the Creative Commons Attribution 4.0 License.
the Creative Commons Attribution 4.0 License.
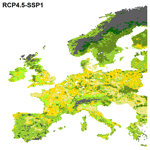
Societal breakdown as an emergent property of large-scale behavioural models of land use change
Bumsuk Seo
Mark Rounsevell
Human land use has placed enormous pressure on natural resources and ecosystems worldwide and may even prompt socio-ecological collapses under some circumstances. Efforts to avoid such collapses are hampered by a lack of knowledge about when they may occur and how they may be prevented. Computational models that illuminate potential future developments in the land system are invaluable tools in this context. While such models are widely used to project biophysical changes, they are currently less able to explore the social dynamics that will be key aspects of future global change. As a result, strategies for navigating a hazardous future may suffer from “blind spots” at which individual, social and political behaviours divert the land system away from predicted pathways.
We apply CRAFTY-EU, an agent-based model of the European land system, in order to investigate the effects of human behavioural aspects of land management at the continental scale. We explore a range of potential futures using climatic and socio-economic scenarios and present a coherent set of cross-sectoral projections without imposed equilibria or optimisation. These projections include various behavioural responses to scenarios including non-economic motivations, aversion to change and heterogeneity in decision-making. We find that social factors and behavioural responses have dramatic impacts on simulated dynamics and can contribute to a breakdown of the land system's essential functions in which shortfalls in food production of up to 56 % emerge. These impacts are largely distinct from, and at least as large as, those of projected climatic change. We conclude that the socio-economic aspects of future scenarios require far more detailed and varied treatment. In particular, deviation from simple economic rationality at individual and aggregate scales may profoundly alter the nature of land system development and the achievability of policy goals.
- Article
(3157 KB) - Full-text XML
-
Supplement
(6027 KB) - BibTeX
- EndNote
The human use of land resources has led to the transformation of much of the Earth's surface (Hooke and Martín-Duque, 2012; Pongratz et al., 2008; Ramankutty et al., 2008). This transformation has enabled rapid rises in human population sizes and some living standards but has also been a driving force of climate change and mass extinction (Newbold et al., 2016; Steffen et al., 2015). These consequences have become so severe that they threaten the continued provision of many of the essential “contributions to people” that terrestrial environments make (Díaz et al., 2018). Societies now face the enormous challenge of sustaining these contributions while simultaneously overcoming ingrained inequalities in their distribution (United Nations, 2017).
Computational models play a crucial role in understanding global change and identifying strategies to avoid its worst impacts. However, the systemic complexity that makes these models essential also makes them difficult to verify, inevitably incomplete and therefore of limited accuracy (Beven, 2007; Brown et al., 2016a; Smith, 2001). Indeed, recent research suggests that land system models tend to produce unrealistic and inconsistent projections of human behaviour in particular (Alexander et al., 2017; Brown et al., 2019a; Searchinger et al., 2017; Turner et al., 2018). This may make these models inapplicable in exactly the circumstances under which they are most required: when socio-ecological dynamics cause systemic change, regime shifts or breakdowns (Cumming and Peterson, 2017).
One necessary improvement in modelling practice is the adoption of a wider range of conceptual and technical approaches (Alexander et al., 2017; Huber et al., 2018; Meyfroidt et al., 2018). At present, a small number of simplifying assumptions have become standard in land system modelling, allowing models to operate over large geographical extents and thematic areas without becoming computationally intractable. Broad assumptions about human behaviour are particularly common, usually following a paradigmatic reductionist approach that emphasises the role of macroeconomic drivers of land use change (Brown et al., 2016a, 2017; Calvin and Bond-Lamberty, 2018). These assumptions tightly constrain the representation of human decision-making, often requiring it to adhere to exogenously imposed equilibria. Furthermore, a focus on the agricultural sector has meant that other sectors (e.g. forestry, urban development) have generally been treated as separate systems rather than interacting components of the land system as a whole (Brown et al., 2017; Smith et al., 2010; van Vliet et al., 2019).
These shortcomings particularly constrain the exploration of the effects of the social aspects of future scenarios, which, while often quite dramatic, are not reproducible through the predominantly biophysical parameters of most land use models (Müller-Hansen et al., 2017; Riahi et al., 2017). Alternative, well-supported conceptualisations of the human land use system are available, and some have been formalised in agent-based or behavioural models that focus on individual-level decisions from which system properties emerge (e.g. Arneth et al., 2014; Brown et al., 2016b, 2017; Fagiolo and Roventini, 2017; Magliocca, 2015; Rounsevell et al., 2012b). To date, these models have been limited in scope, mainly operating only in specific contexts or over small geographical areas (e.g. An, 2012; Brown et al., 2017; Robinson et al., 2018). However, their focus on underlying processes makes them suitable for scaling out and scaling up across entire coherent land systems (Rounsevell et al., 2012a). Recent conceptual and technical developments make this scaling feasible (Arneth et al., 2014; Verburg et al., 2015), and associated studies suggest that microscale behavioural processes can have significant macroscale effects (Bai et al., 2016; Blanco et al., 2017a; Brown et al., 2018b; Calvin and Bond-Lamberty, 2018).
If a new generation of behavioural models is to make a substantial contribution to Earth system modelling, it must satisfy a number of requirements. First and foremost, models must achieve accuracy in their representation of basic processes that transcend land sectors, geographical areas and scenario conditions. Given this, models can move beyond context-specific calibrations and retain sufficient flexibility to explore land system development under uncertain future global change. By the same token, these models need to incorporate relevant decision-making processes at a range of scales, from individual to community and government, thereby minimising the role of exogenous and potentially inconsistent assumptions about nested actions (Galaz et al., 2012; Lippe et al., 2019; Rounsevell et al., 2014). Beyond behaviour, models must also reflect the true range of land use options, including gradients from subsistence production to profit maximisation, highly extensive to highly intensive management, and entirely uni-functional (monocultural) to highly multifunctional or mosaic land systems (McDermid et al., 2017; Verburg et al., 2013).
In order to move towards these goals, we have developed CRAFTY-EU, a continental-scale, agent-based model of the European land system based on the CRAFTY modelling framework (Murray-Rust et al., 2014). We describe the design, calibration and evaluation of this model before using it to explore future developments in Europe's land system under a range of climatic and socio-economic scenarios. We assess the sensitivities of these developments to scenario conditions and various forms of land manager behaviours, as well as their implications for the supply of a range of ecosystem services and land system stability. We then discuss the possible impacts of human behaviour within the land system, as well as the value of novel modelling approaches of this kind for understanding and managing Earth system change.
CRAFTY-EU is an application of the CRAFTY framework for the agent-based modelling of land use change (Murray-Rust et al., 2014; Blanco et al., 2017a; Brown et al., 2018b; Holzhauer et al., 2019). The CRAFTY framework allows land use outcomes to be modelled as the result of decision-making and competition among individual agents, each of which can represent an individual or multiple land managers and which produce a range of ecosystem services. Production levels are determined by the productivity of the land (defined through a range of natural and anthropogenic capitals, as described below), the intensity of land management, and agent willingness or ability to produce certain ecosystem services. Agents are grouped into agent functional types (AFTs) (Arneth et al., 2014) on the basis of their management intensity and decision-making characteristics, such as the degree of focus on profit generation and desire to maintain an existing land use. Variation within AFTs allows for individual differences in production levels and land management decisions. Therefore, the model allows for emergent land system properties that are not constrained by assumptions about economic optimality, equilibrium or rationality, in the sense of satisfying generic economic conditions. The main components of the applied model are summarised in dedicated sections below.
CRAFTY-EU is calibrated using outputs from the IMPRESSIONS Integrated Assessment Platform (IAP), a cross-sectoral, multi-model tool for simulating European land system change (Harrison et al., 2015, 2019; Holman et al., 2017). All necessary input data (described below) are derived from this source, ensuring the transparency and internal consistency of the implementation. This model pairing also allows socio-economic and climatic scenarios to be defined on the basis of comprehensive, cross-sectoral simulations of the European land system that have been extensively evaluated, validated and utilised (Brown et al., 2014a; Harrison et al., 2012, 2016, 2019; Kebede et al., 2015; Pedde et al., 2019b). Changes in the modelled land system are therefore attributable either to CRAFTY model dynamics (investigated below) or scenario conditions, rather than internal inconsistencies in input data from different sources. Full details of the calibration of CRAFTY-EU are given in Appendix A.
2.1 European application
CRAFTY-EU covers the European Union 27 (EU member states that include the UK but exclude Croatia) together with Norway and Switzerland. The model operates at a 10′ (arcmin) resolution, with 23 871 grid cells in total. This resolution was selected for its consistency with input data, all of which had the same resolution, for its low computational demands, allowing multiple model runs to be carried out quickly, and because of a shortage of appropriate calibration data at finer resolutions. Nevertheless, this resolution is relatively coarse for an agent-based model application and means that modelled agents cannot be seen as representative of individual real-world land managers in most cases. Instead, they are drawn from semi-aggregated AFTs designed to represent coherent localised land use systems (Letourneau et al., 2012), with management and behavioural characteristics expressed at appropriate generality, as described below.
2.2 Agent functional types
The agent functional types used in CRAFTY-EU were designed to provide generic coverage of the major sectoral and cross-sectoral land systems at local (10′) scale across Europe. Key distinctions were made between levels of management intensity and between the ranges of ecosystem services produced (Arneth et al., 2014; Letourneau et al., 2012; Murray-Rust et al., 2014; Paul et al., 2017). The final typology was intended to capture the primary form of land management within each grid cell, while allowing for secondary land uses and variation in local land management practices (Table 1; Appendix A). The initial distribution of these AFTs across the modelled land surface was based on the distribution of land use categories modelled by the IAP under baseline conditions (Appendix A), ensuring consistency across initial simulation conditions and comparability with subsequent scenario-based changes.
Table 1Details of the agent functional types (AFTs) used in CRAFTY-EU. Ecosystem services are represented as follows: crops: ; meat:
;
timber:
; carbon sequestration:
;
diversity:
;
recreation:
. The primary ecosystem services of each AFT are those produced in quantities at least 50 % of the maximum of any other AFT and are shown in black. Secondary ecosystem services are those produced in lower quantities and are shown in green. The initial distribution of these AFTs across modelled grid cells as well as the full parameterisation of capital sensitivities and production levels are described in full in Appendix A. The conceptualisation and parameterisation of AFTs allow for some variation in capital sensitivities, service production abilities and land uses within each AFT. The urban (not shown) and peri-urban AFTs are included only as placeholders for urban modelling in the IAP and are constrained to reproduce the same results here, with peri-urban also allowing for the surrounding production of other ecosystem services as shown.
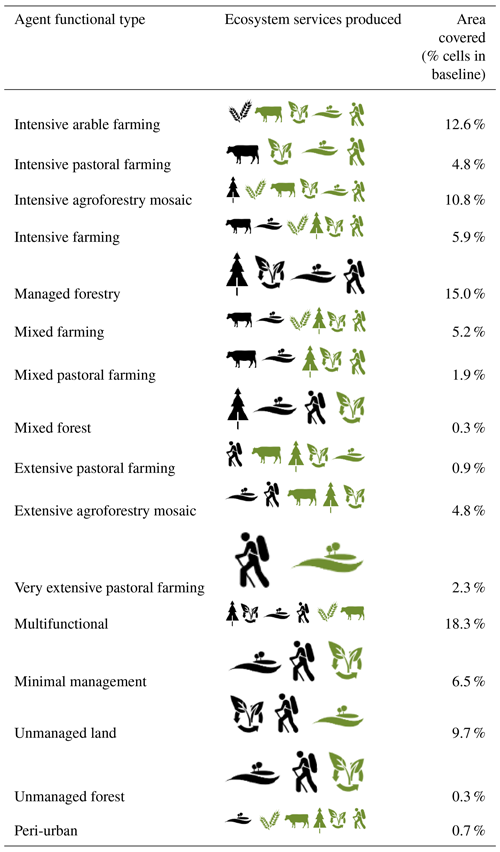
The abilities of different AFTs to utilise capitals and produce ecosystem services were defined via capital sensitivity and productive ability parameters (summarised in Table 1 with further details and exact parameterisations in Appendix A; see also Murray-Rust et al., 2014). Where possible, values were derived from simulated production data in the IAP and otherwise assumed on the basis of land management intensity and diversity. Behavioural differences between AFTs were also introduced to assess the robustness of model outcomes. These differences, described in Sect. 2.6, affected agents' ecosystem service production levels and their willingness to change land use or abandon land, and they were intended to approximate differences in productive abilities, priorities and sensitivities between and within agent types. Urban land use was not actively modelled but constrained to follow the results of the IAP, which includes advanced modelling of urban development (Terama et al., 2019).
