the Creative Commons Attribution 4.0 License.
the Creative Commons Attribution 4.0 License.
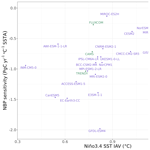
Compensatory effects conceal large uncertainties in the modelled processes behind the relationship between the El Niño–Southern Oscillation (ENSO) and CO2
Ana Bastos
Tatiana Ilyina
A large fraction of the interannual variation in the global carbon cycle can be explained and predicted by the impact of the El Niño–Southern Oscillation (ENSO) on net biome production (NBP). It is therefore crucial that the relationship between ENSO and NBP is correctly represented in Earth system models (ESMs). In this work, we look beyond the top-down ENSO–CO2 relationship by describing the characteristic ENSO–NBP pathways in 22 Coupled Model Intercomparison Project Phase 6 (CMIP6) ESMs. These pathways result from the configuration of three interacting processes that contribute to the overall ENSO–CO2 relationship: ENSO strength, ENSO-induced climate anomalies, and the sensitivity of NBP to climate. The analysed ESMs agree on the direction of the sensitivity of global NBP to ENSO but exhibit very high uncertainty with regard to its magnitude, with a global NBP anomaly of −0.15 to −2.13 Pg C yr−1 per standardised El Niño event. The largest source of uncertainty lies in the differences in the sensitivity of NBP to climate. This uncertainty among the ESMs increases even further when only the differences in NBP sensitivity to climate are considered. This is because differences in the climate sensitivity of NBP are partially compensated for by ENSO strength. A similar phenomenon occurs regarding the distribution of ENSO-induced climate anomalies. We show that even models that agree on global NBP anomalies exhibit strong disagreement with regard to the contributions of different regions to the global anomaly. This analysis shows that while ESMs can have a comparable ENSO-induced CO2 anomaly, the carbon fluxes contributing to this anomaly originate from different regions and are caused by different drivers. These alternative ENSO–NBP pathways can lead to a false confidence in the reproduction of CO2 by assimilating the ocean and the dismissal of predictive performance offered through ENSO. We suggest improving the underlying processes by using large-scale carbon flux data for model tuning in order to capture the ENSO-induced NBP anomaly patterns. The increasing availability of carbon flux data from atmospheric inversions and remote sensing products makes this a tangible goal that could lead to a better representation of the processes driving interannual variability in the global carbon cycle.
- Article
(2587 KB) - Full-text XML
-
Supplement
(3714 KB) - BibTeX
- EndNote
The relationship between the El Niño–Southern Oscillation (ENSO) and atmospheric CO2 observations at Mauna Loa was first reported by Bacastow (1976). Altered atmospheric circulation patterns during El Niño events cause warm and dry conditions across the tropics, leading to a reduction in net biome production (NBP) due to reduced net primary productivity (NPP) and increased or decreased heterotrophic respiration (Rh) (Qian et al., 2008; Bastos et al., 2018). ENSO-induced climate anomalies have a significant impact on the gross primary productivity (GPP) of 32 % of the vegetated land area and can explain up to 26 % of the interannual variation in global GPP (Zhang et al., 2019). Some El Niño events can be severe enough to turn the Amazon Basin, a carbon sink of global importance, into a net carbon source (Tian et al., 1998).
However, ENSO not only explains a large fraction of the NBP variability but also is the main source of seasonal-to-decadal predictability in the Earth system (Manzanas et al., 2014; Zeng et al., 2008; Spring and Ilyina, 2020; Li et al., 2022). Tropical carbon flux anomalies lag behind ENSO by 3 to 6 months (Zhu et al., 2017; Wang et al., 2016), meaning that even without further knowledge of the evolution of ENSO, near-term carbon flux variability can be anticipated based on present ENSO conditions. On top of this lag effect, Earth system model (ESM) simulations starting in winter can predict ENSO conditions for up to 1 year (Barnston et al., 2019).
Further predictability is added to the system by the land surface, which prolongs the ENSO-induced climate anomalies. The larger the anomaly, the longer it takes for soil moisture and conditions to return to normal, and ENSO years are often among the most extreme years of variability (Holmgren et al., 2001). Even longer predictability mechanisms might be triggered through vegetation dynamics (Holmgren et al., 2001). This can happen in dry years through the lasting impacts of defoliation and tree mortality (Wigneron et al., 2020; Santos et al., 2018) or through wildfires, which require decades of recovery (Silva et al., 2018). Wet events, on the other hand, can provide long-term predictability as these events drive plant recruitment in semi-arid ecosystems (Holmgren et al., 2001). Extreme events play a crucial role in the vegetation dynamics of these ecosystems, where the establishment of trees and shrubs needs sustained wet conditions (Chang-Yang et al., 2016; López et al., 2006).
Because ENSO plays such a large role in the variability and predictability of NBP, the correct representation of related processes in ESMs is especially important. Three key processes that explain the relationship between ENSO and NBP can be arranged hierarchically. At the highest level of this hierarchy is the strength of the ENSO events. Despite considerable advancements in our understanding of ENSO dynamics, there remains a wide range of simulated ENSO amplitudes in ESMs (Beobide-Arsuaga et al., 2021). The amplitude of ENSO can be measured as the standard deviation (SD) of sea surface temperature anomalies (SSTAs) in the Niño3.4 region (5° N–5° S, 170–120° W) and ranges from 0.4 to 1.4 °C in models from the sixth phase of the Coupled Model Intercomparison Project (CMIP6) (Brown et al., 2020; Beobide-Arsuaga et al., 2021; Cai et al., 2022).
The second process that explains the impact of ENSO on NBP involves ENSO-induced climate anomalies. Most ENSO teleconnections are caused by a reorganisation of tropical convection patterns (Perry et al., 2020). These patterns create temperature and precipitation anomalies in northern South America, Southeast Asia, and northern Australia. However, ENSO-induced changes in the upper atmosphere can create Rossby waves that propagate polewards and lead to climate anomalies in the midlatitudes. ENSO teleconnection strengths show high uncertainty among CMIP5 models, with an average correlation with observed teleconnection patterns of 0.7 for temperature and 0.46 for precipitation (Perry et al., 2020). Although the representation of the relationship between ENSO and tropical precipitation improved from CMIP5 to CMIP6, there are still considerable deviations from the observed relationship (Yang and Huang, 2022). The impact of ENSO on eastern Asian summer rainfall, for example, can only be captured by 11 out of 20 CMIP6 models (Fu et al., 2021).
The last stage within the hierarchy of processes that shape the ENSO–NBP relationship involves differences in biogeochemistry – specifically, the sensitivity of NBP to local climatic anomalies caused by ENSO. Due to limitations in carbon flux observations and the covariability of climatic conditions, the contributions of temperature and moisture to driving the carbon cycle remain a debated topic in the literature (Piao et al., 2020). This uncertainty can be demonstrated by the sensitivity of the atmospheric CO2 growth rate to tropical temperatures from reanalysis data, where determined sensitivities differ by a factor of 2 (Piao et al., 2020). Regional differences in the sensitivity of carbon fluxes to climate depend on the ecosystem type and climate. Semi-arid ecosystems and tropical forests, which cover most of the land area affected by ENSO, show the highest sensitivity to climate variability (Bastos et al., 2013; Poulter et al., 2014; Ahlström et al., 2015; O'Sullivan et al., 2020). However, these biomes are also where carbon flux sensitivities have the highest inter-model spread and bias relative to observations (O'Sullivan et al., 2020; Koirala et al., 2022).
While the land carbon sink variability in ESMs can be constrained using the sensitivity of the entire tropical carbon sink to ENSO-driven climate anomalies (Cox et al., 2013; Zechlau et al., 2022), we aim to test whether a spatially explicit constraint is feasible. The aim of this study is to look beyond the relationship between ENSO and the global atmospheric CO2 growth rate in ESMs and reveal the sources of uncertainty in the ENSO–NBP relationship. We quantify the specific ENSO–NBP pathways that describe the locations and drivers of the ENSO-induced NBP anomalies. These pathways are characterised by three main processes that shape the ENSO–NBP relationship: ENSO strength, ENSO-induced climate anomalies, and biogeochemistry. We quantify how much the uncertainties in these three processes contribute to the uncertainty in the ENSO–NBP relationship among the ESMs and compare the ESMs with observations.
Ziehn et al. (2020)Shi et al. (2020)Wu et al. (2019)Swart et al. (2019)Danabasoglu et al. (2020)Lovato et al. (2022)Cherchi et al. (2019)Séférian et al. (2019)Golaz et al. (2019)Döscher et al. (2022)Dunne et al. (2020)Orbe et al. (2020)Volodin et al. (2018)Boucher et al. (2020)Watanabe et al. (2021)Hajima et al. (2020)Mauritsen et al. (2019)Yukimoto et al. (2019)Bethke et al. (2021)Seland et al. (2020)Seland et al. (2020)Sellar et al. (2019)2.1 Data
We measure the interactions between ENSO, temperature, precipitation, and NBP across 22 CMIP6 ESMs and compare them with observation-based data sources. In order to have a large sample size of ENSO conditions, we use unforced pre-industrial control (piControl) simulations. The analysed ESMs, their variant labels, and the simulation lengths are listed in Table 1. We decompose the NBP anomalies into the following components:
Because some ESMs simulate types of disturbances other than fires, Eq. (1) does not lead to an exact reproduction of NBP but provides an approximate value for the contribution of the large carbon fluxes to the land–air CO2 exchange. Fire emissions are only available for 13 of the analysed ESMs. Although CMCC-CM2-SR5 simulates fire emissions, the data are not available online. Instead, we calculate fire emissions for CMCC-CM2-SR5 by inverting the mass balance in Eq. (1).
