the Creative Commons Attribution 4.0 License.
the Creative Commons Attribution 4.0 License.
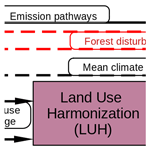
Fire weather compromises forestation-reliant climate mitigation pathways
Jonas Schwaab
Yann Quilcaille
Michael Windisch
Jonathan Doelman
Stefan Frank
Mykola Gusti
Petr Havlik
Florian Humpenöder
Andrey Lessa Derci Augustynczik
Christoph Müller
Kanishka Balu Narayan
Ryan Sebastian Padrón
Alexander Popp
Detlef van Vuuren
Michael Wögerer
Sonia Isabelle Seneviratne
Forestation can contribute to climate change mitigation. However, increasing frequency and intensity of climate extremes are posed to have profound impact on forests and consequently on the mitigation potential of forestation efforts. In this perspective, we critically assess forestation-reliant climate mitigation scenarios from five different integrated assessment models (IAMs) by showcasing the spatially explicit exposure of forests to fire weather and the simulated increase in global annual burned area. We provide a detailed description of the feedback from climate change to forest carbon uptake in IAMs. Few IAMs are currently accounting for feedback mechanisms like loss from fire disturbance. Consequently, many forestation areas proposed by IAM scenarios will be exposed to fire-promoting weather conditions and without costly prevention measures might be object to frequent burning. We conclude that the actual climate mitigation portfolio in IAM scenarios is subject to substantial uncertainty and that the risk of overly optimistic estimates of negative emission potential of forestation should be avoided. As a way forward we propose how to integrate more detailed climate information when modeling climate mitigation pathways heavily relying on forestation.
- Article
(3186 KB) - Full-text XML
-
Supplement
(16108 KB) - BibTeX
- EndNote
Negative emissions, i.e., carbon uptake from the atmosphere, are essential for ambitious climate change mitigation (van Vuuren et al., 2017a). Integrated assessment models (IAMs) are commonly used to derive emission scenarios compatible with maximum warming levels of 1.5 °C or well below 2.0 °C of global warming relative to pre-industrial levels (IPCC, 2018), which were set as targets as part of the Paris Agreement (UNFCCC, 2015). These simulations, which provide forcing datasets for greenhouse gas (GHG) emissions and land use in the Coupled Model Intercomparison Project Phase 6 (CMIP6; see Fig. 2), include a substantial amount of carbon removal from the atmosphere for reaching net-zero GHG emissions within the next 20 to 40 years. In addition to bioenergy with carbon capture and storage (BECCS; Vaughan et al., 2018), the assessed IAMs project afforestation and reforestation (forestation, A/R) over an area ranging from 2.6 to 14 Mkm2 (Figs. 1 and S9 in the Supplement, Popp et al., 2017) as a nature-based carbon storage strategy. This considerable spread across an order of magnitude is also present in terms of carbon sequestration potential (approximately 0.5 to 12 Gt CO2 a−1 by 2100) (Griscom et al., 2017; Fuss et al., 2018). Sources of uncertainty lie in both future socioeconomic dynamics (Popp et al., 2017; Brown et al., 2021) and vegetation responses to disturbances including those that are induced or exacerbated by climate extremes (Anderegg et al., 2020).
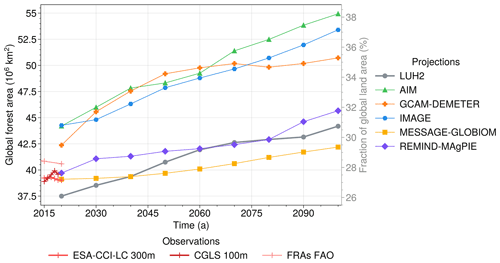
Figure 1Projected and observed (red) global forest area. Six projections under SSP1-2.6 are shown for LUH2 and five different IAMs. Two observational datasets from satellite imagery (Harper et al., 2023; Buchhorn, 2020) and data from forest resource assessments (FRAs) of the FAO (FAO, 2016, 2020) are shown for the past and present.
Fire as a prominent hazard for carbon accumulation in forests is influenced by the increasing intensity and frequency of climate extremes (Seneviratne et al., 2021). Gross fire emissions amounted to 1.8 Gt C a−1 on average between 2003 and 2022 and reached 1.9–2.3 Gt C a−1 in 2023 (Friedlingstein et al., 2023). Although only some of these emissions are from climate-change driven fires and a post-fire vegetation recovery sink is unaccounted for, it is important to note the relevance of fire for the land carbon sink. For example, anomalously high fire emissions, such as those from recent boreal forest fires, could contribute to large biomes ceasing to act as carbon sinks (Fan et al., 2023). Generally, wildfire with increasing frequency and intensity is reducing biomass and soil carbon stocks (Walker et al., 2019; Pellegrini et al., 2022), limiting long-term carbon uptake (Koch and Kaplan, 2022). Furthermore, fire in some places is a key factor determining whether forests can exist or not (Murphy and Bowman, 2012) and can substantially reduce tree and forest cover (Lasslop et al., 2020). Intensifying fire weather as observed (Bedia et al., 2015; Abatzoglou et al., 2018; Jones et al., 2022) and projected (Son et al., 2021;Davies-Barnard et al., 2023) hence puts increasing pressure on forests and their associated carbon storage. Thus, depending on the region, future fire regimes might significantly influence whether long-term global-scale carbon capturing by A/R is feasible in the projected ranges with the projected costs and deployment speed.
A debate about the conditions and feasibility of large-scale A/R under projected future climate conditions is therefore needed. Comparing maps of fire weather change and forestation potential, Hermoso et al. (2021) have recently brought forward their concern regarding increasing fire disturbance in forest restoration projects in Europe. Meanwhile, the science of drivers of future fire risk for forest carbon sinks, e.g., Clarke et al. (2022) identifying high water vapor pressure deficit as a major threat, goes on. Arguing against major concerns, Golub et al. (2022) presented a land use allocation assessment that calculates die-back rates for various biomes as a function of changes in global mean temperature without considering local extreme conditions. Their findings suggest that wildfires may not compromise forest-based climate strategies. In this context, it is particularly concerning that the negative influence of increasing climate extremes such as fires on the mitigation potential of forests is currently under-represented in IAMs. A typical channel for such natural hazards to be included in IAMs is via adjusted long-term carbon stock potential of certain land use types. Since IAMs are broadly used for emission projections to inform climate model experiments as well as international climate policies, it is essential to better understand and quantify uncertainties in their modeling process and assumptions about the potential for A/R.