2.3 Land productivities (capitals)
The productive potential of each modelled grid cell was described via five capitals: natural capital (crop productivity, grassland productivity, forest productivity), human capital, social capital, manufactured capital and financial capital. Each capital was derived from the IAP as described in Appendix A. Scenario-specific changes in capital values were produced by running the IAP under each scenario in turn and repeating the derivation process. Each of the productivity capitals accounts for climate-induced changes during the period of simulation, including the effects of changes in temperature, precipitation and CO2 levels. These changes were simulated for the IAP by combinations of global and regional climate models: EC_Earth/RCA4 for RCP2.6 and HADGEM2-ES/RCA4 for RCP4.5 and 8.5 (Harrison et al., 2019). Socio-economic conditions (as defined by the SSPs; Riahi et al., 2017) affected anthropogenic capitals (human, social, manufactured and financial) as determined via a stakeholder-led elaboration of scenario narratives and a subsequent uncertainty-based quantification (Harrison et al., 2019; Pedde et al., 2019b). Because IAP outputs were only available at three time slices (2020s, 2050s and 2080s), capital values were linearly interpolated to give annual values for each grid cell over the period 2010–2086.
2.4 Ecosystem services, demand and supply
The CRAFTY framework is designed to account for the demand and supply of a range of ecosystem services, and we incorporate a representative group for which calibration data are available or for which assumptions related to calibrated land management can be made: timber, meat, crops, carbon sequestration, landscape diversity and recreation. Annual demand levels for each of these services were derived from IAP outputs via the conversion of simulated land cover to service production levels as described in Appendix A. All demand levels are available in Supplement 1.
Demand levels were converted via mathematical functions to “benefit” values for each agent and cell throughout the simulations, taking account of current supply–demand gaps, assumed societal valuations of each service and agent abilities to provide each service on each cell (Appendix A). In this case, linear functions of supply–demand gaps were used to calculate benefit values, and these functions were calibrated to ensure the equal relative valuation of services (i.e. the production of an equal proportion of unmet demand was assigned an equal benefit value whatever the service). This created a balanced competition between agents that was not skewed towards any particular service(s), with no benefit accruing from production when there was no unmet demand, prompting production under shortfalls but not under surpluses.
The resulting benefit values were then used as a basis for competition between agents for the ownership of cells, which occurred on a defined proportion of cells at each time step (Sect. 2.6). Agents producing combinations of services with the highest benefit values were best placed to win this competition but did not necessarily do so because existing agents could choose to persist with their existing land use rather than submit to competition. This process was controlled by “abandonment” and “competition” thresholds describing the ranges of benefit values within which agents would abandon their land, persist with their existing land use or cede their land to another agent (representing an alternative land use) (Holzhauer et al., 2019; Murray-Rust et al., 2014). These thresholds were initially fixed across agent functional types and then varied at typological and individual levels as described below. Therefore, agents did not necessarily optimise their land uses according to benefit values, and these values were not used to ensure full supply of each service. With thresholds set to zero, individual agents would choose a more beneficial land use when made aware of one through the competition process (i.e. when their cell was selected for competition by another agent type) but would not necessarily do so under non-zero thresholds. In neither case was any system-level optimisation involved. Therefore, benefit values, responding to changes in demand and supply levels, stimulated production but did not guarantee a given production level. The model therefore contains no assumptions that override the emergence of suboptimal or non-equilibrium outcomes from scenario conditions. Service production in any part of the EU contributed to satisfying demand levels, representing an assumption of free trade across the modelled area (constrained by the infrastructure and transportation networks described in the manufactured capital values). This is a reasonable assumption given that the EU is a free trade zone. A full description of the valuation and competition process is given in Appendix A.
2.5 Model evaluation
The CRAFTY modelling framework has been extensively evaluated and applied in previous studies (e.g. Alexander et al., 2017; Blanco et al., 2017a; Brown et al., 2014b, 2018b; Holzhauer et al., 2019; Murray-Rust et al., 2014), as has the IAP upon which this application of CRAFTY is based (e.g. Brown et al., 2014a; Harrison et al., 2016; Holman et al., 2017; Kebede et al., 2015). Both sets of evaluations have included sensitivity and uncertainty analyses (Brown et al., 2014a, b, 2018b; Kebede et al., 2015; Synes et al., 2019), comparisons to empirical data and to the results of other models (Alexander et al., 2017; Blanco et al., 2017a), full descriptions of model design and functioning (Harrison et al., 2015; Murray-Rust et al., 2014), and full free access to the models themselves including interactive online systems for exploring model outputs (Holzhauer et al., 2016; IMPRESSIONS Project, 2018; https://landchange.earth/CRAFTY, last access: 3 December 2019). Both models have also been extensively used in, and informed by, a stakeholder engagement process that has occurred over several years across the EU (Kok et al., 2019).
Here, additional model evaluation focused on the behaviour, stability and interpretability of the European application of CRAFTY. These characteristics were primarily assessed through two sets of runs under static baseline conditions, starting from an unassigned (empty) land use map and from the baseline land use map derived from the IAP. The purpose of these two exercises was, respectively, (1) to check whether baseline conditions would generate a “realistic” land use configuration purely on the basis of capital levels and AFT characteristics (i.e. in the absence of any spatial information about land management) and (2) to check for divergence in outcomes from a common starting point consistent with the starting point of other scenario runs. Model dynamics were checked visually and statistically using the numbers of agents within each AFT and levels of service provision. Both evaluation exercises are described in detail in Appendix B.
2.6 Simulation schedule
CRAFTY-EU runs on annual time steps at which a proportion of cells are subject to potential abandonment, adoption or competition (Murray-Rust et al., 2014). In the first evaluation exercise, the model was run over 800 time steps, with 20 % of cells being randomly selected for potential change (i.e. the maximum number of cells that could change at each time step if required by the competition process). This arbitrary but high rate of competition allowed for rapid changes to the simulated land system, ensuring model dynamics could be clearly perceived. The period required for the model to reach a steady state was identified, and 10 further independent simulations were then run to this point using different random number generator seed values. The second evaluation exercise was performed over 100 time steps, again with a 20 % rate of cell selection. This exercise was designed to run a sufficient number of replicates to identify and understand any divergence from stationarity in model dynamics.
Following the evaluation exercises, simulations were run for 71 time steps, representing the period 2016–2086, with 5 % of cells selected for potential change at each of these time steps. As an upper limit, this rate is up to an order of magnitude greater than observed (e.g. Loveland et al., 2012) and projected land use changes (e.g. Schmitz et al., 2014), allowing for the majority of potential changes to be rejected while maintaining scope for rapid land use change under extreme scenarios. These simulations all began from the baseline land use map (Fig. A1.2) and proceeded according to scenario conditions in terms of ecosystem service demand levels and capital values (Supplement 1). Seven distinct scenarios were simulated, each of which comprised a combination of Representative Concentration Pathways (RCPs) and Shared Socioeconomic Pathways (SSPs) (O'Neill et al., 2017) as described in Table 2. Socio-economic scenarios were developed from the SSPs through a stakeholder engagement process described in detail in Kok et al. (2019). These scenarios were first run through the IAP as described above in order to produce representative levels of capitals and demands for use in CRAFTY-EU. Throughout each simulation, land use maps, numbers of agents in each AFT, ecosystem service production levels and fragmentation indices (fractal dimensions) were recorded. Fractal dimension (Mandelbrot, 1983) was used to capture the patch sizes of each land use, with higher values indicating more complex patterns, and was compared on the basis of relative change from the baseline year using the R package SDMTools (Vanderwal et al., 2014).
Table 2Identities and characteristics of Representative Concentration Pathway–Shared Socioeconomic Pathway combinations used in the CRAFTY-EU simulations presented here. Graphical results are shown in Supplement 2, and full descriptions of the scenarios used can be found in Kok et al. (2019).
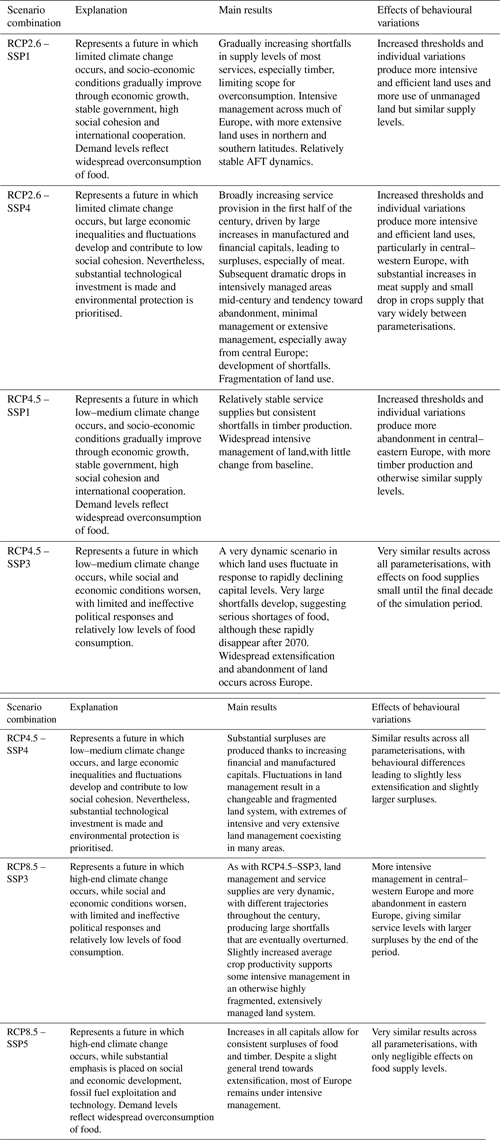
For each scenario, five distinct parameter sets were applied to assess the effects of variations in modelled agent behaviours (full parameterisations for each of these are given in Appendix A and a description of each behavioural parameter in Table 3). These parameter sets differed in terms of the abilities of agents to produce services and their tolerance of low benefit values and of competition, all of which varied at AFT and individual agent levels. These variations were designed to represent general behavioural effects arising from land manager decision-making, accounting for aggregation to the model's spatial resolution, but with a focus on sensitivity analysis across a large range rather than calibration at levels thought to be realistic. Parameter set 1 represented a behavioural “baseline” in which agents responded directly to benefit values with no additional individual or typological behaviour (i.e. the land use with the greatest benefit value was adopted in each case). Parameter set 2 (“increased thresholds”) introduced differences in abandonment and competition thresholds to induce the abandonment of land when benefit values fell below the abandonment threshold and persistence of an existing land use unless an alternative had an additional benefit value of at least the competition threshold. Intensive land use agents were parameterised to be less tolerant of low benefit values and more willing to switch to a land use with higher benefit values. Parameter set 3 (“individual variation”) introduced differences between individual agents in terms of their levels of production of different ecosystem services as well as their abandonment and competition thresholds. In this case, individual values were randomly assigned from a Gaussian distribution centred on the mean value for each type (Appendix A). Parameter sets 4 and 5 replicated sets 2 and 3, respectively, but with higher values for thresholds and variations (up to 10 times higher, with thresholds of the same order of magnitude as mean benefit values).
3.1 Model evaluation
3.1.1 Simulations with no initial land use map
Simulations initiated under all baseline conditions, but without the initial land use map, were found to quickly converge to an approximate steady state (Fig. B1) but not to achieve formal stationarity over 800 time steps (Box–Ljung test p values < 0.01 for numbers of agents belonging to each AFT and service production levels over 50-time-step periods). This appeared to be due to path-dependent oscillations (over short and long time spans) that, while statistically significant, were small relative to total agent numbers and rarely affected the relative rank of each AFT (Fig. B1). These oscillations were amplified by the high rate of competition for cells allowed in the evaluation simulations (20 % of cells at each time step), and as such they remained broadly in line with expectations, with no evidence of either ongoing systematic change or dramatic regime shifts.