The carbon fluxes of the ESMs are compared with two observation-based data sets, referred to as observations here. These are land–atmosphere carbon fluxes from the Copernicus Atmosphere Monitoring Service (CAMS) (Chevallier et al., 2005), an atmospheric inversion product, and net ecosystem exchange (NEE) from upscaled flux tower measurements (FLUXCOM version: ANN.CRUNCEPv6; Jung et al., 2019). Although NEE includes land–atmosphere carbon fluxes that are not part of NBP (Ciais et al., 2022), such as geological CO2 release and lateral fluxes, these fluxes only play a minor role in the global carbon cycle and are unlikely to significantly affect the sensitivity to ENSO (Canadell et al., 2021). We also compare the carbon fluxes to NBP from the TRENDY v6 ensemble of land surface models (Sitch et al., 2015). This data product contains the results of land surface models that are also components of the ESMs used in this work. Due to this dependence, we do not treat the TRENDY results as observations. To account for uncertainty in meteorological data, we use different reanalysis products. We use sea surface temperature (SST) reanalysis data (Hadley Centre Sea Ice and Sea Surface Temperature (HadISST); Rayner et al., 2003); temperatures from the ERA-Interim reanalysis (1979–2018; Dee et al., 2011); version 4 of the Climatic Research Unit gridded Time Series (CRU TS v4 (1959–2019); Harris et al., 2020); the Japanese 55-year Reanalysis (JRA-55 (1958–2019); Kobayashi et al., 2015); version 2 of the Modern-Era Retrospective analysis for Research and Applications (MERRA-2 (1980–2021); Gelaro et al., 2017); the National Centers for Environmental Prediction–National Center for Atmospheric Research (NCEP–NCAR) 40-Year Reanalysis Project (1948–2021; Kalnay et al., 1996); bias-adjusted ERA5 reanalysis data (WFDE5 (1979–2018); Cucchi et al., 2020); and precipitation data from the ERA-Interim reanalysis, JRA-55, MERRA2, the NCEP–NCAR 40-Year Reanalysis Project, and the Rainfall Estimates on a Gridded Network (REGEN) data set (1950–2016; Contractor et al., 2020).
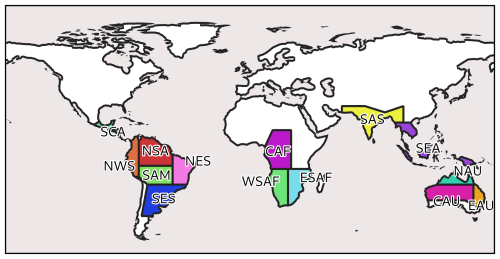
Figure 1The 14 Intergovernmental Panel on Climate Change (IPCC) climate reference regions with the largest ENSO-induced NBP anomalies: SE Asia (SEA), northern South America (NSA), NE South America (NES), eastern southern Africa (ESAF), the South American monsoon (SAM) region, northern Australia (NAU), NW South America (NWS), central Africa (CAF), South Asia (SAS), central Australia (CAU), eastern Australia (EAU), southern Central America (SCA), western southern Africa (WSAF), and SE South America (SES).
2.2 Processing and analysis
We calculate the annual anomalies in Niño3.4 SST, temperature, precipitation, and carbon fluxes for the analysis. In this study, we base annual averages on a time window from July to June of the following year, rather than on a window from January to December. We use this definition of a year to better capture distinct ENSO events. ENSO SSTAs usually peak during boreal winter, and warm El Niño events are often followed by a cold La Niña event (An and Kim, 2017). Years starting in January are, therefore, not centred around event peaks and could thus contain the tail of an El Niño event and the beginning of a La Niña event. Annual anomalies in the data are obtained by subtracting the climatology from the ESM data and removing the linear trend from the observational products. ENSO strength is calculated as the SD of annual SSTAs for the Niño3.4 region. NBP and climate anomalies are aggregated into regional averages using the boundaries of the updated Intergovernmental Panel on Climate Change (IPCC) reference regions (Iturbide et al., 2020). For this analysis, we only focus on the regions with the strongest ENSO–NBP relationships. These regions are identified by averaging the ENSO-induced NBP anomalies across all data sources and selecting the 14 regions with the highest absolute ENSO-induced NBP anomalies (Fig. 1). We calculate the ENSO-induced climate and carbon anomalies using the coefficients of a linear regression model with a zero intercept:
where ΔXijp represents the annual anomaly, ΔENSOjp represents the annual mean Niño3.4 SSTA, the regression coefficient () corresponds to the region (, i.e. all IPCC regions with land surface) and data source (, i.e. 22 ESMs and three observational NBP products using an ERA-Interim climate), and p represents the year. Although El Niño and La Niña events do not produce entirely symmetrical responses in the atmosphere and the land system, we use this method for the sake of simplified results, assuming that El Niño and La Niña events are similar.
We describe the ENSO–NBP pathways using three distinct processes: ENSO strength, ENSO-induced climate anomalies, and NBP sensitivity to climate (biogeochemistry). To quantify these pathways, we calculate the ENSO-induced NBP anomalies due to the differences in each of these processes (ΔNBPENSO, ΔNBPCLIM, and ΔNBPBIO). This is done by considering the differences in only one of the processes at a time while using the mean conditions across all ESMs for the other processes. To compare the effects of similar ENSO events across the ESMs, we calculate the 90th percentile of Niño3.4 SSTAs for each ESM and for HadISST. These standardised ENSO events are referred to as “ENSO-90” here and have a return interval of around 11 years. We calculate ΔNBPENSO by multiplying the mean global NBP sensitivity to ENSO () with the ENSO-90 SSTA (ΔENSOj90) values for each model:
The differences due to ENSO-induced climate anomalies and NBP sensitivity to climate are assessed by fitting a multiple linear regression model of NBP (MLRNBP) for each region and data source. These models predict NBP based on annual temperature and precipitation anomalies as follows:
where ΔNBPijp represents the NBP anomaly and its sensitivity to temperature (precipitation) is denoted by (). To assess the differences due to ENSO-induced climate anomalies, we use MLRNBP with temperature and precipitation anomalies from an ENSO-90 event for each ESM and averaged sensitivity values across all ESMs:
Conversely, the differences due to biogeochemistry are calculated using temperature and precipitation anomalies from an ENSO-90 event averaged across all ESMs with the following model-specific NBP sensitivities to climate:
We compare the contributions of the three processes to the overall uncertainty by measuring the spread of the ENSO–NBP relationship among the ESMs as the coefficient of variation (CV), i.e. the ratio of the standard deviation to the mean (expressed as a percentage).
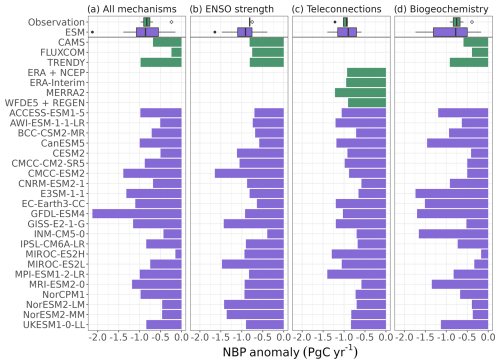
Figure 2ENSO-induced NBP anomalies across ESMs (green) and observational products (purple) for (a) an El Niño event with a 90th-percentile intensity (CV of 48 % (39 % without GFDL-ESM4)), as well as the contributions of three processes to differences in the ENSO–NBP relationship: (b) differences in ENSO strength (CV of 32 %), (c) differences in ENSO-induced climate anomaly patterns (teleconnections (CV of 27 %)), and (d) differences in biogeochemistry (CV of 56 %). Results from the TRENDY ensemble are denoted as diamonds.
3.1 Global ENSO–NBP relationship
The NBP anomalies of a standardised ENSO-90 event range from −0.15 to −2.13 Pg C yr−1, with a CV of 48 %, which we use as the reference value for the uncertainty in the ENSO–NBP relationship (Fig. 2a). The mean ESM ENSO-90 NBP anomaly has a value of −0.88 Pg C yr−1, which is between the CAMS and TRENDY values. The mean ENSO-90 NBP anomaly from the observations is pulled down by FLUXCOM, which is reported to underestimate the interannual variability in carbon fluxes (Jung et al., 2019). There is strong disagreement regarding the regional contribution to global NBP anomalies (Fig. 3). This disagreement is exemplified in SE Asia (SEA) and northern South America (NSA), the two regions contributing the most to global NBP anomalies. The combined NBP anomalies of these two regions account for 48 % of the global NBP anomalies across all ESMs. However, the global contribution of SEA and NSA ranges from −23 % in MIROC-ES2H (unlike the other ESMs, MICRO-ES2H has positive NBP anomalies in SEA during El Niño events), to 70 % in NorESM2-LM. There is also little agreement regarding the ratio of SEA to NSA anomalies. Although the mean NBP anomaly from SEA is 21 % larger than the mean anomaly from NSA, half of the 22 ESMs have larger anomalies in NSA than in SEA.
Although the method used here does not take the asymmetry of ENSO events into account, we found this effect to be negligible on the global scale for most ESMs (Fig. S1 in the Supplement).
3.2 ENSO strength
ENSO strength varies between 0.35 °C (INM-CM5-0) and 1.39 °C (CMCC-ESM2), while the ESM mean (0.86 °C) is slightly higher than that of the HadISST reanalysis (0.76 °C; Fig. 4). Despite the wide variation in ENSO strength, these differences are partially offset by the sensitivity of global NBP to ENSO. ESMs with strong ENSO values tend to have a lower NBP sensitivity to Niño3.4 SSTAs.
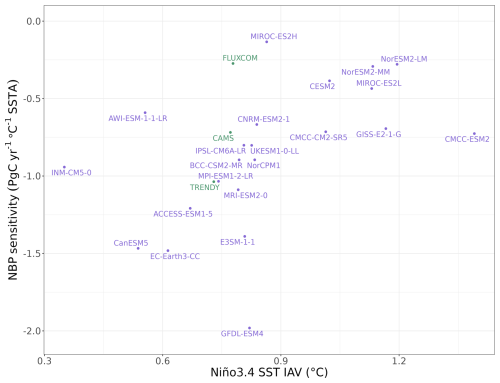
Figure 4ENSO strength and the sensitivity of global NBP to ENSO in 17 ESMs, FLUXCOM, CAMS, and TRENDY. The strength of the ENSO events is given as the SD of the mean annual sea surface temperatures in the Niño3.4 region (x axis). NBP sensitivity to ENSO is given as the global NBP anomaly relative to a 1 °C anomaly in the Niño3.4 region (y axis). A correlation of 0.46 between ENSO amplitude and NBP sensitivity to ENSO compensates for some of the differences between ESMs. IAV: interannual variability.
