the Creative Commons Attribution 4.0 License.
the Creative Commons Attribution 4.0 License.
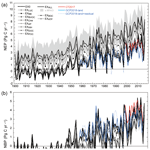
Disequilibrium of terrestrial ecosystem CO2 budget caused by disturbance-induced emissions and non-CO2 carbon export flows: a global model assessment
The global carbon budget of terrestrial ecosystems is chiefly determined by major flows of carbon dioxide (CO2) such as photosynthesis and respiration, but various minor flows exert considerable influence in determining carbon stocks and their turnover. This study assessed the effects of eight minor carbon flows on the terrestrial carbon budget using a process-based model, the Vegetation Integrative SImulator for Trace gases (VISIT), which included non-CO2 carbon flows, such as methane and biogenic volatile organic compound (BVOC) emissions and subsurface carbon exports and disturbances such as biomass burning, land-use changes, and harvest activities. The range of model-associated uncertainty was evaluated through parameter-ensemble simulations and the results were compared with corresponding observational and modeling studies. In the historical period of 1901–2016, the VISIT simulation indicated that the minor flows substantially influenced terrestrial carbon stocks, flows, and budgets. The simulations estimated mean net ecosystem production in 2000–2009 as 3.21±1.1 Pg C yr−1 without minor flows and 6.85±0.9 Pg C yr−1 with minor flows. Including minor carbon flows yielded an estimated net biome production of 1.62±1.0 Pg C yr−1 in the same period. Biomass burning, wood harvest, export of organic carbon by water erosion, and BVOC emissions had impacts on the global terrestrial carbon budget amounting to around 1 Pg C yr−1 with specific interannual variabilities. After including the minor flows, ecosystem carbon storage was suppressed by about 440 Pg C, and its mean residence time was shortened by about 2.4 years. The minor flows occur heterogeneously over the land, such that BVOC emission, subsurface export, and wood harvest occur mainly in the tropics, and biomass burning occurs extensively in boreal forests. They also differ in their decadal trends, due to differences in their driving factors. Aggregating the simulation results by land-cover type, cropland fraction, and annual precipitation yielded more insight into the contributions of these minor flows to the terrestrial carbon budget. Considering their substantial and unique roles, these minor flows should be taken into account in the global carbon budget in an integrated manner.
- Article
(6875 KB) - Full-text XML
-
Supplement
(3628 KB) - BibTeX
- EndNote
The terrestrial ecosystem is a substantial sink of atmospheric carbon dioxide (CO2) at decadal or longer scales and is mainly responsible for interannual variability of the global carbon budget (Schimel et al., 2001; Le Quéré et al., 2018). The current and future carbon budgets of terrestrial ecosystems have a feedback effect on the ongoing climate change, and they thus affect the effectiveness of climate mitigation policies such as the Paris Agreement (Friedlingstein et al., 2014; Seneviratne et al., 2016; Schleussner et al., 2016). Many studies have been conducted to elucidate the present global carbon budget, which is necessary for making reliable climate predictions (e.g., Sitch et al., 2015). Advances in flux-tower measurement networks, satellite observations, and data–model fusion have greatly improved our understanding of the terrestrial carbon budget and our ability to quantify it (Ciais et al., 2014; Li et al., 2016; Sellers et al., 2018).
However, large uncertainties remain in the current accounting of the global carbon budget. Present estimates of terrestrial gross primary production (GPP), the largest component of the ecosystem carbon cycle, range from 105 to 170 Pg C yr−1 (Baldocchi et al., 2015), and present estimates of soil organic carbon, a large stock in the global biogeochemical carbon cycle, range from 425 to 3040 Pg C (Todd-Brown et al., 2013; Tian et al., 2015). The implication is that detecting deviations of a few petagrams of carbon with high confidence is problematic. Recent products of remote-sensing and upscaled flux measurement data (e.g., Zhao et al., 2006; Tramontana et al., 2016) are fairly consistent in their spatial patterns of terrestrial carbon flows, but they still differ in their average magnitudes and interannual variability. Observations of isotopes and covarying tracers (e.g., carbonyl sulfide) provide supporting data (e.g., Welp et al., 2011; Campbell et al., 2017), but estimates have not converged to a consistent value. Quantifying the net carbon balance is even more difficult, primarily because it is a small difference between large sink and source fluxes that vary spatially and temporally. A recent synthesis of the global carbon budget using both top–down and bottom–up data (Le Quéré et al., 2018) gives a plausible estimate for the terrestrial carbon budget: a net sink of 3.0±0.8 Pg C yr−1 in 2007–2016. However, it has the largest range of uncertainty among the components of the global carbon cycle.
The uncertainty in the terrestrial carbon budget arises not only from inadequacies in the observational data, but also from an oversimplified conceptual framework. A common index of the net ecosystem carbon budget, net ecosystem production (NEP), is defined as the difference between GPP and ecosystem respiration (RE), which places plant and soil CO2 exchange, as determined by their physiological properties, in the sole controlling role (Gower, 2003). NEP is expected to be equal to the change in the ecosystem carbon stocks of biomass and soil organic matter. This conceptual framework has been widely used in flux measurement, biometric, and modeling studies. However, as quantification of the carbon budget has become more sophisticated and accurate, minor carbon flows (MCFs), consisting of relatively small non-CO2 flows and disturbance-associated emissions, have grown in importance to close the budget. Among these, emissions and ecosystem dynamics associated with wildfires and land-use change have been investigated for decades in various ecosystems such as tropical and boreal forests (e.g., Houghton et al., 1983; Randerson et al., 2005). Subsurface riverine export from the land to the ocean also has been long investigated from biogeochemical and agricultural perspectives (e.g., Meybeck, 1993; Lal, 2003). Many subsequent studies have addressed the biogeochemical mechanisms and spatial–temporal patterns of different MCFs at ecosystem to global scales (e.g., Raymond et al., 2013; Galy et al., 2015; Arneth et al., 2017; Saunois et al., 2017). Accordingly, a revised concept of the net terrestrial carbon budget called net biome production (NBP) has been proposed (Schulze et al., 2000) to account for the effects of MCFs. Because NBP covers non-CO2, disturbance-induced emissions, and lateral transportations, this term is applicable to both natural and managed agricultural ecosystems. Although there remain controversies in the conceptual framework (Randerson et al., 2002; Lovett et al., 2006), NEP and NBP provide a useful basis for integrating carbon flows, carbon pools, and the carbon budget.
Few studies have assessed the importance of MCFs in the global carbon cycle in a quantitative, integrated manner. Several studies have implied that the magnitude of MCFs, while small in comparison with gross flows (about 100 Pg C yr−1), is comparable to the net budget (around 1 Pg C yr−1). It appears, then, that neglecting MCFs can lead to serious accounting biases and misunderstanding of regional carbon budgets. Previous studies of carbon observations (e.g., Chu et al., 2015; Webb et al., 2018) and syntheses (e.g., Jung et al., 2011; Piao et al., 2012; Zhang et al., 2014) have recognized the significance of certain MCFs, such as land-use emissions, but have not integrated them into a single framework (Kirschbaum et al., 2019).
This study estimated MCFs and assessed their influence on the global terrestrial carbon budget in an integrated manner. In this paper I describe a series of simulations conducted with a process-based terrestrial biogeochemical model, in which various MCFs were incorporated into the carbon balance, to distinguish the effect of each MCF and its driving forces. The temporal variability and geographic patterns of these MCFs were clarified. Finally, I discuss methodological uncertainty, potential leakage and duplication in the MCF accounting, linkages with observations and climate predictions, and future research opportunities.
2.1 Model description
This study adopted the Vegetation Integrative SImulator for Trace gases (VISIT), a process-based terrestrial ecosystem model that is more fully described elsewhere (Ito, 2010; Inatomi et al., 2010; a schematic diagram is shown in Fig. S1 in the Supplement). In comparison to other carbon cycle models, the model has a computationally efficient structure, making it feasible to conduct large numbers of long-term simulations. The model has participated in several model intercomparison projects, making it possible to assess the limitations of a single-model study. The model is composed of biophysical and biogeochemical modules that simulate atmosphere–ecosystem exchange and matter flows within ecosystems. The hydrology module simulates land-surface radiation and water budgets using forcing meteorological data such as incoming radiation, precipitation, air temperature, humidity, cloudiness, and wind speed and biophysical properties such as fractional vegetation coverage, albedo, and soil water-holding capacity. The land-surface water budget is simulated using a two-layer soil water scheme that calculates evapotranspiration by the Penman–Monteith equation and runoff discharge by the bucket model (Manabe, 1969). Snow accumulation and melting are also simulated.
The carbon cycle is simulated with a box-flow scheme composed of eight carbon pools (leaf, stem, and root carbon for both C3 and C4 plants, plus soil litter and humus) and gross and net carbon flows. An early version of the model simulated only major carbon flows related to CO2 exchange (Ito and Oikawa, 2002), such as photosynthesis, plant (autotrophic) respiration (RA), and microbial (heterotrophic) respiration (RH). Net ecosystem production (NEP) is defined as follows:
The total respiratory CO2 efflux (RA + RH) is called ecosystem respiration (RE). Thus, NEP represents net CO2 exchange with the atmosphere through ecosystem physiological processes (Gower, 2003). In the model, these processes are calculated using equations that include terms for responsiveness to environmental conditions such as light, temperature, CO2 concentration, and humidity.
Following carbon fixation by GPP, photosynthate is partitioned to the six plant carbon pools on the basis of production optimization and allometric constraints at every time step. Plant leaf phenology from leaf display to shedding is simulated in deciduous forests and grasslands, using an empirical procedure based mainly on threshold cumulative temperatures. From each vegetation carbon pool, a certain fraction of carbon is transferred to the soil litter pool, which has a specific turnover rate or residence time representing the decomposition of litter carbon into soil humus and eventually CO2. The VISIT model includes a nitrogen dynamics module that simulates nitrous oxide emission from the soil surface and other nitrogen flows, but this study was primarily focused on the carbon budget.
Note that the model has two separate layers: one for natural ecosystems and another for croplands. Almost all biogeochemical processes are simulated separately in the two layers and then weighted by their respective areas to obtain mean values for each grid cell. A transitional change in the fractions of natural ecosystems and cropland, associated with land-use conversion, results in interactions between the layers.
The VISIT model has been calibrated and validated with field data mostly related to the carbon cycle, such as plant productivity, biomass, leaf area index, and ecosystem CO2 fluxes (e.g., Ito and Oikawa, 2002; Inatomi et al., 2010; Hirata et al., 2014). Also, at regional to global scales, the model has been examined by comparisons with network and remote-sensing data (e.g., Ichii et al., 2013; Ito et al., 2017). Furthermore, the model has been part of model intercomparison projects. One was the Multi-scale Terrestrial Model Intercomparison Project, which examined terrestrial models in terms of the CO2 fertilization effect on GPP and its seasonal-cycle amplitude (Huntzinger et al., 2017; Ito et al., 2016) and soil carbon dynamics (Tian et al., 2015). Another was the Inter-Sectoral Impact Model Intercomparison Project, which compared terrestrial impact assessment models with various observational data such as satellite- and ground-measured GPP for benchmarking (Chen et al., 2017), responses to El Niño events (Fang et al., 2017), and turnover of carbon pools (Thurner et al., 2017). Moreover, the model participated in the TRENDY vegetation model intercomparison project and then contributed to the global CO2 synthesis (Le Quéré et al., 2018).
2.2 Minor carbon flows
The VISIT version used in this study includes various MCFs, which play unique and important roles in terrestrial ecosystems. Eight MCFs were included in the VISIT model in a common manner (Fig. 1): emissions associated with land-use change (FLUC), biomass burning by wildfire (FBB), emission of biogenic volatile organic compounds or biogenic volatile organic compounds (BVOCs) (FBVOC), methane emissions from wetlands and methane oxidation in uplands (), agricultural practices from cropping to harvesting (FAP), wood harvesting in forests (FWH), export of dissolved organic carbon (DOC) by rivers (FDOC), and displacement of soil particulate organic carbon (POC) by water erosion (FPOC). The net carbon balance including MCFs, called net biome production (NBP: Schulze et al., 2000), is more closely related than NEP to the changes in the ecosystem carbon pool. Note that NBP has similarities with and differences from other terms such as NEP, which has scale dependence (Randerson et al., 2002), and net ecosystem carbon balance (Chapin et al., 2006). As discussed later (Sect. 4.4), there remain inconsistencies in the definition of net terrestrial productions, including riverine export, inland water sedimentation, and human harvest and consumption. In this study, NBP is defined as
The MCFs differ markedly in their biogeochemical properties and therefore should be evaluated individually. For example, the first four flows are vertical exchanges with the atmosphere (FLUC, FBB, FBVOC, and ), whereas the second four are lateral transportations induced by water and human activities (FAP, FWH, FDOC, and FPOC). Flows associated with disturbances, such as wildfire (FBB) and land-use conversion (FLUC), are heterogeneous in space and time. To avoid double counting, these two flows were calculated separately: FLUC includes burning of debris after deforestation, and FBB excludes human-induced ignition.
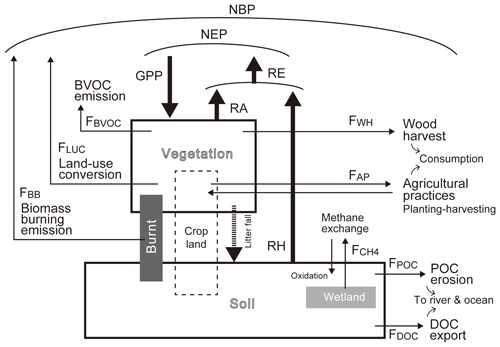
Figure 1Schematic diagram of the carbon budget of the terrestrial ecosystem as simulated in this study. Thick lines show major carbon flows, and thin lines show minor carbon flows.
2.2.1 Land-use change (FLUC)
Carbon emissions associated with land-use conversion were estimated for the historical period on the basis of a protocol proposed by McGuire et al. (2001), using the Land Use Harmonization (LUH) dataset (Hurtt et al., 2006). The LUH dataset provides both land-use states and their mutual transition matrix. First, the annual transition rate from primary and secondary lands to other land-use types was determined by the LUH dataset. This transition rate was multiplies by the average carbon stock in natural lands simulated by the VISIT model to estimate the amount of carbon affected by land-use conversion. This carbon was then separated into three components with different residence times from less than 1 year (detritus) to 100 years (wood products). The detritus, including dead root biomass, was transferred to the soil litter pool and then decomposed. The fractions of wood products with 10- and 100-year residence times are biome dependent (McGuire et al., 2001). Note that wood harvest not associated with land-use change (e.g., selective cutting) was separately evaluated as the FWH term (Sect. 2.2.6). The VISIT model has been used to assess the effects of land-use change from the point scale (Adachi et al., 2011; Hirata et al., 2014) to the global scale (Kato et al., 2013; Arneth et al., 2017).