In this perspective, we therefore assess the plausibility of forestation-reliant climate mitigation scenarios as used within CMIP6. We do this by analyzing whether the effectiveness of forestation in the current spatially explicit representation of IAM projections could be compromised by fire disturbance based on available scenarios from a range of state-of-the-art IAMs. First, we present an overview of the amounts of forestation as projected by IAMs and how sensitive they are to climate change effects (Sect. 2). In Sect. 3, we introduce a climatological measure for atmospheric pressure on forests to burn, called a seasonally extreme fire weather index, and analyze its behavior in Earth system models (ESMs) during both historical and future periods for comparison with climate data. By combining these datasets, we evaluate the extent and locations of increased exposure of global forests to fire weather, discerning the relative contributions of exposure change (forest expansion) and hazard change (fire intensification due to climate change) to the rise in forest fire danger (Sect. 4). To put this into perspective, we provide an overview of the modeling landscape on how spatially explicit information on forest disturbances and climate change is treated in state-of-the-art land use allocation in IAMs (Sect. 5). Finally, we discuss how the representation of climate impacts on forestation can be improved to arrive at more substantiated climate mitigation scenarios (Sect. 6).
IAMs project global forest area to be vastly expanded under SSP1-1.9 and SSP1-2.6 (Fig. 1), which are roughly compatible with maximum warming levels of 1.5 and 2 °C of global mean surface temperature above pre-industrial levels, respectively (building on Shared Socioeconomic Pathway 1, SSP1; van Vuuren et al., 2017b; IPCC, 2018). To arrive at this result, we assembled several land use projections to analyze A/R scenarios including the Land Use Harmonization (LUH) dataset 2 and five IAM datasets under SSP1-2.6. A detailed description of the setup used in these models (Table C1) and a discussion of inter-model differences in forest cover are given in Appendix C.
The global forest area in 2020 in the scenarios is comparable to observational datasets and forest resource assessments (with deviations at the local scale; see also Chen et al., 2020). The forest area in SSP1-2.6 grows from 39 to 44 Mkm2 in 2020 to 42 to 55 Mkm2 in 2090. This is an expansion of 3 to 10 Mkm2 corresponding to 7 to 22 %. Similar but even stronger trends are found in the SSP1-1.9 projections, especially for IMAGE and AIM (see Fig. S9).
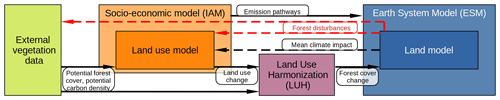
Figure 2Information flow of forest-related data in the typical model chain from IAMs to ESMs for scenarios used in CMIP6. Black arrows indicate established data exchange, and red arrows indicate the data exchange proposed here in the context of forest fire risk. Dashed arrows indicate partly established links in some of the IAMs. While some IAMs include basic information about the overall fire regime encoded in input data about vegetation potential or impacts by mean climate, most are lacking representation of geographically explicit disturbance regimes changing over time.
The forestation pathways considered here are simulated by state-of-the-art IAMs, which provide the scenarios underlying the CMIP6 simulations within the model chain towards Earth system models (ESMs) (emission and land use change pathways; see Fig. 2). In the typical setup, input data on potential vegetation is used in the land use models within socioeconomic models to simulate land use change within a certain climate mitigation scenario. This land use change is harmonized with datasets on historical land use and land cover change by LUH for the generation of one forcing dataset fed to the land models in ESMs. Along this chain, IAM outputs also influence the main future climate simulations assessed in the 6th Assessment Report of the IPCC (IPCC, 2021). In most of the assessed frameworks however, forest-related feedback from climate to land use and vegetation potential modeling is lacking or only implemented partially (red and dashed arrows in Fig. 2).
Accounting for future climate changes – particularly climate extremes – is likely to significantly alter the carbon sequestration potential and the employed forestation in scenarios produced by these IAMs. In a comparison of land use projections with and without all implemented climate impacts, the global forest expansion of MESSAGE-GLOBIOM and REMIND-MAgPIE showed very large sensitivity of 25 % and up to 50 %, respectively (see Fig. S7 in the Supplement). Consequently, both climate-impacted grid-cell-level and globally aggregated carbon sequestration potential estimates matter for the overall mitigation portfolio in ambitious climate change mitigation scenarios.
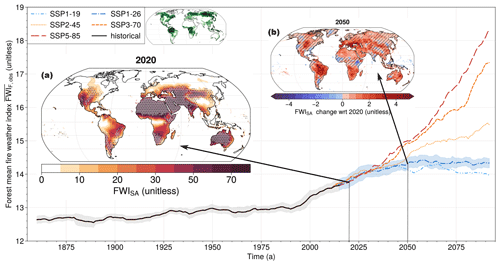
Figure 3Fire weather intensifying over time in the historical simulation and in five future scenarios. The focus of this study is SSP1-2.6 (dark blue, roughly 2 °C global warming scenario). The global forest-area-weighted mean FWI, , is computed over forest area from satellite-observed tree cover (ESA-CCI land cover; Harper et al., 2023) in 2020 (inset next to legend). Around the lines representing the multi-model median the shading indicates typical year-to-year variability (multi-model mean running 10-year standard deviation) of the global aggregate value in the historical and the SSP1-2.6 scenario. Maps show the distribution of the 10-year mean as well as the multi-model median FWISA over land in 2020 (a) and its changes under SSP1-2.6 until 2050 (b). Stippling in (a) rules out regions of low (< 5 %) forest cover. Hatching in (b) indicates areas where fewer than 8 ESMs out of 10 agree on the sign of change, while the global rise in is found robustly across ESMs.