To single out the effect of the differences in ENSO strength, we multiply the ENSO-90 SSTAs by the mean NBP sensitivity (−0.86 Pg C yr−1 °C−1). The resulting ENSO-induced NBP anomalies range from −0.39 to −1.64 Pg C yr−1. Considering only the differences in ENSO strength leads to a CV of 32 %, which is a 33 % reduction compared to the overall ENSO–NBP CV (Fig. 2b).
3.3 ENSO-induced climate anomalies
We compare the regional patterns of ENSO-induced climate anomalies between ESMs and observations and assess how these differences affect the ENSO–NBP relationship. The ESMs generally capture the sign and strength of ENSO-induced temperature and precipitation anomalies (Figs. S2 and S3). There are, however, regional differences in the uncertainty in climate anomalies among the ESMs. The spread in ENSO-induced temperature anomalies is especially high in central Australia (CAU), northern Australia (NAU), and NSA, and there are high uncertainties in the ENSO-induced precipitation anomalies in SEA and NW South America (NWS).
We use a statistical model with uniform NBP sensitivities to determine the effect of differences in ENSO-induced climate anomaly patterns on NBP (Figs. 2c and S4). Differences in ENSO-induced climate anomalies contribute less to the uncertainty in the ENSO–NBP relationship than the other two processes (CV of 27 %). Most of the global NBP anomalies fall within a similar range, except those from MPI-ESM1-2-LR. The above-average NBP anomalies in MPI-ESM1-2-LR are caused by strong ENSO-induced temperature anomalies in several regions (Fig. S2).
The comparison of the data sources used has some limitations because the reanalysis-based observation data include climate forcing, while the ESM data from piControl runs do not. However, the measured changes to ENSO teleconnection patterns remain weak and are only expected to alter by the mid-21st century (Yeh et al., 2018).
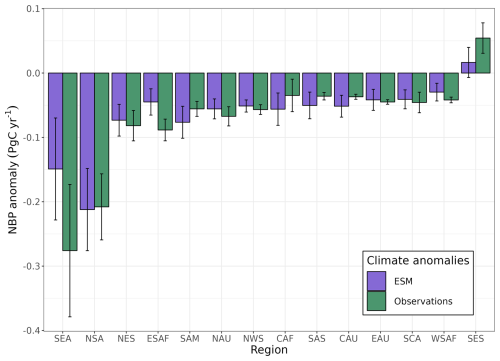
Figure 5Differences in regional NBP anomalies based on ENSO-induced climate anomalies from ESMs and observations.
We find some notable biases in the ENSO-induced climate anomalies among the ESMs. Figure 5 shows the ENSO-90 NBP anomalies from the MLRNBP model using mean values for NBP sensitivity and ENSO-induced climate anomalies from ESMs and observations. The strongest biases are found in SEA, where the observed ENSO-induced climate anomalies result in NBP anomalies almost twice as strong as those in the ESM climate. This bias is mostly due to stronger ENSO-induced precipitation anomalies in the observations, especially in MERRA2 (Fig. S3). Other areas with biases include eastern southern Africa (ESAF) and SE South America (SES), where ENSO-induced NBP anomalies are stronger in the observed climate than in the ESM climate, as well as the South American monsoon (SAM) region and central Africa (CAF), where the ESM climate creates stronger NBP anomalies than the observed climate.
3.4 Biogeochemistry
Differences in biogeochemistry lead to a CV of 56 %, representing a 17 % increase compared to the differences in the overall ENSO–NBP relationship (Fig. 2d). This makes biogeochemistry the largest source of uncertainty in the ENSO–NBP relationship. Indeed, considering only differences in biogeochemistry produces a larger uncertainty than the apparent uncertainty in the ENSO–NBP relationship. This highlights the compensatory effects that offset some of the differences among the ESMs.
We decomposed the ENSO-90 NBP anomalies into NPP, Rh, and fire emissions to reveal the factors driving the differences in biogeochemistry (Fig. 6). Global ENSO-induced NPP anomalies are relatively consistent, except in the MIROC ESMs. Fire emissions make up 43 % and 32 % of the ENSO-induced NBP anomalies in E3SM-1-1 and NorCPM1, respectively, while in 7 out of the 13 ESMs (with fire emissions), fire explains fewer than 4 % of the ENSO-induced NBP anomalies. ENSO-induced fire emissions originate mostly from SEA. Notable deviations among the NBP anomalies are due to uncertainties in the sign of Rh. Rh anomalies can either increase or dampen the effect of reduced NPP. In particular, the high NBP anomalies in GFDL-ESM4 result from increased Rh, most of which originates from SEA. However, there is no consistency in the role of Rh with respect to ENSO-induced NBP anomalies. While GFDL-ESM4 and UKESM1-0-LL have comparable NPP anomalies for SEA, NBP anomalies are 20 times higher in GFDL-ESM4 because the NPP anomalies in UKESM1-0-LL are offset by Rh. This demonstrates that while the NBP sensitivity to climate is suitable for describing CO2 dynamics, it fails to capture the underlying processes.
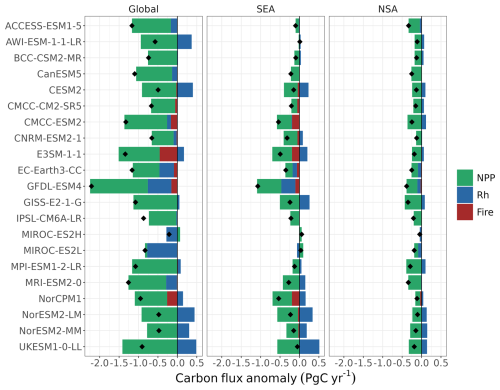
Figure 6The decomposition of ENSO-induced NBP fluxes (diamonds) into net primary production (NPP), heterotrophic respiration (Rh), and fire for global fluxes, Southeast Asia (SEA), and northern South America (NSA). The anomalies represent the carbon fluxes of an ENSO event with a 90th-percentile intensity. Negative values indicate reduced NPP and increased Rh and fire emissions.
3.5 SEA and NSA
Since the majority of the ENSO-induced NBP anomalies originate from SEA and NSA, we will examine the cause of these anomalies in more detail. Figure 7 shows the ENSO-induced precipitation anomalies and the sensitivity of NBP to precipitation for these two regions. The NBP anomalies in SEA are less constrained than those in NSA due to both the uncertainty in the ENSO-induced precipitation anomalies and the sensitivity of NBP to precipitation. While the differences in the ENSO-induced climate anomalies have a small impact on the uncertainty in the global ENSO–NBP relationship, we find large uncertainties on a regional scale. The ENSO-induced precipitation anomalies range in value from 100 to −210 mm yr−1 in SEA and from −33 to −180 mm yr−1 in NSA.
Although the ENSO-induced NBP anomalies from IPSL-CM6A-LR for SEA reflect the mean NBP anomalies from the other ESMs (Fig. 3), they result from the compensation of two anomalous behaviours. In IPSL-CM6A-LR, unlike in most ESMs, ENSO creates positive precipitation anomalies in SEA. However, this atypical behaviour is cancelled out by the negative sensitivity of NBP to precipitation.
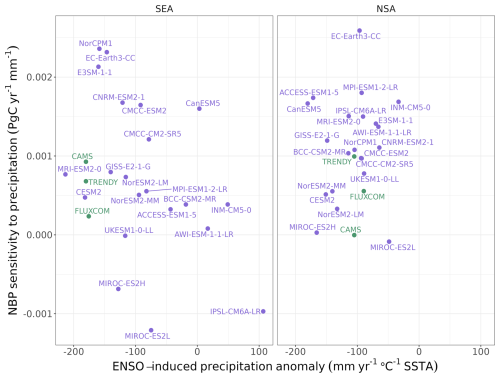
Figure 7Compositions of ENSO-induced NBP anomalies with respect to SE Asia (SEA) and northern South America (NSA). The x axis shows the ENSO-induced precipitation anomalies, and the y axis shows the sensitivity of NBP to precipitation.
These results highlight the need to describe the complete ENSO–NBP pathway to fully understand the relationship between ENSO and CO2. The pathways describe not only the strength but also the where and why of the ENSO–NBP relationship. We demonstrate the extent of these differences qualitatively by comparing the ENSO–NBP pathways of the four ESMs that are most similar in terms of apparent ENSO–NBP strength (Table 2). The ENSO-90 NBP anomalies of these four ESMs fall within a very narrow range of −0.97 to −0.99 Pg C, which encompasses the TRENDY anomaly (−0.98 Pg C). Within these four ESMs, we focus on the role of SEA as this is the region with the strongest ENSO-induced NBP fluxes. The share of SEA in the global NBP anomaly is 9 % in ACCESS-ESM1-5, 12 % in MPI-ESM1-2-LR, 19 % in CanESM5, and 56 % in NorCPM1. We further use the MLRNBP model to separate the SEA NBP anomalies into components caused by either temperature or precipitation. Temperature anomalies explain 74 % and 86 % of the NBP anomalies in MPI-ESM1-2-LR and ACCESS-ESM1-5, respectively. While the high NBP anomaly in NorCPM1 is exclusively caused by precipitation, the positive precipitation anomaly in CanESM5 even mitigates the overall NBP anomaly caused by temperature. Lastly, we break down whether the temperature-related NBP anomalies are caused by strong ENSO-related temperature anomalies or by a high sensitivity of NBP to temperature. Although CanESM5 has by far the highest temperature-related NBP anomalies, the actual temperature anomalies are 2 and 4 times smaller than those in ACCESS-ESM1-5 and MPI-ESM1-2-LR, respectively. The temperature-related NBP anomalies in CanESM5 can be attributed to the high sensitivity of NBP to temperature. The remaining ESMs, ACCESS-ESM1-5 and MPI-ESM1-2-LR, are the most similar in terms of temperature-related NBP anomalies. However, this is because the 2.5-times-higher ENSO-induced temperature anomalies in MPI-ESM1-2-LR are somewhat compensated for by differences in the sensitivity of NBP to temperature.