2.2.2 Biomass burning (FBB)
Wildfire and associated biomass burning have been studied with respect to their effects on land disturbance, carbon biogeochemistry, and climatic interactions (e.g., Randerson et al., 2006; Knorr et al., 2016). The biomass burning scheme of the VISIT model has been described and evaluated by Kato et al. (2013). Biomass burning emission was calculated as follows:
where fBurned is the burned area fraction in natural vegetation, DC is the area-based carbon density, BI is the burned intensity (fraction of fire-affected carbon), and EFBB is the emission factor (emission per unit burned biomass). fBurned is estimated in a prognostic manner using an empirical fire scheme originally developed by Thonicke et al. (2001) for the Lund–Potsdam–Jena dynamic global vegetation model. This scheme estimates the length of the fire season and the corresponding burned area fraction from monthly values of soil water content and fuel load. Agricultural waste burning and prescribed fires for ecosystem management are not considered here. Differences in fire susceptibility among biomes are characterized by a parameter of critical moisture content for fire ignition. DC, fuel carbon stock per area, is obtained from the VISIT simulation; it is assumed that the plant leaf, stem, root, and soil litter stocks are subject to biomass burning. BI is a biome- and stock-specific parameter obtained from Hoelzemann et al. (2004), ranging from 0.0 for humid forest root to 1.0 for forest and grassland litter. The emission factor EFBB is also a biome- and stock-specific parameter and differs among emission substances; this study considered CO2, carbon monoxide, black carbon, and methane. EFBB values for each biome and stock were obtained from Hoelzemann et al. (2004). Other carbon flows associated with biomass burning, such as production and burial of charcoal, were not considered.
2.2.3 BVOC emission (FBVOC)
Emissions of BVOCs, such as isoprene and monoterpene, attract particular attention from atmospheric chemists, and several emission schemes have been developed. Here, a convenient scheme of Guenther (1997) was incorporated into the VISIT model with a few modifications. The scheme estimates BVOC emission as follows:
where EFBVOC is the emission factor of BVOC, FD is foliar density, DL is day length, and fPPFD, fTMP, and fPhenology are scalar coefficients for light (photosynthetic photon flux density), temperature, and phenological factors, respectively. EFBVOC was derived from Lathiére et al. (2006) for representative species such as isoprene, monoterpene, methanol, and acetone. FD, leaf carbon stock per ground area, and DL were from the VISIT simulation. Due to the difference in biochemical pathways, only isoprene emission is responsive to light intensity (fPPFD = 0–1), while other species are insensitive (fPPFD = 1). BVOC emission increases with temperature, and fTMP differs between isoprene and other monoterpene families. fPhenology, the effect of leaf aging, differs between evergreen and deciduous vegetation. Here, based on the model simulation, leaf age distribution was modified to consider this difference explicitly; fPhenology values ranged from 0.05 for immature leaves (leaf age <1 month) to 1.2 for mature leaves (leaf age 2–10 months for deciduous and 3–24 months for evergreen leaves). Emission reduction due to leaf senescence is evaluated by decreasing fPhenology value. FBVOC was extracted from the leaf carbon pool in the model, and impacts of released BVOCs on atmospheric chemistry and their climatic feedback were ignored.
2.2.4 Methane emission ()
Methane is a greenhouse gas second to CO2 in importance, but here I focus on methane exchange in terms of the carbon budget. Land-surface CH4 exchange was simulated separately for wetland (Fwetland, source) and upland (Fupland, sink) fractions within each grid cell, as described in Ito and Inatomi (2012):
where fwetland is the wetland fraction within a grid cell. In the wetland fraction, Fwetland was simulated using a mechanistic scheme developed by Walter and Heimann (2000) that uses a multi-layer soil model and simulates gaseous methane emission by physical diffusion, ebullition, and plant-mediated transportation. The same scheme was applied to paddy fields, found mostly in Asia, using seasonal inundation by irrigation. In this study, the top 1 m of soil was divided into 20 layers, and methane gas diffusion was solved numerically with a finite-difference method including the vertical gradient of diffusivity. Microbial methane production occurs below the water table and is sensitive to moisture, temperature, and plant activities (substrate supply). It is assumed to increase exponentially with the temperature, and it stops below the freezing point. Ebullition is assumed to occur when the methane concentration exceeds 500 µmol L−1. Plant-mediated transport depends on the methane concentration gradient between the atmosphere and soil layers and is strongly influenced by plant type and rooting depth. Above the water table, methane oxidation by aerobic soil is calculated as a function of soil temperature and the methane concentration of the air space. In the upland fraction such as forests and grasslands, Fupland is calculated using a semi-mechanistic scheme (Curry, 2007) that calculates methane uptake as a vertical diffusion process affected by soil porosity and microbial activity. The wetland fraction fwetland was derived from the Global Lake and Wetland Dataset (Lehner and Döll, 2004) and was held fixed throughout the simulation period. Temporal variations in the inundation area and water table depth in the wetland fraction are key factors in estimating Fwetland. In this study, seasonal variation in the inundated area was prescribed by satellite data by microwave remote sensing (Prigent et al., 2001), and the temporal variability of water table depth was determined by the water budget estimated by the VISIT model (Ito and Inatomi, 2012). Therefore, interannual variability in inundation area, such as that due to droughts and floods, could have been underrepresented in this study.
2.2.5 Agricultural carbon flows (FAP)
Agricultural practices, including cropping, harvesting, and consumption, are an important component in the global carbon budget (Ciais et al., 2007; Wolf et al., 2015). The VISIT model uses a simplified agriculture scheme, in which global croplands are aggregated, on the basis of physiology and cultivation practices, into three types: C3-plant cropland (e.g., wheat), C4-plant cropland (e.g., maize), and paddy field. The scheme assumes a single-cropping cultivation system in temperate regions, where the growing period is determined by a critical mean monthly temperature of 5 ∘C. In tropical regions (annual mean temperature >20 ∘C), a continuous (nonseasonal) cropping system is assumed in which planting and harvesting occur at constant rates in every month. Irrigation is not explicitly included in the model; instead the water-stress factor for cropland plants is relaxed from its value for natural vegetation. At the start of the growing period, a certain amount of carbon is added to plant biomass pools to represent planting. The crops are harvested when the surface temperature falls below the critical temperature. This study used a single value of 0.45 for the harvest index (fraction of harvested biomass); however, this index varies among crop types and regions, and the uncertainties in this parameter are considered in Sect. 4.5. Residual plant biomass was transferred to the litter pool as agricultural detritus, and this study ignored manure production and consumption processes. Harvested crops were exported from the ecosystem, and the complexities of horizontal food displacement and consumption were also ignored.
2.2.6 Wood harvest (FWH)
Timber harvest by logging in forested lands was evaluated primarily from the LUH dataset (Hurtt et al., 2006), in which the annual wood harvest rate was derived from national data compiled by the United Nations Food and Agricultural Organization. Hurtt et al. (2006) estimated the spatial pattern of wood harvest in each country from land-use data. In this study, regrowth and carbon accumulation of forests after logging was simulated as a recovery of the carbon stock to its previous level of mature forest. As was done for crops, the harvested wood biomass was assumed to be exported from the ecosystem, specifically the stem carbon pool; horizontal transportation to and consumption in other grid cells were ignored. Note that emissions from harvested timber associated with land-use change were evaluated as part of the FLUC term.
2.2.7 Dissolved organic carbon export (FDOC)
Production and consumption of DOC are important processes in terrestrial ecosystems, in terms of soil formation and riverine transport (Nelson et al., 1993). In this study, the VISIT model included a simple scheme of DOC dynamics developed by Grieve (1991) and Boyer et al. (1996), in which the DOC concentration in soil water is determined by the balance of production, decay, and export. The production and decay rates are determined by soil temperature, and the export rate is determined by runoff discharge. In this study, net carbon export by DOC was extracted from the mineral soil pool. Because the VISIT model does not include a river routing scheme, DOC extraction was independently evaluated at each grid cell, and lateral transportation and decay processes were not simulated.
2.2.8 Particulate organic carbon export (FPOC)
Export of POC is assumed to occur mainly in association with soil displacement by water erosion, which can cause soil degradation. The VISIT model incorporates the Revised Universal Soil Loss Equation (Renard et al., 1997) to estimate the rate of soil displacement by water erosion (Ito, 2007). Annual displacement of soil carbon is calculated by
where fC is soil carbon content and R, L, S, K, C, and P are coefficient factors of rainfall, slope length, slope steepness, soil erodibility, vegetation coverage, and conservation practices, respectively, as described in Ito (2007). fC is obtained from the VISIT simulation, and FPOC is extracted from the soil surface litter pool. Although it was developed for management of local croplands, this practical scheme and its derivatives have been used for continental-scale studies (e.g., Yang et al., 2003; Schnitzer et al., 2013; Naipal et al., 2018). Transport of terrestrial carbon to inland waters or the ocean is, however, a complicated process (Berhe et al., 2018); for example, large fractions of displaced soil are redistributed in riverbanks, lake shores, and estuaries. The fate of eroded carbon is assumed to be 20 % in CO2 evasion by decomposition, 60 % in sedimentation, and 20 % in export to lakes and oceans (Lal, 2003; Kirkels et al., 2014). The export fraction is highly uncertain and is discussed further in the parameter uncertainty analysis of Sect. 4.5.
2.3 Simulations and analyses
Global simulations were conducted from 1901 to 2016 at a spatial resolution of in latitude and longitude. The VISIT model was applied to each grid cell, and lateral interactions such as riverine transport, food and timber export, and animal migration were ignored. To obtain the initial stable carbon balance, a spin-up calculation under stationary conditions was conducted for each grid cell for 300 to 3000 years, depending on climate conditions and the biome type. This section describes sensitivity simulations to analyze the impacts of different forcing variables, ensemble perturbation simulations to assess the effect of parameter uncertainty, and several supplementary simulations.
All simulations used climate conditions from CRU TS 3.25 (Harris et al., 2014), consisting of monthly temperature, precipitation, vapor pressure, and cloudiness. The historical change in atmospheric CO2 concentration was taken from observations (e.g., Keeling et al., 2009). The global distribution of natural vegetation was determined by Ramankutty and Foley (1998) for potential vegetation types and Olson et al. (1983) for actual vegetation types. This study classified natural vegetation into 28 types after Olson et al. (1983). Historical land-use status, transitional changes, and wood harvest in each grid cell were derived from the LUH data (Sect. 2.2.1). Until 2005, land-use data were compiled on the basis of statistics and various ancillary data, and after 2006 the data were extended by using an intermediate projection scenario (RCP4.5) produced with an integrated assessment model. The distribution of dominant crop types was determined from the global dataset of Monfreda et al. (2008) and used to calculate FAP and (for paddy field). For the calculation of , the wetland fraction in each grid cell was determined from the GLWD (Global Lake and Wetland Dataset) (Sect. 2.2.4). For the estimation of FPOC, slope factors (L and K) were calculated from the GTOPO30 topography data (https://doi.org/10.5066/F7DF6PQS; USGS, 1996), and the erodibility factor (S) was calculated from soil composition data (Reynolds et al., 1999). Vegetation coverage (C) and conservation practice (P) factors were determined from the dominant natural vegetation and cropland types, also considering the difference in management intensity between developed and developing countries.
This study focused on the carbon budget of terrestrial ecosystems and analyzed the following variables: GPP, RE, NEP, NBP, biomass carbon stock, and soil carbon stock. The mean residence time (MRT) of the biomass, soil, and total ecosystem carbon stocks at transitional states were approximately calculated in a similar manner to Carvalhais et al. (2014):
where flux is net primary production (NPP) for biomass (= GPP − RA), RH for soil, and the sum of these fluxes (NPP + RH) for the total ecosystem carbon stock.
2.3.1 Sensitivity simulations
To evaluate and separate the effects of MCFs, 12 simulation experiments were conducted:
-
EX0: no MCF was included, and the terrestrial carbon budget was determined by GPP, RA, and RH, such that NBP was identical to NEP.
-
EXLUC: only FLUC was added to EX0.
-
EXBB: only FBB was added to EX0.
-
EXBVOC: only FBVOC was added to EX0.
-
EX: only was added to EX0.
-
EXAP: only FAP was added to EX0.
-
EXWH: only FWH was added to EX0.
-
EXDOC: only FDOC was added to EX0.
-
EXPOC: only FPOC was added to EX0.
-
EXALL: all eight MCFs were considered, equivalent to the baseline simulation.
-
EXBGC: biogeochemical flows (FBVOC, , FDOC, and FPOC) were added to EX0.
-
EXATP: anthropogenic (human-dominated) flows (FLUC, FBB, FAP, and FHW) were added to EX0.
The differences between EX0 and the next eight simulations indicate the effects of individual MCFs, and the difference between EXALL and EX0 shows the combined effect of these MCFs. Interactions among the MCFs through changes in the terrestrial carbon stock may mean that their effects are not linearly additive. For example, land-use changes have indirect impacts on biomass burning, BVOC emission, and water erosion (e.g., Nadeu et al., 2015). Also, the inclusion of the MCFs affects the major flows of primary production and respiration. For example, BVOC emission reduces the carbon stored in leaves, which leads to reductions in light absorption and GPP. In croplands, planting and harvest substantially influence GPP and respiration. The last two simulations (EXBGC and EXATP) sought to evaluate the relative contributions of what are conventionally considered biogeochemical and human-affected processes.
2.3.2 Parameter-ensemble simulations
Large uncertainties remain in the estimates for each MCF and its impacts. These uncertainties can emerge among different models, forcing data, and parameters, and evaluating them is important but difficult. The schemes used in this study include empirical formulations and parameters, some of which are not well constrained by observational data. Upscaling locally adapted schemes and parameters can lead to biased results at the global scale. To characterize the range of uncertainty caused by poorly determined parameters, I conducted a set of ensemble simulations, based on EXALL, in which the values of the following representative parameters of the eight MCFs were randomly perturbated at the same time: annual deforestation rate in FLUC, biomass burning emission factors in FBB, BVOC emission factors in FBVOC, wood harvest rate in FWH, crop harvest index in FAP, methane production and oxidation potentials in , DOC export rate in FDOC, and erodibility and land-export fraction in FPOC. It should be noted here that other parameters have their own uncertainties and that this study focused on these eight representative parameters for explanatory purposes. Also, these uncertainties may increase as they incorporate the differences among models with differing structures and assumptions. A total of 146 ensemble simulations were conducted (Fig. S2) in which these parameters were perturbed by randomly selecting values from the Gaussian distribution within the range of ±30 %. All other configurations were those of EXALL. Means, medians, and 95 % confidence intervals were calculated from the 146 resulting terrestrial carbon budgets.
2.3.3 Supplementary simulations
To further investigate the characteristics and influence of MCFs, five supplementary simulations were conducted. In the first, based on the protocol of EXALL, land-use status was held fixed at its initial state in 1901 (EXfxLUC). This simulation differs from EXLUC by also removing the effects of land-use change on FAP and FPOC from alterations in cropland area. In the second, the climate condition was held fixed at its initial state in 1901 (EXfxCL). This simulation removed the effect of temperature and precipitation changes on MCFs and the terrestrial carbon budget. Many carbon flows, including the major ones (GPP, RA, and RH) as well as minor ones (FBB, FBVOC, , FAP, FDOC, and FPOC), are more or less influenced by climate conditions. In the third simulation, atmospheric CO2 concentration was held fixed at its level in 1901 (EX). Although no MCFs are directly sensitive to ambient CO2 conditions, the fertilization effect of rising CO2 concentration affects GPP and related carbon dynamics, including MCFs.