Here, we use annual values of maximum seasonally averaged (SA) Canadian Fire Weather Index (FWI) (Abatzoglou et al., 2019; Quilcaille and Batibeniz, 2022), FWISA, to analyze the fire hazard for forests in IAM projections. FWI combines atmospheric conditions (heat, air dryness, lacking precipitation and wind speed; Wagner, 1987; Wang et al., 2015), which are a crucial driver of forest fire activity. FWI is one of the most widely used indicators for fire weather, which shows strong links to actual fire impacts (Bedia et al., 2015; Abatzoglou et al., 2018; Jones et al., 2022; see also Sect. S1 in the Supplement). In this study we mainly use FWI because fire weather simulations by ESMs relying only on atmospheric conditions are significantly more robust than ESM fire impact simulations due to limited performance of fire modules within CMIP6 models and dependence on land cover (Hantson et al., 2020; Gallo et al., 2023; Spafford and MacDougall, 2021).
To represent global forest fire danger, we compute the weighted average of FWISA over the global forest area, i.e., (see Appendix A for formula). This global indicator proves to be highly correlated with global burned forest area share. Also locally, FWI is spatially consistently in positive interannual correlation (weighted mean across forest areas R ≈ 0.3) with burned area (Abatzoglou et al., 2018; Bedia et al., 2015; Grillakis et al., 2022; Jones et al., 2022; see Fig. S1 in the Supplement and Sect. S1).
Compared to early industrial times, , computed over constant tree cover of 2020 from the satellite product ESA-CCI-LC (Harper et al., 2023), has substantially risen (Fig. 3, historical). For the period 2020 until 2050 under SSP1-2.6 the available CMIP6 models project a robust increase in fire weather intensity in most land areas, including all of South America, parts of North America, northern and southern Africa, western Eurasia, and Australia (Fig. 3b). Under higher warming scenarios, the danger increase would be substantially stronger (Fig. 3; see also Abatzoglou et al., 2019). From 2050 until the end of the century, the five climate scenarios from SSP1-1.9 (slight decline in global danger indicator ) to SSP5-8.5 ( increase doubles again) diverge substantially, mainly driven by heating.
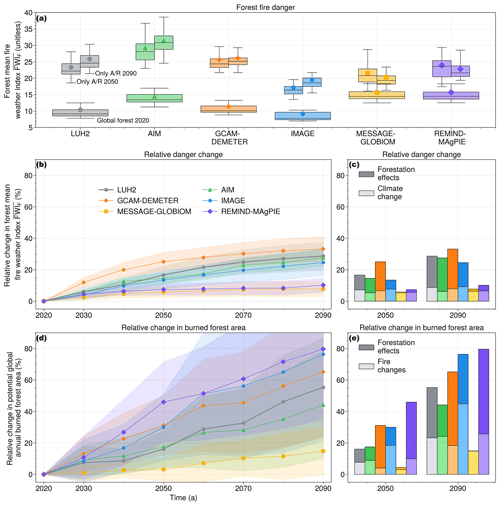
Figure 4(a) for all forests in 2020 (wide boxes) and for A/R areas only in 2050 and 2090 under SSP1-2.6 (upper small boxes). The distribution is given by fire weather projections from 22 Earth system models. For each IAM, the marker points to the multi-ESM mean, whereas the lines indicate the minimum as well as the 25th, 50th, and 75th percentile and the maximum. (b) and (d) Apot.burned change relative to 2020 under SSP1-2.6 for different forest projections. The shading indicates 1 standard deviation from the ESM uncertainty of FWI to burned area change. (c, e) Contributions to the relative change in and Apot.burned until 2050 and 2090 from forestation (top) and from fire (weather) change (bottom) are shown for the six datasets. For readability and interpretability, the range of relative change is cropped to 90 % in (b–e). A version with the full range including information on the share of annual burned forest area in 2020 is given in Fig. S3 in the Supplement. For the corresponding information on in SSP1-1.9, see Fig. S10 in the Supplement.
Over the course of the century, the projected forested regions in IAMs are increasingly under danger from fire weather (relative increase in of up to more than 30 %; Fig. 4b).
There are two reasons for this finding, which is robust against inter-ESM differences in fire weather (Fig. 4) and restrictions to areas where FWI is strongly correlated with fire impacts (Fig. S13 in the Supplement and Sect. S1 in the Supplement): first, fire weather intensifies through heating and drying trends over forest areas that already existed in 2020 as a consequence of climate change (hazard as a driver). Second, and more important, most of the increase in danger under SSP1-2.6 is driven by A/R in regions of already high and/or intensifying fire weather (exposure as a driver; see narrow boxes in Fig. 4a that are higher than broad boxes and darker than lighter bars in Figs. 4c; see also S5 in the Supplement for spatially explicit contributions, S10 for the scenario SSP1-19, and Sect. S2 in the Supplement for explanatory text and formula).
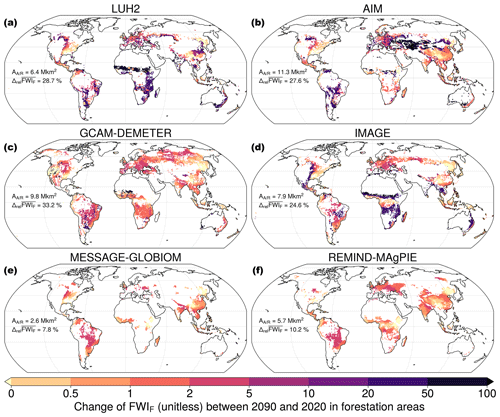
Figure 5Absolute change in danger (FWIF = FWI⋅aF), the product of the fire weather index (hazard) and forest grid cell share (exposure) under SSP1-2.6 in 2090 with respect to 2020 in forestation areas (ΔaF > 5 %) for LUH2 and five different IAMs. AA/R is the overall forestation area since 2020 and is the relative change in global forest-weighted mean FWI of each model.