Table 2Differences in the ENSO-induced NBP anomaly pathways for four ESMs. All models have an ENSO-induced NBP anomaly of around −0.98 Pg C yr−1 per standardised El Niño event. SEA NBP refers to the ENSO-induced NBP anomaly originating from Southeast Asia (SEA), Tfrac represents the fraction of NBP anomalies in SEA that can be attributed to temperature, ΔTemp denotes the ENSO-induced temperature anomaly, and βNT represents the sensitivity of NBP to temperature.
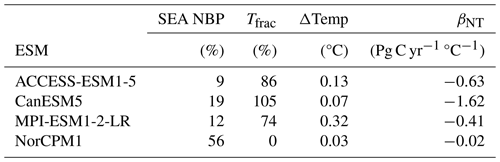
We compared the strength and characteristics of the ENSO–NBP relationship across 22 CMIP6 ESMs. The largest source of uncertainty in the simulated ENSO–NBP relationship is due to differences in biogeochemistry. Although differences in ENSO-induced climate anomalies are strong at the regional scale, these errors cancel out globally.
The sensitivity of NBP to Niño3.4 SSTAs is still poorly constrained, with SSTA values ranging from −0.13 to −2.00 Pg C °C−1 (with an SD of 0.44 Pg C °C−1). A possible explanation for the negative relationship between ENSO strength and the sensitivity of global NBP to Niño3.4 SSTAs could be an artefact of model tuning. Although we could not find direct evidence that the climate sensitivity of the terrestrial carbon cycle is directly tuned to ENSO, it is possible that this happens indirectly by constraining the overall variability in atmospheric CO2. Some of the spread in the sensitivity of NBP to Niño3.4 SSTAs can be explained by differences in individual processes, such as ENSO, or the overall sensitivity of carbon fluxes to climatic drivers (Padrón et al., 2022). Although this type of error leads to a large uncertainty in the ENSO–NBP relationship, the errors introduced by a single process do not compromise the consistency of the results. Differences in ENSO strength, for example, could be balanced out with a single scaling factor.
Another type of error is based on differences in the ENSO–NBP pathways. The combined differences in ENSO-induced climate anomaly patterns and biogeochemistry lead to high uncertainty in the processes behind the ENSO–NBP relationship. Our exemplified descriptions of four ENSO–NBP pathways show that there is little agreement regarding the origin and drivers of ENSO-induced NBP anomalies, even if they result in similar CO2 growth. The disagreements in the pathways primarily affect the estimation of regional carbon dynamics. However, the differences in the ENSO–NBP pathways can also distort the prediction of atmospheric CO2 in initialised prediction systems. This results from the interaction of initial conditions and ESM-specific ENSO teleconnection patterns. Although ESMs might exhibit comparable relationships between ENSO and global NBP, a specific ENSO event can still result in different NBP anomalies. This can be exemplified by the pathways of ACCESS-ESM1-5 and NorCPM1 (Table 2). While both ESMs exhibit a similar average response to ENSO, the NBP anomaly is almost exclusively from SEA in NorCPM1; however, SEA does not play a large role in ACCESS-ESM1-5. Consequentially, the initial conditions and large-scale weather patterns that influence SEA interact with the ENSO-induced climate anomalies in SEA and co-determine the global NBP anomalies. An initial positive water storage anomaly in NorCMP1, for example, can mitigate the impact of reduced precipitation in SEA and limit the reduction in global NBP.
The main challenge in improving the representation of the ENSO–NBP relationship is addressing the errors in the biogeochemistry. The differences in the sensitivity of NBP to climate drive the uncertainty in the overall ENSO–NBP relationship. A large portion of this uncertainty can be attributed to the partitioning of NBP. While there is some deviation in the climate sensitivity of NPP, there is no consensus on the sign of Rh sensitivity. Under normal circumstances, NPP and Rh are positively correlated (Baldocchi et al., 2018) because, firstly, similar climatic conditions favour both types of fluxes and, secondly, the organic material required for Rh is provided through NPP. An exception to this behaviour was proposed by Zeng et al. (2005) and results from a “conspiracy” between ENSO-induced climate anomalies and plant and soil physiology. Increased temperatures and reduced precipitation during El Niño events in the tropics can limit NPP while enhancing Rh. However, this additive effect of NPP and Rh is only observed in SEA in GFDL-ESM4 and EC-Earth3-CC. In most other ESMs, the ENSO-induced reduction in NPP is partially compensated for by the negative Rh anomaly.
A smaller but still significant portion of the uncertainty can be attributed to ENSO-induced climate anomalies. While the extent of global climate anomalies does not vary much among the ESMs, there is high uncertainty in the spatial distribution of the ENSO-induced anomaly patterns. Although ENSO-induced climate anomalies affect several regions across the globe, they induce the strongest NBP anomalies in SEA and NSA. Important steps in model development include reflecting the observed balance of ENSO-induced climate anomalies for these regions and reducing the strong bias in climate anomalies in SEA.
The uncertainty among observed carbon fluxes is mostly due to the low interannual variability in NBP in FLUXCOM. Although FLUXCOM data are not suitable for estimating absolute ENSO-induced NBP anomalies, they can still be used to assess the relative contributions of individual regions to the global ENSO–NBP signal. The ENSO-induced NBP anomalies in CAMS are also below the mean ESM values. This generally lower response of land carbon fluxes to ENSO in inversion data, compared with ESMs, has been reported by others (Bastos et al., 2018, 2020). Although there are differences between inversion products based on atmospheric transport models, assimilated observations, prior carbon fluxes, and fossil fuel emission data (Gaubert et al., 2019), the differences among inversion products are small compared to the differences among ESMs (Bastos et al., 2020).
Although ESMs are able to reproduce the relationship between ENSO SSTAs and CO2, there is little agreement with regard to the processes behind this relationship. While some of the ESMs and the multi-model mean reflect the observed ENSO–NBP pathways well, individual ESMs deviate substantially. This is because uncertainties on regional scales are balanced out when fluxes are aggregated globally. Consequentially, the correct reproduction of atmospheric CO2 variability in assimilation runs forced by SSTAs does not necessarily indicate a good representation of atmospheric circulation patterns and biogeochemistry. It could also result from an alternative ENSO–NBP pathway that does not reflect observable processes. We attribute this high uncertainty in the ENSO–NBP relationship to our limited understanding of the sensitivity of terrestrial carbon fluxes to climate. This ongoing challenge is due to the low availability and quality of carbon flux observations, limiting the ability of ESMs to reproduce interannual variability in the terrestrial carbon cycle. This is, however, where the ENSO–NBP relationship provides untapped potential. Instead of tuning ESMs with local observations of carbon flux data, the models could be optimised to reproduce the large-scale ENSO-induced patterns. This could be a favourable alternative to the traditional approach due to the availability of high-accuracy data from atmospheric CO2 measurements and continental carbon flux anomalies from inversion products. Improving the reproduction of the regional response of terrestrial carbon fluxes to ENSO-induced climate anomalies is not only a tangible goal but also one that may lead to ESMs with both a better ability to simulate interannual variability in global carbon fluxes and improved predictability of the global carbon cycle.
The data and code for producing the figures shown in this study are available at https://doi.org/10.5281/zenodo.14070579 (Dunkl, 2024).
The supplement related to this article is available online at: https://doi.org/10.5194/esd-16-151-2025-supplement.
The study was conceptualised by ID, AB, and TI. ID developed the methodology, performed the analysis, and wrote the original draft. AB and TI reviewed and edited the paper.
The contact author has declared that none of the authors has any competing interests.
Publisher's note: Copernicus Publications remains neutral with regard to jurisdictional claims made in the text, published maps, institutional affiliations, or any other geographical representation in this paper. While Copernicus Publications makes every effort to include appropriate place names, the final responsibility lies with the authors.
The authors thank David Nielsen for the internal review of the paper.
This project has received funding from the European Union's Horizon 2020 research and innovation programme (4C project; grant no. 821003).
The article processing charges for this open-access publication were covered by the Max Planck Society.
This paper was edited by Richard Betts and reviewed by Chris Jones and one anonymous referee.