The fourth and fifth simulations focused on biomass burning. As explained earlier, the fire scheme in the VISIT model does not explicitly consider human activities such as prescribed fires and fire prevention, probably leading to biases in burned area and subsequent emission patterns. For example, the fire scheme poorly captures the recent declining trend in burned area (Andela et al., 2017) due to human suppression. These two simulations used satellite remote-sensing data to evaluate the effect of model-estimated burned area. In the fourth simulation, based on EXALL, interannual variability in burned area was prescribed by the Global Fire Emission Database 4s (GFED4s) remote-sensing product (Randerson et al., 2012) during the period 1998–2016 (EXBB1). In the fifth simulation (EXBB2), the simulated mean burned area for 1901–2016 was adjusted with respect to GFED4s. For example, if the control run (EXALL) had estimated burned areas that averaged 20 % higher than GFED4s, an adjustment coefficient of 100∕120 would have been applied to the burned area simulated in this run to remove the systematic overestimation.
3.1 Global terrestrial carbon budgets
The mean annual global terrestrial GPP in 1990–2013 (a period when comparative estimates were available) was simulated as 144.0±4.4 Pg C yr−1 in EX0 and 125.4±4.0 Pg C yr−1 in EXALL (mean ± standard deviation of interannual variability). Ecosystem respiration (RE) was simulated as 141.0±3.6 Pg C yr−1 in EX0 and 118.8±3.2 Pg C yr−1 in EXALL. Mean vegetation and soil carbon storage differed in the two simulations: EX0 produced 648 Pg C in vegetation and 1560 Pg C in soil organic matter, and EXALL produced 477 Pg C in vegetation and 1290 Pg C in soil organic matter. The mean annual net CO2 budget determined by the major flows, NEP (= GPP − RE), was simulated as 2.99±1.18 Pg C yr−1 in EX0 (which ignores MCFs) and 6.57±1.07 Pg C yr−1 in EXALL. Because both simulations used the same climate, atmospheric CO2, and land-use data, these differences – lower carbon stocks, smaller GPP and RE flows, and a large sink by NEP – are attributable to the inclusion of the MCFs.
The individual MCFs had different impacts on the global terrestrial carbon budget. For the vegetation carbon stock, impacts were negligible (<1 Pg C) from methane emission, DOC and POC exports by water movement, and agricultural practices, whereas impacts were substantial from land-use change (−88.5 Pg C), biomass burning (−46.4 Pg C), wood harvest (−28.5 Pg C), and BVOC emission (−24.2 Pg C). For the soil carbon stock, the two largest negative impacts were from land-use change (−108 Pg C) and biomass burning (−71.2 Pg C). Interestingly, the inclusion of BVOC emission reduced the soil carbon stock (−18.1 Pg C) through the loss of photosynthate carbon and decreased carbon supply to the soil. The inclusion of agricultural carbon flows (planting and harvesting, other than land-use change) decreased the soil carbon stock (−55.6 Pg C), although planting enhanced vegetation productivity and carbon supply to the soil. The inclusion of DOC and POC exports moderately reduced the soil carbon stock (−5.9 and −3.6 Pg C, respectively).
Most of the difference in GPP between EX0 and EXALL was attributable to land-use change (−12.8 Pg C yr−1), wood harvest (−0.9 Pg C yr−1), and BVOC emission (−0.9 Pg C yr−1). Biomass burning, though it has substantial impacts on biomass, also slightly decreased GPP (−0.75 Pg C yr−1). The simulated impacts of MCFs on RE were mostly similar to those for GPP. The relatively high NEP in EXALL was largely attributable to compensatory regrowth in response to biomass burning (2.03 Pg C yr−1), BVOC emission (0.69 Pg C yr−1), and wood harvest (0.41 Pg C yr−1).
Human activities (EXATP) had greater impacts on terrestrial carbon stocks than biogeochemical processes (EXBGC), as mean ecosystem carbon stock decreased by 172 Pg C in EXBGC and 296 Pg C in EXATP. The sum of these two depressions in carbon stock, 467 Pg C, was larger than that estimated in the all-inclusive experiment (EXALL), 440 Pg C, which points to nonlinear offsetting effects among the MCFs.
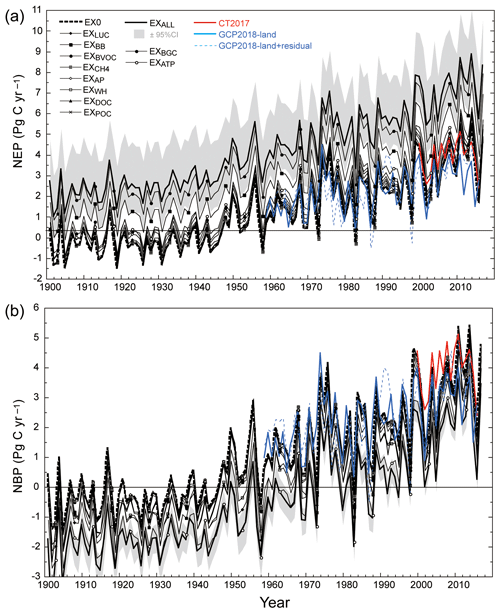
Figure 2Temporal changes in the simulated global terrestrial carbon budget from this study (black lines), CarbonTracker 2017 (CT2017; Peters et al., 2007; red lines), and the Global Carbon Project (GCP; blue lines). (a) NEP and (b) NBP. See the text for the simulation experiments. Figure S3 presents extracted results for the period 1980–2016.
The carbon budget including the MCFs (NBP) in 1990–2013 was estimated as 1.36±1.12 Pg C yr−1 of net sink in EXALL, that is, 20.7 % of NEP (see Table 1 for decadal summary). Figure 2 shows the temporal change in global annual NEPs and NBPs in each experiment for the 1901–2016 study period (see Fig. S3 for details of the 1990–2013 period). The inclusion of MCFs considerably altered the mean state of the terrestrial carbon budget throughout the simulation period. Little difference was found among the experiments in interannual variability and decadal trends. For example, linear trends of NBP in 1980–2013 were estimated as (0.0783 Pg C yr−1) yr−1 in EX0 and (0.0890 Pg C yr−1) yr−1 in EXALL. Interestingly, the larger differences among experiments for NEP (±1.15 Pg C yr−1, standard deviation among EX0 to EXALL) than for NBP (±0.52 Pg C yr−1) indicated a convergence of estimated carbon budgets after including MCFs.
Table 1Decadal summary of simulation results of net global terrestrial carbon budget (Pg C yr−1).
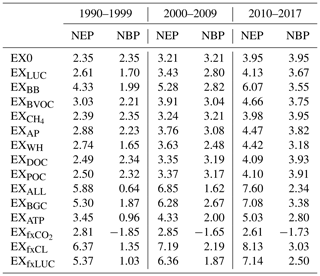
NEP, net ecosystem production; NBP, net biome production. Model designations are defined in the text.
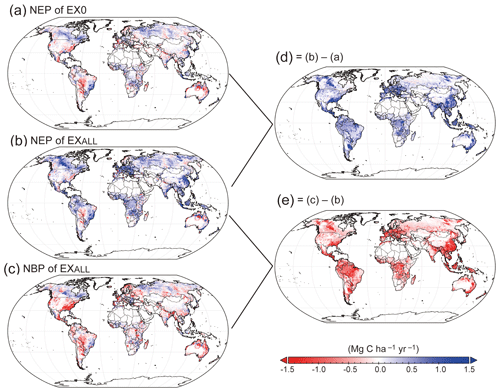
Figure 3Global distribution of simulated terrestrial carbon budget in the 2000s. (a) NEP in EX0, (b) NEP in EXALL, (c) NBP in EXALL, (d) difference between panels (b) and (a) showing the apparent effects of MCFs on NEP, and (e) difference between panels (c) and (b) showing the apparent effects of MCFs on NBP.
The spatial distribution of carbon budgets shows that EX0 identified a vast area of tropical, temperate, and boreal forests as moderate net carbon sinks (Fig. 3a). The inclusion of MCFs in EXALL (Fig. 3b) intensified this net sink in tropical forests and parts of the temperate and boreal forests, but it decreased NEP in grasslands and pastures in central North America and Europe, turning parts of them into net carbon sources (Fig. 3d). The spatial distribution of NBP in EXALL (Fig. 3c) was a heterogeneous pattern of sink and source. Several tropical and subtropical forests had negative NBP, although NEP in these areas was estimated as positive or neutral. As shown in Fig. 3e, with the addition of MCFs, a large part of the terrestrial ecosystem was simulated to lose carbon. The contributions of each flow are described in the next section.
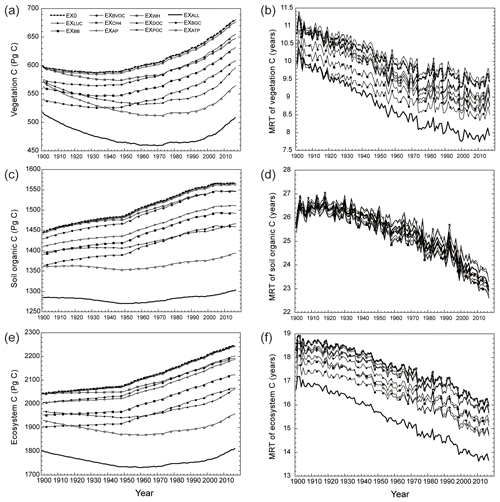
Figure 4Time series of simulated carbon stocks and their mean residence time (MRT) in different experiments. (a) Vegetation biomass and (b) its MRT, (c) soil organic carbon and (d) its MRT, and (e) total ecosystem carbon stock and (f) its MRT.
The decrease in carbon stocks in terrestrial ecosystems after the addition of MCFs indicates that the mean residence time (MRT) of these stocks became shorter than would be estimated solely from major carbon flows (see Fig. S4 for the spatial distribution of stocks and MRTs). As shown in Fig. 4, simulated terrestrial carbon stocks in EXALL were steady or slightly declining until around 1960, especially when land-use change (e.g., tropical deforestation) was included. After 1960, carbon stocks in vegetation and soil began to gradually increase. As described earlier, the simulated carbon stocks differed among the experiments by as much as 440 Pg C as a consequence of including MCFs. Also, the inclusion of MCFs made large impacts on GPP and RE (Fig. S5) by altering vegetation structure and soil carbon storage. Simulated MRTs grew clearly shorter (i.e., turnover was accelerated), as a result of global changes such as temperature rise enhancing respiratory emissions. Note that MRTs also grew shorter in the result of EX0, which ignored MCFs, but including the MCFs increased the difference in MRT among the experiments. For example, the difference in MRT of vegetation biomass between EX0 and EXALL grew from 0.89 years in the 1900s to 1.54 years in the 2000s, and the difference for soil carbon stock grew from 0.10 years in the 1900s to 0.24 years in the 2000s. The definition of MRT (Eq. 7) means that shortened MRTs could result from increases in NPP and respiration.
3.2 Simulated patterns of MCFs
Figure 5 shows the temporal changes in the eight simulated MCFs in their individual sensitivity simulations (EXLUC to EXPOC) as well as the EXALL simulation. The emissions associated with land-use change (FLUC) peaked around the 1950s at 1.2–1.4 Pg C yr−1 and then gradually decreased. Biomass burning emission (FBB) remained around 1 Pg C yr−1 until the 1970s and then increased slightly to 1.5 Pg C yr−1, with a large interannual variability. BVOC emission (FBVOC) increased gradually from 0.5 Pg C yr−1 in the early 20th century to 0.6 Pg C yr−1 in the 21st century. Methane emission () gradually increased from 0.11 Pg C yr−1 in the first decades of the 1900s to 0.13 Pg C yr−1 in the 2000s (representing 160–170 Tg CH4 yr−1). Wood harvest (FWH) likewise increased from 0.5 Pg C yr−1 in the 1900s to 1.1 Pg C yr−1 in the 2000s, as did POC export by water erosion (FPOC), which increased from 0.55 Pg C yr−1 in the 1900s to 0.95 Pg C yr−1 in the 2000s. Crop planting and harvest (FAP) had a mixed effect on the terrestrial carbon budget because planting enhances productivity, whereas harvesting is a direct carbon loss. As a result, FAP had both negative (net uptake) and positive (net emission) values. DOC export (FDOC) remained steady at around 0.14±0.004 Pg C yr−1 throughout the simulation period.
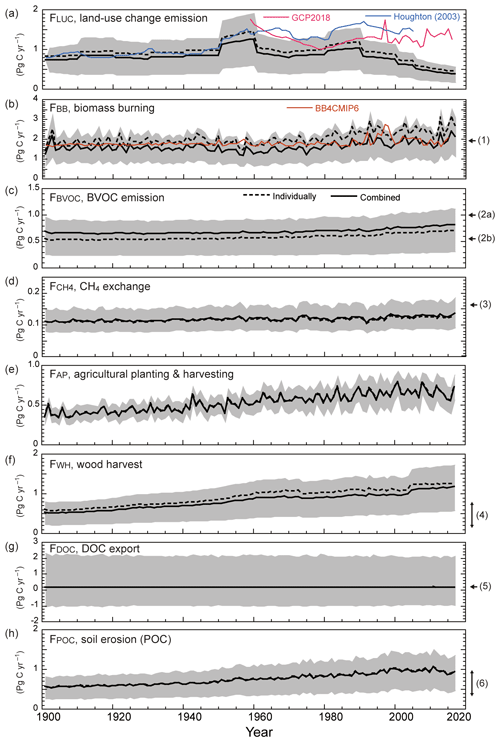
Figure 5Time series of minor carbon flows simulated by the VISIT model and previous studies. Dashed lines are results of individually simulated flows, and solid lines are results of the EXALL simulated, and shading shows the 95 % confidence interval for the EXALL result obtained from ensemble simulations (Fig. S2). Blue and red lines in panel (a) show data of the Global Carbon Project (GCP2018) and Houghton (2003). The orange line in panel (b) shows data of BB4CMIP6 (van Marle et al., 2017). Arrows indicate the values of (1) biomass burning emission by Randerson et al. (2012), (2a) total BVOC and (2b) isoprene emissions by Guenther et al. (2012), (3) wetland and paddy methane emission by Saunois et al. (2017), (4) wood harvest by Arneth et al. (2017), (5) DOC export by Dai et al. (2012), and (6) soil erosion by Chappell et al. (2016).
The supplementary simulations showed that temporal changes in the MCFs were caused by different forcing factors. For example, when the atmospheric CO2 concentration was fixed at its level in 1901 (EX, data not shown), the increasing trend in FBVOC (Fig. 5c) nearly vanished, whereas other flows such as FWH and FPOC were insensitive to CO2. When climate conditions were held fixed (EXfxCL), FBB showed only a decadal trend in response to changes in fuel load, and climate-induced interannual variability in burned area and fire-induced emissions (Fig. 5b) disappeared.
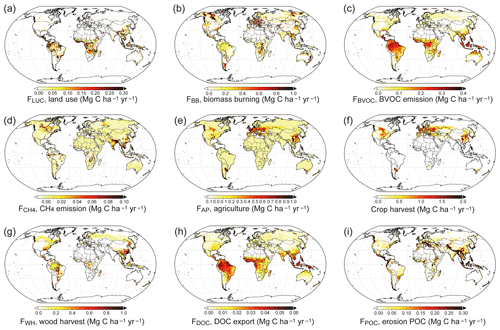
Figure 6Global distribution of the simulated MCFs (plus crop harvest) in 2000–2009. Results of EXALL are shown.