The 300th time step was chosen as representative of model outcomes following the initial period of rapid change, and the numbers of agents belonging to each AFT at this point in each of the 10 independent simulations were then plotted (Fig. B2a) along with the proportional supply levels of each service (Fig. B2b). These results showed strong convergence between simulation outcomes, with both relative and (approximate) absolute numbers of agents being reproduced in each simulation. Service levels remained between 95 % and 110 % of demand levels in all cases. In these relatively unconstrained circumstances, the model tended to produce a slight excess of meat and carbon sequestration services, with a predominance of multifunctional AFTs and a relative lack of intensive-management AFTs. However, aggregated AFTs showed not only spatial consistency across the simulations, but also agreement with the (unutilised) baseline map (Fig. B3), suggesting that the model produced realistic land use configurations on the basis of land productivities, AFT parameterisations and demand levels. These major drivers of land use therefore appear to have similar, decisive effects in the model as in the real world, without preventing the model from producing reasonable counterfactual land use configurations in the absence of an initial map.
3.1.2 Simulations from baseline map
The simulation initialised with the baseline land use map and run under static conditions remained stationary (Box–Ljung test p values > 0.1 for numbers of agents belonging to each AFT and service production levels) (Fig. B4). The total number of agents within each AFT barely changed, with the maximum range in the number of agents over the course of the simulation being 2. Further realisations were not generated given this lack of variation and the model stability that it demonstrated under static conditions.
3.2 Scenario simulations
Scenario simulations showed considerable divergence between land systems in the mid-2080s under different scenario combinations, and this was not substantially altered by behavioural variations between agents (Table 2, Supplement 2). These differences were primarily driven by socio-economic scenario conditions, but also by different levels of climate change between the three climate scenarios used (Figs. 1 and 2). Broadly, where socio-economic capital levels were maintained or increased, the land system diverged from the baseline scenario by a relatively limited amount, with widespread intensive management of land and small shortfalls or surpluses of most modelled services. Conversely, where these capitals declined substantially, widespread extensification and abandonment of land occurred, and large shortfalls in service levels developed (Fig. 1, Table 2, Supplement 2). These dynamics were partly ameliorated by increases in productivity in some areas associated with high-end climate change, especially north-western Europe.
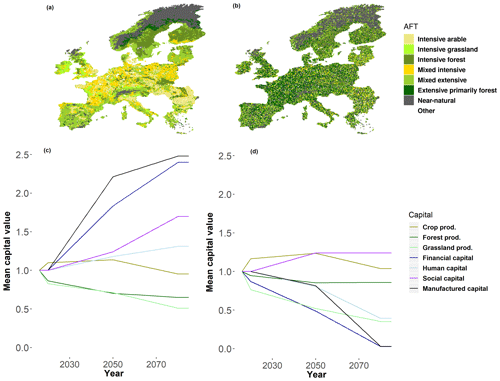
Figure 1Maps of simulated land cover in 2086 under the RCP4.5–SSP1 scenario combination (a) and the RCP4.5–SSP3 scenario combination (b), showing the two extremes of modelled outcomes across the simulated scenarios. These extremes are driven by the radically different socio-economic capital levels within the two scenarios (c, d; capitals shown as mean values normalised by their initial mean value). Changes in capital trends occur due to their interpolation between discrete IAP data points.
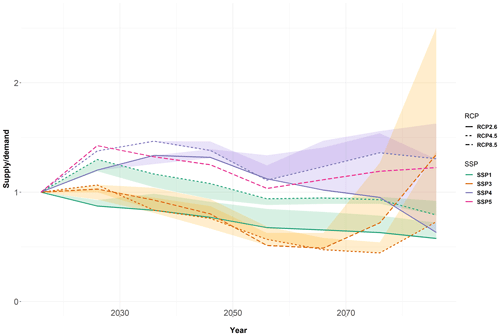
Figure 2Food (meat and crops) supply as a proportion of demand in all simulated scenario combinations. Values less than 1 indicate a shortfall. Shaded areas show the ranges of results produced by simulated behavioural variations within each scenario, with the range in RCP8.5–SSP5 being too small to be visible. Absolute per capita demand levels (including non-food usage and waste of food crops) are approximately twice as large by 2080 in SSPs 1 and 5 as in SSPs 3 and 4.
Of particular importance were manufactured and financial capitals, which increase greatly (up to 250 %) in some scenarios (e.g. SSP1) and decrease (by around 90 %) in others (e.g. SSP3) depending on scenario storylines (Fig. 1 and Table 2). These capitals are crucial in supporting intensive land management in CRAFTY-EU (Appendix A) and so determine the scope for the most productive uses of land. Where these capitals increased, surpluses of services (especially food) developed, and where they decreased, shortfalls developed, reaching 56 % of food demand in the RCP4.5–SSP3 scenario combination (Fig. 2). These capital dynamics are also linked to reduced per capita food consumption in SSP3 (Table 2), suggesting that shortfalls in this context translate more directly into hunger than they do in other scenarios in which overconsumption provides a buffer to any health impacts of underproduction.
We simulated three socio-economic scenarios in different climate scenarios and all showed notable similarities between climates. SSP1 had the clearest differences; this scenario has high demands for all services, and the difference between climate scenarios was due to increases in average crop and forest productivity capitals under RCP4.5 relative to RCP2.6. These productivity changes increased the competitiveness of intensive management enough to allow it to outcompete more extensive, multifunctional land uses and so allowed production to meet 15 %–30 % more of the demand than under RCP2.6.
The most consistent and most negative scenario was SSP3, in which economic and social challenges led to disintegration of the land system across much of Europe, with large areas being abandoned, managed extensively or fluctuating over time (Fig. 1, Table 2, Supplement 2). These dynamics were particularly pronounced in more fertile areas of Europe, where currently dominant intensive management declined dramatically during the first half of the century. As a result, similar shortfalls of almost 50 % of food demand were found between 2050 and 2080 in both RCP4.5 and RCP8.5, suggesting that changes in climate were minor in comparison to the almost complete loss of financial and manufactured capitals that undermines the productive use of land in SSP3. Nevertheless, supply levels increased markedly towards the end of the century in RCP8.5, as increased natural capitals (i.e. yield increases) offset some of the losses from declining socio-economic capitals. Conversely, in technologically advanced scenarios (e.g. SSP4), in which manufactured and financial capitals increase greatly, demands for services could be met relatively easily during most of the century, leading to a decline in intensive management because of a lack of need rather than a lack of opportunity.
Results also show some broad geographical patterns. While the most unproductive areas of Europe (e.g. mountain ranges, high latitudes) were the most resistant to change under any scenario, other areas responded differently depending on the scenario conditions. South-eastern Europe (Greece, Bulgaria, Romania and Hungary) was slightly more vulnerable to extensification and abandonment, whereby supply levels matched demands as in SSP4 (i.e. when the benefit or profit levels were low), but were more robust to low levels of capitals in SSP3. In contrast, western Europe (particularly Germany, France, England and intensively managed areas of Spain) suffered widespread abandonment in SSP3. As climate change increased in magnitude through RCP2.6, 4.5 and 8.5, land management in north-eastern Europe (Poland, Lithuania, Latvia, Estonia and southern Finland) tended towards forestry, as increases in forest productivity and decreases in crop productivity made arable agriculture less competitive.
Behavioural parameter variations had distinct effects in different scenarios and on different metrics (Table 2, Fig. 2 and Appendix C). In general, land use patterns in scenarios with less intensive management (and also lower land use fragmentation as measured by the fractal dimension) were less affected by behavioural parameter changes; these scenarios included RCP4.5–SSP3, RCP8.5–SSP3 and RCP4.5–SSP4. Conversely, scenarios with more intensive management (RCP4.5–SSP1, RCP2.6–SSP1 and RCP2.6–SSP4) were more affected, producing more fragmented land systems. These differences were not necessarily reflected in ecosystem service supply levels, however (Table 2, Fig. 2). Instead, these showed increasing effects of parameter variations over the simulation period, with the largest effects occurring in SSP3 and 4 and the smallest in SSP1. In two scenarios (RCP2.6–SSP4 and RCP4.5–SSP3), behavioural parameterisations determined whether food was oversupplied or undersupplied by the 2080s, with increasing variation between agents leading to increased food supply. These differences were also partially correlated with climatic scenarios, with land systems that were more productive under high-end scenarios proving more robust to behavioural differences (particularly RCP4.5–SSP4, RCP4.5–SSP1 and RCP8.5–SSP5, in which variations had almost no impact on food supply). Of the two forms of variation simulated, increased requirements for benefit from land management (thresholds) led to increased fragmentation within scenarios on average but also increased differences in fragmentation between them. Individual variation increased the differences in fragmentation between scenarios more than it did average levels across them, but at the higher strength these differences were reduced to approximately baseline levels, suggesting a “peak effect” under small levels of individual variation beyond which the effects of behavioural parameters were reduced (Fig. C1). Plots of the evolution in behavioural parameter values during the simulations (Fig. C2) show that this effect was partially due to a context-specific “selection pressure” towards particular parameter values, especially in the SSP3 simulations. In this context, the initially random distribution of agent parameters became skewed towards higher values of competition thresholds, lower values of abandonment thresholds and lower variation between agents, demonstrating a disproportionate persistence of agents who were relatively unlikely to respond to benefit values. We did not observe the converse persistence of more “optimising” agents in any scenario.
In all cases, the delicate balance between food and timber production highlights the sensitivity of results to demand levels for ecosystem services derived from agriculture and from forestry. In many cases, simulations resulted in the widespread adoption of multifunctional land uses that provide both sets of services to some extent, with the locations of these being scenario dependent. The levels of demand, relative valuation and production of these services therefore appear to be major determinants of the nature of European land systems in this model.
The work presented here highlights the importance of both model design and scenario conditions for understanding possible future change in large-scale land systems. This complements previous findings that model design and initial data conditions had a greater impact than scenarios on simulated land use change (Alexander et al., 2017) but extends the comparison to new design and scenario components. Until now, the exploration of these has been generally limited to optimising pattern-based models and the biophysical and economic factors that they incorporate, neglecting the social conditions and processes that often vary dramatically between scenarios (Brown et al., 2017; von Lampe et al., 2014; Pedde et al., 2019a).
This model implementation demonstrates that the agent-based modelling of socio-ecological systems at continental scales is both a feasible and informative method for scenario exploration, producing clear and distinct outcomes that respond directly to scenario definitions. These responses include the breakdown of the simulated land system, in which rapid and suboptimal land use changes lead to severe shortages of ecosystem services including food. While such breakdown is occasionally a feature of real-world land systems and a plausible result of severe pressures in the future (Ehrlich and Ehrlich, 2013; Hazell and Wood, 2008; Weiss and Bradley, 2001), it is largely precluded by equilibrium or optimisation assumptions in conventional modelling approaches (Balint et al., 2017; Brown et al., 2016a; Farmer and Geanakoplos, 2009). The ability to explore such breakdowns, whether in agent-based or flexible economic models, is clearly necessary for attempts to achieve the converse: stability and sustainability in socio-ecological systems.
To allow proper interpretation, the remainder of the Discussion section is divided between technical considerations relating to model design and parameterisation and reflection on the results produced in this study.
4.1 Model design
CRAFTY-EU is an explorative model and is not designed to predict (inherently unpredictable) land system changes (Brown et al., 2016a). Further, the CRAFTY framework is intended to provide relatively simple, generic methods for exploring land manager decision-making over large geographical extents (Murray-Rust et al., 2014) and is used here to represent decision-making within local land systems rather than at the level of individual managers. As such, this model application is a first step towards an improved understanding of behavioural processes within large-scale land systems.
At a general level, the results presented here are realisations of a single approach to land systems modelling, which complement alternative projections made by other models (e.g. Harrison et al., 2019; Stürck et al., 2018; Verkerk et al., 2018). In particular, conceptual or theoretical frameworks within which behavioural modelling can occur are diverse and disputed, and a universally applicable representation of the complex social processes involved in land use change is not available or even necessarily possible (Brown et al., 2016a; Huber et al., 2018; Meyfroidt et al., 2018). Even given this caveat, our exploration of behavioural parameters is illustrative rather than exhaustive, intended to reveal the implications of basic assumptions more than exact parameter values. We do not attempt to derive realistic parameter values but instead assess model sensitivity across a large range, from identical agents attempting to maximise their benefit, to highly diverse agents tolerating benefit differences of around 100 % of mean values (Table A4). In this respect, we deliberately build on earlier studies of behavioural processes in CRAFTY, including in a similar scenario context (e.g. Brown et al., 2014b, 2018b). While the CRAFTY framework is specifically designed to allow for the exploration of abstracted behaviours that do not require precise parameterisation, linking these values to empirical data is clearly desirable even where apparent effects on model outcomes are small.