Ahlström, A., Raupach, M. R., Schurgers, G., Smith, B., Arneth, A., Jung, M., Reichstein, M., Canadell, J. G., Friedlingstein, P., Jain, A. K., Kato, E., Poulter, B., Sitch, S., Stocker, B. D., Viovy, N., Wang, Y. P., Wiltshire, A., Zaehle, S., and Zeng, N.: The dominant role of semi-arid ecosystems in the trend and variability of the land CO2 sink, Science, 6237, 895–899, https://doi.org/10.1126/science.aaa1668, 2015. a
An, S.-I. and Kim, J.-W.: Role of nonlinear ocean dynamic response to wind on the asymmetrical transition of El Niño and La Niña, Geophys. Res. Lett., 44, 393–400, https://doi.org/10.1002/2016GL071971, 2017. a
Bacastow, R. B.: Modulation of atmospheric carbon dioxide by the Southern Oscillation, Nature, 261, 116–118, https://doi.org/10.1038/261116a0, 1976. a
Baldocchi, D., Chu, H., and Reichstein, M.: Inter-annual variability of net and gross ecosystem carbon fluxes: A review, Agric. Forest Meteorol., 249, 520–533, https://doi.org/10.1016/j.agrformet.2017.05.015, 2018. a
Barnston, A. G., Tippett, M. K., Ranganathan, M., and L'Heureux, M. L.: Deterministic skill of ENSO predictions from the North American Multimodel Ensemble, Clim. Dynam., 53, 7215–7234, https://doi.org/10.1007/s00382-017-3603-3, 2019. a
Bastos, A., Running, S. W., Gouveia, C., and Trigo, R. M.: The global NPP dependence on ENSO: La Niña and the extraordinary year of 2011, J. Geophys. Res.-Biogeo., 118, 1247–1255, https://doi.org/10.1002/jgrg.20100, 2013. a
Bastos, A., Friedlingstein, P., Sitch, S., Chen, C., Mialon, A., Wigneron, J.-P., Arora, V. K., Briggs, P. R., Canadell, J. G., Ciais, P., Chevallier, F., Cheng, L., Delire, C., Haverd, V., Jain, A. K., Joos, F., Kato, E., Lienert, S., Lombardozzi, D., Melton, J. R., Myneni, R., Nabel, J. E. M. S., Pongratz, J., Poulter, B., Rödenbeck, C., Séférian, R., Tian, H., van Eck, C., Viovy, N., Vuichard, N., Walker, A. P., Wiltshire, A., Yang, J., Zaehle, S., Zeng, N., and Zhu, D.: Impact of the 2015/2016 El Niño on the terrestrial carbon cycle constrained by bottom-up and top-down approaches, Philos. T. Roy. Soc. B, 373, 20170304, https://doi.org/10.1098/rstb.2017.0304, 2018. a, b
Bastos, A., O'Sullivan, M., Ciais, P., Makowski, D., Sitch, S., Friedlingstein, P., Chevallier, F., Rödenbeck, C., Pongratz, J., Luijkx, I. T., Patra, P. K., Peylin, P., Canadell, J. G., Lauerwald, R., Li, W., Smith, N. E., Peters, W., Goll, D. S., Jain, A., Kato, E., Lienert, S., Lombardozzi, D. L., Haverd, V., Nabel, J. E. M. S., Poulter, B., Tian, H., Walker, A. P., and Zaehle, S.: Sources of Uncertainty in Regional and Global Terrestrial CO2 Exchange Estimates, Global Biogeochem. Cycles, 34, e2019GB006393, https://doi.org/10.1029/2019GB006393, 2020. a, b
Beobide-Arsuaga, G., Bayr, T., Reintges, A., and Latif, M.: Uncertainty of ENSO-amplitude projections in CMIP5 and CMIP6 models, Clim. Dynam., 56, 3875–3888, https://doi.org/10.1007/s00382-021-05673-4, 2021. a, b
Bethke, I., Wang, Y., Counillon, F., Keenlyside, N., Kimmritz, M., Fransner, F., Samuelsen, A., Langehaug, H., Svendsen, L., Chiu, P.-G., Passos, L., Bentsen, M., Guo, C., Gupta, A., Tjiputra, J., Kirkevåg, A., Olivié, D., Seland, Ø., Solsvik Vågane, J., Fan, Y., and Eldevik, T.: NorCPM1 and its contribution to CMIP6 DCPP, Geosci. Model Dev., 14, 7073–7116, https://doi.org/10.5194/gmd-14-7073-2021, 2021. a
Boucher, O., Servonnat, J., Albright, A. L., Aumont, O., Balkanski, Y., Bastrikov, V., Bekki, S., Bonnet, R., Bony, S., Bopp, L., Braconnot, P., Brockmann, P., Cadule, P., Caubel, A., Cheruy, F., Codron, F., Cozic, A., Cugnet, D., D'Andrea, F., Davini, P., Lavergne, C. d., Denvil, S., Deshayes, J., Devilliers, M., Ducharne, A., Dufresne, J.-L., Dupont, E., Éthé, C., Fairhead, L., Falletti, L., Flavoni, S., Foujols, M.-A., Gardoll, S., Gastineau, G., Ghattas, J., Grandpeix, J.-Y., Guenet, B., Guez, E., L., Guilyardi, E., Guimberteau, M., Hauglustaine, D., Hourdin, F., Idelkadi, A., Joussaume, S., Kageyama, M., Khodri, M., Krinner, G., Lebas, N., Levavasseur, G., Lévy, C., Li, L., Lott, F., Lurton, T., Luyssaert, S., Madec, G., Madeleine, J.-B., Maignan, F., Marchand, M., Marti, O., Mellul, L., Meurdesoif, Y., Mignot, J., Musat, I., Ottlé, C., Peylin, P., Planton, Y., Polcher, J., Rio, C., Rochetin, N., Rousset, C., Sepulchre, P., Sima, A., Swingedouw, D., Thiéblemont, R., Traore, A. K., Vancoppenolle, M., Vial, J., Vialard, J., Viovy, N., and Vuichard, N.: Presentation and Evaluation of the IPSL-CM6A-LR Climate Model, J. Adv. Model. Earth Sy., 12, e2019MS002010, https://doi.org/10.1029/2019MS002010, 2020. a
Brown, J. R., Brierley, C. M., An, S.-I., Guarino, M.-V., Stevenson, S., Williams, C. J. R., Zhang, Q., Zhao, A., Abe-Ouchi, A., Braconnot, P., Brady, E. C., Chandan, D., D'Agostino, R., Guo, C., LeGrande, A. N., Lohmann, G., Morozova, P. A., Ohgaito, R., O'ishi, R., Otto-Bliesner, B. L., Peltier, W. R., Shi, X., Sime, L., Volodin, E. M., Zhang, Z., and Zheng, W.: Comparison of past and future simulations of ENSO in CMIP5/PMIP3 and CMIP6/PMIP4 models, Clim. Past, 16, 1777–1805, https://doi.org/10.5194/cp-16-1777-2020, 2020. a
Cai, W., Ng, B., Wang, G., Santoso, A., Wu, L., and Yang, K.: Increased ENSO sea surface temperature variability under four IPCC emission scenarios, Nat. Clim. Change, 12, 228–231, https://doi.org/10.1038/s41558-022-01282-z, 2022. a
Canadell, J. G., Monteiro, P. M. S., Costa, M. H., Cunha, L. C. D., Cox, P. M., Eliseev, A. V., Henson, S., Ishii, M., Jaccard, S., Koven, C., Lohila, A., Patra, P. K., Piao, S., Syampungani, S., Zaehle, S., Zickfeld, K., Alexandrov, G. A., Bala, G., Bopp, L., Boysen, L., Cao, L., Chandra, N., Ciais, P., Denisov, S. N., Dentener, F. J., Douville, H., Fay, A., Forster, P., Fox-Kemper, B., Friedlingstein, P., Fu, W., Fuss, S., Garçon, V., Gier, B., Gillett, N. P., Gregor, L., Haustein, K., Haverd, V., He, J., Hewitt, H. T., Hoffman, F. M., Ilyina, T., Jackson, R., Jones, C., Keller, D. P., Kwiatkowski, L., Lamboll, R. D., Lan, X., Laufkötter, C., Quéré, C. L., Lenton, A., Lewis, J., Liddicoat, S., Lorenzoni, L., Lovenduski, N., Macdougall, A. H., Mathesius, S., Matthews, D. H., Meinshausen, M., Mokhov, I. I., Naik, V., Nicholls, Z. R. J., Nurhati, I. S., O'sullivan, M., Peters, G., Pongratz, J., Poulter, B., Sallée, J.-B., Saunois, M., Schuur, E. A. G., I.Seneviratne, S., Stavert, A., Suntharalingam, P., Tachiiri, K., Terhaar, J., Thompson, R., Tian, H., Turnbull, J., Vicente-Serrano, S. M., Wang, X., Wanninkhof, R. H., Williamson, P., Brovkin, V., Feely, R. A., and Lebehot, A. D.: Global Carbon and other Biogeochemical Cycles and Feedbacks, Chap. 5, https://hal.science/hal-03336145 (last access: 3 June 2023), 2021. a
Chang-Yang, C.-H., Sun, I.-F., Tsai, C.-H., Lu, C.-L., and Hsieh, C.-F.: ENSO and frost codetermine decade-long temporal variation in flower and seed production in a subtropical rain forest, J. Ecol., 104, 44–54, https://doi.org/10.1111/1365-2745.12481, 2016. a
Cherchi, A., Fogli, P. G., Lovato, T., Peano, D., Iovino, D., Gualdi, S., Masina, S., Scoccimarro, E., Materia, S., Bellucci, A., and Navarra, A.: Global Mean Climate and Main Patterns of Variability in the CMCC-CM2 Coupled Model, J. Adv. Model. Earth Sy., 11, 185–209, https://doi.org/10.1029/2018MS001369, 2019. a
Chevallier, F., Fisher, M., Peylin, P., Serrar, S., Bousquet, P., Bréon, F.-M., Chédin, A., and Ciais, P.: Inferring CO2 sources and sinks from satellite observations: Method and application to TOVS data, J. Geophys. Res.-Atmos., 110, https://doi.org/10.1029/2005JD006390, 2005. a
Ciais, P., Bastos, A., Chevallier, F., Lauerwald, R., Poulter, B., Canadell, J. G., Hugelius, G., Jackson, R. B., Jain, A., Jones, M., Kondo, M., Luijkx, I. T., Patra, P. K., Peters, W., Pongratz, J., Petrescu, A. M. R., Piao, S., Qiu, C., Von Randow, C., Regnier, P., Saunois, M., Scholes, R., Shvidenko, A., Tian, H., Yang, H., Wang, X., and Zheng, B.: Definitions and methods to estimate regional land carbon fluxes for the second phase of the REgional Carbon Cycle Assessment and Processes Project (RECCAP-2), Geosci. Model Dev., 15, 1289–1316, https://doi.org/10.5194/gmd-15-1289-2022, 2022. a