This can be attributed to very strong land use policies in SSP1 (Popp et al., 2017), i.e., carbon pricing strongly rewarding expected carbon sequestration for A/R. We found to increase over the course of the century in both scenarios, SSP1-1.9 and SSP1-2.6, for all datasets. Moreover, in projected future afforestation areas is more than 1.3 times the for the 2020 forested area (Fig. 4a). Thus, the exposure to intense fire weather in the regions where A/R is projected to occur drives the overall increase in .
This increase in danger, mainly driven by exposure increases, is likely to propagate to fire impacts. Burned forest area is posed to rise under this pressure (according to our analysis by 10 %–80 %, depending on IAM and ESM projection) (Fig. 4d and e; see Appendix B and the Supplement for details). Typically, about half of this change can be attributed to A/R in areas of large or increasing annual burned area.
We illustrate A/R regions particularly endangered in the models with the absolute change in the product of hazard and exposure, FWI times aF over time (Fig. 5). The rise in danger is driven by a regionally varying intersection of up-sloping FWISA (hazard-driven) and forest cover share (exposure-driven; see Fig. S5 for spatially explicit signal decomposition and Fig. S8 in the Supplement for the full signal in 2050).
If areas for forestation were excluded when located in regions of extreme fire regimes (FWI > 47 in 2090, 95th percentile of historical conditions), the overall forestation would be reduced by 4 % (IMAGE) to 20 % (AIM) in 2090, clearly illustrating the potential impact of fire danger on forestation allocation.
Table 1Climate feedbacks into land use decisions in five IAMs for their most recent versions. The versions used in CMIP6 did not include any climate feedbacks (except IMAGE).
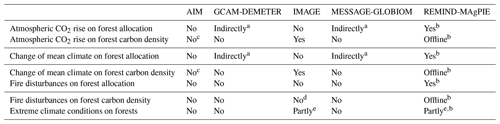
a Through impacts on productivity and water availability in agriculture. b Through impacts on the potential carbon density, which are provided by an offline simulation using the land model LPJmL. c AIM in principle includes this feedback. For the simulations assessed here it was not used for consistency within the multi-IAM mitigation scenario assessment. d In existing forests yes, in forest plantations used for afforestation no. e Through extreme temperature and water conditions limiting productivity and enhancing background mortality. Boreal trees can die from heat stress.
Generally, a higher FWI regime favors less dense forests and subsequently less effective A/R for negative emissions (Koch and Kaplan, 2022; Lasslop et al., 2020). Although forests exposed to high FWI are not guaranteed to burn more intensely or more often in every location, a long-term and large-scale average response in this direction is very likely, particularly in the absence of fire prevention measures (IAMs do not include the costs for these to date). Such fire prevention may be effective in the medium term (Wu et al., 2021) but is often unsustainable on longer timescales (Moya et al., 2019; Bowman et al., 2009).
Fire weather and burned area used here are examples to highlight this deficiency. Beyond fire, we expect impacts from other extreme events (e.g., droughts, heatwaves, and heavy precipitation) to also play a role in land use decisions (Dale et al., 2001; Johnstone et al., 2016; Seidl et al., 2017; Thom and Seidl, 2016; Hammond et al., 2022). Therefore, we highlight the need to implement more climate impacts into IAMs, which helps to avoid an overestimation of the negative emission potential of A/R.
None of the modeling frameworks to date explicitly include a broad range of impacts from climate extremes on vegetation. This is troublesome given that increases in climate extremes are already expected in all regions at 1.5 or 2 °C of global warming (Seneviratne et al., 2021). Here, we provide a short overview of whether the analyzed scenarios are produced considering climate effects (feedbacks) for A/R decisions in the associated IAM versions (see Table 1, and for more details, please refer to Appendix C).
A main entry point for climate feedbacks into IAMs is to modify carbon densities because the total amount of carbon stored in forests in IAM projections is typically estimated using forest carbon density ([ρC] = t ha−1) and forest fractional coverage ([aF] = ha ha−1) (Humpenöder et al., 2014; see external vegetation data in Fig. 2). Typically, the carbon content of forests is modeled to monotonously grow towards the determined maximum estimate given by vegetation models, which might include upward or downward impacts from productivity and disturbance shifts under climate change. For long-term and large-scale averaged results, this provides an approximation relevant for impacts on A/R allocation for carbon uptake. The long-term increase in the carbon stored through A/R is counted as negative emission in IAMs. As a low-cost negative emission option in ambitious mitigation scenarios, A/R allows for residual hard-to-avoid GHG emissions compatible with the target of net-zero emissions. The more areas available for low-cost A/R potential, the larger the potential for residual GHG emissions compatible with a low warming target (Humpenöder et al., 2014; Doelman et al., 2020; Frank et al., 2021).
Among the models analyzed here, only IMAGE (existing forests, not in A/R areas) and REMIND-MAgPIE (all forests) account for carbon losses from changing climate conditions including fire in their estimates of forest carbon density. IMAGE and REMIND-MAgPIE include information on climate impacts from the vegetation model LPJmL (Doelman et al., 2020; Humpenöder et al., 2022), which accounts for day-to-day variability but does not include natural disturbances like fire on this timescale (Schaphoff et al., 2018; Braakhekke et al., 2019). In other IAMs, such processes are not included. Therefore, these models are expected to produce overly optimistic estimates of A/R effectiveness. Except IMAGE 3.0, the IAM providing the SSP1 simulations (Stehfest et al., 2014; O'Neill et al., 2016), the model versions used for projections under CMIP6 and LUH2 did not include any climate feedbacks.
The forestation allocation in REMIND-MAgPIE among the other models shows comparably high consistency and flexibility, which is also reflected by its performance to distribute large-scale A/R to comparably mild fire conditions (compare AA/R and in Fig. 5 and Ω in Table S1 in the Supplement and Sect. S4 in the Supplement). The model shows a comparably small increase in , partially owing to incorporated climate information, but also due to the incorporation of national tree planting pledges and a high baseline (2020). Only MESSAGE-GLOBIOM has even smaller , likely stemming from the much smaller A/R volume in that projection.