In this case, more precise parameterisation might necessitate finer spatial and behavioural resolution, as is typical of many agent-based models. Nevertheless, conceptual and practical limits to behavioural parameterisation exist, and the aggregation of agents by location, land use and behaviour (or combinations such as functional type) is an established approach to large-scale modelling (Arneth et al., 2014; Valbuena et al., 2010). Identifying an appropriate balance between scale and resolution in the agent-based modelling of the European land system certainly requires further investigation of behavioural processes and data across scales, but the fact that we find clear systemic impacts of behaviours simulated at 10 arcmin resolution implies that finer resolutions may well be informative, as they have proved in previous applications of the CRAFTY framework (e.g. Blanco et al., 2017). At present, data availability is the greatest barrier to the adoption of these resolutions in European modelling.
A number of more specific considerations are also important for interpreting our findings. Most significantly, the simulations presented here form an experiment into the effects of simulating land management as the provision of multiple (but arbitrarily limited) ecosystem services, which depend upon a set of scenario-dependent capitals and which are valued equally per standardised unit of demand. This design ensures that trade-offs between services are clear but does not assume the preferential production of some services (such as food) when supply levels are equally insufficient. As a result, scenarios in which shortfalls in service provision exist might represent an artificially balanced outcome, with real-world equivalents potentially diverging towards more homogeneous land uses. In this respect, our findings suggest that the further exploration of trade-offs between service provisions, in terms of both production systems and valuation, should be a priority for land system modelling. This is especially important given potential changes in current valuation practices, for example through carbon pricing or payments for ecosystem services, which could transform the competitiveness of currently minor land uses and require models to account for the services that they produce (Kay et al., 2019).
Beyond Europe, neither CRAFTY-EU nor the IAP that is used to calibrate it explicitly represent production and trade. While scenario-specific import levels are assumed, these are likely to be overestimates in challenging scenarios with large shortfalls in service provision that imply shortages elsewhere in the world (Dellink et al., 2017; Harrison et al., 2015; Stevanović et al., 2016). This would reduce supply levels even more than simulated here. Furthermore, alternative treatments of international trade based on assumptions of economic equilibrium would be inconsistent with the supra-economic behavioural approach used in CRAFTY-EU (Arthur, 2006). The relative provision of different services is also subject to substantial uncertainty in our representation of forest growth, with assumed adaptation to changes in species suitability likely to overestimate real-world adaptation (Schelhaas et al., 2015), as the CRAFTY framework has previously been used to demonstrate (Blanco et al., 2017a, b).
Notwithstanding the above limits on the model's accuracy, the robust, cross-sectoral nature of the model, building on the established and evaluated IAP and CRAFTY framework, means that it is capable of providing well-founded and novel insight into land system dynamics. Model evaluation performed for this and earlier studies has revealed no clear biases or instabilities, with CRAFTY-EU producing realistic outcomes in the absence of information about baseline land uses. The responses of the model to the scenarios can be seen as coherent responses to a set of land system drivers that are fully interpretable in light of transparent model assumptions.
4.2 Model results
A key finding of this work is that the sensitivity of land use to social (as well as economic and climatic) conditions makes land systems vulnerable to breakdown when these conditions worsen substantially. Such worsening is a key characteristic of some future scenarios (e.g. SSP3) but one that has generally only been explored through qualitative scenario descriptions (e.g. Cradock-Henry et al., 2018; Kebede et al., 2018; Pedde et al., 2019a). In SSP3, declines in socio-economic capitals are so precipitate and substantial that the resulting breakdown of the simulated land system is highly plausible and proves almost impossible to avoid in our modelling, regardless of specific parameterisations. In other model projections of this scenario, similar outcomes are avoided only by very large increases in food prices that compensate for relatively low crop yields and stimulate food production at the expense of forest cover (Doelman et al., 2018; Fujimori et al., 2017; Harrison et al., 2019; Hasegawa et al., 2015; Popp et al., 2017). These large increases are generally enabled by an assumption of economic equilibrium and not constrained by financial or other productive limitations related to the scenario.
This implies that scenario modelling using economic equilibrium assumptions could prove misleading, and scenario conditions place potentially non-equilibrium limits on price or production levels. Substantial declines in financial and manufactured capitals, for instance, may effectively preclude the necessary economic stimuli or production responses to meet demand in SSP3. While this problem is starkly illustrated by non-equilibrium modelling such as that presented here, it is not in principle impossible to account for it in models that assume equilibrium dynamics within scenario-specific constraints (Heistermann et al., 2006). However, knock-on effects on consumption, demand and supply (and wider socio-economic systems) are obscured by the predefinition of those factors in scenario storylines. The CRAFTY-EU model therefore makes only one of two crucial connections, linking social conditions and supply levels through capital–production relationships without completing the link back to demand. Most starkly, if insufficient food is produced to maintain population levels, populations and subsequent demand would inevitably decrease – a fundamental feedback that remains absent from scenario modelling.
Such an effect is particularly pertinent to our simulations of SSP3. In the storylines of SSP3 and 4, large populations suffer low and unequally distributed economic growth, leaving per capita demand for agricultural production approximately half that in the wealthier and more equitable contexts of SSP1 and 5 (Table 2; Kok et al., 2019; Riahi et al., 2017). The large and long-lasting shortfalls in food production generated by SSP3 simulations therefore imply far greater challenges than the shortfalls generated by SSP1 simulations because the latter allows adequate (if undesirable) levels of nutrition to be maintained, while the former does not. This is clearly an important area for further research, albeit one that requires much improved treatment of food security and its consequences as well as fully internally consistent scenario simulations that account for a range of economic and social factors.
In addition to identifying very large negative impacts of some scenario combinations, we also find that these impacts differ widely across Europe. Some areas appear to face high likelihoods of substantial changes; for example, we find that eastern Europe is broadly more vulnerable to changes in demand levels (and hence benefit or price levels) and western Europe broadly more vulnerable to changes in capital levels. Many of the worst simulated outcomes have notable mirror images in history, whereby land systems gradually became more intensive, homogeneous and efficient as financial, technological and social capitals developed (e.g. Petit and Lambin, 2002). Projected declines of these capitals produce a return to fragmented, extensive production in our simulations; this is a reverse precedent that adds some credibility to model responses, while clearly not suggesting predictive accuracy. It is also notable that greater climatic change can actually ameliorate the worst outcomes in some cases (e.g. SSP3) because it allows higher yields in parts of Europe to offset losses and socio-economic difficulties elsewhere – at least in the absence of the pests, diseases and extreme weather events that may be associated with such climatic change (Donatelli et al., 2017). Similarly, technologically advanced scenarios (e.g. SSP4) allow for relative ease of production and therefore free up land, leading to some extensification and abandonment.
Within these broad findings, variations in behaviour can have substantial effects. These are generally more pronounced in low-end climate change scenarios that rely on slight competitive advantages of intensive land systems to meet service demand levels and which are therefore sensitive even to slight deviations from simple economic rationality in management decisions. The literature suggests that intensive farmers are more vulnerable to changing price levels (van Vliet et al., 2015), and this vulnerability is amplified here, as it may be in reality, by a reliance on socially mediated capitals that support farming (Sutherland and Burton, 2011). It is also notable that the behavioural effects we observe are similar at both simulated strengths of behaviour, suggesting that even small differences in land manager responses to scenario conditions can have substantial consequences. Indeed, increased behavioural differences do not necessarily persist in the face of challenging scenario conditions, under which some behavioural responses may consistently outperform others. In our SSP3 simulations, such an effect is apparent in the emergence of greater homogeneity and tenacity in the agent population and the loss of more responsive, economically rational agents (Fig. C2). Widespread evidence already exists of deviation from economically optimal behaviour amongst land managers in the real world, as in the selection of economically inferior options for social reasons or socially mediated uptake that spans long time periods (Brown et al., 2018a, 2019a; Sereke et al., 2016). Our findings further show that the effects of such behaviours on supply levels can accumulate over time (Fig. 2) when permitted to through a path-dependent and non-optimising modelling approach. The inclusion of more realistic and heterogeneous behaviours in land system models therefore appears to be a prerequisite for accurate assessments of future scenarios and so for effective land management planning and policy making.
The application of an agent-based model to simulate future European land use change suggests an important role for large-scale behavioural models of this kind. CRAFTY-EU is developed here to investigate broad forms of human behaviour in the context of land management decision-making and demonstrates that such behaviours can have multiple substantial effects in different scenario contexts. Furthermore, the most notable of these effects were linked to basic model assumptions rather than exact design or parameterisation choices. The inclusion of socio-economic aspects of future scenarios as active drivers of land use decision-making had impacts at least as large as simulated climate change, with behavioural effects further shaping trajectories within those scenarios. Competition between a cross-sectoral, multifunctional range of land uses highlighted the critical importance of the relative valuation of ecosystem services and the ability of models to represent a relevant range of services. Most prominent, however, was the effect of allowing land use decisions to occur without enforced equilibria or optimisation. In scenarios with challenging socio-economic conditions, this led almost invariably to the breakdown of the simulated land system and severe shortages of food and other services. These effects were apparent even at low levels of behavioural complexity and persisted across tested parameterisations. Indeed, we find some evidence that behavioural effects may be partially “self-correcting”, with some behaviours being selected out by a competitive process. These findings show a clear need and scope to consider the role of human behaviour in shaping land system development, whether through the development of new large-scale behavioural models or the introduction of additional behavioural and scenario-related constraints in economic models. Although this task remains challenging, the data and tools to explore the social dimensions of scenario space are developing rapidly and appear capable of providing important new insights into the future development of large-scale land systems.
The full model code and date are available for download and visualisation at https://landchange.earth/CRAFTY (Brown et al., 2019b).
This Appendix describes the parameterisation of CRAFTY-EU, including the derivation of agent functional types (AFTs). As outlined in the main text, AFT identities were designed to capture important sectoral and cross-sectoral land systems at local (10′) scale. The initial distribution of these AFTs across the modelled land surface was then determined on the basis of land use categories modelled by the IMPRESSIONS Integrated Assessment Platform (IAP) under baseline conditions (Harrison et al., 2015) (Fig. A1). This distribution ensured a common starting point for the two models that was fully consistent with the capital levels, demand levels and scenario conditions applied here so that subsequent simulated changes could be attributed to changes in those conditions rather than inconsistencies in calibration data. The mapping of IAP output land use categories to AFTs is described in Table A1.
The abilities of these AFTs to utilise capitals (Table A2) and produce ecosystem services were defined via capital sensitivity and productive ability parameters (given for each AFT in Table A3). Where possible, values were derived from the IAP and so preserved common forms of secondary land management and ecosystem service production within each AFT. Values that had no equivalent in the IAP (e.g. recreation service provision levels) were assumed on the basis of land management intensity and diversity, with variations used to understand the significance of these assumptions. This was also the case with the modelled biodiversity ecosystem service, which was represented here through the proxy of land use diversity (labelled “diversity” below) within each AFT.
In CRAFTY-EU, the modelled production of ecosystem services occurs subject to capital levels according to the equation
where represents the level of production of ecosystem service s in cell i by AFT t, calculated as the product across all capitals c of cell-specific capital levels ci weighted by the sensitivity λc,t (black rows in the tables below) of the AFT t to the capital c, multiplied by the maximum level of production os,t (red rows in tables below) that the AFT is able to produce. Maximum production levels os,t and capital sensitivities λc,t are constant throughout simulations, while capital levels ci vary according to scenario and, potentially, previous production levels and institutional intervention. Maximum production levels can, however, vary across individual agents within AFTs and do so here, in some experiments, randomly according to Gaussian distributions around the mean value (Tables A3 and A4).
The ability of an AFT to produce a service was first established by checking the average production level of each service across cells assigned to that AFT under baseline conditions. If this average value was greater than or equal to 1 % of the largest value produced by any AFT, that service was added to the AFT's productive abilities. The exact AFT-specific maximum production value (os,t) was calculated by extracting the 100 most productive cells for AFT t of service s and fitting a Gaussian distribution to the production levels in those cells using the R package fitdistrplus (Delignette-Muller and Dutang, 2015). The mean of this fitted distribution was taken as the value of os,t, while the standard deviation was retained for the introduction of random variation in production levels. This procedure was used under the assumption that the 100 most productive cells represented optimal production conditions and therefore provided a suitable basis to estimate production levels in the effective absence of capital constraints.
Capital levels were derived from outputs of the IAP to provide baseline and scenario-specific values (capitals are defined in Table A2). IAP results were interpolated to provide annual values for each capital on each grid cell within each scenario for the period 2010–2100. Where the derivation of capital values involves simulated quantities of production, these were normalised by the terrestrial area available in each cell (also an output of the IAP).