Contractor, S., Donat, M. G., Alexander, L. V., Ziese, M., Meyer-Christoffer, A., Schneider, U., Rustemeier, E., Becker, A., Durre, I., and Vose, R. S.: Rainfall Estimates on a Gridded Network (REGEN) – a global land-based gridded dataset of daily precipitation from 1950 to 2016, Hydrol. Earth Syst. Sci., 24, 919–943, https://doi.org/10.5194/hess-24-919-2020, 2020. a
Cox, P. M., Pearson, D., Booth, B. B., Friedlingstein, P., Huntingford, C., Jones, C. D., and Luke, C. M.: Sensitivity of tropical carbon to climate change constrained by carbon dioxide variability, Nature, 494, 341–344, https://doi.org/10.1038/nature11882, 2013. a
Cucchi, M., Weedon, G. P., Amici, A., Bellouin, N., Lange, S., Müller Schmied, H., Hersbach, H., and Buontempo, C.: WFDE5: bias-adjusted ERA5 reanalysis data for impact studies, Earth Syst. Sci. Data, 12, 2097–2120, https://doi.org/10.5194/essd-12-2097-2020, 2020. a
Danabasoglu, G., Lamarque, J.-F., Bacmeister, J., Bailey, D. A., DuVivier, A. K., Edwards, J., Emmons, L. K., Fasullo, J., Garcia, R., Gettelman, A., Hannay, C., Holland, M. M., Large, W. G., Lauritzen, P. H., Lawrence, D. M., Lenaerts, J. T. M., Lindsay, K., Lipscomb, W. H., Mills, M. J., Neale, R., Oleson, K. W., Otto-Bliesner, B., Phillips, A. S., Sacks, W., Tilmes, S., Kampenhout, L. v., Vertenstein, M., Bertini, A., Dennis, J., Deser, C., Fischer, C., Fox-Kemper, B., Kay, J. E., Kinnison, D., Kushner, P. J., Larson, V. E., Long, M. C., Mickelson, S., Moore, J. K., Nienhouse, E., Polvani, L., Rasch, P. J., and Strand, W. G.: The Community Earth System Model Version 2 (CESM2), J. Adv. Model. Earth Sy., 12, e2019MS001916, https://doi.org/10.1029/2019MS001916, 2020. a
Dee, D. P., Uppala, S. M., Simmons, A. J., Berrisford, P., Poli, P., Kobayashi, S., Andrae, U., Balmaseda, M. A., Balsamo, G., Bauer, P., Bechtold, P., Beljaars, A. C. M., van de Berg, L., Bidlot, J., Bormann, N., Delsol, C., Dragani, R., Fuentes, M., Geer, A. J., Haimberger, L., Healy, S. B., Hersbach, H., Hólm, E. V., Isaksen, L., Kållberg, P., Köhler, M., Matricardi, M., McNally, A. P., Monge-Sanz, B. M., Morcrette, J.-J., Park, B.-K., Peubey, C., de Rosnay, P., Tavolato, C., Thépaut, J.-N., and Vitart, F.: The ERA-Interim reanalysis: configuration and performance of the data assimilation system, Q. J. Roy. Meteorol. Soc., 137, 553–597, https://doi.org/10.1002/qj.828, 2011. a
Döscher, R., Acosta, M., Alessandri, A., Anthoni, P., Arsouze, T., Bergman, T., Bernardello, R., Boussetta, S., Caron, L.-P., Carver, G., Castrillo, M., Catalano, F., Cvijanovic, I., Davini, P., Dekker, E., Doblas-Reyes, F. J., Docquier, D., Echevarria, P., Fladrich, U., Fuentes-Franco, R., Gröger, M., v. Hardenberg, J., Hieronymus, J., Karami, M. P., Keskinen, J.-P., Koenigk, T., Makkonen, R., Massonnet, F., Ménégoz, M., Miller, P. A., Moreno-Chamarro, E., Nieradzik, L., van Noije, T., Nolan, P., O'Donnell, D., Ollinaho, P., van den Oord, G., Ortega, P., Prims, O. T., Ramos, A., Reerink, T., Rousset, C., Ruprich-Robert, Y., Le Sager, P., Schmith, T., Schrödner, R., Serva, F., Sicardi, V., Sloth Madsen, M., Smith, B., Tian, T., Tourigny, E., Uotila, P., Vancoppenolle, M., Wang, S., Wårlind, D., Willén, U., Wyser, K., Yang, S., Yepes-Arbós, X., and Zhang, Q.: The EC-Earth3 Earth system model for the Coupled Model Intercomparison Project 6, Geosci. Model Dev., 15, 2973–3020, https://doi.org/10.5194/gmd-15-2973-2022, 2022. a
Dunkl, I.: Compensatory effects conceal large uncertainties in the modelled processes behind the ENSO-CO2 relationship, Zenodo [code and data set], https://doi.org/10.5281/zenodo.14070579, 2024. a
Dunne, J. P., Horowitz, L. W., Adcroft, A. J., Ginoux, P., Held, I. M., John, J. G., Krasting, J. P., Malyshev, S., Naik, V., Paulot, F., Shevliakova, E., Stock, C. A., Zadeh, N., Balaji, V., Blanton, C., Dunne, K. A., Dupuis, C., Durachta, J., Dussin, R., Gauthier, P. P. G., Griffies, S. M., Guo, H., Hallberg, R. W., Harrison, M., He, J., Hurlin, W., McHugh, C., Menzel, R., Milly, P. C. D., Nikonov, S., Paynter, D. J., Ploshay, J., Radhakrishnan, A., Rand, K., Reichl, B. G., Robinson, T., Schwarzkopf, D. M., Sentman, L. T., Underwood, S., Vahlenkamp, H., Winton, M., Wittenberg, A. T., Wyman, B., Zeng, Y., and Zhao, M.: The GFDL Earth System Model Version 4.1 (GFDL-ESM 4.1): Overall Coupled Model Description and Simulation Characteristics, J. Adv. Model. Earth Sy., 12, e2019MS002015, https://doi.org/10.1029/2019MS002015, 2020. a
Fu, Y., Lin, Z., and Wang, T.: Simulated Relationship between Wintertime ENSO and East Asian Summer Rainfall: From CMIP3 to CMIP6, Adv. Atmos. Sci., 38, 221–236, https://doi.org/10.1007/s00376-020-0147-y, 2021. a
Gaubert, B., Stephens, B. B., Basu, S., Chevallier, F., Deng, F., Kort, E. A., Patra, P. K., Peters, W., Rödenbeck, C., Saeki, T., Schimel, D., Van der Laan-Luijkx, I., Wofsy, S., and Yin, Y.: Global atmospheric CO2 inverse models converging on neutral tropical land exchange, but disagreeing on fossil fuel and atmospheric growth rate, Biogeosciences, 16, 117–134, https://doi.org/10.5194/bg-16-117-2019, 2019. a
Gelaro, R., McCarty, W., Suárez, M. J., Todling, R., Molod, A., Takacs, L., Randles, C. A., Darmenov, A., Bosilovich, M. G., Reichle, R., Wargan, K., Coy, L., Cullather, R., Draper, C., Akella, S., Buchard, V., Conaty, A., Silva, A. M. d., Gu, W., Kim, G.-K., Koster, R., Lucchesi, R., Merkova, D., Nielsen, J. E., Partyka, G., Pawson, S., Putman, W., Rienecker, M., Schubert, S. D., Sienkiewicz, M., and Zhao, B.: The Modern-Era Retrospective Analysis for Research and Applications, Version 2 (MERRA-2), J. Climate, 30, 5419–5454, https://doi.org/10.1175/JCLI-D-16-0758.1, 2017. a
Golaz, J.-C., Caldwell, P. M., Roekel, L. P. V., Petersen, M. R., Tang, Q., Wolfe, J. D., Abeshu, G., Anantharaj, V., Asay-Davis, X. S., Bader, D. C., Baldwin, S. A., Bisht, G., Bogenschutz, P. A., Branstetter, M., Brunke, M. A., Brus, S. R., Burrows, S. M., Cameron-Smith, P. J., Donahue, A. S., Deakin, M., Easter, R. C., Evans, K. J., Feng, Y., Flanner, M., Foucar, J. G., Fyke, J. G., Griffin, B. M., Hannay, C., Harrop, B. E., Hoffman, M. J., Hunke, E. C., Jacob, R. L., Jacobsen, D. W., Jeffery, N., Jones, P. W., Keen, N. D., Klein, S. A., Larson, V. E., Leung, L. R., Li, H.-Y., Lin, W., Lipscomb, W. H., Ma, P.-L., Mahajan, S., Maltrud, M. E., Mametjanov, A., McClean, J. L., McCoy, R. B., Neale, R. B., Price, S. F., Qian, Y., Rasch, P. J., Eyre, J. E. J. R., Riley, W. J., Ringler, T. D., Roberts, A. F., Roesler, E. L., Salinger, A. G., Shaheen, Z., Shi, X., Singh, B., Tang, J., Taylor, M. A., Thornton, P. E., Turner, A. K., Veneziani, M., Wan, H., Wang, H., Wang, S., Williams, D. N., Wolfram, P. J., Worley, P. H., Xie, S., Yang, Y., Yoon, J.-H., Zelinka, M. D., Zender, C. S., Zeng, X., Zhang, C., Zhang, K., Zhang, Y., Zheng, X., Zhou, T., and Zhu, Q.: The DOE E3SM Coupled Model Version 1: Overview and Evaluation at Standard Resolution, J. Adv. Model. Earth Sy., 11, 2089–2129, https://doi.org/10.1029/2018MS001603, 2019. a
Hajima, T., Watanabe, M., Yamamoto, A., Tatebe, H., Noguchi, M. A., Abe, M., Ohgaito, R., Ito, A., Yamazaki, D., Okajima, H., Ito, A., Takata, K., Ogochi, K., Watanabe, S., and Kawamiya, M.: Development of the MIROC-ES2L Earth system model and the evaluation of biogeochemical processes and feedbacks, Geosci. Model Dev., 13, 2197–2244, https://doi.org/10.5194/gmd-13-2197-2020, 2020. a
Harris, I., Osborn, T. J., Jones, P., and Lister, D.: Version 4 of the CRU TS monthly high-resolution gridded multivariate climate dataset, Sci. Data, 7, 109, https://doi.org/10.1038/s41597-020-0453-3, 2020. a