The majority of models used in the scenario framework under CMIP6 are at the beginning of including more climate change impact information into their modeling schemes. In addition to IMAGE and REMIND-MAgPIE, GCAM-DEMETER (Chen et al., 2022, 2019) and MESSAGE-GLOBIOM (Frank et al., 2021) already include some scheme for climate impacts on water availability and productivity in agriculture, but not in forestry (see Table 1 and Figs. S7 and S12 in the Supplement as well as Sect. S8 in the Supplement). In the MESSAGE-GLOBIOM framework, there are efforts underway to make the forestry model, G4M, climate-informed. Those models that already include detailed climate information typically find increases in carbon density due to carbon fertilization from increased CO2 levels in the atmosphere. Even though detailed, these estimates might be overly optimistic because they exclude other disturbances leading to carbon losses from explicit modeling, which enables accounting for regimes shifts, disturbance interaction, and changes over time.
So how can information on disturbances of the carbon pools in forests be propagated into globally modeled land use and land management? Only one of the assessed models covers the impact of changing carbon sequestration potential on land use decisions. REMIND-MAgPIE assumes a 30-year horizon of foresight of expected future carbon stocks for the distribution of land use including afforestation. Following this example, we argue for a consistent and physically meaningful integration of climate impacts through the effect on carbon density of vegetation. This will also support the tracking of contributions to uncertainty along the model cycle from vegetation to land use to climate and back to vegetation (Fig. 2; see the Supplement for an example of climate model uncertainty implementation). Sensitivity studies using explicit climate impact representations in IAMs, e.g., modeling carbon density evolution interactively in REMIND-MAgPIE, including probabilistic estimates of climate impacts on land use, would help quantitatively evaluate the sensitivity and reliability of IAM results across spatial and temporal scales. Additionally internalizing the expected increase in costs in fire prevention measures and changes to ecosystem services from, e.g., biodiversity, could help to make forestation projections more comprehensive (Pawson et al., 2013; Hansen et al., 2001; Prestele et al., 2016).
Our work highlights the need for improvements in the difficult task of estimating forestation potential in multi-sectoral assessments (on non-technical challenges; see, e.g., Hollnaicher, 2022) and proposes ways to address this issue. We showed the forestation potential under SSP1-2.6 modeled in the presented IAM simulations to be severely compromised by fire risk. Including such risk into the assessment will likely diminish the role of forestation in the mitigation portfolio. Given the closing window of opportunity for limiting global warming to 1.5 °C, these results demonstrate that a climate mitigation strategy minimizing the risks of temperature overshoot must be centered on rapid reduction of carbon emissions.
To get a global indicator for forest exposure to fire weather, we calculate a mean FWI weighted by forest fractional area. For fire weather we use an annual indicator of fire season intensity, namely the yearly maximum of the 90 d running average daily Canadian FWI (Quilcaille and Batibeniz, 2022). While this index of the seasonal fire weather intensity best fits our long-term and global-scale goals, our analysis is not sensitive to the use of other measures for fire season intensity or length aggregated from daily FWI data (see, e.g., Quilcaille and Batibeniz, 2022, for such indicators). We are not interested in single extreme days but in the smooth trends of extreme fire hazard from changing atmospheric conditions on a heated planet. This is why the time series of annual values is smoothed with a running 10-year mean such that the 10-yearly maps of forest exposure from IAMs can be matched with climatic conditions representing changes on the same timescale. Additionally, FWI data were regridded to the 0.5° × 0.5° mesh the IAM forest cover data were assembled on. The FWI is computed from temperature, precipitation, relative humidity, and surface wind projections of Earth system models (ESMs) participating in CMIP6 and providing the necessary variables. For our analysis we either used 10 models that had produced simulations not only for SSP1-2.6, SSP2-4.5, SSP3-7.0, and SSP5-8.5, but also for SSP1-1.9, a Tier 2 numerical experiment in CMIP6 (Fig. 3), or we used all 22 models providing the SSP1-2.6 simulation (Figs. 4 and 5). The 10 models providing SSP1-1.9 are CanESM5 (50), EC-Earth3 (1), FGOALS-g3 (1), GFDL-ESM4 (1), IPSL-CM6A-LR (6), MIROC-ES2L (10), MIROC6 (3), MPI-ESM1-2-LR (30), MRI-ESM2-0 (5), and UKESM1-0-LL (5), with the respective number of ensemble members in parentheses. For the main analysis of danger evolution under SSP1-2.6, we used ACCESS-CM2 (5), ACCESS-ESM1-5 (40), CMCC-CM2-SR5 (1), CMCC-ESM2 (1), CanESM5 (50), EC-Earth3 (6), FGOALS-g3 (3), GFDL-ESM4 (1), HadGEM3-GC31-LL (1), HadGEM3-GC31-MM (1), INM-CM4-8 (1), INM-CM5-0 (1), IPSL-CM6A-LR (6), KACE-1-0-G (3), MIROC-ES2L (10), MIROC6 (50), MPI-ESM1-2-HR (2), MPI-ESM1-2-LR (30), MRI-ESM2-0 (5), NorESM2-MM (1), TaiESM1 (1), and UKESM1-0-LL (13) to avoid a bias in the model selection. The model mean and median were computed over ensemble mean values per model. We did not account for model similarities with further weighting among ESMs. However, the qualitative findings about the relative change in danger from forest expansion and fire weather intensification of the order of 10 % are not sensitive to model ensemble design choices.
The mean FWI weighted by forest fractional area aF is then computed by global integration of the product of and :
where dA is the areal increment, a product of the length increments in the zonal (x) and meridional (y) direction.
The value of global weighted mean FWI, , was tested to be good predictor of global burned area share. The Pearson correlation is above 0.75 for five out of six IAMs. The value for MESSAGE-GLOBIOM still showing a correlation of 0.56 could arise from generally low levels of burned area. This suggests that long-term and large-scale signals of global forest-area-weighted mean FWI change propagate to burned area change (Fig. 4d and e). While this holds for global values, local vulnerability, which is not included in this work, is expected to significantly modulate the combination of hazard and exposure we present here. For example, Zheng et al. (2023) find different responses of North American and Siberian boreal forests to climatic water deficits and extreme temperature, suggesting different vulnerabilities.