The MCFs considered in this study showed distinct spatial patterns (Fig. 6). FLUC occurred mainly in the tropical forests of South America, Africa, and South Asia. FBB occurred in subtropical areas in Africa, tropical forests in South America and Southeast Asia, the Mediterranean area, and boreal forests in North America and east Siberia. FBVOC was highest in tropical forests and elevated in other forested areas. For , major sources included monsoon-affected parts of Asia dominated by paddy fields, tropical wetlands including floodplains of big rivers, and northern wetlands, whereas other uplands were weak sinks. For FAP, croplands in Europe, East Asia, and North America exported large amounts of carbon (see Fig. 6f for the crop harvesting effect alone). FWH occurred mainly in tropical forests in southern East Asia, South America, and southern North America. FPOC occurred mainly in humid and steep areas such as mountainous regions of monsoon Asia and cultivated areas. FDOC occurred mainly in warm and humid areas such as tropical forests in South America, Africa, and Southeast Asia.
3.3 Effects of MCFs on the carbon budget
The effects of the eight studied MCFs on the global carbon budget, resulting in a lower net sink by NBP than by NEP, were dominated by five MCFs: biomass burning (FBB), wood harvest (FWH), POC export by water erosion (FPOC), BVOC emission (FBVOC), and emission caused by land-use change (FLUC) (Fig. 7a). The contributions of MCFs differed among regions. FAP and FBB had dominant effects in Europe (Fig. 7b) and North America (Fig. 7g), where the effects of FLUC and FDOC were negligible. In Africa (Fig. 7c), South America (Fig. 7h), and the global tropics (Fig. 7i), all five MCFs had similar effects. In Asia (Fig. 7e), FPOC and FAP had the largest effects, and emissions from the vast area of paddy fields were considerable. In semiarid regions (Fig. 7j), FAP and FBB were the largest.
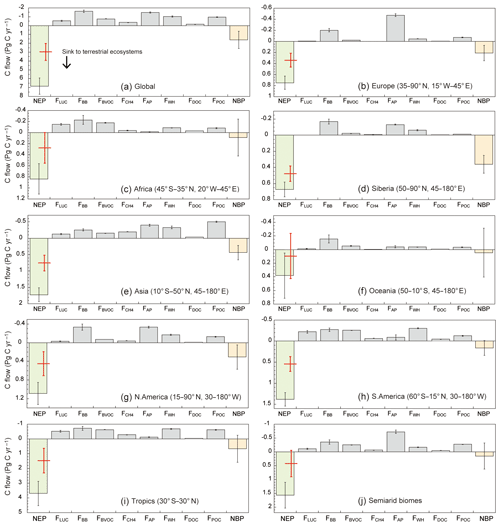
Figure 7Regional portions of the terrestrial carbon budget in 2000–2009. Columns show the mean results of EXALL and error bars show the standard deviation of interannual variability. Red lines show the mean and standard deviation of NEP in EX0. Note the differences in vertical scale.
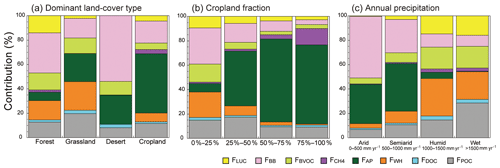
Figure 8Relative contribution of MCFs to the terrestrial carbon budget simulated by EXALL in 2000–2009: (a) aggregated by dominant land-cover type, (b) aggregated by cropland fraction within grid cells, and (c) aggregated by annual precipitation.
Certain spatial tendencies become clearer in a global aggregation of the simulated results (Fig. 8) related to the dominant land-cover type in each grid cell, the cropland fraction, and aridity represented by annual precipitation. In forest-dominated grid cells (Fig. 8a), FBB made the largest (>30 %) contribution, followed by FWH, FBVOC, and FLUC, and in cropland-dominated cells, about half of the influence of MCFs was due to agricultural practices (FAP). Because grassland-dominated cells contain fractions of woodland and cropland, FAP and FWH as well as FPOC made contributions in these cells. In desert-dominated cells, FBB made up the majority of the contributions. In cells with small fractions of cropland including tropical forests (Fig. 8b), FWH, FBB, and FBVOC made strong contributions, whereas in cells dominated by crops, made a substantial contribution reflecting the vast area of paddy fields in Asia. FPOC made large contributions at all cultivation intensities, but particularly in moderately cultivated areas. An analysis based on precipitation was also informative (Fig. 8c). In arid areas (annual precipitation <500 mm), FBB had the largest impacts, as expected from the dominance of fire-prone ecosystems such as boreal forests and subtropical woodlands. In wet areas (precipitation >1500 mm), FLUC and FPOC made large contributions, and FBB had a minor effect. The influence of FWH was strongest in moderately humid to wet areas.
4.1 Comparison with previous carbon studies
This study showed that MCFs have notable impacts on the terrestrial carbon budget; they disequilibrate ecosystem carbon stocks and affect MRTs. Most of the simulated magnitudes of MCFs were comparable to results of previous studies (Fig. 5 and Table 2), and their impacts on the carbon budget were consistent with other model studies (e.g., Yue et al., 2015; Naipal et al., 2018). In terms of FLUC, the model estimated clearly lower emissions than the Global Carbon Project (GCP) synthesis (Le Quéré et al., 2018) and other studies, surely because this study did not use actual land-use data after 2005. Updated data would likely improve the VISIT model's performance. The fact that the simulated FBB was slightly low compared to previous estimates implies that there is a need to refine the fire module in the model (discussed further in Sect. 4.5). The simulated FPOC was comparable to results in other studies, but there remain inconsistencies in the fate terms (riverine transport, burial, and CO2 evasion) and the ratio of ocean and inland water export. Similarly, the simulated FAP and FWH appear comparable to results in other studies, but this study largely ignored their transport and consumption. Further detailed comparisons and comprehensive assessments are clearly required.
Most models have been calibrated and validated with observational data of major carbon flows (e.g., GPP, RE, and NEP) and carbon stocks. Although recent models have begun to take account of land-use change and biomass burning, most still ignore the contributions of many other minor flows. The global GPP simulated in this study is similar to a satellite-based product of the Breathing Earth System Simulator (BESS) of Jiang and Ryu (2016): for the 2001–2013 period, the coefficient of determination (R2) was 0.77 for EX0 and 0.71 for EXALL (Fig. S5). All three simulations show increasing trends. In contrast, the upscaled flux measurement data of FLUXCOM (Tramontana et al., 2016) and the MOD15 satellite product (Zhao et al., 2006) show smaller interannual variability and trends, and they were only weakly correlated with the VISIT simulations (R2=0.21–0.39). Compared with the terrestrial carbon budget in the integrated synthesis of the GCP for 1959–2016 (Le Quéré et al., 2018), the simulated NEP in EXALL was much higher in the same period: 5.7 Pg C yr−1 in EXALL and 2.1 Pg C yr−1 in GCP. Removing the land-use emission of 1.3 Pg C yr−1 would reduce the provisional NBP from GCP to 0.85 Pg C yr−1, putting it closer to the simulated NBP in EXALL (0.68 Pg C yr−1) than to the NBP in EX0 (2.33 Pg C yr−1). (Figures S6 and S7 compare the results of NEP and FLUC from the individual models in the GCP synthesis.) EXALL successfully captured the large aboveground vegetation biomass stock in the tropics and the small stock in boreal zones seen in observations (Fig. S8a). A similar comparison of soil carbon (Fig. S8b) also indicates the model's ability to capture the spatial gradient in this stock; an overestimation in the northern midlatitudes (around 30∘ N) is attributable to high soil carbon accumulation in the Tibetan Plateau simulated by the model in frigid regions. It is not clear, however, whether EXALL (with MCFs) captured the global patterns with greater accuracy than EX0 (without MCFs), because observational datasets show considerable discrepancies, and the differences between the model simulations were relatively small. The estimated MRT of the ecosystem carbon stock in EXALL (14–17 years) was shorter than the MRT of 23 years (95 % confidence interval, 18–29 years) found by the data-oriented study of Carvalhais et al. (2014). This difference is attributable to the high soil carbon stock in the latter study (2397 Pg C) rather than to differences in the vegetation carbon stock and flows; both studies had similar spatial patterns of MRT.
Considering the remaining uncertainties in observational terrestrial carbon accounting, it is still difficult to perform a conclusive validation. Nevertheless, this study demonstrated the possibility of integrating various carbon flows into a single model framework.
4.2 Impacts of MCFs on regional and global carbon budgets
The simulated MCFs affect the amount of the terrestrial carbon stock by as much as 440 Pg C. The size of this difference is comparable to differences, or the model estimation uncertainty, found among biome models (e.g., Friend et al., 2014; Tian et al., 2015). By definition, NBP including the effect of MCFs is likely to correspond closely to carbon stock change as well as carbon budgets obtained by atmospheric inversions. MCFs affect the carbon budget in two major ways: first by their instantaneous carbon exports and second by the ensuing carbon uptake during recovery from these disturbances, which occurs with time lags of decadal to centennial scale, depending on the types of disturbance and their intensities (e.g., Fu et al., 2017). Assessments of MCFs would help characterize the “missing sink”, which is now primarily ascribed to terrestrial carbon uptake (Houghton et al., 1998; Le Quéré et al., 2018) by mechanisms that are still arguable. Although previous studies (e.g., Jung et al., 2011; Zscheischler et al., 2017) have noted the potential importance of MCFs and the difference between NEP and NBP (or corresponding metrics such as the net ecosystem carbon balance of Chapin et al., 2006), these issues have not been comprehensively evaluated by global and regional carbon syntheses, such as the REgional Carbon Cycle Assessment and Processes (RECCAP; Sitch et al., 2015). Indeed, biome models used to simulate the terrestrial carbon cycle in RECCAP differ in how they parameterize the MCFs, and their estimations of net budget are not easily compared.
In the VISIT model simulation, interannual variability of NBP and NEP were closely correlated (Fig. S9), although several MCFs such as FBB and did not share in that correlation. These interannual variations were largely determined by the major flows, except for extreme events such as huge fires in 1997 and 2015 (e.g., Huijnen et al., 2016). Therefore, establishing an empirical model may make it possible to approximately estimate NBP from NEP. To evaluate the similarities and differences between these two quantities, further observation data are required for each flow and its determinant processes.
This study demonstrated that the VISIT modeling approach is effective in integrating the major and minor carbon flows into a single framework and obtaining a consistent carbon budget, although this approach has its own uncertainties and biases, as shown by benchmarking and intercomparison studies (e.g., Arneth et al., 2017; Huntzinger et al., 2017). Biogeochemical models like VISIT have advantages in reconciling inconsistencies, filling gaps, and specifying underlying mechanisms, as well as reconstructing historical changes and making future projections. Intimate collaborations between modeling and observational studies (Sitch et al., 2015; Schimel et al., 2015) should lead to more reliable carbon accounting.
4.3 Ancillary impacts on hydrology
This study focused on the terrestrial carbon budget, but the MCFs also affect the hydrological properties of land systems. As shown in Fig. S10, land-use change, biomass burning, and BVOC emission lead to a loss of vegetation leaf area, except in croplands. The loss in turn decreases evapotranspiration and increases runoff discharge regionally by as much as 20 mm yr−1. In the simulation, runoff discharge increased through time, more steeply in EXALL than in EX0. This effect was evident in many tropical to temperate regions, implying the importance of a comprehensive understanding of carbon–water interactions.
However, it should be noted that the actual impacts of MCFs on land systems can be much more complicated than assumed here. For example, loss of soil organic carbon by biomass burning and water erosion may decrease the water-holding capacity of soils, leading to higher runoff discharge and lower tolerance to droughts. Also, several MCFs should change along with translocations and biogeochemical reactions of nutrients such as nitrogen and phosphorus, followed by changes in vegetation productivity and water use. To fully include these processes in the model, a comprehensive understanding of biogeochemistry and ecohydrology is required.
4.4 Complexities of MCF accounting
Although this study incorporated some of the known MCFs, fully or partially, others are unrecognized or assumed to be negligible. Indeed, many studies have investigated MCFs that were not included in this and most previous carbon cycle studies (Table 3). Few studies have taken comprehensive account of all carbon flows. For example, for lack of parameterization data, this study did not explicitly consider carbon sequestration in pyrogenic organic matter or charcoal (e.g., Santín et al., 2015), in phytoliths (Song et al., 2017), or by means of abiotic geochemical processes (Schlesinger, 2017). This study tried to include the effects of DOC and POC exports and obtained results comparable to other studies (e.g., Dai et al., 2012; Galy et al., 2015; Chappell et al., 2016). However, this study did not explicitly consider lateral displacement of carbon between adjacent grid cells and associated emissions, such as river transport and international commerce (e.g., Battin et al., 2009; Bastviken et al., 2011; Peters et al., 2012), and reservoir effects on riverine transport (e.g., Mendonça et al., 2017). In this regard, modeling of agricultural practices should be improved to obtain more reliable regional carbon budgets. It is particularly important to evaluate efforts to enhance harvest index and to raise carbon sequestration into cropland soils, as proposed by the “4 per 1000” initiative (Dignac et al., 2017; Minasny et al., 2017).
More clarity is needed in the parameterization of disturbances. This study considered the impacts of wildfires and land-use conversion, but in a conventional manner, possibly leading to biased results (see Sect. 4.5 for biomass burning). Other potentially influential disturbances, such as pest outbreaks and drought-induced dieback associated with climate extremes, were not explicitly considered, although they can perturb ecosystem carbon budgets (Reichstein et al., 2013). In the long term, ecosystem degradation induced by forest fragmentation, overgrazing, and soil loss by wind erosion can further affect carbon budgets (e.g., Paustian et al., 2016; Brinck et al., 2017). Integration of these processes awaits future studies.
4.5 Uncertainties and possibility of constraints
This study is an early attempt to evaluate the effects of various MCFs. The results have convinced me that changes in MCFs will have considerable influences on the global carbon budget (e.g., Piao et al., 2018; Lal, 2019; Pugh et al., 2019), and more such attempts are required to improve our understanding of the global carbon cycle, which plays a critical role in future climate projections. However, given the imperfect state of knowledge about these MCFs, their inclusion can introduce other errors and biases. I took the estimation uncertainty into account by perturbing representative parameters, but this study did not examine other sources of uncertainties such as differences among ecosystem models and forcing data. Indeed, many ecosystem models have been developed with different degrees of complexity (e.g., dynamic global vegetation models), and intercomparison studies have shown that existing ecosystem models differ widely in their environmental responsiveness to changes in major carbon flows (e.g., Friend et al., 2014; Huntzinger et al., 2017). For example, the models differ in global GPP by more than 30 %, even though the processes contributing to primary production are well understood and increasingly constrained by observations (Anav et al., 2015). This single-model study was necessarily limited in searching the full range of estimation uncertainty, and further studies using multiple MCF-implemented models are highly desirable.
