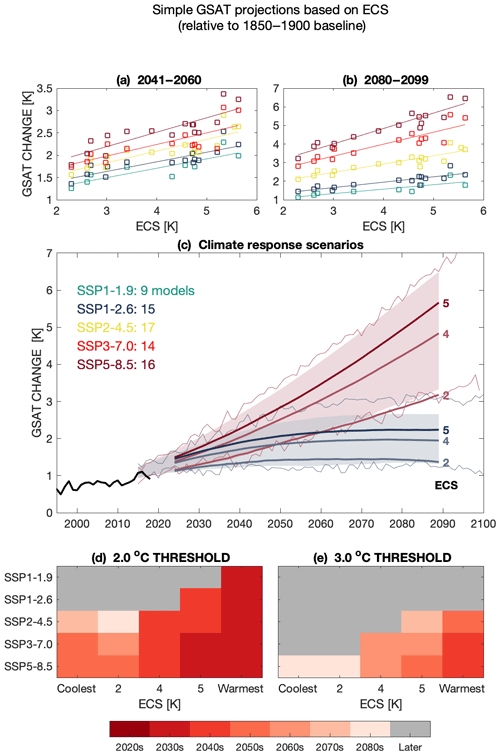
Figure 1Scenarios for global mean surface air temperature (GSAT) derived from CMIP6 projections. Panels (a, b) show regressions, for each SSP, of simulated mean GSAT for a range of models onto each model's own estimated ECS value, for two example 20-year periods. Where multiple ensemble members are available, we have used the ensemble mean response. The simulations are first referenced to the mean of 1995–2014 and baselined to an approximate pre-industrial level using an observed change from 1850–1900 to 1995–2014 of 0.76 ∘C using HadCRUT4 (Morice et al., 2012). Panel (c) shows three climate response scenarios (thick lines) assuming ECS values of 2, 4 and 5 ∘C, for two SSPs (SSP5-8.5, red, and SSP1-2.6, blue), along with the 5 %–95 % simulated range (shaded) and the simulations with the largest and smallest responses at the end of the century (thin lines). Panels (d, e) show the decade in which 2 and 3 ∘C GSAT thresholds are first crossed as a function of climate response scenario and emissions scenario. Grey shading indicates that the threshold is not crossed by 2090 (i.e. the 20-year average of 2081–2100). Note that (1) the SSPs considered are not equally spaced in terms of estimated radiative forcing; (2) GSAT declines in the latter part of the century for some SSPs and, in some cases, may fall back below one of the thresholds shown, but we do not include that possibility in panels (d, e); and (3) a different reference period choice would produce different ranges (Hawkins and Sutton, 2016), especially for the near term; we do not consider this sensitivity here and do not analyse a threshold crossing of 1.5 ∘C for this reason.
Figure 1 also illustrates projections for the most and least rapidly warming models under each SSP. In the absence of counter evidence, these projections might also be considered physically plausible, so these projections offer alternative – more extreme – choices for high-impact scenarios. Such scenarios are likely to be less robust because of their reliance on single model results.
To inform risk assessments, scenarios must be combined with quantification of impacts. There is no single metric of impact: many variables are relevant to policy and decision making. As a simple illustration, we consider here the time of crossing specific temperature thresholds. This variable is important for climate policy following the framing of the Paris Agreement in terms of ambitions to stay below specific levels of GSAT relative to pre-industrial climate. Figure 1d and e illustrate the year in which the 2 and 3 ∘C warming thresholds are crossed, as a function of socioeconomic scenario and climate response scenario. It is immediately apparent that whether and when the thresholds are crossed depend as much on the response scenario as on the forcing scenario. For example, under SSP1-2.6, the 2.0 ∘C threshold is only crossed under the highest ECS scenarios; under SSP3-7.0 and SSP5-8.5, the 5 ∘C ECS scenario yields crossing times 2–3 decades earlier than the 2 ∘C ECS scenario. A notable feature of Fig. 1c is that, before 2060, the 5 ∘C ECS scenario for SSP1-2.6 (low emissions) is warmer than the 2 ∘C ECS scenario for SSP5-8.5 (high emissions). These results illustrate very clearly that climate response scenarios are just as relevant to mitigation policy as are socioeconomic scenarios. The development of robust policies must consider both factors, including explicit attention to high-impact scenarios, such as the 2 and 5 ∘C ECS scenarios considered here. To fully explore the consequences of climate response scenarios obviously requires the expertise of all three IPCC Working Groups. Therefore, it would be extremely valuable if a common set of climate response scenarios could be investigated and assessed by all three groups. Such an approach would aid development of coherent IPCC Synthesis Reports.