To get an estimate of the order of magnitude of change in burned area (BA) from A/R and climate change under SSP1-26, we use the output of land models within CMIP6 ESMs, BurntFractionAll, which is the monthly grid cell share burned in fire. Assuming that an area already burned in 1 year cannot burn again in the same one, we treat these monthly values as additive to reach annual values for six different ESMs, namely CESM2 (3), CESM2-WACCM (1), CMCC-CM2-SR5 (1), CMCC-ESM2 (1), CNRM-ESM2-1 (5), and EC-Earth3-Veg (2), with the respective number of ensemble members in parentheses. While this approach can give an indication of the order of magnitude of relative change, because these models reproduce overall patterns and natural trends, the performance of CMIP6 models concerning BA must be critically reflected upon (Hantson et al., 2020; Spafford and MacDougall, 2021). Here, we included all models providing BurntFractionAll and ESM tree fraction, treeFrac. Furthermore, we assume grasslands and other natural land as given by the IAM land cover and land use datasets to burn before forest is affected (threshold burning in Fig. S2 in the Supplement).
Note that BurntFractionAll from the CMIP6 land models relies on different spatial patterns and grid cell shares of forest cover from the IAM projection. Hence, a transfer of this value and its application to IAM land cover and land use can only serve as a first-order estimate and cannot replace a work-intense detailed vegetation modeling exercise with the IAM's land cover projection as input, which is left for future work and out of scope for this perspective. While such choices of method (proportional vs. threshold burning, which other land cover types to burn before forest, projecting BurntFractionAll directly on IAM land cover) seem to affect our results quantitatively, the overall finding of significantly up-sloping global areas of burned forest from A/R leading to increased risk (10 % < ΔrelApot.burned < 100 % between 2020 and 2090) is robustly maintained under our sensitivity assessments.
This section gives an overview of the configurations and specifications of the models considered in this study (see Table C1), which can help us to better understand the land use and land cover projections (see also Fig. S6 in the Supplement).
Hurtt et al. (2020)Fujimori et al. (2017)Chen et al. (2022, 2019)Doelman et al. (2022)Schaphoff et al. (2018)Frank et al. (2021); Rogelj et al. (2018)Humpenöder et al. (2022)Table C1Configurations of land use models used in IAMs for the datasets included in this study.
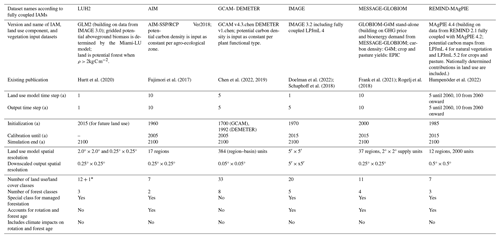
∗ LUH2 treats added tree cover separated from other forest classes to fit forest expansion in the IMAGE SSP1-2.6 and SSP1-1.9 land use simulation.
In most IAMs the amount of A/R results from land use decisions based on cost minimization (Humpenöder et al., 2015; Hasegawa et al., 2017; Doelman et al., 2020). The presented ensemble of large-scale afforestation scenarios has shared assumptions encoded in the Shared Socioeconomic Pathway 1 (SSP1, van Vuuren et al., 2017b) with the climate mitigation policy options SSP1-2.6 and SSP1-1.9. Most importantly, these assumptions include a global price on carbon emissions and emission budgets in the mitigation scenarios that are roughly compatible with warming levels of 2.0 and 1.5 °C. Still, there are many differences in model setup and in the underlying assumptions remaining. The value of a growing forest carbon stock is implemented using a carbon price, which, depending on the model, is determined either in the energy sector model (Popp et al., 2017) or from a comprehensive simulation of energy sector and land use (Krey et al., 2020; Wise et al., 2009). This price is designed to keep carbon emissions compatible with a certain carbon budget and a climate target (here with 1.5 or 2 °C of global warming) across economic sectors. Changing land use from pasture to managed forest, for example, is rewarded with a price corresponding to the additional amount of carbon stored or expected to be stored. The equivalent is included in the computation as a cost in the case of deforestation and the corresponding drop in carbon density. Consequently, the level of the carbon price drives modeled A/R, especially where potential revenues from forestry aside from this price are comparable with or lower than those from other land uses. Ultimately, global estimates of A/R volume are sensitive to its local effectiveness to store carbon and to deliver multiple forest products (Humpenöder et al., 2014; Doelman et al., 2020; Frank et al., 2021).
In the model chain from IAMs to ESMs (Fig. 2), LUH was developed to harmonize historical land information and the land use outputs of IAMs for use within ESMs (Hurtt et al., 2011). The second generation of LUH under CMIP6, LUH2, provides land use and land cover change (LULCC) information in one classification for all scenarios, which was computed by different models with different land use and land cover classifications (Hurtt et al., 2020). A harmonized dataset fit to the original land use datasets was produced using the Global Land Model. While globally aggregated LULCC (e.g., global pasture or forest area change) was conserved, the spatial patterns of forest were not. This leads to LUH2 projections, also assessed here, showing different forest exposures to climate impacts such as fire weather than the IAM projections.