Considering the shortcomings of broad-scale and long-term observations of MCFs, estimation uncertainties could be larger than presently thought. For example, each of the coefficient factors of the erosion scheme (Eq. 6) can be expected to have large ranges of uncertainty, and few data are available to constrain for the fate of laterally transported POC and DOC. Data related to land-use changes (e.g., gross vs. net land-use transition) and procedures to implement them in models are not standardized (e.g., Fuchs et al., 2015). One exception is that multiple satellites have produced long global records of biomass burning. Indeed, a comparison of FBB in the VISIT model simulation and these observations clearly shows a problem in this study (Fig. 5b): the VISIT model systematically underestimated fire-induced CO2 emission in most years relative to the BB4CMIP6 multi-satellite (combined with proxies) product of biomass burning (van Marle et al., 2017). It also showed an increasing trend of fire activity after 1998, a trend inconsistent with a recent analysis of global burned area (Andela et al., 2017) that showed a declining trend of burned area due to human activities such as agricultural expansion and intensification.
To evaluate the bias caused by this inconsistency, a simulation was conducted (EXBB1) in which interannual anomalies of burned area were prescribed by the GFED4s satellite product in 1998–2016 (Fig. S11, green line). As a result, the model-simulated FBB showed a decreasing trend, implying that prognostic modeling of fire regimes is problematic. Additionally, the high fire-induced emission in 1998, a strong El Niño year, was appropriately captured. The model, however, was likely to overestimate average burned area (561×106 ha yr−1) relative to satellite-based estimates. Therefore, another simulation was conducted (EXBB2) in which not only anomalous but also average burned area were prescribed by GFED4s. That simulation (Fig. S11, orange line) yielded an even lower FBB resulting from a smaller burned area (437×106 ha yr−1), although its interannual variability was little changed. The low FBB despite a large burned area indicates that fire intensity or emission factors in the model were not properly determined. Such estimation biases and uncertainties can remain in other carbon flows and should be clarified and reduced using observational data.
4.6 Implications for observations
This study has implications not only for improving models, but also for strategic observations of the carbon cycle. MCFs may account for much or all of the discrepancy among top–down atmospheric inversions, CO2 flux measurements, and bottom–up biometric carbon stock surveys (e.g., Jung et al., 2011; Kondo et al., 2015; Takata et al., 2017). Furthermore, investigations of MCFs may help reveal the mechanisms underlying the apparent net carbon sequestration by mature forests (Luyssaert et al., 2008), as observed in CO2 flux measurements and biometric surveys. Major carbon flows (GPP, RE, and NEP) have been observed using the standardized FLUXNET method at many flux measurement sites (Baldocchi et al., 2001). These observations have given us an overview of the terrestrial carbon budget and its tendencies (e.g., Jung et al., 2017). Recent satellite observations allow us to monitor vegetation coverage and biomass globally at fine spatial resolutions (e.g., Saatchi et al., 2011; Baccini et al., 2017). Nevertheless, it is still difficult to directly observe some MCFs, including non-CO2 trace gases, disturbance-induced non-periodic emissions, and subsurface transport and sequestration. For example, flux measurements of BVOC emissions are technically challenging (Guenther et al., 1996; Geron et al., 2016) because of the low concentrations of BVOC compounds, their wide variety, and their spatial and temporal heterogeneity. Quantification of DOC and POC dynamics at the landscape scale appears to require intensive observation networks (e.g., Dai et al., 2012; Raymond et al., 2013). Emissions associated with land-use change, which have attracted much attention from researchers, still have large uncertainties (Houghton and Nassikas, 2017; Erb et al., 2018). Further integrated studies of ground-based, airborne, and satellite observations of carbon flows are necessary that include minor flows, pools, and relevant properties (e.g., isotope ratios). The spatial and temporal patterns of influential MCFs obtained in this study will be useful for planning effective observational strategies.
Simulation code and data are available on request from the author. The CRU TS3.25 dataset was from the Climate Research Unit, University of East Anglia (refer to https://crudata.uea.ac.uk/cru/data/hrg/; CRU, 2019). The land-use dataset was from the University of Maryland (http://luh.umd.edu/data.shtml; Hurtt et al., 2006). The Global Lake and Wetland Database was from the World Wildlife Fund (https://www.worldwildlife.org/pages/global-lakes-and-wetlands-database; Lehner and Döll, 2004).
The supplement related to this article is available online at: https://doi.org/10.5194/esd-10-685-2019-supplement.
The author declares that there is no conflict of interest.
This article is part of the special issue “The 10th International Carbon Dioxide Conference (ICDC10) and the 19th WMO/IAEA Meeting on Carbon Dioxide, other Greenhouse Gases and Related Measurement Techniques (GGMT-2017) (AMT/ACP/BG/CP/ESD inter-journal SI)”. It is a result of the 10th International Carbon Dioxide Conference, Interlaken, Switzerland, 21–25 August 2017.
This research has been supported by the KAKENHI grant (no. 17H01867) of the Japan Society for the Promotion of Science and Environmental Research Fund (2-1710) of the Ministry of the Environment, Japan, and the Environmental Restoration and Conservation Agency.
This paper was edited by Ning Zeng and reviewed by two anonymous referees.
Adachi, M., Ito, A., Ishida, A., Kadir, W. R., Ladpala, P., and Yamagata, Y.: Carbon budget of tropical forests in Southeast Asia and the effects of deforestation: an approach using a process-based model and field measurements, Biogeosciences, 8, 2635–2647, https://doi.org/10.5194/bg-8-2635-2011, 2011.
Anav, A., Friedlingstein, P., Beer, C., Ciais, P., Harper, A., Jones, C., Murray-Tortarolo, G., Papale, D., Parazoo, N. C., Peylin, P., Piao, S., Sitch, S., Viovy, N., Wiltshire, A., and Zhao, M.: Spatio-temporal patterns of terrestrial gross primary production: A review, Rev. Geophys., 53, 785–818, https://doi.org/10.1002/2015RG000483, 2015.
Andela, N., Morton, D. C., Giglio, L., Chen, Y., van der Werf, G. R., Kasibhatla, P. S., DeFries, R. S., Collatz, G. J., Hantson, S., Kloster, S., Bachelet, D., Forrest, M., Lasslop, G., Li, F., Mangeon, S., Melton, J. R., Yue, C., and Randerson, J. T.: A human-driven decline in global burned area, Science, 356, 1356–1362, https://doi.org/10.1126/science.aal4108, 2017.
Arneth, A., Sitch, S., Pongratz, J., Stocker, B. D., Ciais, P., Poulter, B., Bayer, A. D., Bondeau, A., Calle, L., Chini, L. P., Gasser, T., Fader, M., Friedlingstein, P., Kato, E., Li, W., Lindeskog, M., Nabel, J. E. M. S., Pugh, T. A. M., Robertson, E., Viovy, N., Yue, C., and Zaehle, S.: Historical carbon dioxide emissions caused by land-use changes are possibly larger than assumed, Nat. Geosci., 10, 79–84, https://doi.org/10.1038/NGEO2882, 2017.
Baccini, A., Walker, W., Carvalho, I., Farina, M., Sulla-Menashe, D., and Houghton, R. A.: Tropical forests are a net carbon source based on aboveground measurements of gain and loss, Science, 358, 230–234, https://doi.org/10.1126/science.aam5962, 2017.
Baldocchi, D., Falge, E., Gu, L., Olson, R., Hollinger, D., Running, S., Anthoni, P., Bernhofer, C., Davis, K., Evans, R., Fuentes, J., Goldstein, A., Katul, G., Law, B., Lee, X., Malhi, Y., Meyers, T., Munger, W., Oechel, W., Pau U, K. T., Pilegaard, K., Schmid, H. P., Valentini, R., Verma, S., Vesala, T., Wilson, K., and Wofsy, S.: FLUXNET: a new tool to study the temporal and spatial variability of ecosystem-scale carbon dioxide, water vapor, and energy flux densities, B. Am. Meteorol. Soc., 82, 2415–2434, 2001.
Baldocchi, D., Sturtevant, C., and Fluxnet-contributors: Does day and night sampling reduce spurious correlation between canopy photosynthesis and ecosystem respiration?, Agr. Forest Meteorol., 207, 117–126, https://doi.org/10.1016/j.agrformet.2015.03.010, 2015.
Bastviken, D., Tranvik, L. J., Downing, J. A., Crill, P. M., and Enrich-Prast, A.: Freshwater methane emissions offset the continental carbon sink, Science, 331, p. 50, https://doi.org/10.1126/science.1196808, 2011.
Battin, T. J., Luyssaert, S., Kaplan, L. A., Aufdenkampe, A. K., Richter, A., and Tranvik, L. J.: The boudless carbon cycle, Nat. Geosci., 2, 598–600, 2009.
Berhe, A. A., Barnes, R. T., Six, J., and Marín-Spiotta, E.: Role of soil erosion in biogeochemical cycling of essential elements: carbon, nitrogen, and phosphorus, Annu. Rev. Earth Pl. Sc., 46, 521–548, https://doi.org/10.1146/annurev-earth-082517-010018, 2018.
Bondeau, A., Smith, P. C., Zaehle, S., Schaphoff, S., Lucht, W., Cramer, W., Gerten, D., Lotze-Campen, H., Müller, C., Reichstein, M., and Smith, B.: Modelling the role of agriculture for the 20th century global terrestrial carbon balamce, Glob. Change Biol., 13, 679–706, https://doi.org/10.1111/j.1365-2486.2006.01305.x, 2007.
Bouillon, S., Borges, A. V., Castañeda-Moya, E., Diele, K., Dittmar, T., Duke, N. C., Kristensen, E., Lee, S. Y., Marchand, C., Middelburg, J. J., Rivera-Monroy, V. H., Smith, T. J. I., and Twilley, R. R.: Mangrove production and carbon sinks: A revision of global budget estimates, Global Biogeochem. Cy., 22, GB2013, https://doi.org/10.1029/2007GB003052, 2008.
Boyer, E. W., Hornberger, G. M., Bencala, K. E., and McKnight, D.: Overview of a simple model describing variation of dissolved organic carbon in an upland catchment, Ecol. Model., 86, 183–188, 1996.
Brinck, K., Fischer, R., Groeneveld, J., Lehmann, S., De Paula, M. D., Pütz, S., Sexton, J. O., Song, D., and Huth, A.: High resolution analysis of tropical forest fragmentation and its impact on the global carbon cycle, Nat. Commun., 8, 14855, https://doi.org/10.1038/ncomms14855, 2017.
Campbell, J. E., Berry, J. A., Seibt, U., Smith, S. J., Montzka, S. A., Launois, T., Belviso, S., Bopp, L., and Laine, M.: Large historical growth in global terrestrial gross primary production, Nature, 544, 84–87, https://doi.org/10.1038/nature22030, 2017.
Carvalhais, N., Forkel, M., Khomik, M., Bellarby, J., Jung, M., Migliavacca, M., Mu, M., Saatchi, S., Santoro, M., Thurner, M., Weber, U., Ahrens, B., Beer, C., Cescatti, A., Randerson, J. T., and Reichstein, M.: Global covariation of carbon turnover times with climate in terrestrial ecosystems, Nature, 514, 213–217, https://doi.org/10.1038/nature13731, 2014.
Chapin, F. S. III, Woodwell, G. M., Randerson, J. T., Rastetter, E. B., Lovett, G. M., Baldocchi, D. D., Clark, D. A., Harmon, M. E., Schimel, D. S., Valentini, R., Wirth, C., Aber, J. D., Cole, J. J., Goulden, M. L., Harden, J. W., Heimann, M., Howarth, R. W., Matson, P. A., McGuire, A. D., Melillo, J. M., Mooney, H. A., Neff, J. C., Houghton, R. A., Pace, M. L., Ryan, M. G., Running, S. W., Sala, O. E., Schlesinger, W. H., and Schulze, E.-D.: Reconciling carbon-cycle concepts, terminology, and methods, Ecosystems, 9, 1041–1050, https://doi.org/10.1007/s10021-005-0105-7, 2006.
Chappell, A., Baldock, J., and Sanderman, J.: The global significance of omitting soil erosion from soil organic carbon cycling schemes, Nat. Clim. Change, 6, 187–191, https://doi.org/10.1038/NCLIMATE2829, 2016.
Chen, M., Rafique, R., Asrar, G. R., Bond-Lamberty, B., Ciais, P., Zhao, F., Reyer, C. P. O., Ostberg, S., Chang, J., Ito, A., Yang, J., Zeng, N., Kalnay, E., West, T., Leng, G., Francois, L., Munhoven, G., Henrot, A., Tian, H., Pan, S., Nishida, K., Viovy, N., Morfopoulos, C., Betts, R., Schaphoff, S., Steinkamp, J., and Hickler, T.: Regional contribution to variability and trends of global gross primary productivity, Environ. Res. Lett., 12, 105005, https://doi.org/10.1088/1748-9326/aa8978, 2017.
Chu, H., Gottgens, J. F., Chen, J., Sun, G., Deai, A. R., Ouyang, Z., Shao, C., and Czajkowski, K.: Climatic variability, hydrologic anomaly, and methane emission can turn productive freshwater marshes into net carbon sources, Glob. Change Biol., 21, 1165–1181, https://doi.org/10.1111/gcb.12760, 2015.
Ciais, P., Bousquet, P., Freibauer, A., and Naegler, T.: Horizontal displacement of carbon associated with agriculture and its impact on atmospheric CO2, Global Biogeochem. Cy., 21, GB2014, https://doi.org/10.1029/2006GB002741, 2007.
Ciais, P., Dolman, A. J., Bombelli, A., Duren, R., Peregon, A., Rayner, P. J., Miller, C., Gobron, N., Kinderman, G., Marland, G., Gruber, N., Chevallier, F., Andres, R. J., Balsamo, G., Bopp, L., Bréon, F.-M., Broquet, G., Dargaville, R., Battin, T. J., Borges, A., Bovensmann, H., Buchwitz, M., Butler, J., Canadell, J. G., Cook, R. B., DeFries, R., Engelen, R., Gurney, K. R., Heinze, C., Heimann, M., Held, A., Henry, M., Law, B., Luyssaert, S., Miller, J., Moriyama, T., Moulin, C., Myneni, R. B., Nussli, C., Obersteiner, M., Ojima, D., Pan, Y., Paris, J.-D., Piao, S. L., Poulter, B., Plummer, S., Quegan, S., Raymond, P., Reichstein, M., Rivier, L., Sabine, C., Schimel, D., Tarasova, O., Valentini, R., Wang, R., van der Werf, G., Wickland, D., Williams, M., and Zehner, C.: Current systematic carbon-cycle observations and the need for implementing a policy-relevant carbon observing system, Biogeosciences, 11, 3547–3602, https://doi.org/10.5194/bg-11-3547-2014, 2014.
Climate Research Unit (CRU): CRU TS3.25: Climatic Research Unit (CRU) Time-Series (TS) Version 3.25 of High-Resolution Gridded Data of Month-by-month Variation in Climate (Jan. 1901–Dec. 2016), University of East Anglia, available at: https://crudata.uea.ac.uk/cru/data/hrg/, last access: 1 November 2019.
Curry, C. L.: Modeling the soil consumption of atmospheric methane at the global scale, Global Biogeochem. Cy., 21, GB4012, https://doi.org/10.1029/2006GB002818, 2007.
Dai, M., Yin, Z., Meng, F., Liu, Q., and Cai, W.-J.: Spatial distribution of riverine DOC inputs to the ocean: an updated global synthesis, Curr. Opin. Env. Sust., 4, 170–178, https://doi.org/10.1016/j.cosust.2012.03.003, 2012.