AFT-specific capital sensitivities λc,t were then estimated by plotting all production levels of service s by AFT t against each capital in turn (e.g. Fig. A1), with relationships quantified between the extremes of linear relationships (which were assigned a sensitivity value of 1.0) and random relationships (which were assigned a sensitivity value of 0.0). This procedure did not, and was not intended to, replicate the land use allocation methods applied in the IAP, but to generate similar sensitivities on the basis of which agent decision-making could proceed.
Once these relationships were established, IAP output maps were used to quantify demand levels for each of the modelled ecosystem services by calculating service production levels according to the optimal production and capital sensitivity values described above. This was repeated at each time step (2020s, 2050s and 2080s in the IAP, which were linearly interpolated to annual values between 2016 and 2086 for CRAFTY-EU). Where the IAP projected a shortfall in service production, the supply was calculated and then scaled up to the equivalent of 100 % to give a figure for demand. For the services not directly simulated by the IAP (recreation and diversity), the supply levels calculated from IAP output maps were taken as being equal to demand.
These demand levels (given in full in Appendix C) were then used to calculate context-specific benefit values of production as a basis for competition between agents. Benefit functions were defined to give the value of a certain level of production under a certain level of unmet demand according to the equation
where ms is the marginal benefit for service s, us is a function that describes the benefit (utility) of production of service s and rs is the residual demand for service s (Murray-Rust et al., 2014). Linear forms of us were used here, calibrated to ensure equal relative valuation of services; i.e. the production of an equal proportion of unmet demand was assigned an equal benefit value whatever the service. This created a balanced competition between agents that was not skewed towards any particular service(s), with no benefit accruing from production when there was no unmet demand, prompting production under shortfalls but not under surpluses.
Table A1The composition of agent functional types (AFTs) in CRAFTY-EU in terms of baseline IAP land use categories. In any case in which the given IAP categories occupy more than 70 % of a cell, that cell is allocated to the corresponding AFT in the baseline map of CRAFTY-EU, except in the case of the peri-urban AFT, for which the threshold (of urban area) is 40 %.
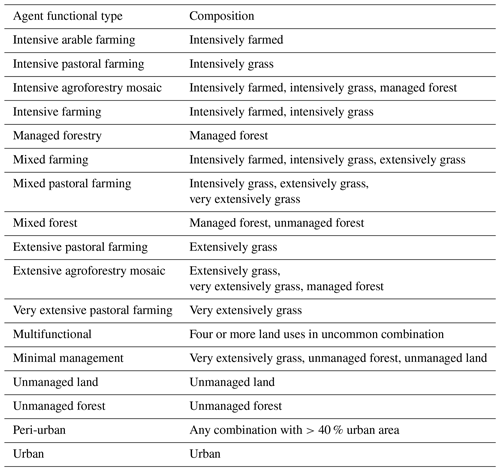
Table A2Identities and details of modelled capitals. Exact parallels for some capitals were available in the IAP.
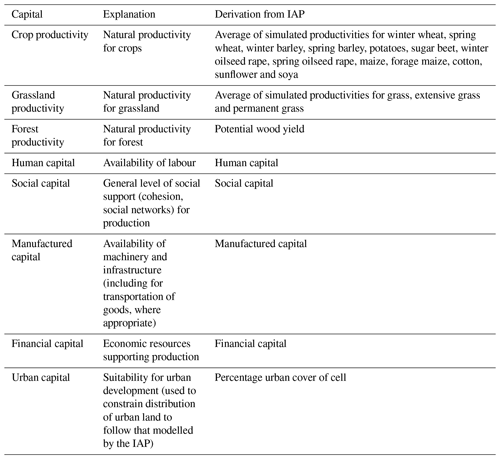
Table A3(a)–(q) Tables showing the sensitivities λc,t of each AFT to capital levels and maximum service production levels os,t (italics) (Eq. 1). Values in brackets are the standard deviations of Gaussian distributions used in some simulations to randomly assign production levels to individual agents. Standardised units of production are shown for comparability and consistency with model calculations.

Table A4Behavioural parameter variations used in the simulations. Parameter set 1 is the default from which main results are derived; in this setup agents respond directly to benefit values with no additional individual or typological behaviour. In parameter set 2, abandonment and competition thresholds are altered to introduce abandonment of land when benefit values fall below the abandonment threshold value and resistance to change unless a competing land use has an additional benefit value of at least the competition threshold. Intensive land use agents are parameterised to be less tolerant of low benefit values and more willing to switch to a land use with higher benefit values. In parameter set 3, individual agents differ from one another in terms of their abilities to produce different ecosystem services as well as their abandonment and competition thresholds. Parameter sets 4 and 5 replicate parameter sets 2 and 3, respectively, but with larger values for thresholds and variations, with threshold values of up to approximately 100 % of mean benefit values.
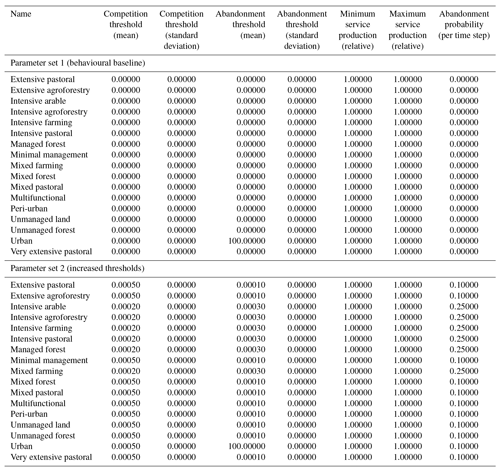
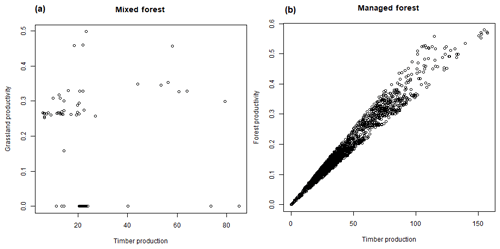
Figure A1Example capital–service relationships in IAP output data used to quantify the capital sensitivities for AFTs in CRAFTY-EU. Timber production by mixed forest agents (a) is found to be almost completely insensitive to grassland productivity capital, giving a λc,t value of 0, while timber production by managed forest agents (b) is highly sensitive to forest productivity, giving a λc,t value of 1.
The evaluation of CRAFTY-EU builds on previous evaluations of the agent-based modelling framework from which CRAFTY-EU is implemented, as well as the evaluation of previous comparable implementations. These evaluations have included sensitivity and uncertainty analyses (Arneth et al., 2014; Brown et al., 2014b, 2018b; Murray-Rust et al., 2014a), model inter-comparison (Alexander et al., 2017; Holman et al., 2017), and validation against independently simulated and empirical data (Blanco et al., 2017a, b). These evaluations are wholly or partially relevant to CRAFTY-EU as they deal, at least in part, with the basic architecture and parameters of the modelling framework, which are shared between all applications. The model is also fully open access, with the code and (ODD+) descriptions of the previous versions published (Murray-Rust et al., 2014b; Blanco et al., 2017a; Holzhauer, Brown and Rounsevell, 2019), and with CRAFTY-EU itself available in full (code base) or for immediate use (interactive mode) online (from https://bitbucket.org/geoslurg/crafty_cobra_impressions_kit/, last access: 3 December 2019 and https://landchange.imk-ifu.kit.edu/CRAFTY, last access: 3 December 2019). Furthermore, input data have been independently verified and evaluated during the development of the IMPRESSIONS IAP, from which CRAFTY-EU is calibrated (Harrison et al., 2012; Brown et al., 2014a; Kebede et al., 2015; Holman et al., 2017).
The evaluation here therefore focuses on the specific European implementation of CRAFTY. As described in the main text, the evaluation comprised two main exercises involving runs under static baseline conditions, the first starting from an unassigned (empty) land use map and the second from the baseline land use map derived from the IAP. The purpose of these two exercises was, respectively, (1) to check whether baseline conditions would generate a “realistic” land use configuration purely on the basis of capital levels and AFT characteristics (i.e. in the absence of any spatial information about land management) and (2) to check for divergence in outcomes from a common starting point consistent with other scenario runs.
The first exercise was conducted 10 times to check the magnitude of stochastic variation in model outputs and was expected to produce more variable outcomes for two reasons. Firstly, a number of potential “solutions” exist to the problem of producing given levels of ecosystem services from a given landscape, and while reality represents one of these, models unconstrained by initial land use maps should be able to produce – and potentially transition between – many others. This is particularly likely here given the dependencies of simulated land use decisions on several different factors (multiple capitals, demand levels and competition between agents). Furthermore, CRAFTY is a non-optimising and stochastic modelling framework with path dependencies in outcomes, allowing individual simulations to diverge when initial conditions are unstable, as is the case here. Nevertheless, the degree of conformance in the general characteristics of these simulations illuminates an important aspect of model stability, as well as revealing the predictability of model responses to spatial input conditions.
The second exercise was simpler to interpret, with large differences in land use between the start and end of the simulation taken to indicate model instability under static conditions. Systematic changes would suggest an inconsistency between CRAFTY-EU parameterisation and baseline conditions, and random change would suggest a more general instability. Either of these would also suggest an innate bias in model outputs with the potential to obscure the impacts of simulated scenarios. Model outputs were therefore assessed in terms of the number of agents within each AFT over time.
The first exercise was initially performed over 800 time steps, with 20 % of cells being randomly selected for potential change. This long time span and high rate of competition were chosen to exaggerate model dynamics, ensuring that they could be easily assessed through model outputs. Plots of AFT numbers and service levels were checked visually and statistically for stationarity (using Box–Ljung tests for temporal autocorrelation; Ljung and Box, 1978). Once an appropriate simulation duration had been identified, 10 further independent simulations were run to this point using different random number generator seed values. The outputs of these simulations were then compared in terms of total numbers of agents within each AFT, total service production levels, the spatial consistency of aggregated AFT classes across the 10 simulations and the similarity of these spatial patterns to that in the independent baseline map (to check for spontaneous convergence, which would suggest a broadly realistic response to initial conditions). The second exercise was performed over 100 time steps, again with a 20 % rate of cell selection. This exercise was designed to run in a sufficient number of replicates to identify and understand any divergence from stationarity in terms of numbers of agent per AFT, with stationarity again checked both visually and statistically and further runs used only when non-stationarity was detected.
Evaluations against historical data were not performed due to the lack of comprehensive data describing capital levels, demand levels and land use maps, other than those produced by alternative models (e.g. Fuchs et al., 2015).
B1 Results
B1.1 First evaluation exercise
The first evaluation exercise did not result in stationarity during the 800-time-step run period (as confirmed by Box–Ljung tests, in which most AFT time series had p values < 0.01 throughout the simulation). This suggests a tendency for ongoing oscillations in agent numbers (and hence service levels). Nevertheless, after an initial period of rapid change, all AFT numbers remained broadly consistent over time, with remaining short-term and apparent long-term fluctuations being small in comparison to overall agent numbers (Fig. B1). A cut-off of 300 time steps (equivalent to year 2300 in the simulations) was chosen for further analysis, as AFT numbers had achieved representative values by this point.
The numbers of agents belonging to each AFT at the 300th time step of each of the 10 replicate simulations were very similar (Fig. B2a), as were the service levels produced (Fig. B2b). Furthermore, the spatial consistency of aggregated AFT classes was high, and locations frequently agreed with those in the independent baseline land use map (Fig. B3). Aggregated land use classes were used here to check the assignment of land uses rather than specific agent types, which, being considerably more numerous and less discrete, speak to a different aspect of model behaviour (the balance between competitive and productive behaviours of different AFTs, rather than the appropriateness of ecosystem service production in particular locations under given demand levels).
B1.2 Second evaluation exercise
The second evaluation exercise (running the model under baseline conditions starting from the baseline land use map) showed stationarity throughout the simulation period (Fig. B4), and this was confirmed by Box–Ljung tests that showed no evidence of dependence in the time series of any of the AFTs. Absolute numbers of agents remained within 15 of the initial number in all cases. This was taken to demonstrate stability in the initial configuration of CRAFTY-EU, implying that changes observed during scenario simulations were fully attributable to the parameterisation of those scenarios rather than inherent variability or trends in model dynamics.