IMAGE and REMIND-MAgPIE include information on climate impacts from the vegetation model LPJmL (Doelman et al., 2020; Humpenöder et al., 2022). LPJmL computes in daily time steps and hence accounts for day-to-day variability. This allows the modeling of vegetation response to climate on a sub-annual timescale via altered productivity and background mortality. Natural disturbances, such as fire and heat stress for boreal trees, are nevertheless modeled in annual time steps (Schaphoff et al., 2018; Braakhekke et al., 2019). Although this has not been assessed for all circumstances of post-disturbance vegetation dynamics, LPJmL in principle has the mechanisms implemented to represent some climate-specific vegetation response to disturbance (Ostberg et al., 2015). In the IMAGE framework, LPJmL in an online mode informs the land use module annually, e.g., about carbon density using daily values emulated from annual climate provided by MAGICC, a simple climate model (Doelman et al., 2020). Fire impacts on carbon density are included in natural forest dynamics but are excluded in forest plantations used for A/R. REMIND-MAgPIE is informed by crop and vegetation data modeled offline by LPJmL (Humpenöder et al., 2015), which is operated in daily time steps with daily climate data (Schaphoff et al., 2018). The limitation for the representation of vegetation impacts of climate extremes on sub-annual timescale therefore lies in the climate variables used and the physical processes represented in LPJmL. REMIND-MAgPIE offers an option to model fire emissions and heat stress of boreal forests but currently no additional explicit disturbance processes which lead to carbon losses. Typically, extreme conditions significantly lower the modeled productivity and enhance background mortality (Schaphoff et al., 2018). With a corresponding calibration (Forkel et al., 2019) LPJmL allows for an implicit representation of disturbances within the regimes provided by the calibration datasets. REMIND-MAgPIE uses annual potential carbon density, the maximum attainable carbon density of forests, which is modulated by changing climate in the model LPJmL and determined by aggregating from daily to annual values.
In GCAM-DEMETER and AIM the potential carbon stocks are represented more simply and are not even spatially explicit; they are assumed to be constant within forest classes and/or economic regions.
The assessed versions of MESSAGE-GLOBIOM and GCAM-DEMETER only include climate impacts on croplands, not on forests. In MESSAGE-GLOBIOM this leads to significant shifts from forest to cropland in the comparison of a climate impact (in this model mainly CO2 fertilization and mean climate; see Table 1) to a no-climate-impact scenario. This shows that differential treatment of land types can lead to imbalances in land use changes, in this case leading to less forestation on former cropland under a simulation including climate impacts on agriculture. We refer to this effect as “indirect impacts on forest allocation” in Table 1. Note that even this indirect tendency of less forestation under climate change, likely induced by productivity-enhancing climate impacts on cropland, is significantly stronger than variations generated by the six different climate models forcing the climate impacts (see Figs. S7, S12 and Sect. S8).
Overall, IAMs and LUH2 have different initial land cover and land use inventories, starting dates, time steps, modeling procedures, spatial resolutions, and classification schemes, leading to differences in both past and present land use (Bayer et al., 2021; Brown et al., 2021). For example, as the only IAM in this ensemble, REMIND-MAgPIE includes nationally determined contributions (NDCs) in land use in the model. For the present study the gridded forest cover data were aggregated to the finest common resolution in space and time: 0.5° × 0.5° and 10 years. The available forest classes were aggregated into one. The expansion of forest area in these afforestation scenarios is dominated by managed forestation in the datasets which provide such separate classes.
Some forest management options beyond A/R might be at risk in a more fire-prone future climate. Four out of six IAM datasets account for rotations and forest age, but none of them have any mechanism of climate impacts on management in forestry.
We compare present-day values of forest area in IAM projections with the forest area in observational datasets to assess the plausibility of past and present land cover and land use. It should be noted though that there is also substantial disagreement between observational datasets themselves. For example, satellite imagery cannot distinguish all kinds of land cover and different classification schemes give different global tree cover areas (Harper et al., 2023; Buchhorn, 2020, Fig. S6). National inventories combined in FAO forest resource assessments (FAO, 2020) give slightly higher values. Locally, i.e., at grid cell scale (≈ 0.5°), the representation of forest cover in IAM datasets compared to satellite products is moderate to weak owing to the substantial differences in ecosystem and landscape classification. Overall, the range of global forest areas in the IAM datasets for the present day is compatible with observational datasets while showing a much larger spread. In 2020, the first common date of the model outputs, the standard deviation of global forest area among the datasets amounts to 3.2 Mkm2. Under SSP1-2.6 it grows to 6.2 Mkm2 over the course of the century.
Having mentioned the limitations of our analysis underlying the perspective along the main text, we summarize them here for a concise overview and argue why our perspective holds under these limitations.
Our focus on land use projections by models that provided land inputs to CMIP6 limits our analysis to these few most prominent models. Our analysis is limited by the choice of climatic variables. Fire weather is a measure for fire hazard together with exposure, not fully capturing fire risk (in terms of, e.g., carbon emissions) or covering the entire spectrum of environmental stress. Using burned area from fire models within ESMs bridges the gap to fire impact but is heavily reliant on the assumptions around impact transfer from ESM to IAM land cover. More importantly, the performance of fire impacts from ESMs is very limited (Hantson et al., 2020; Spafford and MacDougall, 2021). However, as fire models in state-of-the-art ESMs tend to underestimate fire impacts, our assessment remains a conservative one; hence, this limitation does not affect the stringency of our main line of thought, namely the argument for more precise input and use of climate-related forest impacts in IAMs.
Additionally, we take feedbacks of A/R on climate and changing vulnerabilities into account only to a limited extent. For example, temperature and precipitation are expected to change in tropical regions with changed forest cover (Li et al., 2022). While we do use the climate projections under SSP1-2.6, which include land cover change (and A/R) according to the LUH2 dataset, these do not match the A/R patterns of the IAMs exactly. However, as our analysis of the LUH2 forest cover does not show significantly lower danger from land-cover-change-induced climate changes, we expect this to be a minor issue. Importantly, the identified signal of increasing danger, mainly driven by exposure increase, is extraordinarily strong and emphasizes the need to consider more climate information in land use projections. This is particularly relevant given the range of other regional increases in climate extremes, such as heatwaves, droughts, and heavy precipitation events, that are projected even at 1.5°C or 2°C of global warming (Seneviratne et al., 2021).