Dignac, M.-F., Derrien, D., Barré, P., Barot, S., Cécillon, L., Chenu, C., Chevallier, T., Freschet, G. T., Garnier, P., Guenet, B., Hedde, M., Klumpp, K., Lashermes, G., Maron, P.-A., Nunan, N., Roumet, C., and Basile-Doelsch, I.: Increasing soil carbon storage: mechanisms, effects of agricultural practices and proxies. A review, Agron. Sustain. Dev., 37, 14, https://doi.org/10.1007/s13593-017-0421-2, 2017.
Drake, T. W., Raymond, P. A., and Spencer, R. G. M.: Terrestrial carbon inputs to inland waters: A current synthesis of estimates and uncertainty, Limnol. Oceanogr. Lett., 3, 132–142, https://doi.org/10.1002/lol2.10055, 2018.
Elbert, W., Weber, B., Burrows, S., Steinkamp, J., Büdel, B., Andreae, M. O., and Pöschl, U.: Contribution of cryptogamic covers to the global cycles of carbon and nitrogen, Nat. Geosci., 5, 459–462, https://doi.org/10.1038/NGEO1486, 2012.
Erb, K.-H., Kastner, T., Plutzar, C., Bais, A. L. S., Carvalhais, N., Fetzel, T., Gingrich, S., Haberl, H., Lauk, C., Niedertscheider, M., Pongratz, J., Thurner, M., and Luyssaert, S.: Unexpectedly large impact of forest management and grazing on global vegetation biomass, Nature, 553, 73–76, https://doi.org/10.1038/nature25138, 2018.
Fang, Y., Michalak, A. M., Schwalm, C. R., Huntzinger, D. N., Berry, J. A., Ciais, P., Piao, S., Poulter, B., Fisher, J. B., Cook, R. B., Hayes, D., Huang, M., Ito, A., Jain, A., Lei, H., Lu, C., Mao, J., Parazoo, N. C., Peng, S., Ricciuto, D. M., Shi, X., Tao, B., Tian, H., Wang, W., Wei, Y., and Yang, J.: Global land carbon sink response to temperature and precipitation varies with ENSO phase, Environ. Res. Lett., 12, 064007, https://doi.org/10.1088/1748-9326/aa6e8e, 2017.
Friedlingstein, P., Meinshausen, M., Arora, V. K., Jones, C. D., Anav, A., Liddicoat, S. K., and Knutti, R.: Uncertainties in CMIP5 climate projections due to carbon cycle feedbacks, J. Climate, 27, 511–526, 2014.
Friend, A. D., Lucht, W., Rademacher, T. T., Keribin, R. M., Betts, R., Cadule, P., Ciais, P., Clark, D. B., Dankers, R., Falloon, P., Ito, A., Kahana, R., Kleidon, A., Lomas, M. R., Nishina, K., Ostberg, S., Pavlick, R., Peylin, P., Schaphoff, S., Vuichard, N., Warszwski, L., Wiltshire, A., and Woodward, F. I.: Carbon residence time dominates uncertainty in terrestrial vegetation responses to future climate and atmospheric CO2, P. Natl. Acad. Sci. USA, 111, 3280–3285, https://doi.org/10.1073/pnas.1222477110, 2014.
Fu, Z., Li, D., Hararuk, O., Schwalm, C., Luo, Y., Yan, L., and Niu, S.: Recovery time and state change of terrestrial carbon cycle after disturbance, Environ. Res. Lett., 12, 104004, https://doi.org/10.1088/1748-9326/aa8a5c, 2017.
Fuchs, R., Herold, M., Verburg, P. H., Clevers, J. G. P. W., and Eberle, J.: Gross changes in reconstructions of historic land cover/use for Europe between 1900 and 2010, Glob. Change Biol., 21, 299–313, https://doi.org/10.1111/gcb.12714, 2015.
Fung, I., John, J., Lerner, J., Matthews, E., Prather, M., Steele, L. P., and Fraser, P. J.: Three-dimensional model synthesis of the global methane cycle, J. Geophys. Res., 96, 13033–13065, 1991.
Galy, V., Peucker-Ehrenbrink, B., and Eglinton, T.: Global carbon export from the terrestrial biosphere controlled by erosion, Nature, 521, 204–207, https://doi.org/10.1038/nature14400, 2015.
Geron, C. D., Daly, R. W., Arnts, R. R., Guenther, A. B., and Mowry, F. L.: Canopy level emissions of 2-methyl-3-buten-2-ol, monoterpenes, and sesquiterpenes from an experimental Pinus taeda plantation, Sci. Total Environ., 565, 730–741, https://doi.org/10.1016/j.scitotenv.2016.05.034, 2016.
Gower, S. T.: Patterns and mechanisms of the forest carbon cycle, Annu. Rev. Env. Resour., 28, 169–204, https://doi.org/10.1146/annurev.energy.28.050302.105515, 2003.
Grieve, I. C.: A model of dissolved organic carbon concentrations in soil and stream waters, Hydrol. Process., 5, 301–307, 1991.
Guenther, A.: Seasonal and spatial variations in natural volatile organic compound emissions, Ecol. Appl., 7, 34–45, 1997.
Guenther, A., Baugh, W., Davis, K., Hampton, G., Harley, P., Klinger, L., Vierling, L., Zimmerman, P., Allwine, E., Dilts, S., Lamb, B., Westberg, H., Baldocchi, D., Geron, C., and Pierce, T.: Isoprene fluxes measured by enclosure, relaxed eddy accumulation, surface layer gradient, mixed layer gradient, and mixed layer mass balance techniques, J. Geophys. Res., 101, 18555–18567, 1996.
Guenther, A. B., Jiang, X., Heald, C. L., Sakulyanontvittaya, T., Duhl, T., Emmons, L. K., and Wang, X.: The Model of Emissions of Gases and Aerosols from Nature version 2.1 (MEGAN2.1): an extended and updated framework for modeling biogenic emissions, Geosci. Model Dev., 5, 1471–1492, https://doi.org/10.5194/gmd-5-1471-2012, 2012.
Harris, I., Jones, P. D., Osborn, T. J., and Lister, D. H.: Updated high-resolution grids of monthly climatic observations – the CRU TS3.10 Dataset, Int. J. Climatol., 34, 623–642, https://doi.org/10.1002/joc.3711, 2014.
Hartmann, J., Jansen, N., Dürr, H. H., Kempe, S., and Köhler, P.: Global CO2-consumption by chemical weathering: What is the contribution of highly active weathering regions?, Global Planet. Change, 69, 185–194, https://doi.org/10.1016/j.gloplacha.2009.07.007, 2009.
Hirata, R., Takagi, K., Ito, A., Hirano, T., and Saigusa, N.: The impact of climate variation and disturbances on the carbon balance of forests in Hokkaido, Japan, Biogeosciences, 11, 5139–5154, https://doi.org/10.5194/bg-11-5139-2014, 2014.
Hoelzemann, J. J., Schultz, M. G., Brasseur, G. P., Granier, C., and Simon, M.: Global Wildland Fire Emission Model (GWEM): Evaluating the use of global area burnt satellite data, J. Geophys. Res., 109, D14S04, https://doi.org/10.1029/2003JD003666, 2004.
Houghton, R. A.: Revised estimates of the annual net flux of carbon to the atmosphere from changes in land use and land management 1850–2000, Tellus, 55B, 378–390, 2003.
Houghton, R. A. and Nassikas, A. A.: Global and regional fluxes of carbon from land use and land cover change 1850–2015, Global Biogeochem. Cy., 31, 456–472, https://doi.org/10.1002/2016GB005546, 2017.
Houghton, R. A., Hobbie, J. E., Melillo, J. M., Moore, B., Peterson, B. J., Shaver, G. R., and Woodwell, G. M.: Changes in the carbon content of terrestrial biota and soils between 1860 and 1980: a net release of CO2 to the atmosphere, Ecol. Monogr., 53, 235–262, 1983.
Houghton, R. A., Davidson, E. A., and Woodwell, G. M.: Missing sinks, feedbacks, and understanding the role of terrestrial ecosystems in the global carbon balance, Global Biogeochem. Cy., 12, 25–34, 1998.
Huijnen, V., Wooster, M. J., Kaiser, J. W., Gaveau, D. L. A., Flemming, J., Parrington, M., Inness, A., Murdiyarso, D., Main, B., and van Weele, M.: Fire carbon emissions over maritime southeast Asia in 2015 largest since 1997, Sci. Rep., 6, 26886, https://doi.org/10.1038/srep26886, 2016.
Huntzinger, D. N., Michalak, A. M., Schwalm, C., Ciais, P., King, A. W., Fang, Y., Schaefer, K., Wei, Y., Cook, R. B., Fisher, J. B., Hayes, D., Huang, M., Ito, A., Jain, A. K., Lei, H., Lu, C., Maignan, F., Mao, J., Parazoo, N., Peng, S., Poulter, B., Ricciuto, D., Shi, X., Tian, H., Wang, W., Zeng, N., and Zhao, F.: Uncertainty in the response of terrestrial carbon sink to environmental drivers undermines carbon-climate feedback predictions, Sci. Rep., 7, 4765, https://doi.org/10.1038/s41598-017-03818-2, 2017.
Hurtt, G. C., Frolking, S., Fearon, M. G., Moore, B., Shevliakova, E., Malyshev, S., Pacala, S. W., and Houghton, R. A.: The underpinnings of land-use history: three centuries of global gridded land-use transitions, wood-harvest activity, and resulting secondary lands, Glob. Change Biol., 12, 1–22, https://doi.org/10.1111/j.1365-2486.2006.01150.x, 2006 (data available at: http://luh.umd.edu/data.shtml, last access: 1 November 2019).
Ichii, K., Kondo, M., Lee, Y.-H., Wang, S.-Q., Kim, J., Ueyama, M., Lim, H.-J., Shi, H., Suzuki, T., Ito, A., Ju, W., Huang, M., Sasai, T., Asanuma, J., Han, S., Hirano, T., Hirata, R., Kato, T., Kwon, H., Li, S.-G., Li, Y.-N., Maeda, T., Miyata, A., Matsuura, Y., Murayama, S., Nakai, Y., Ohta, T., Saitoh, T. M., Saigusa, N., Takagi, K., Tang, Y.-H., Wang, H.-M., Yu, G.-R., Zhang, Y.-P., and Zaho, F.-H.: Site-level model-data synthesis of terrestrial carbon fluxes in the CarboEastAsia eddy-covariance observation network: Toward future modeling efforts, J. Forest Res., 18, 13–20, https://doi.org/10.1007/s10310-012-0367-9, 2013.
Inatomi, M., Ito, A., Ishijima, K., and Murayama, S.: Greenhouse gas budget of a cool temperate deciduous broadleaved forest in Japan estimated using a process-based model, Ecosystems, 13, 472–483, https://doi.org/10.1007/s10021-010-9332-7, 2010.
Ito, A.: Simulated impacts of climate and land-cover change on soil erosion and implication for the carbon cycle, 1901 to 2100, Geophys. Res. Lett., 34, L09403, https://doi.org/10.1029/2007GL029342, 2007.
Ito, A.: Changing ecophysiological processes and carbon budget in East Asian ecosystems under near-future changes in climate: Implications for long-term monitoring from a process-based model, J. Plant Res., 123, 577–588, https://doi.org/10.1007/s10265-009-0305-x, 2010.
Ito, A. and Inatomi, M.: Use of a process-based model for assessing the methane budgets of global terrestrial ecosystems and evaluation of uncertainty, Biogeosciences, 9, 759–773, https://doi.org/10.5194/bg-9-759-2012, 2012.
Ito, A. and Oikawa, T.: A simulation model of the carbon cycle in land ecosystems (Sim-CYCLE): A description based on dry-matter production theory and plot-scale validation, Ecol. Model., 151, 147–179, 2002.
Ito, A., Inatomi, M., Huntzinger, D. N., Schwalm, C., Michalak, A. M., Cook, R., King, A. W., Mao, J., Wei, Y., Post, W. M., Wang, W., Arain, M. A., Huang, M., Lei, H., Tian, H., Lu, C., Yang, J., Tao, B., Jain, A., Poulter, B., Peng, S., Ciais, P., Fisher, J. B., Parazoo, N., Schaefer, K., Peng, C., Zeng, N., and Zhao, F.: Decadal trends in the seasonal-cycle amplitude of terrestrial CO2 exchange resulting from the ensemble of terrestrial biosphere models, Tellus B, 68, 28968, https://doi.org/10.3402/tellusb.v68.28968, 2016.
Ito, A., Nishina, K., Reyer, C. P. O., François, L., Henrot, A.-J., Munhoven, G., Jacquemin, I., Tian, H., Yang, J., Pan, S., Morfopoulos, C., Betts, R., Hickler, T., Steinkamp, J., Ostberg, S., Schaphoff, S., Ciais, P., Chang, J., Rafique, R., Zeng, F., and Zhao, F.: Photosynthetic productivity and its efficiencies in ISIMIP2a biome models: benchmarking for impact assessment studies, Environ. Res. Lett., 12, 085001, https://doi.org/10.1088/1748-9326/aa7a19, 2017.
Jiang, C. and Ryu, Y.: Multi-scale evaluation of global gross primary productivity and evapotranspiration products derived from Breathing Earth System Simulator (BESS), Remote Sens. Environ., 186, 528–547, https://doi.org/10.1016/j.rse.2016.08.030, 2016.
Jung, M., Reichstein, M., Margolis, H. A., Cescatti, A., Richardson, A. D., Arain, M. A., Arneth, A., Bernhofer, C., Bonal, D., Chen, J., Gianelle, D., Gobron, N., Kiely, G., Kutsch, W., Lasslop, G., Law, B. E., Lindroth, A., Merbold, L., Montagnani, L., Moors, E. J., Papale, D., Sottocornola, M., Vaccari, F., and Williams, C.: Global patterns of land-atmosphere fluxes of carbon dioxide, latent heat, and sensible heat derived from eddy covariance, satellite, and meteorological observations, J. Geophys. Res., 116, G00J07, https://doi.org/10.1029/2010JG001566, 2011.
Jung, M., Reichstein, M., Schwalm, C. R., Huntingford, C., Sitch, S., Ahlström, A., Arneth, A., Camps-Valls, G., Ciais, P., Friedlingstein, P., Gans, F., Ichii, K., Jain, A. K., Kato, E., Papale, D., Poulter, B., Raduly, B., Rödenbeck, C., Tramontana, G., Viovy, N., Wang, Y.-P., Weber, U., Zaehle, S., and Zeng, N.: Compensatory water effects link yearly global land CO2 sink changes to temperature, Nature, 541, 516–520, https://doi.org/10.1038/nature20780, 2017.
Kato, E., Kinoshita, T., Ito, A., Kawamiya, M., and Yamagata, T.: Evaluation of spatially explicit emission scenario of land-use change and biomass burning using a process based biogeochemical model, J. Land Use Sci., 8, 104–122, https://doi.org/10.1080/1747423X.2011.628705, 2013.
Keeling, R. F., Piper, S. C., Bollenbacher, A. F., and Walker, J. S.: Atmospheric carbon dioxide record from Mauna Loa, in, Carbon Dioxide Information Analysis Center, Oak Ridge, USA, 2009.
Kirkels, F. M. S. A., Cammeraat, L. H., and Kuhn, N. J.: The fate of soil organic carbon upon erosion, transport and deposition in agricultural landscapes – A review of different concepts, Geomorphol., 226, 94–105, https://doi.org/10.1016/j.geomorph.2014.07.023, 2014.