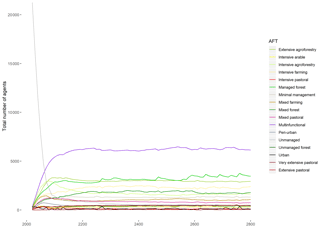
Figure B1Numbers of agents belonging to each agent functional type throughout an 800-time-step simulation to check for stationarity.
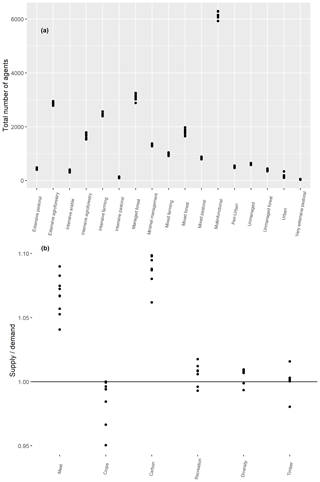
Figure B2Numbers of agents belonging to each agent functional type at the 300th time step of each of the 10 independent simulations with no initial land use map (a) and service levels as a proportion of demand levels at the same points (b).
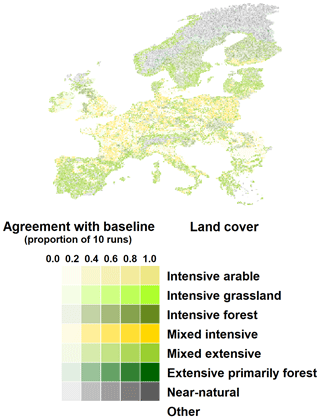
Figure B3Map of aggregated simulated land cover across the 10 evaluation simulations initialised with no baseline land use map. Baseline land cover is shown on the map, with opacity scaled to show the number of evaluation simulations in which that land cover occurred at the 300th time step.
The behavioural parameter variations explored in this study (and shown in Table A4) were analysed through three main outputs: their effect on food supply levels (Fig. 2), their effect on land use fragmentation (Fig. C1 below) and the emergent changes in those parameter values during simulations (Fig. C2 below).
Fragmentation varied broadly between parameterisations, as shown in Fig. C1, while behavioural parameter values themselves varied systematically in some scenarios (Fig. C2). This was especially the case in SSP3, for which the mean abandonment threshold decreased during simulations, the mean competition threshold increased, and the standard deviation of both decreased, indicating a more similar and persistent population of agents than was present at the start of the simulations.
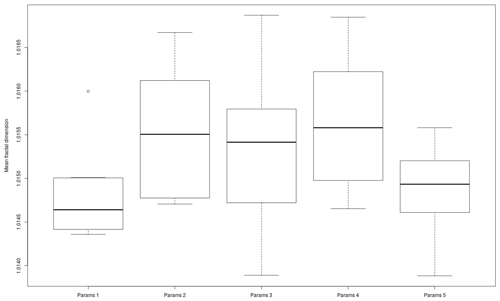
Figure C1Mean fractal dimension in each of the five behavioural parameter sets (parameters 1–5) measuring the fragmentation of land uses in 2086.
Supplement 1: demand files, giving ecosystem service demand values for each scenario and year. Supplement 2: further graphical results summaries. The supplement related to this article is available online at: https://doi.org/10.5194/esd-10-809-2019-supplement.
CB developed the model and drafted the paper; BS and CB performed sensitivity analyses, and BS developed calibration routines and the web platform; MR advised on model development and interpretation, and all authors finalised the paper.
The authors declare that they have no conflict of interest.
We acknowledge support by the KIT Publication Fund of the Karlsruhe Institute of Technology.
This research has been supported by the EU Framework Programme 7 (grant no. 603416) and the Helmholtz Association.
The article processing charges for this open-access
publication were covered by a Research
Centre of the Helmholtz Association.
This paper was edited by Michel Crucifix and reviewed by Gunnar Dressler and Patrick Meyfroidt.
Alexander, P., Prestele, R., Verburg, P. H., Arneth, A., Baranzelli, C., Batista e Silva, F., Brown, C., Butler, A., Calvin, K., Dendoncker, N., Doelman, J. C., Dunford, R., Engström, K., Eitelberg, D., Fujimori, S., Harrison, P. A., Hasegawa, T., Havlik, P., Holzhauer, S., Humpenöder, F., Jacobs-Crisioni, C., Jain, A. K., Krisztin, T., Kyle, P., Lavalle, C., Lenton, T., Liu, J., Meiyappan, P., Popp, A., Powell, T., Sands, R. D., Schaldach, R., Stehfest, E., Steinbuks, J., Tabeau, A., van Meijl, H., Wise, M. A., and Rounsevell, M. D. A.: Assessing uncertainties in land cover projections, Global Change Biol., 23, 767–781, https://doi.org/10.1111/gcb.13447, 2017.
An, L.: Modeling human decisions in coupled human and natural systems: Review of agent-based models, Ecol. Model., 229, 25–36, https://doi.org/10.1016/j.ecolmodel.2011.07.010, 2012.
Arneth, A., Brown, C., and Rounsevell, M. D. A.: Global models of human decision-making for land-based mitigation and adaptation assessment, Nat. Clim. Change, 4, 550–557, https://doi.org/10.1038/nclimate2250, 2014.
Arthur, W. B.: Chapter 32 Out-of-Equilibrium Economics and Agent-Based Modeling, Handb. Comput. Econ., 2, 1551–1564, https://doi.org/10.1016/S1574-0021(05)02032-0, 2006.
Bai, X., van der Leeuw, S., O'Brien, K., Berkhout, F., Biermann, F., Brondizio, E. S., Cudennec, C., Dearing, J., Duraiappah, A., Glaser, M., Revkin, A., Steffen, W., and Syvitski, J.: Plausible and desirable futures in the Anthropocene: A new research agenda, Global Environ. Change, 39, 351–362, https://doi.org/10.1016/J.GLOENVCHA.2015.09.017, 2016.
Balint, T., Lamperti, F., Mandel, A., Napoletano, M., Roventini, A., and Sapio, A.: Complexity and the Economics of Climate Change: A Survey and a Look Forward, Ecol. Econ., 138, 252–265, https://doi.org/10.1016/J.ECOLECON.2017.03.032, 2017.
Beven, K.: Towards integrated environmental models of everywhere: uncertainty, data and modelling as a learning process, Hydrol. Earth Syst. Sci., 11, 460–467, https://doi.org/10.5194/hess-11-460-2007, 2007.
Blanco, V., Holzhauer, S., Brown, C., Lagergren, F., Vulturius, G., Lindeskog, M., and Rounsevell, M. D. A.: The effect of forest owner decision-making, climatic change and societal demands on land-use change and ecosystem service provision in Sweden, Ecosyst. Serv., 23, 174–208, https://doi.org/10.1016/j.ecoser.2016.12.003, 2017a.
Blanco, V., Brown, C., Holzhauer, S., Vulturius, G., and Rounsevell, M. D. A.: The importance of socio-ecological system dynamics in understanding adaptation to global change in the forestry sector, J. Environ. Manage., 196, 36–47, https://doi.org/10.1016/j.jenvman.2017.02.066, 2017b.
Brown, C., Brown, E., Murray-Rust, D., Cojocaru, G., Savin, C., and Rounsevell, M.: Analysing uncertainties in climate change impact assessment across sectors and scenarios, Climatic Change, 128, 293–306, https://doi.org/10.1007/s10584-014-1133-0, 2014a.
Brown, C., Murray-Rust, D., Van Vliet, J., Alam, S. J., Verburg, P. H., and Rounsevell, M. D.: Experiments in globalisation, food security and land use decision making, PLoS One, 9, 12, https://doi.org/10.1371/journal.pone.0114213, 2014b.
Brown, C., Brown, K., and Rounsevell, M.: A philosophical case for process-based modelling of land use change, Model. Earth Syst. Environ., 2, 50, https://doi.org/10.1007/s40808-016-0102-1, 2016a.
Brown, C., Bakam, I., Smith, P., and Matthews, R.: An agent-based modelling approach to evaluate factors influencing bioenergy crop adoption in north-east Scotland, GCB Bioenergy, 8, 226–244, https://doi.org/10.1111/gcbb.12261, 2016b.
Brown, C., Alexander, P., Holzhauer, S., and Rounsevell, M. D. A.: Behavioral models of climate change adaptation and mitigation in land-based sectors, Wiley Interdiscip. Rev. Clim. Change, 8, 2, https://doi.org/10.1002/wcc.448, 2017.
Brown, C., Alexander, P., and Rounsevell, M.: Empirical evidence for the diffusion of knowledge in land use change, J. Land Use Sci., 13, 269–283, https://doi.org/10.1080/1747423X.2018.1515995, 2018a.
Brown, C., Holzhauer, S., Metzger, M. J., Paterson, J. S., and Rounsevell, M.: Land managers' behaviours modulate pathways to visions of future land systems, Reg. Environ. Change, 18, 831–845, https://doi.org/10.1007/s10113-016-0999-y, 2018b.
Brown, C., Alexander, P., Arneth, A., Holman, I., and Rounsevell, M.: Achievement of Paris climate goals unlikely due to time lags in the land system, Nat. Clim. Change, 9, 203–208, https://doi.org/10.1038/s41558-019-0400-5, 2019a.
Brown, C., Seo, B., and Rounsevell, M.: Competition for Resources Between Agent Functional Types (CRAFTY), available at: https://landchange.imk-ifu.kit.edu/CRAFTY (last access: 3 December 2019), 2019b.
Calvin, K. and Bond-Lamberty, B.: Integrated human-earth system modeling – state of the science and future directions, Environ. Res. Lett., 13, 063006, https://doi.org/10.1088/1748-9326/aac642, 2018.
Cradock-Henry, N. A., Frame, B., Preston, B. L., Reisinger, A., and Rothman, D. S.: Dynamic adaptive pathways in downscaled climate change scenarios, Climatic Change, 150, 333–341, https://doi.org/10.1007/s10584-018-2270-7, 2018.
Cumming, G. S. and Peterson, G. D.: Unifying Research on Social–Ecological Resilience and Collapse, Trends Ecol. Evol., 32, 695–713, https://doi.org/10.1016/J.TREE.2017.06.014, 2017.
Delignette-Muller, M. L. and Dutang, C.: fitdistrplus: An R Package for Fitting Distributions, J. Stat. Softw., 64, 1–34, https://doi.org/10.18637/jss.v064.i04, 2015.
Dellink, R., Hwang, H., Lanzi, E., and Chateau, J.: International trade consequences of climate change, available at: https://www.oecd-ilibrary.org/trade/international-trade-consequences-of-climate-change_9f446180-en (last access: 17 December 2018), 2017.
Díaz, S., Pascual, U., Stenseke, M., Martín-López, B., Watson, R. T., Molnár, Z., Hill, R., Chan, K. M. A., Baste, I. A., Brauman, K. A., Polasky, S., Church, A., Lonsdale, M., Larigauderie, A., Leadley, P. W., van Oudenhoven, A. P. E., van der Plaat, F., Schröter, M., Lavorel, S., Aumeeruddy-Thomas, Y., Bukvareva, E., Davies, K., Demissew, S., Erpul, G., Failler, P., Guerra, C. A., Hewitt, C. L., Keune, H., Lindley, S., and Shirayama, Y.: Assessing nature's contributions to people, Science, 359, 270–272, https://doi.org/10.1126/science.aap8826, 2018.
Doelman, J. C., Stehfest, E., Tabeau, A., van Meijl, H., Lassaletta, L., Gernaat, D. E. H. J., Hermans, K., Harmsen, M., Daioglou, V., Biemans, H., van der Sluis, S., and van Vuuren, D. P.: Exploring SSP land-use dynamics using the IMAGE model: Regional and gridded scenarios of land-use change and land-based climate change mitigation, Global Environ. Change, 48, 119–135, https://doi.org/10.1016/J.GLOENVCHA.2017.11.014, 2018.
Donatelli, M., Magarey, R. D., Bregaglio, S., Willocquet, L., Whish, J. P. M., and Savary, S.: Modelling the impacts of pests and diseases on agricultural systems, Agric. Syst., 155, 213–224, https://doi.org/10.1016/J.AGSY.2017.01.019, 2017.
Ehrlich, P. R. and Ehrlich, A. H.: Can a collapse of global civilization be avoided?, Proc. Roy. Soc. B, 280, 20122845, https://doi.org/10.1098/rspb.2012.2845, 2013.