Holmgren, M., Scheffer, M., Ezcurra, E., Gutiérrez, J. R., and Mohren, G. M. J.: El Niño effects on the dynamics of terrestrial ecosystems, Trends Ecol. Evol., 16, 89–94, https://doi.org/10.1016/S0169-5347(00)02052-8, 2001. a, b, c
Iturbide, M., Gutiérrez, J. M., Alves, L. M., Bedia, J., Cerezo-Mota, R., Cimadevilla, E., Cofiño, A. S., Di Luca, A., Faria, S. H., Gorodetskaya, I. V., Hauser, M., Herrera, S., Hennessy, K., Hewitt, H. T., Jones, R. G., Krakovska, S., Manzanas, R., Martínez-Castro, D., Narisma, G. T., Nurhati, I. S., Pinto, I., Seneviratne, S. I., van den Hurk, B., and Vera, C. S.: An update of IPCC climate reference regions for subcontinental analysis of climate model data: definition and aggregated datasets, Earth Syst. Sci. Data, 12, 2959–2970, https://doi.org/10.5194/essd-12-2959-2020, 2020. a
Jung, M., Koirala, S., Weber, U., Ichii, K., Gans, F., Camps-Valls, G., Papale, D., Schwalm, C., Tramontana, G., and Reichstein, M.: The FLUXCOM ensemble of global land-atmosphere energy fluxes, Sci. Data, 6, 74, https://doi.org/10.1038/s41597-019-0076-8, 2019. a, b
Kalnay, E., Kanamitsu, M., Kistler, R., Collins, W., Deaven, D., Gandin, L., Iredell, M., Saha, S., White, G., Woollen, J., Zhu, Y., Chelliah, M., Ebisuzaki, W., Higgins, W., Janowiak, J., Mo, K. C., Ropelewski, C., Wang, J., Leetmaa, A., Reynolds, R., Jenne, R., and Joseph, D.: The NCEP/NCAR 40-Year Reanalysis Project, B. Am. Meteorol. Soc., 77, 437–472, https://doi.org/10.1175/1520-0477(1996)077<0437:TNYRP>2.0.CO;2, 1996. a
Kobayashi, S., Ota, Y., Harada, Y., Ebita, A., Moriya, M., Onoda, H., Onogi, K., Kamahori, H., Kobayashi, C., Endo, H., Miyaoka, K., and Takahashi, K.: The JRA-55 Reanalysis: General Specifications and Basic Characteristics, J. Meteorol. Soc. Jpn. Ser. II, 93, 5–48, https://doi.org/10.2151/jmsj.2015-001, 2015. a
Koirala, S., Jones, C., Ahrens, B., Fan, N., Brovkin, V., Delire, C., Fan, Y., Gayler, V., Joetzjer, E., Lee, H., Materia, S., Nabel, J., Peano, D., Peylin, P., Wårlind, D., Wiltshire, A., Zaehle, S., Reichstein, M., and Carvalhais, N.: Underrepresented controls of aridity in climate sensitivity of carbon cycle models, in review, https://doi.org/10.21203/rs.3.rs-2013805/v1, 2022. a
Li, N., Sippel, S., Winkler, A. J., Mahecha, M. D., Reichstein, M., and Bastos, A.: Interannual global carbon cycle variations linked to atmospheric circulation variability, Earth Syst. Dynam., 13, 1505–1533, https://doi.org/10.5194/esd-13-1505-2022, 2022. a
López, B. C., Rodríguez, R., Gracia, C. A., and Sabaté, S.: Climatic signals in growth and its relation to ENSO events of two Prosopis species following a latitudinal gradient in South America, Global Change Biol., 12, 897–906, https://doi.org/10.1111/j.1365-2486.2006.01138.x, 2006. a
Lovato, T., Peano, D., Butenschön, M., Materia, S., Iovino, D., Scoccimarro, E., Fogli, P. G., Cherchi, A., Bellucci, A., Gualdi, S., Masina, S., and Navarra, A.: CMIP6 Simulations With the CMCC Earth System Model (CMCC-ESM2), J. Adv. Model. Earth Sy., 14, e2021MS002814, https://doi.org/10.1029/2021MS002814, 2022. a
Manzanas, R., Frías, M. D., Cofiño, A. S., and Gutiérrez, J. M.: Validation of 40 year multimodel seasonal precipitation forecasts: The role of ENSO on the global skill, J. Geophys. Res.-Atmos., 119, 1708–1719, https://doi.org/10.1002/2013JD020680, 2014. a
Mauritsen, T., Bader, J., Becker, T., Behrens, J., Bittner, M., Brokopf, R., Brovkin, V., Claussen, M., Crueger, T., Esch, M., Fast, I., Fiedler, S., Fläschner, D., Gayler, V., Giorgetta, M., Goll, D. S., Haak, H., Hagemann, S., Hedemann, C., Hohenegger, C., Ilyina, T., Jahns, T., Jimenéz-de-la-Cuesta, D., Jungclaus, J., Kleinen, T., Kloster, S., Kracher, D., Kinne, S., Kleberg, D., Lasslop, G., Kornblueh, L., Marotzke, J., Matei, D., Meraner, K., Mikolajewicz, U., Modali, K., Möbis, B., Müller, W. A., Nabel, J. E. M. S., Nam, C. C. W., Notz, D., Nyawira, S.-S., Paulsen, H., Peters, K., Pincus, R., Pohlmann, H., Pongratz, J., Popp, M., Raddatz, T. J., Rast, S., Redler, R., Reick, C. H., Rohrschneider, T., Schemann, V., Schmidt, H., Schnur, R., Schulzweida, U., Six, K. D., Stein, L., Stemmler, I., Stevens, B., Storch, J.-S. V., Tian, F., Voigt, A., Vrese, P., Wieners, K.-H., Wilkenskjeld, S., Winkler, A., and Roeckner, E.: Developments in the MPI-M Earth System Model version 1.2 (MPI-ESM1.2) and Its Response to Increasing CO2, J. Adv. Model. Earth Sy., 11, 998–1038, https://doi.org/10.1029/2018MS001400, 2019. a
Orbe, C., Rind, D., Jonas, J., Nazarenko, L., Faluvegi, G., Murray, L. T., Shindell, D. T., Tsigaridis, K., Zhou, T., Kelley, M., and Schmidt, G. A.: GISS Model E2.2: A Climate Model Optimized for the Middle Atmosphere – 2. Validation of Large-Scale Transport and Evaluation of Climate Response, J. Geophys. Res.-Atmos., 125, e2020JD033151, https://doi.org/10.1029/2020JD033151, 2020. a
O'Sullivan, M., Smith, W. K., Sitch, S., Friedlingstein, P., Arora, V. K., Haverd, V., Jain, A. K., Kato, E., Kautz, M., Lombardozzi, D., Nabel, J. E. M. S., Tian, H., Vuichard, N., Wiltshire, A., Zhu, D., and Buermann, W.: Climate-Driven Variability and Trends in Plant Productivity Over Recent Decades Based on Three Global Products, Global Biogeochem. Cycles, 34, e2020GB006613, https://doi.org/10.1029/2020GB006613, 2020. a, b
Padrón, R. S., Gudmundsson, L., Liu, L., Humphrey, V., and Seneviratne, S. I.: Drivers of intermodel uncertainty in land carbon sink projections, Biogeosciences, 19, 5435–5448, https://doi.org/10.5194/bg-19-5435-2022, 2022. a
Perry, S. J., McGregor, S., Sen Gupta, A., England, M. H., and Maher, N.: Projected late 21st century changes to the regional impacts of the El Niño-Southern Oscillation, Clim. Dynam., 54, 395–412, https://doi.org/10.1007/s00382-019-05006-6, 2020. a, b
Piao, S., Wang, X., Wang, K., Li, X., Bastos, A., Canadell, J. G., Ciais, P., Friedlingstein, P., and Sitch, S.: Interannual variation of terrestrial carbon cycle: Issues and perspectives, Global Change Biol., 26, 300–318, https://doi.org/10.1111/gcb.14884, 2020. a, b
Poulter, B., Frank, D., Ciais, P., Myneni, R. B., Andela, N., Bi, J., Broquet, G., Canadell, J. G., Chevallier, F., Liu, Y. Y., Running, S. W., Sitch, S., and van der Werf, G. R.: Contribution of semi-arid ecosystems to interannual variability of the global carbon cycle, Nature, 509, 600–603, https://doi.org/10.1038/nature13376, 2014. a
Qian, H., Joseph, R., and Zeng, N.: Response of the terrestrial carbon cycle to the El Niño-Southern Oscillation, Tellus B, 60, 537–550, https://doi.org/10.1111/j.1600-0889.2008.00360.x, 2008. a
Rayner, N. A., Parker, D. E., Horton, E. B., Folland, C. K., Alexander, L. V., Rowell, D. P., Kent, E. C., and Kaplan, A.: Global analyses of sea surface temperature, sea ice, and night marine air temperature since the late nineteenth century, J. Geophys. Res.-Atmos., 108, https://doi.org/10.1029/2002JD002670, 2003. a
Santos, V. A. H. F. d., Ferreira, M. J., Rodrigues, J. V. F. C., Garcia, M. N., Ceron, J. V. B., Nelson, B. W., and Saleska, S. R.: Causes of reduced leaf-level photosynthesis during strong El Niño drought in a Central Amazon forest, Global Change Biol., 24, 4266–4279, https://doi.org/10.1111/gcb.14293, 2018. a
Séférian, R., Nabat, P., Michou, M., Saint-Martin, D., Voldoire, A., Colin, J., Decharme, B., Delire, C., Berthet, S., Chevallier, M., Sénési, S., Franchisteguy, L., Vial, J., Mallet, M., Joetzjer, E., Geoffroy, O., Guérémy, J.-F., Moine, M.-P., Msadek, R., Ribes, A., Rocher, M., Roehrig, R., Salas-y Mélia, D., Sanchez, E., Terray, L., Valcke, S., Waldman, R., Aumont, O., Bopp, L., Deshayes, J., Éthé, C., and Madec, G.: Evaluation of CNRM Earth System Model, CNRM-ESM2-1: Role of Earth System Processes in Present-Day and Future Climate, J. Adv. Model. Earth Sy., 11, 4182–4227, https://doi.org/10.1029/2019MS001791, 2019. a