Kirschbaum, M. U. F., Zeng, G., Ximenes, F., Giltrap, D. L., and Zeldis, J. R.: Towards a more complete quantification of the global carbon cycle, Biogeosciences, 16, 831–846, https://doi.org/10.5194/bg-16-831-2019, 2019.
Knorr, W., Arneth, A., and Jiang, L.: Demograhic controls of future global fire risk, Nat. Clim. Change, 6, 781–785, https://doi.org/10.1038/NCLIMATE2999, 2016.
Kondo, M., Ichii, K., Takagi, H., and Sasakawa, M.: Comparison of the data-driven top-down and bottom-up global terrestrial CO2 exchanges: GOSAT CO2 inversion and empirical eddy flux upscaling, J. Geophys. Res., 120, 1226–1245, https://doi.org/10.1002/2014JG002866, 2015.
Lal, R.: Soil erosion and the global carbon budget, Environ. Int., 29, 437–450, 2003.
Lal, R.: Accelerated soil erosion as a source of atmospheric CO2, Soil Till. Res., 188, 35–40, https://doi.org/10.1016/j.still.2018.02.001, 2019.
Lathière, J., Hauglustaine, D. A., Friend, A. D., De Noblet-Ducoudré, N., Viovy, N., and Folberth, G. A.: Impact of climate variability and land use changes on global biogenic volatile organic compound emissions, Atmos. Chem. Phys., 6, 2129–2146, https://doi.org/10.5194/acp-6-2129-2006, 2006.
Lehner, B. and Döll, P.: Development and validation of a global database of lakes, reservoirs and wetlands, J. Hydrol., 296, 1–22, https://doi.org/10.1016/j.jhydrol.2004.03.028, 2004 (data available at: https://www.worldwildlife.org/pages/global-lakes-and-wetlands-database, last access: 1 November 2019).
Le Quéré, C., Andrew, R. M., Friedlingstein, P., Sitch, S., Hauck, J., Pongratz, J., Pickers, P. A., Korsbakken, J. I., Peters, G. P., Canadell, J. G., Arneth, A., Arora, V. K., Barbero, L., Bastos, A., Bopp, L., Chevallier, F., Chini, L. P., Ciais, P., Doney, S. C., Gkritzalis, T., Goll, D. S., Harris, I., Haverd, V., Hoffman, F. M., Hoppema, M., Houghton, R. A., Hurtt, G., Ilyina, T., Jain, A. K., Johannessen, T., Jones, C. D., Kato, E., Keeling, R. F., Goldewijk, K. K., Landschützer, P., Lefèvre, N., Lienert, S., Liu, Z., Lombardozzi, D., Metzl, N., Munro, D. R., Nabel, J. E. M. S., Nakaoka, S., Neill, C., Olsen, A., Ono, T., Patra, P., Peregon, A., Peters, W., Peylin, P., Pfeil, B., Pierrot, D., Poulter, B., Rehder, G., Resplandy, L., Robertson, E., Rocher, M., Rödenbeck, C., Schuster, U., Schwinger, J., Séférian, R., Skjelvan, I., Steinhoff, T., Sutton, A., Tans, P. P., Tian, H., Tilbrook, B., Tubiello, F. N., van der Laan-Luijkx, I. T., van der Werf, G. R., Viovy, N., Walker, A. P., Wiltshire, A. J., Wright, R., Zaehle, S., and Zheng, B.: Global Carbon Budget 2018, Earth Syst. Sci. Data, 10, 2141–2194, https://doi.org/10.5194/essd-10-2141-2018, 2018.
Li, W., Ciais, P., Wang, Y., Peng, S., Broquet, G., Ballantyne, A. P., Canadell, J. G., Cooper, L., Friedlingstein, P., Le Quéré, C., Myneni, R. B., Peters, G. P., Piao, S., and Pongratz, J.: Reducing uncertainties in decadal variability of the global carbon budget with multiple datasets, P. Natl. Acad. Sci. USA, 113, 13104–13108, https://doi.org/10.1073/pnas.1603956113, 2016.
Lovett, G. M., Cole, J. J., and Pace, M. L.: Is net ecosystem production equal to ecosystem carbon accumulation?, Ecosystems, 9, 152–155, 2006.
Luyssaert, S., Schulze, E.-D., Börner, A., Knohl, A., Hessenmöller, D., Law, B. E., Ciais, P., and Grace, J.: Old-growth forests as global carbon sinks, Nature, 455, 213–215, https://doi.org/10.1038/nature07276, 2008.
Maavara, T., Lauerwald, R., Regnier, P., and Van Cappellen, P.: Global perturbation of organic carbon cycling by river damming, Nat. Commun., 8, 15347, https://doi.org/10.1038/ncomms15347, 2017.
Manabe, S.: Climate and the ocean circulation I. the atmospheric circulation and the hydrology of the earth's surface, Mon. Weather Rev., 97, 739–774, 1969.
McGuire, A. D., Sitch, S., Clein, J. S., Dargaville, R., Esser, G., Foley, J., Heimann, M., Joos, F., Kaplan, J., Kicklighter, D. W., Meier, R. A., Melillo, J. M., Moore, B. I., Williams, L. J., and Wittenberg, U.: Carbon balance of the terrestrial biosphere in the twentieth century: analysis of CO2, climate and land use effects with four process-based ecosystem models, Global Biogeochem. Cy., 15, 183–206, 2001.
Mendonça, R., Müller, R. A., Clow, D., Verpoorter, C., Raymond, P., Tranvik, L. J., and Sobek, S.: Organic carbon burial in global lakes and reservoirs, Nat. Commun., 8, 1694, https://doi.org/10.1038/s41467-017-01789-6, 2017.
Meybeck, M.: Riverine transport of atmospheric carbon: sources, global typology and budget, Water Air Soil Poll., 70, 443–463, 1993.
Minasny, B., Malone, B. P., McBratney, A. B., Angers, D. A., Arrouays, D., Chambers, A., Chaplot, V., Chen, Z.-S., Cheng, K., Das, B. S., Field, D. J., Gimona, A., Hedley, C. B., Hong, S. Y., Mandal, B., Marchant, B. P., Martin, M., McConkey, B. G., Mulder, V. L., O'Rourke, S., Richer-de-Forges, A. C., Odeh, I., Padarian, J., Paustian, K., Pan, G., Poggio, L., Savin, I., Stolbovoy, V., Stockmann, U., Sulaeman, Y., Tsui, C.-C., Vågen, T.-G., van Wesemael, B., and Winowiecki, L.: Soil carbon 4 per mille, Geoderma, 292, 59–86, https://doi.org/10.1016/j.geoderma.2017.01.002, 2017.
Monfreda, C., Ramankutty, N., and Foley, J. A.: Farming the planet: 2. Geographic distribution of crop areas, yields, physiological types, and net primary production in the year 2000, Global Biogeochem. Cy., 22, GB1022, https://doi.org/10.1029/2007GB002947, 2008.
Nadeu, E., Gobin, A., Fiener, P., van Wesemael, B., and van Oost, K.: Modelling the impact of agricultural management on soil carbon stocks at the regional scale: the role of lateral fluxes, Glob. Change Biol., 21, 3181–3192, https://doi.org/10.1111/gcb.12889, 2015.
Naipal, V., Ciais, P., Wang, Y., Lauerwald, R., Guenet, B., and Van Oost, K.: Global soil organic carbon removal by water erosion under climate change and land use change during AD 1850–2005, Biogeosciences, 15, 4459–4480, https://doi.org/10.5194/bg-15-4459-2018, 2018.
Nelson, P. N., Baldock, J. A., and Oades, J. M.: Concentration and composition of dissolved organic carbon in stream in relation to catchment soil properties, Biogeochem., 19, 27–50, 1993.
Olson, J. S., Watts, J. A., and Allison, L. J.: Carbon in live vegetation of major world ecosystems, Oak Ridge National Laboratory, USA, ORNL-5862, 1983.
Pan, Y., Birdsey, R. A., Fang, J., Houghton, R., Kauppi, P. E., Kurz, W. A., Phillips, O. L., Shvidenko, A., Lewis, S. L., Canadell, J. G., Ciais, P., Jackson, R. B., Pacala, S. W., McGuire, A. D., Piao, S., Rautiainen, A., Sitch, S., and Hayes, D.: A large and persistent carbon sink in the world's forests, Science, 333, 988–993, https://doi.org/10.1126/science.1201609, 2011.
Paustian, K., Lehmann, J., Ogle, S., Reay, D., Robertson, G. P., and Smith, P.: Climate–smart soils, Nature, 532, 49–57, https://doi.org/10.1038/nature17174, 2016.
Peters, G. P., Davis, S. J., and Andrew, R.: A synthesis of carbon in international trade, Biogeosciences, 9, 3247–3276, https://doi.org/10.5194/bg-9-3247-2012, 2012.
Peters, W., Jacobson, A. R., Sweeney, C., Andrews, A. E., Conway, T. J., Masarie, K., Miller, J. B., Bruhwiler, L. M. P., Pétron, G., Hirsch, A. I., Worthy, D. E. J., van der Werf, G. R., Randerson, J. T., Wennberg, P. O., Krol, M. C., and Tans, P. P.: An atmospheric perspective on North American carbon dioxide exchange: CarbonTracker, P. Natl. Acad. Sci. USA, 104, 18925–18930, https://doi.org/10.1073/pnas.0708986104, 2007.
Piao, S., Huang, M., Liu, Z., Wang, X., Ciais, P., Canadell, J. G., Wang, K., Bastos, A., Friedlingstein, P., Houghton, R. A., Le Quéré, C., Liu, Y., Myneni, R. B., Peng, S., Pongratz, J., Sitch, S., Yan, T., Wang, Y., Zhu, Z., Wu, D., and Wang, T.: Lower land-use emissions responsible for increased net land carbon sink during the slow warming period, Nat. Geosci., 11, 739–743, https://doi.org/10.1038/s41561-018-0204-7, 2018.
Piao, S. L., Ito, A., Li, S. G., Huang, Y., Ciais, P., Wang, X. H., Peng, S. S., Nan, H. J., Zhao, C., Ahlström, A., Andres, R. J., Chevallier, F., Fang, J. Y., Hartmann, J., Huntingford, C., Jeong, S., Levis, S., Levy, P. E., Li, J. S., Lomas, M. R., Mao, J. F., Mayorga, E., Mohammat, A., Muraoka, H., Peng, C. H., Peylin, P., Poulter, B., Shen, Z. H., Shi, X., Sitch, S., Tao, S., Tian, H. Q., Wu, X. P., Xu, M., Yu, G. R., Viovy, N., Zaehle, S., Zeng, N., and Zhu, B.: The carbon budget of terrestrial ecosystems in East Asia over the last two decades, Biogeosciences, 9, 3571–3586, https://doi.org/10.5194/bg-9-3571-2012, 2012.
Prigent, C., Matthews, E., Aires, F., and Rossow, W. B.: Remote sensing of global wetland dynamics with multiple satellite data sets, Geophys. Res. Lett., 28, 4631–4634, 2001.
Pugh, T. A. M., Lindeskog, M., Smith, B., Poulter, B., Arneth, A., Haverd, V., and Calle, L.: Role of forest regrowth in global carbon sink dynamics, P. Natl. Acad. Sci. USA, 116, 4382–4387, https://doi.org/10.1073/pnas.1810512116, 2019.
Ramankutty, N. and Foley, J. A.: Characterizing patterns of global land use: An analysis of global croplands data, Global Biogeochem. Cy., 12, 667–685, 1998.
Randerson, J. T., Chapin, F. S. I., Harden, J. W., Neff, J. C., and Harmon, M. E.: Net ecosystem production: a comprehensive measure of net carbon accumulation by ecosystems, Ecol. Appl., 12, 937–947, 2002.
Randerson, J. T., van der Werf, G. R., Collatz, G. J., Giglio, L., Still, C. J., Kasibhatla, P., Miller, J. B., White, J. W. C., DeFries, R. S., and Kasischke, E. S.: Fire emissions from C3 and C4 vegetation and their influence on interannual variability of atmospheric CO2 and δ13CO2, Global Biogeochem. Cy., 19, GB2019, https://doi.org/10.1029/2004GB002366, 2005.
Randerson, J. T., Liu, H., Flanner, M. G., Chambers, S. D., Jin, Y., Hess, P. G., Pfister, G., Mack, M. C., Treseder, K. K., Welp, L. R., Chapin, F. S., Harden, J. W., Goulden, M. L., Lyons, E., Neff, J. C., Schuur, E. A. G., and Zender, C. S.: The impact of boreal forest fire on climate warming, Science, 314, 1130–1132, 2006.
Randerson, J. T., Chen, Y., van der Werf, G. R., Rogers, B. M., and Morton, D. C.: Global burned area and biomass burning emissions from small fires, J. Geophys. Res., 117, G04012, https://doi.org/10.1029/2012JG002128, 2012.
Raymond, P. A., Hartmann, J., Lauerwald, R., Sobek, S., McDonald, C., Hoover, M., Butman, D., Striegl, R., Mayorga, E., Humborg, C., Kortelainen, P., Dürr, H., Meybeck, M., Ciais, P., and Guth, P.: Global carbon dioxide emissions from inland waters, Nature, 503, 355–359, https://doi.org/10.1038/nature12760, 2013.
Regnier, P., Friedlingstein, P., Ciais, P., Mackenzie, F. T., Gruber, N., Janssens, I. A., Laruelle, G. G., Lauerwald, R., Luyssaert, S., Andersson, A. J., Arndt, S., Arnosti, C., Borges, A. V., Dale, A. W., Gallego-Sala, A., Goddéris, Y., Goossens, N., Hartmann, J., Heinze, C., Ilyina, T., Joos, F., LaRowe, D. E., Leifeld, J., Meysman, F. J. R., Munhoven, G., Raymond, P. A., Spahni, R., Suntharalingam, P., and Thullner, M.: Anthropogenic perturbation of the carbon fluxes from land to ocean, Nat. Geosci., 6, 597–607, https://doi.org/10.1038/NGEO1830, 2013.
Reichstein, M., Bahn, M., Ciais, P., Frank, D., Mahecha, M. D., Seneviratne, S. I., Zscheischler, J., Beer, C., Buchmann, N., Frank, D. C., Papale, D., Rammig, A., Smith, P., Thonicke, K., van der Velde, M., Vicca, S., Walz, A., and Wattenbach, M.: Climate extremes and the carbon cycle, Nature, 500, 287–295, https://doi.org/10.1038/nature12350, 2013.
Renard, K. G., Foster, G. R., Weesies, G. A., McCool, D. K., and Yoder, D. C.: Predicting Erosion by Water: A Guide to Conservation Planning with the Revised Universal Soil Loss Equation (RUSLE). Handbook 703, US Department of Agriculture, Washington, D.C., USA, 1997.
Reynolds, C. A., Jackson, T. J., and Rawls, W. J.: Estimated Available Water Content from the FAO Soil Map of the World, Global Soil Profile Databases, Pedo-transfer Functions, USDA Agricultural Research Service, Washington, D.C., USA, 1999.