Fagiolo, G. and Roventini, A.: Macroeconomic Policy in DSGE and Agent-Based Models Redux: New Developments and Challenges Ahead, J. Artif. Soc. Soc. Simul., 20, 1, https://doi.org/10.18564/jasss.3280, 2017.
Farmer, J. D. and Geanakoplos, J.: The virtues and vices of equilibrium and the future of financial economics, Complexity, 14, 11–38, https://doi.org/10.1002/cplx.20261, 2009.
Fuchs, R., Herold, M., Verburg, P. H., Clevers, J. G. P. W., and Eberle, J.: Gross changes in reconstructions of historic land cover/use for Europe between 1900 and 2010, Global Change Biol., 21, 299–313, https://doi.org/10.1111/gcb.12714, 2015.
Fujimori, S., Hasegawa, T., Masui, T., Takahashi, K., Herran, D. S., Dai, H., Hijioka, Y., and Kainuma, M.: SSP3: AIM implementation of Shared Socioeconomic Pathways, Global Environ. Change, 42, 268–283, https://doi.org/10.1016/J.GLOENVCHA.2016.06.009, 2017.
Galaz, V., Biermann, F., Crona, B., Loorbach, D., Folke, C., Olsson, P., Nilsson, M., Allouche, J., Persson, Å., and Reischl, G.: `Planetary boundaries' — exploring the challenges for global environmental governance, Curr. Opin. Environ. Sustain., 4, 80–87, https://doi.org/10.1016/J.COSUST.2012.01.006, 2012.
Harrison, P. A., Holman, I. P., Cojocaru, G., Kok, K., Kontogianni, A., Metzger, M. J., and Gramberger, M.: Combining qualitative and quantitative understanding for exploring cross-sectoral climate change impacts, adaptation and vulnerability in Europe, Reg. Environ. Change, 13, 761–780, https://doi.org/10.1007/s10113-012-0361-y, 2012.
Harrison, P. A., Holman, I. P., and Berry, P. M.: Assessing cross-sectoral climate change impacts, vulnerability and adaptation: an introduction to the CLIMSAVE project, Climatic Change, 128, 153–167, https://doi.org/10.1007/s10584-015-1324-3, 2015.
Harrison, P. A., Dunford, R. W., Holman, I. P., and Rounsevell, M. D. A.: Climate change impact modelling needs to include cross-sectoral interactions, Nat. Clim. Change, 6, 885–890, https://doi.org/10.1038/nclimate3039, 2016.
Harrison, P. A., Dunford, R. W., Holman, I. P., Cojocaru, G., Madsen, M. S., Chen, P. Y., Pedde, S., and Sandars, D.: Differences between low-end and high-end climate change impacts in Europe across multiple sectors, Reg. Environ. Change, 19, 695–709, https://doi.org/10.1007/s10113-018-1352-4, 2019.
Hasegawa, T., Fujimori, S., Takahashi, K., and Masui, T.: Scenarios for the risk of hunger in the twenty-first century using Shared Socioeconomic Pathways, Environ. Res. Lett., 10, 014010, https://doi.org/10.1088/1748-9326/10/1/014010, 2015.
Hazell, P. and Wood, S.: Drivers of change in global agriculture, Philos. T. Roy. Soc. B, 363, 495–515, https://doi.org/10.1098/rstb.2007.2166, 2008.
Heistermann, M., Müller, C. and Ronneberger, K.: Land in sight: Achievements, deficits and potentials of continental to global scale land-use modeling, Agr. Ecosyst. Environ., 114, 141–158, https://doi.org/10.1016/J.AGEE.2005.11.015, 2006.
Holman, I. P., Brown, C., Janes, V., and Sandars, D.: Can we be certain about future land use change in Europe? A multi-scenario, integrated-assessment analysis, Agric. Syst., 151, 126–135, https://doi.org/10.1016/j.agsy.2016.12.001, 2017.
Holzhauer, S., Brown, C., Murray-Rust, D., and Rounsevell, M.: Home – CRAFTY – Wiki Service, available at: https://www.wiki.ed.ac.uk/display/CRAFTY/Home (last access: 24 July 2018), 2016.
Holzhauer, S., Brown, C., and Rounsevell, M.: Modelling dynamic effects of multi-scale institutions on land use change, Reg. Environ. Change, 19, 733–746, https://doi.org/10.1007/s10113-018-1424-5, 2019.
Hooke, R. L. and Martín-Duque, J. F.: Land transformation by humans: A review, GSA Today, 12, 4–10, https://doi.org/10.1130/GSAT151A.1, 2012.
Huber, R., Bakker, M., Balmann, A., Berger, T., Bithell, M., Brown, C., Grêt-Regamey, A., Xiong, H., Le, Q. B., Mack, G., Meyfroidt, P., Millington, J., Müller, B., Polhill, J. G., Sun, Z., Seidl, R., Troost, C., and Finger, R.: Representation of decision-making in European agricultural agent-based models, Agric. Syst., 167, 143–160, https://doi.org/10.1016/J.AGSY.2018.09.007, 2018.
IMPRESSIONS Project: IAP2, available at: http://5.2.157.195/betaIAP2/, last access: 24 July 2018.
Kay, S., Graves, A., Palma, J. H. N., Moreno, G., Roces-Díaz, J. V., Aviron, S., Chouvardas, D., Crous-Duran, J., Ferreiro-Domínguez, N., García de Jalón, S., Măcicăşan, V., Mosquera-Losada, M. R., Pantera, A., Santiago-Freijanes, J. J., Szerencsits, E., Torralba, M., Burgess, P. J., and Herzog, F.: Agroforestry is paying off – Economic evaluation of ecosystem services in European landscapes with and without agroforestry systems, Ecosyst. Serv., 36, 100896, https://doi.org/10.1016/J.ECOSER.2019.100896, 2019.
Kebede, A. S., Dunford, R., Mokrech, M., Audsley, E., Harrison, P. A., Holman, I. P., Nicholls, R. J., Rickebusch, S., Rounsevell, M. D. A., Sabaté, S., Sallaba, F., Sanchez, A., Savin, C., Trnka, M., and Wimmer, F.: Direct and indirect impacts of climate and socio-economic change in Europe: a sensitivity analysis for key land- and water-based sectors, Climatic Change, 128, 261–277, https://doi.org/10.1007/s10584-014-1313-y, 2015.
Kebede, A. S., Nicholls, R. J., Allan, A., Arto, I., Cazcarro, I., Fernandes, J. A., Hill, C. T., Hutton, C. W., Kay, S., Lázár, A. N., Macadam, I., Palmer, M., Suckall, N., Tompkins, E. L., Vincent, K., and Whitehead, P. W.: Applying the global RCP–SSP–SPA scenario framework at sub-national scale: A multi-scale and participatory scenario approach, Sci. Total Environ., 635, 659–672, https://doi.org/10.1016/J.SCITOTENV.2018.03.368, 2018.
Kok, K., Pedde, S., Gramberger, M., Harrison, P. A., and Holman, I. P.: New European socio-economic scenarios for climate change research: operationalising concepts to extend the shared socio-economic pathways, Reg. Environ. Change, 19, 643–654, https://doi.org/10.1007/s10113-018-1400-0, 2019.
Letourneau, A., Verburg, P. H., and Stehfest, E.: A land-use systems approach to represent land-use dynamics at continental and global scales, Environ. Model. Softw., 33, 61–79, https://doi.org/10.1016/j.envsoft.2012.01.007, 2012.
Lippe, M., Bithell, M., Gotts, N., Natalini, D., Barbrook-Johnson, P., Giupponi, C., Hallier, M., Hofstede, G. J., Le Page, C., Matthews, R. B., Schlüter, M., Smith, P., Teglio, A., and Thellmann, K.: Using agent-based modelling to simulate social-ecological systems across scales, Geoinformatica, 23, 269–298, https://doi.org/10.1007/s10707-018-00337-8, 2019.
Ljung, G. M. and Box, G. E. P.: On a Measure of Lack of Fit in Time Series Models, Biometrika, 65, 297, https://doi.org/10.2307/2335207, 1978.
Loveland, T., Mahmood, R., Patel-Weynand, T., Karstensen, K., Beckendorf, K., Bliss, N., and Carleton, A.: National climate assessment technical report on the impacts of climate and land use and land cover change, US Geological Survey Open-File Report 2012-1155, US Geological Survey, Reston, Virginia, 2012.
Magliocca, N.: Model-Based Synthesis of Locally Contingent Responses to Global Market Signals, Land, 4, 807–841, https://doi.org/10.3390/land4030807, 2015.
Mandelbrot, B. B.: The fractal geometry of nature, WH Freeman, New York, 1983.
McDermid, S. S., Mearns, L. O., and Ruane, A. C.: Representing agriculture in Earth System Models: Approaches and priorities for development, J. Adv. Model. Earth Syst., 9, 2230–2265, https://doi.org/10.1002/2016MS000749, 2017.
Meyfroidt, P., Roy Chowdhury, R., de Bremond, A., Ellis, E. C., Erb, K.-H., Filatova, T., Garrett, R. D., Grove, J. M., Heinimann, A., Kuemmerle, T., Kull, C. A., Lambin, E. F., Landon, Y., le Polain de Waroux, Y., Messerli, P., Müller, D., Nielsen, J. Ø., Peterson, G. D., Rodriguez García, V., Schlüter, M., Turner, B. L., and Verburg, P. H.: Middle-range theories of land system change, Global Environ. Change, 53, 52–67, https://doi.org/10.1016/J.GLOENVCHA.2018.08.006, 2018.
Müller-Hansen, F., Schlüter, M., Mäs, M., Donges, J. F., Kolb, J. J., Thonicke, K., and Heitzig, J.: Towards representing human behavior and decision making in Earth system models – an overview of techniques and approaches, Earth Syst. Dynam., 8, 977–1007, https://doi.org/10.5194/esd-8-977-2017, 2017.
Murray-Rust, D., Brown, C., van Vliet, J., Alam, S. J., Robinson, D. T., Verburg, P. H., and Rounsevell, M.: Combining agent functional types, capitals and services to model land use dynamics, Environ. Model. Softw., 59, 187–201, https://doi.org/10.1016/j.envsoft.2014.05.019, 2014.
Newbold, T., Hudson, L. N., Arnell, A. P., Contu, S., De Palma, A., Ferrier, S., Hill, S. L. L., Hoskins, A. J., Lysenko, I., Phillips, H. R. P., Burton, V. J., Chng, C. W. T., Emerson, S., Gao, D., Pask-Hale, G., Hutton, J., Jung, M., Sanchez-Ortiz, K., Simmons, B. I., Whitmee, S., Zhang, H., Scharlemann, J. P. W., and Purvis, A.: Has land use pushed terrestrial biodiversity beyond the planetary boundary? A global assessment, Science, 353, 288–291, https://doi.org/10.1126/science.aaf2201, 2016.
O'Neill, B. C., Kriegler, E., Ebi, K. L., Kemp-Benedict, E., Riahi, K., Rothman, D. S., van Ruijven, B. J., van Vuuren, D. P., Birkmann, J., Kok, K., Levy, M., and Solecki, W.: The roads ahead: Narratives for shared socioeconomic pathways describing world futures in the 21st century, Global Environ. Change, 42, 169–180, https://doi.org/10.1016/j.gloenvcha.2015.01.004, 2017.
Paul, C., Weber, M., and Knoke, T.: Agroforestry versus farm mosaic systems – Comparing land-use efficiency, economic returns and risks under climate change effects, Sci. Total Environ., 587–588, 22–35, https://doi.org/10.1016/j.scitotenv.2017.02.037, 2017.
Pedde, S., Kok, K., Hölscher, K., Frantzeskaki, N., Holman, I., Dunford, R., Smith, A., and Jäger, J.: Advancing the use of scenarios to understand society's capacity to achieve the 1.5 degree target, Global Environ. Change, 56, 75–85, https://doi.org/10.1016/J.GLOENVCHA.2019.03.010, 2019a.
Pedde, S., Kok, K., Onigkeit, J., Brown, C., Holman, I., and Harrison, P. A.: Bridging uncertainty concepts across narratives and simulations in environmental scenarios, Reg. Environ. Change, 19, 655–666, https://doi.org/10.1007/s10113-018-1338-2, 2019b.
Petit, C. C. and Lambin, E. F.: Long-term land-cover changes in the Belgian Ardennes (1775–1929): model-based reconstruction vs. historical maps, Global Change Biol., 8, 616–630, https://doi.org/10.1046/j.1365-2486.2002.00500.x, 2002.