Seland, Ø., Bentsen, M., Olivié, D., Toniazzo, T., Gjermundsen, A., Graff, L. S., Debernard, J. B., Gupta, A. K., He, Y.-C., Kirkevåg, A., Schwinger, J., Tjiputra, J., Aas, K. S., Bethke, I., Fan, Y., Griesfeller, J., Grini, A., Guo, C., Ilicak, M., Karset, I. H. H., Landgren, O., Liakka, J., Moseid, K. O., Nummelin, A., Spensberger, C., Tang, H., Zhang, Z., Heinze, C., Iversen, T., and Schulz, M.: Overview of the Norwegian Earth System Model (NorESM2) and key climate response of CMIP6 DECK, historical, and scenario simulations, Geosci. Model Dev., 13, 6165–6200, https://doi.org/10.5194/gmd-13-6165-2020, 2020. a, b
Sellar, A. A., Jones, C. G., Mulcahy, J. P., Tang, Y., Yool, A., Wiltshire, A., O'Connor, F. M., Stringer, M., Hill, R., Palmieri, J., Woodward, S., Mora, L. d., Kuhlbrodt, T., Rumbold, S. T., Kelley, D. I., Ellis, R., Johnson, C. E., Walton, J., Abraham, N. L., Andrews, M. B., Andrews, T., Archibald, A. T., Berthou, S., Burke, E., Blockley, E., Carslaw, K., Dalvi, M., Edwards, J., Folberth, G. A., Gedney, N., Griffiths, P. T., Harper, A. B., Hendry, M. A., Hewitt, A. J., Johnson, B., Jones, A., Jones, C. D., Keeble, J., Liddicoat, S., Morgenstern, O., Parker, R. J., Predoi, V., Robertson, E., Siahaan, A., Smith, R. S., Swaminathan, R., Woodhouse, M. T., Zeng, G., and Zerroukat, M.: UKESM1: Description and Evaluation of the U.K. Earth System Model, J. Adv. Model. Earth Sy., 11, 4513–4558, https://doi.org/10.1029/2019MS001739, 2019. a
Shi, X., Lohmann, G., Sidorenko, D., and Yang, H.: Early-Holocene simulations using different forcings and resolutions in AWI-ESM, The Holocene, 30, 996–1015, https://doi.org/10.1177/0959683620908634, 2020. a
Silva, C. V. J., Aragão, L. E. O. C., Barlow, J., Espirito-Santo, F., Young, P. J., Anderson, L. O., Berenguer, E., Brasil, I., Foster Brown, I., Castro, B., Farias, R., Ferreira, J., França, F., Graça, P. M. L. A., Kirsten, L., Lopes, A. P., Salimon, C., Scaranello, M. A., Seixas, M., Souza, F. C., and Xaud, H. A. M.: Drought-induced Amazonian wildfires instigate a decadal-scale disruption of forest carbon dynamics, Philos. T. Roy. Soc. B, 373, 20180043, https://doi.org/10.1098/rstb.2018.0043, 2018. a
Sitch, S., Friedlingstein, P., Gruber, N., Jones, S. D., Murray-Tortarolo, G., Ahlström, A., Doney, S. C., Graven, H., Heinze, C., Huntingford, C., Levis, S., Levy, P. E., Lomas, M., Poulter, B., Viovy, N., Zaehle, S., Zeng, N., Arneth, A., Bonan, G., Bopp, L., Canadell, J. G., Chevallier, F., Ciais, P., Ellis, R., Gloor, M., Peylin, P., Piao, S. L., Le Quéré, C., Smith, B., Zhu, Z., and Myneni, R.: Recent trends and drivers of regional sources and sinks of carbon dioxide, Biogeosciences, 12, 653–679, https://doi.org/10.5194/bg-12-653-2015, 2015. a
Spring, A. and Ilyina, T.: Predictability Horizons in the Global Carbon Cycle Inferred From a Perfect-Model Framework, Geophys. Res. Lett., 47, e2019GL085311, https://doi.org/10.1029/2019GL085311, 2020. a
Swart, N. C., Cole, J. N. S., Kharin, V. V., Lazare, M., Scinocca, J. F., Gillett, N. P., Anstey, J., Arora, V., Christian, J. R., Hanna, S., Jiao, Y., Lee, W. G., Majaess, F., Saenko, O. A., Seiler, C., Seinen, C., Shao, A., Sigmond, M., Solheim, L., von Salzen, K., Yang, D., and Winter, B.: The Canadian Earth System Model version 5 (CanESM5.0.3), Geosci. Model Dev., 12, 4823–4873, https://doi.org/10.5194/gmd-12-4823-2019, 2019. a
Tian, H., Melillo, J. M., Kicklighter, D. W., McGuire, A. D., Helfrich, J. V. K., Moore, B., and Vörösmarty, C. J.: Effect of interannual climate variability on carbon storage in Amazonian ecosystems, Nature, 396, 664–667, https://doi.org/10.1038/25328, 1998. a
Volodin, E. M., Mortikov, E. V., Kostrykin, S. V., Galin, V. Y., Lykossov, V. N., Gritsun, A. S., Diansky, N. A., Gusev, A. V., Iakovlev, N. G., Shestakova, A. A., and Emelina, S. V.: Simulation of the modern climate using the INM-CM48 climate model, Russian Journal of Numerical Analysis and Mathematical Modelling, 33, 367–374, https://doi.org/10.1515/rnam-2018-0032, 2018. a
Wang, J., Zeng, N., and Wang, M.: Interannual variability of the atmospheric CO2 growth rate: roles of precipitation and temperature, Biogeosciences, 13, 2339–2352, https://doi.org/10.5194/bg-13-2339-2016, 2016. a
Watanabe, S., Hajima, T., Sudo, K., Abe, M., Arakawa, O., Ogochi, K., Arakawa, T., Tatebe, H., Ito, A., Ito, A., Komuro, Y., Nitta, T., Noguchi, M. A., Ogura, T., Ohgaito, R., Sekiguchi, M., Suzuki, T., Tachiiri, K., Takata, K., Takemura, T., Watanabe, M., Yamamoto, A., Yamazaki, D., Yoshimura, K., and Kawamiya, M.: MIROC MIROC-ES2H model output prepared for CMIP6 GeoMIP, WCRP [data set], https://doi.org/10.22033/ESGF/CMIP6.907, 2021. a
Wigneron, J.-P., Fan, L., Ciais, P., Bastos, A., Brandt, M., Chave, J., Saatchi, S., Baccini, A., and Fensholt, R.: Tropical forests did not recover from the strong 2015–2016 El Niño event, Sci. Adv., https://doi.org/10.1126/sciadv.aay4603, 2020. a
Wu, T., Lu, Y., Fang, Y., Xin, X., Li, L., Li, W., Jie, W., Zhang, J., Liu, Y., Zhang, L., Zhang, F., Zhang, Y., Wu, F., Li, J., Chu, M., Wang, Z., Shi, X., Liu, X., Wei, M., Huang, A., Zhang, Y., and Liu, X.: The Beijing Climate Center Climate System Model (BCC-CSM): the main progress from CMIP5 to CMIP6 , Geosci. Model Dev., 12, 1573–1600, https://doi.org/10.5194/gmd-12-1573-2019, 2019. a
Yang, X. and Huang, P.: Improvements in the relationship between tropical precipitation and sea surface temperature from CMIP5 to CMIP6, Clim. Dynam., 60, 3319–3337, https://doi.org/10.1007/s00382-022-06519-3, 2022. a
Yeh, S.-W., Cai, W., Min, S.-K., McPhaden, M. J., Dommenget, D., Dewitte, B., Collins, M., Ashok, K., An, S.-I., Yim, B.-Y., and Kug, J.-S.: ENSO Atmospheric Teleconnections and Their Response to Greenhouse Gas Forcing, Rev. Geophys., 56, 185–206, https://doi.org/10.1002/2017RG000568, 2018. a
Yukimoto, S., Kawai, H., Koshiro, T., Oshima, N., Yoshida, K., Urakawa, S., Tsujino, H., Deushi, M., Tanaka, T., Hosaka, M., Yabu, S., Yoshimura, H., Shindo, E., Mizuta, R., Obata, A., Adachi, Y., and Ishii, M.: The Meteorological Research Institute Earth System Model Version 2.0, MRI-ESM2.0: Description and Basic Evaluation of the Physical Component, J. Meteorol. Soc. Jpn. Ser. II, 97, 931–965, https://doi.org/10.2151/jmsj.2019-051, 2019. a
Zechlau, S., Schlund, M., Cox, P. M., Friedlingstein, P., and Eyring, V.: Do Emergent Constraints on Carbon Cycle Feedbacks Hold in CMIP6?, J. Geophys. Res.-Biogeo., 127, e2022JG006985, https://doi.org/10.1029/2022JG006985, 2022. a
Zeng, N., Mariotti, A., and Wetzel, P.: Terrestrial mechanisms of interannual CO2 variability, Global Biogeochem. Cycles, 19, https://doi.org/10.1029/2004GB002273, 2005. a
Zeng, N., Yoon, J.-H., Vintzileos, A., Collatz, G. J., Kalnay, E., Mariotti, A., Kumar, A., Busalacchi, A., and Lord, S.: Dynamical prediction of terrestrial ecosystems and the global carbon cycle: A 25-year hindcast experiment, Global Biogeochem. Cycles, 22, https://doi.org/10.1029/2008GB003183, 2008. a
Zhang, Y., Dannenberg, M. P., Hwang, T., and Song, C.: El Niño-Southern Oscillation-Induced Variability of Terrestrial Gross Primary Production During the Satellite Era, J. Geophys. Res.-Biogeo., 124, 2419–2431, https://doi.org/10.1029/2019JG005117, 2019. a
Zhu, Z., Piao, S., Xu, Y., Bastos, A., Ciais, P., and Peng, S.: The effects of teleconnections on carbon fluxes of global terrestrial ecosystems, Geophys. Res. Lett., 44, 3209–3218, https://doi.org/10.1002/2016GL071743, 2017. a
Ziehn, T., Chamberlain, M. A., Law, R. M., Lenton, A., Bodman, R. W., Dix, M., Stevens, L., Wang, Y.-P., Srbinovsky, J., Ziehn, T., Chamberlain, M. A., Law, R. M., Lenton, A., Bodman, R. W., Dix, M., Stevens, L., Wang, Y.-P., and Srbinovsky, J.: The Australian Earth System Model: ACCESS-ESM1.5, Journal of Southern Hemisphere Earth Systems Science, 70, 193–214, https://doi.org/10.1071/ES19035, 2020. a