Saatchi, S. S., Harris, N. L., Brown, S., Lefsky, M., Mitchard, E. T. A., Salas, W., Zutta, B. R., Buermann, W., Lewis, S. L., Hagen, S., Petrova, S., White, L., Silman, M., and Morel, A.: Benchmark map of forest carbon stocks in tropical regions across three continents, P. Natl. Acad. Sci. USA, 108, 9899–9904, https://doi.org/10.1073/pnas.1019576108, 2011.
Santín, C., Doerr, S. H., Preston, C. M., and González-Rodríguez, G.: Pyrogenic organic matter production from wildfires: a missing sink in the global carbon cycle, Glob. Change Biol., 21, 1621–1633, https://doi.org/10.1111/gcb.12800, 2015.
Saunois, M., Bousquet, P., Poulter, B., Peregon, A., Ciais, P., Canadell, J. G., Dlugokencky, E. J., Etiope, G., Bastviken, D., Houweling, S., Janssens-Maenhout, G., Tubiello, F. N., Castaldi, S., Jackson, R. B., Alexe, M., Arora, V. K., Beerling, D. J., Bergamaschi, P., Blake, D. R., Brailsford, G., Bruhwiler, L., Crevoisier, C., Crill, P., Covey, K., Frankenberg, C., Gedney, N., Höglund-Isaksson, L., Ishizawa, M., Ito, A., Joos, F., Kim, H.-S., Kleinen, T., Krummel, P., Lamarque, J.-F., Langenfelds, R., Locatelli, R., Machida, T., Maksyutov, S., Melton, J. R., Morino, I., Naik, V., O'Doherty, S., Parmentier, F.-J. W., Patra, P. K., Peng, C., Peng, S., Peters, G. P., Pison, I., Prinn, R., Ramonet, M., Riley, W. J., Saito, M., Santini, M., Schroeder, R., Simpson, I. J., Spahni, R., Takizawa, A., Thornton, B. F., Tian, H., Tohjima, Y., Viovy, N., Voulgarakis, A., Weiss, R., Wilton, D. J., Wiltshire, A., Worthy, D., Wunch, D., Xu, X., Yoshida, Y., Zhang, B., Zhang, Z., and Zhu, Q.: Variability and quasi-decadal changes in the methane budget over the period 2000–2012, Atmos. Chem. Phys., 17, 11135–11161, https://doi.org/10.5194/acp-17-11135-2017, 2017.
Schimel, D., Pavlick, R., Fisher, J. B., Asner, G. P., Saatchi, S., Townsend, P., Miller, C., Frankenberg, C., Hibbard, K., and Cox, P.: Observing terrestrial ecosystems and the carbon cycle from space, Glob. Change Biol., 21, 1762–1776, https://doi.org/10.1111/gcb.12822, 2015.
Schimel, D. S., House, J. I., Hibbard, K. A., Bousquet, P., Ciais, P., Peylin, P., Braswell, B. H., Apps, M. J., Baker, D., Bondeau, A., Canadell, J., Churkina, G., Cramer, W., Denning, A. S., Field, C. B., Friedlingstein, P., Goodale, C., Heimann, M., Houghton, R. A., Melillo, J. M., Moore, B. I., Murdiyarso, D., Noble, I., Pacala, S. W., Prentice, I. C., Raupach, M. R., Rayner, P. J., Scholes, R. J., Steffen, W. L., and Wirth, C.: Recent patterns and mechanisms of carbon exchange by terrestrial ecosystems, Nature, 414, 169–172, 2001.
Schlesinger, W. H.: An evaluation of abiotic carbon sinks in deserts, Glob. Change Biol., 23, 25–27, https://doi.org/10.1111/gcb.13336, 2017.
Schleussner, C.-F., Rogelj, J., Schaeffer, M., Lissner, T., Licker, R., Fischer, E. M., Knutti, R., Levermann, A., Frieler, K., and Hare, W.: Science and policy characteristics of the Paris Agreement temperature goal, Nat. Clim. Change, 6, 827–835, https://doi.org/10.1038/NCLIMATE3096, 2016.
Schnitzer, S., Seitz, F., Eicker, A., Güntner, A., Wattenbach, M., and Menzel, A.: Estimation of soil loss by water erosion in the Chinese Loess Plateau using Universal Soil Loss Equation and GRACE, Geophys. J. Int., 193, 1283–1290, https://doi.org/10.1093/gji/ggt023, 2013.
Schulze, E.-D., Wirth, C., and Heimann, M.: Managing forests after Kyoto, Science, 289, 2058–2059, 2000.
Sellers, P. J., Schimel, D. S., Moore, B. I., Liu, J., and Eldering, A.: Observing carbon cycle – climate feedbacks from space, P. Natl. Acad. Sci. USA, 115, 7860–7868, https://doi.org/10.1073/pnas.1716613115, 2018.
Seneviratne, S. I., Donat, M. G., Pitman, A. J., Knutti, R., and Wilby, R. L.: Allowable CO2 emissions based on regional and impact-related climate targets, Nature, 529, 477–483, https://doi.org/10.1038/nature16542, 2016.
Sindelarova, K., Granier, C., Bouarar, I., Guenther, A., Tilmes, S., Stavrakou, T., Müller, J.-F., Kuhn, U., Stefani, P., and Knorr, W.: Global data set of biogenic VOC emissions calculated by the MEGAN model over the last 30 years, Atmos. Chem. Phys., 14, 9317–9341, https://doi.org/10.5194/acp-14-9317-2014, 2014.
Sitch, S., Friedlingstein, P., Gruber, N., Jones, S. D., Murray-Tortarolo, G., Ahlström, A., Doney, S. C., Graven, H., Heinze, C., Huntingford, C., Levis, S., Levy, P. E., Lomas, M., Poulter, B., Viovy, N., Zaehle, S., Zeng, N., Arneth, A., Bonan, G., Bopp, L., Canadell, J. G., Chevallier, F., Ciais, P., Ellis, R., Gloor, M., Peylin, P., Piao, S. L., Le Quéré, C., Smith, B., Zhu, Z., and Myneni, R.: Recent trends and drivers of regional sources and sinks of carbon dioxide, Biogeosciences, 12, 653–679, https://doi.org/10.5194/bg-12-653-2015, 2015.
Song, Z., Liu, H., Strömberg, C. A. E., Yang, X., and Zhang, X.: Phytolith carbon sequestration in global terrestrial biomes, Sci. Total Environ., 603/604, 502–509, https://doi.org/10.1016/j.scitotenv.2017.06.107, 2017.
Takata, K., Patra, P. K., Kotani, A., Mori, J., Belikov, D., Ichii, K., Saeki, T., Ohta, T., Saito, K., Ueyama, M., Ito, A., Maksyutov, S., Miyazaki, S., Burke, E. J., Ganshin, A., Iijima, Y., Ise, T., Machiya, H., Maximov, T. C., Niwa, Y., O'ishi, R., Park, H., Sasai, T., Sato, H., Tei, S., Zhuravlev, R., Machida, T., Sugimoto, A., and Aoki, S.: Reconstruction of top-down and bottom-up CO2 fluxes in Siberian larch forest, Environ. Res. Lett., 12, 125012, https://doi.org/10.1088/1748-9326/aa926d, 2017.
Thonicke, K., Venevsky, S., Sitch, S., and Cramer, W.: The role of fire disturbance for global vegetation dynamics: coupling fire into a Dynamic Global Vegetation Model, Global Ecol. Biogeogr., 10, 661–677, 2001.
Thurner, M., Beer, C., Ciais, P., Friend, A. D., Ito, A., Kleidon, A., Lomas, M. R., Quegan, S., Rademacher, T. T., Schaphoff, S., Tum, M., Wiltshire, A., and Carvalhais, N.: Evaluation of climate-related carbon turnover processes in global vegetation models for boreal and temperate forests, Glob. Change Biol., 23, 3076–3091, https://doi.org/10.1111/gcb.13660, 2017.
Tian, H., Lu, C., Yang, J., Banger, K., Huntzinger, D. N., Schwalm, C. R., Schwalm, C. R., Michalak, A. M., Cook, R., Ciais, P., Hayes, D., Huang, M., Ito, A., Jain, A., Lei, H., Mao, J., Pan, S., Post, W. M., Peng, S., Poulter, B., Ren, W., Ricciuto, D., Schaefer, K., Shi, X., Tao, B., Wang, W., Wei, Y., Yang, Q., Zhang, B., and Zeng, N.: Global patterns and controls of soil organic carbon dynamics as simulated by multiple terrestrial biosphere models: current status and future directions, Global Biogeochem. Cy., 29, 775–792, https://doi.org/10.1002/2014GB005021, 2015.
Todd-Brown, K. E. O., Randerson, J. T., Post, W. M., Hoffman, F. M., Tarnocai, C., Schuur, E. A. G., and Allison, S. D.: Causes of variation in soil carbon simulations from CMIP5 Earth system models and comparison with observations, Biogeosciences, 10, 1717–1736, https://doi.org/10.5194/bg-10-1717-2013, 2013.
Tramontana, G., Jung, M., Schwalm, C. R., Ichii, K., Camps-Valls, G., Ráduly, B., Reichstein, M., Arain, M. A., Cescatti, A., Kiely, G., Merbold, L., Serrano-Ortiz, P., Sickert, S., Wolf, S., and Papale, D.: Predicting carbon dioxide and energy fluxes across global FLUXNET sites with regression algorithms, Biogeosciences, 13, 4291–4313, https://doi.org/10.5194/bg-13-4291-2016, 2016.
United States Geological Survey (USGS): Global 30 Arc-Second Elevation (GTOPO30), EROS Data Center, Sioux Fall, South Dakota, https://doi.org/10.5066/F7DF6PQS, 1996.
van der Werf, G. R., Randerson, J. T., Giglio, L., van Leeuwen, T. T., Chen, Y., Rogers, B. M., Mu, M., van Marle, M. J. E., Morton, D. C., Collatz, G. J., Yokelson, R. J., and Kasibhatla, P. S.: Global fire emissions estimates during 1997–2016, Earth Syst. Sci. Data, 9, 697–720, https://doi.org/10.5194/essd-9-697-2017, 2017.
van Marle, M. J. E., Kloster, S., Magi, B. I., Marlon, J. R., Daniau, A.-L., Field, R. D., Arneth, A., Forrest, M., Hantson, S., Kehrwald, N. M., Knorr, W., Lasslop, G., Li, F., Mangeon, S., Yue, C., Kaiser, J. W., and van der Werf, G. R.: Historic global biomass burning emissions for CMIP6 (BB4CMIP) based on merging satellite observations with proxies and fire models (1750–2015), Geosci. Model Dev., 10, 3329–3357, https://doi.org/10.5194/gmd-10-3329-2017, 2017.
van Oost, K., Quine, T. A., Govers, G., De Gryze, S., Six, J., Harden, J. W., Ritchie, J. C., McCarty, G. W., Heckrath, G., Kosmas, C., Giraldez, J. V., Marques da Silva, J. R., and Merckx, R.: The impact of agricultural soil erosion on the global carbon cycle, Science, 318, 626–629, https://doi.org/10.1126/science.1145724, 2007.
Walter, B. P. and Heimann, M.: A process-based, climate-sensitive model to derive methane emissions from natural wetlands: application to five wetlands sites, sensitivity to model parameters, and climate, Global Biogeochem. Cy., 14, 745–765, 2000.
Webb, J. R., Santos, I. R., Maher, D. T., Macdonald, B., Robson, B., Isaac, P., and McHugh, I.: Terrestrial versus aquatic carbon fluxes in a subtropical agricultural floodplain over an annual cycle, Agr. Forest Meteorol., 260/261, 262–272, https://doi.org/10.1016/j.agrformet.2018.06.015, 2018.
Welp, L. R., Keeling, R. F., Meijer, H. A. J., Bollenbacher, A. F., Piper, S. C., Yoshimura, K., Francey, R. J., Allison, C. E., and Wahlen, M.: Interannual variability in the oxygen isotopes of atmospheric CO2 driven by El Niño, Nature, 477, 579–582, https://doi.org/10.1038/nature10421, 2011.
Wiedinmyer, C., Akagi, S. K., Yokelson, R. J., Emmons, L. K., Al-Saadi, J. A., Orlando, J. J., and Soja, A. J.: The Fire INventory from NCAR (FINN): a high resolution global model to estimate the emissions from open burning, Geosci. Model Dev., 4, 625–641, https://doi.org/10.5194/gmd-4-625-2011, 2011.
Winjum, J. K., Brown, S., and Schlamadinger, B.: Forest harvests and wood products: Sources and sinks of atmospheric carbon dioxide, Forest Sci., 44, 272–284, 1998.
Wolf, J., West, T. O., Le Page, Y., Kyle, G. P., Zhang, X., Collatz, G. J., and Imhoff, M. L.: Biogenic carbon fluxes from global agricultural production and consumption, Global Biogeochem. Cy., 29, 1617–1639, https://doi.org/10.1002/2015GB005119, 2015.
Xi, F., Davis, S. J., Ciais, P., Carwford-Brown, D., Guan, D., Pade, C., Shi, T., Syddall, M., Lv, J., Ji, L., Bing, L., Wang, J., Wei, W., Yang, K.-H., Lagerblad, B., Galan, I., Andrade, C., Zhang, Y., and Liu, Z.: Substantial global carbon uptake by cement carbonation, Nat. Geosci., 9, 880–883, https://doi.org/10.1038/NGEO2840, 2016.
Yang, D., Kanae, S., Oki, T., Koike, T., and Musiake, K.: Global potential soil erosion with reference to land use and climate changes, Hydrol. Process., 17, 2913–2928, 2003.
Yue, C., Ciais, P., Cadule, P., Thonicke, K., and van Leeuwen, T. T.: Modelling the role of fires in the terrestrial carbon balance by incorporating SPITFIRE into the global vegetation model ORCHIDEE – Part 2: Carbon emissions and the role of fires in the global carbon balance, Geosci. Model Dev., 8, 1321–1338, https://doi.org/10.5194/gmd-8-1321-2015, 2015.
Zhang, H., Liu, S., Yuan, W., Dong, W., Ye, A., Xie, X., Chen, Y., Liu, D., Cia, W., and Mao, Y.: Inclusion of soil carbon lateral movement alters terrestrial carbon budget in China, Sci. Rep., 4, 7247, https://doi.org/10.1038/srep07247, 2014.
Zhao, M., Running, S. W., and Nemani, R. R.: Sensitivity of Moderate resolution Imaging Spectrometer (MODIS) terrestrial primary production to the accuracy of meteorological reanalysis, J. Geophys. Res., 111, G01002, https://doi.org/10.1029/2004JG000004, 2006.
Zscheischler, J., Mahecha, M. D., Avitabile, V., Calle, L., Carvalhais, N., Ciais, P., Gans, F., Gruber, N., Hartmann, J., Herold, M., Ichii, K., Jung, M., Landschützer, P., Laruelle, G. G., Lauerwald, R., Papale, D., Peylin, P., Poulter, B., Ray, D., Regnier, P., Rödenbeck, C., Roman-Cuesta, R. M., Schwalm, C., Tramontana, G., Tyukavina, A., Valentini, R., van der Werf, G., West, T. O., Wolf, J. E., and Reichstein, M.: Reviews and syntheses: An empirical spatiotemporal description of the global surface–atmosphere carbon fluxes: opportunities and data limitations, Biogeosciences, 14, 3685–3703, https://doi.org/10.5194/bg-14-3685-2017, 2017.