Pongratz, J., Reick, C., Raddatz, T., and Claussen, M.: A reconstruction of global agricultural areas and land cover for the last millennium, Global Biogeochem. Cy., 22, GB3018, https://doi.org/10.1029/2007GB003153, 2008.
Popp, A., Calvin, K., Fujimori, S., Havlik, P., Humpenöder, F., Stehfest, E., Bodirsky, B. L., Dietrich, J. P., Doelmann, J. C., Gusti, M., Hasegawa, T., Kyle, P., Obersteiner, M., Tabeau, A., Takahashi, K., Valin, H., Waldhoff, S., Weindl, I., Wise, M., Kriegler, E., Lotze-Campen, H., Fricko, O., Riahi, K., and van Vuuren, D. P.: Land-use futures in the shared socio-economic pathways, Global Environ. Change, 42, 331–345, https://doi.org/10.1016/J.GLOENVCHA.2016.10.002, 2017.
Ramankutty, N., Evan, A. T., Monfreda, C., and Foley, J. A.: Farming the planet: 1. Geographic distribution of global agricultural lands in the year 2000, Global Biogeochem. Cy., 22, GB1003, https://doi.org/10.1029/2007GB002952, 2008.
Riahi, K., van Vuuren, D. P., Kriegler, E., Edmonds, J., O'Neill, B. C., Fujimori, S., Bauer, N., Calvin, K., Dellink, R., Fricko, O., Lutz, W., Popp, A., Cuaresma, J. C., KC, S., Leimbach, M., Jiang, L., Kram, T., Rao, S., Emmerling, J., Ebi, K., Hasegawa, T., Havlik, P., Humpenöder, F., Da Silva, L. A., Smith, S., Stehfest, E., Bosetti, V., Eom, J., Gernaat, D., Masui, T., Rogelj, J., Strefler, J., Drouet, L., Krey, V., Luderer, G., Harmsen, M., Takahashi, K., Baumstark, L., Doelman, J. C., Kainuma, M., Klimont, Z., Marangoni, G., Lotze-Campen, H., Obersteiner, M., Tabeau, A., and Tavoni, M.: The Shared Socioeconomic Pathways and their energy, land use, and greenhouse gas emissions implications: An overview, Global Environ. Change, 42, 153–168, https://doi.org/10.1016/J.GLOENVCHA.2016.05.009, 2017.
Robinson, D. T., Di Vittorio, A., Alexander, P., Arneth, A., Barton, C. M., Brown, D. G., Kettner, A., Lemmen, C., O&apos;Neill, B. C., Janssen, M., Pugh, T. A. M., Rabin, S. S., Rounsevell, M., Syvitski, J. P., Ullah, I., and Verburg, P. H.: Modelling feedbacks between human and natural processes in the land system, Earth Syst. Dynam., 9, 895–914, https://doi.org/10.5194/esd-9-895-2018, 2018.
Rounsevell, M. D. A., Pedroli, B., Erb, K.-H., Gramberger, M., Busck, A. G., Haberl, H., Kristensen, S., Kuemmerle, T., Lavorel, S., Lindner, M., Lotze-Campen, H., Metzger, M. J., Murray-Rust, D., Popp, A., Pérez-Soba, M., Reenberg, A., Vadineanu, A., Verburg, P. H., and Wolfslehner, B.: Challenges for land system science, Land Use Policy, 29, 899–910, https://doi.org/10.1016/j.landusepol.2012.01.007, 2012a.
Rounsevell, M. D. A., Robinson, D. T., and Murray-Rust, D.: From actors to agents in socio-ecological systems models, Philos. T. Roy. Soc. Lond. B, 367, 259–269, 2012b.
Rounsevell, M. D. A., Arneth, A., Alexander, P., Brown, D. G., de Noblet-Ducoudré, N., Ellis, E., Finnigan, J., Galvin, K., Grigg, N., Harman, I., Lennox, J., Magliocca, N., Parker, D., O'Neill, B. C., Verburg, P. H., and Young, O.: Towards decision-based global land use models for improved understanding of the Earth system, Earth Syst. Dynam., 5, 117–137, https://doi.org/10.5194/esd-5-117-2014, 2014.
Schelhaas, M.-J., Nabuurs, G.-J., Hengeveld, G., Reyer, C., Hanewinkel, M., Zimmermann, N. E., and Cullmann, D.: Alternative forest management strategies to account for climate change-induced productivity and species suitability changes in Europe, Reg. Environ. Change, 15, 1581–1594, https://doi.org/10.1007/s10113-015-0788-z, 2015.
Schmitz, C., van Meijl, H., Kyle, P., Nelson, G. C., Fujimori, S., Gurgel, A., Havlik, P., Heyhoe, E., d'Croz, D. M., Popp, A., Sands, R., Tabeau, A., van der Mensbrugghe, D., von Lampe, M., Wise, M., Blanc, E., Hasegawa, T., Kavallari, A., and Valin, H.: Land-use change trajectories up to 2050: insights from a global agro-economic model comparison, Agric. Econ., 45, 69–84, https://doi.org/10.1111/agec.12090, 2014.
Searchinger, T. D., Beringer, T., and Strong, A.: Does the world have low-carbon bioenergy potential from the dedicated use of land?, Energy Policy, 110, 434–446, https://doi.org/10.1016/J.ENPOL.2017.08.016, 2017.
Sereke, F., Dobricki, M., Wilkes, J., Kaeser, A., Graves, A. R., Szerencsits, E., and Herzog, F.: Swiss farmers don't adopt agroforestry because they fear for their reputation, Agrofor. Syst., 90, 385–394, https://doi.org/10.1007/s10457-015-9861-3, 2016.
Smith, L. A.: Disentangling Uncertainty and Error: On the Predictability of Nonlinear Systems, in Nonlinear Dynamics and Statistics, Birkhäuser, Boston, MA, 31–64, 2001.
Smith, P., Gregory, P. J., van Vuuren, D., Obersteiner, M., Havlík, P., Rounsevell, M., Woods, J., Stehfest, E., and Bellarby, J.: Competition for land, Philos. T. Roy. Soc. Lond. B, 365, 2941–2957, https://doi.org/10.1098/rstb.2010.0127, 2010.
Steffen, W., Richardson, K., Rockström, J., Cornell, S. E., Fetzer, I., Bennett, E. M., Biggs, R., Carpenter, S. R., de Vries, W., de Wit, C. A., Folke, C., Gerten, D., Heinke, J., Mace, G. M., Persson, L. M., Ramanathan, V., and Reyers, B.: Planetary boundaries: Guiding human development on a changing planet. Science, Science, 348, 12–14, https://doi.org/10.1126/science.1259855, 2015.
Stevanović, M., Popp, A., Lotze-Campen, H., Dietrich, J. P., Müller, C., Bonsch, M., Schmitz, C., Bodirsky, B. L., Humpenöder, F., and Weindl, I.: The impact of high-end climate change on agricultural welfare, Sci. Adv., 2, e1501452, https://doi.org/10.1126/sciadv.1501452, 2016.
Stürck, J., Levers, C., van der Zanden, E. H., Schulp, C. J. E., Verkerk, P. J., Kuemmerle, T., Helming, J., Lotze-Campen, H., Tabeau, A., Popp, A., Schrammeijer, E., and Verburg, P.: Simulating and delineating future land change trajectories across Europe, Reg. Environ. Change, 18, 733–749, https://doi.org/10.1007/s10113-015-0876-0, 2018.
Sutherland, L.-A. and Burton, R. J. F.: Good Farmers, Good Neighbours? The Role of Cultural Capital in Social Capital Development in a Scottish Farming Community, Sociol. Ruralis, 51, 238–255, https://doi.org/10.1111/j.1467-9523.2011.00536.x, 2011.
Synes, N. W., Brown, C., Palmer, S. C. F., Bocedi, G., Osborne, P. E., Watts, K., Franklin, J., and Travis, J. M. J.: Coupled land use and ecological models reveal emergence and feedbacks in socio-ecological systems, Ecography, 42, 814–825, https://doi.org/10.1111/ecog.04039, 2019.
Terama, E., Clarke, E., Rounsevell, M. D. A., Fronzek, S., and Carter, T. R.: Modelling population structure in the context of urban land use change in Europe, Reg. Environ. Change, 19, 667–677, https://doi.org/10.1007/s10113-017-1194-5, 2019.
Turner, P. A., Field, C. B., Lobell, D. B., Sanchez, D. L., and Mach, K. J.: Unprecedented rates of land-use transformation in modelled climate change mitigation pathways, Nat. Sustain., 1, 240–245, https://doi.org/10.1038/s41893-018-0063-7, 2018.
United Nations: Sustainable Development Goals, available at: http://www.bmz.de/de/ministerium/ziele/2030_agenda/index.html (last access: 24 June 2012), 2017.
Valbuena, D., Verburg, P. H., Bregt, A. K., and Ligtenberg, A.: An agent-based approach to model land-use change at a regional scale, Landsc. Ecol., 25, 185–199, https://doi.org/10.1007/s10980-009-9380-6, 2010.
Vanderwal, J., Falconi, L., Januchowski, S., Shoo, L., and Storlie, C.: Package `SDMTools', Species Distribution Modelling Tools: Tools for processing data associated with species distribution modelling exercises, R-package version 1.1.12, available at: http://www.rforge.net/SDMTools/ (last access: 3 December 2019), 2014.
van Vliet, J., de Groot, H. L. F., Rietveld, P., and Verburg, P. H.: Manifestations and underlying drivers of agricultural land use change in Europe, Landsc. Urban Plan., 133, 24–36, https://doi.org/10.1016/J.LANDURBPLAN.2014.09.001, 2015.
van Vliet, J., Verburg, P. H., Grădinaru, S. R., and Hersperger, A. M.: Beyond the urban-rural dichotomy: Towards a more nuanced analysis of changes in built-up land, Comput. Environ. Urban Syst., 74, 41–49, https://doi.org/10.1016/J.COMPENVURBSYS.2018.12.002, 2019.
Verburg, P. H., van Asselen, S., van der Zanden, E. H., and Stehfest, E.: The representation of landscapes in global scale assessments of environmental change, Landsc. Ecol., 28, 1067–1080, https://doi.org/10.1007/s10980-012-9745-0, 2013.
Verburg, P. H., Dearing, J. A., Dyke, J. G., van der Leeuw, S., Seitzinger, S., Steffen, W., and Syvitski, J.: Methods and approaches to modelling the Anthropocene, Global Environ. Change, 39, 328–340, https://doi.org/10.1016/j.gloenvcha.2015.08.007, 2015.
Verkerk, P. J., Lindner, M., Pérez-Soba, M., Paterson, J. S., Helming, J., Verburg, P. H., Kuemmerle, T., Lotze-Campen, H., Moiseyev, A., Müller, D., Popp, A., Schulp, C. J. E., Stürck, J., Tabeau, A., Wolfslehner, B., and van der Zanden, E. H.: Identifying pathways to visions of future land use in Europe, Reg. Environ. Change, 18, 817–830, https://doi.org/10.1007/s10113-016-1055-7, 2018.
von Lampe, M., Willenbockel, D., Ahammad, H., Blanc, E., Cai, Y., Calvin, K., Fujimori, S., Hasegawa, T., Havlik, P., Heyhoe, E., Kyle, P., Lotze-Campen, H., Mason d'Croz, D., Nelson, G. C., Sands, R. D., Schmitz, C., Tabeau, A., Valin, H., van der Mensbrugghe, D., and van Meijl, H.: Why do global long-term scenarios for agriculture differ? An overview of the AgMIP Global Economic Model Intercomparison, Agric. Econ., 45, 3–20, https://doi.org/10.1111/agec.12086, 2014.
Weiss, H. and Bradley, R. S.: What drives societal collapse?, Science, 291, 609–610, https://doi.org/10.1126/science.1058775, 2001.
- Abstract
- Introduction
- Methods
- Results
- Discussion
- Conclusions
- Code and data availability
- Appendix A: Model parameterisation
- Appendix B: Model evaluation
- Appendix C: Additional behavioural parameter variation results
- Author contributions
- Competing interests
- Acknowledgements
- Financial support
- Review statement
- References
- Supplement
- Abstract
- Introduction
- Methods
- Results
- Discussion
- Conclusions
- Code and data availability
- Appendix A: Model parameterisation
- Appendix B: Model evaluation
- Appendix C: Additional behavioural parameter variation results
- Author contributions
- Competing interests
- Acknowledgements
- Financial support
- Review statement
- References
- Supplement