Land cover satellite products from ESA-CCI and CGLS are available at http://maps.elie.ucl.ac.be/CCI/viewer/download.php (Harper et al., 2023) and https://land.copernicus.eu/global/products/lc (Buchhorn, 2020), respectively. Land cover data from GCAM-DEMETER are published in Chen et al. (2020) under https://doi.org/10.25584/data.2020-07.1357/1644253 (Chen and Vernon, 2020). AIM land use data are available under https://doi.org/10.18959/20180403.001 (National Institute for Environmental Studies, 2018). Harmonized land use datasets from LUH2 are accessible under https://luh.umd.edu/data.shtml (Hurtt et al., 2019). Land use data from IMAGE, MESSAGEix-GLOBIOM, and REMIND-MAgPIE are available in the harmonized multi-IAM land use dataset (https://doi.org/10.5281/zenodo.12627389, Jäger, 2024). Fire weather index data from CMIP6 in Quilcaille and Batibeniz (2022) are available at http://hdl.handle.net/20.500.11850/583391 (Quilcaille et al., 2023). Data from CMIP6 are available at https://esgf-node.llnl.gov/search/cmip6/, last access: 12 May 2022. The experiments and variables used can be found with a search query using the relevant experiment ID (historical, ssp119, ssp126) and variable (treeFrac, BurntFractionAll).
The computer code used for the analysis of the forest-weighted mean fire weather index and a corresponding manual on how to apply this code are openly available at https://doi.org/10.5281/zenodo.11068957 (Jäger et al., 2024).
The supplement related to this article is available online at: https://doi.org/10.5194/esd-15-1055-2024-supplement.
This research is part of the PhD work of FJ under the supervision of SIS and JS at ETH Zurich. FJ, JS, YQ, and SIS conceptualized the perspective and analysis. FJ performed the data analysis, produced the figures, and drafted this manuscript. MWi, FH, JD, SF, MWö, and MG helped with land use and land cover data provision of the different integrated assessment models. YQ calculated the FWI from CMIP6 climate data. JS, YQ, SIS, MWi, JD, SF, PH, FH, ALDA, CM, KBN, RSP, AP, and DvV contributed to the interpretation of the analysis and the overall perspective and improved the manuscript.
At least one of the (co-)authors is a member of the editorial board of Earth System Dynamics. The peer-review process was guided by an independent editor, and the authors also have no other competing interests to declare.
Publisher's note: Copernicus Publications remains neutral with regard to jurisdictional claims made in the text, published maps, institutional affiliations, or any other geographical representation in this paper. While Copernicus Publications makes every effort to include appropriate place names, the final responsibility lies with the authors.
We acknowledge the Potsdam Institute for Climate Impact Research, the PBL Netherlands Environmental Assessment Agency, the International Institute for Applied Systems Analysis, and the Joint Global Change Research Institute for the production and provision of the land use and land cover data. Data on burned area and tree fractional coverage produced under CMIP6 are provided by the WCRP (World Climate Research Program) and ESFG (Earth System Grid Federation). To improve the linguistic expression in some passages within this manuscript, ChatGPT was used on an earlier version of the paper. We acknowledge the development and provision of the Python packages and wrappers maplotlib (Hunter, 2007), numpy (Harris et al., 2020), proplot (Davis, 2021), scipy (Virtanen et al., 2020), and xarray (Hoyer and Hamman, 2017). We thank Louise Parsons Chini, Shinichiro Fujimori, George Hurtt, Andreia Ribeiro, and Marshall Wise as well as Zhengyang Lin and one anonymous reviewer for very helpful comments.
This research has been supported by the H2020 European Research Council (grant nos. 101003687, 101056939, and 101056875), the European Research Council FP7 Ideas: European Research Council (grant no. 964013), and the Staatssekretariat für Bildung, Forschung und Innovation.
This paper was edited by Anping Chen and reviewed by Zhengyang Lin and one anonymous referee.
Abatzoglou, J. T., Williams, A. P., Boschetti, L., Zubkova, M., and Kolden, C. A.: Global patterns of interannual climate–fire relationships, Glob. Change Biol., 24, 5164–5175, https://doi.org/10.1111/gcb.14405, 2018. a, b, c
Abatzoglou, J. T., Williams, A. P., and Barbero, R.: Global Emergence of Anthropogenic Climate Change in Fire Weather Indices, Geophys. Res. Lett., 46, 326–336, https://doi.org/10.1029/2018GL080959, 2019. a, b
Anderegg, W. R., Trugman, A. T., Badgley, G., Anderson, C. M., Bartuska, A., Ciais, P., Cullenward, D., Field, C. B., Freeman, J., Goetz, S. J., Hicke, J. A., Huntzinger, D., Jackson, R. B., Nickerson, J., Pacala, S., and Randerson, J. T.: Climate-driven risks to the climate mitigation potential of forests, Science, 368, 6497, https://doi.org/10.1126/science.aaz7005, 2020. a
Bayer, A. D., Fuchs, R., Mey, R., Krause, A., Verburg, P. H., Anthoni, P., and Arneth, A.: Diverging land-use projections cause large variability in their impacts on ecosystems and related indicators for ecosystem services, Earth Syst. Dynam., 12, 327–351, https://doi.org/10.5194/esd-12-327-2021, 2021. a
Bedia, J., Herrera, S., Gutiérrez, J. M., Benali, A., Brands, S., Mota, B., and Moreno, J. M.: Global patterns in the sensitivity of burned area to fire-weather: Implications for climate change, Agr. Forest Meteorol., 214-215, 369–379, https://doi.org/10.1016/j.agrformet.2015.09.002, 2015. a, b, c
Bowman, D. M. J. S., Balch, J. K., Artaxo, P., Bond, W. J., Carlson, J. M., Cochrane, M. A., D'Antonio, C. M., DeFries, R. S., Doyle, J. C., Harrison, S. P., Johnston, F. H., Keeley, J. E., Krawchuk, M. A., Kull, C. A., Marston, J. B., Moritz, M. A., Prentice, I. C., Roos, C. I., Scott, A. C., Swetnam, T. W., van der Werf, G. R., and Pyne, S. J.: Fire in the Earth System, Science, 324, 481–484, https://doi.org/10.1126/science.1163886, 2009. a
Braakhekke, M. C., Doelman, J. C., Baas, P., Müller, C., Schaphoff, S., Stehfest, E., and van Vuuren, D. P.: Modeling forest plantations for carbon uptake with the LPJmL dynamic global vegetation model, Earth Syst. Dynam., 10, 617–630, https://doi.org/10.5194/esd-10-617-2019, 2019. a, b
Brown, C., Holman, I., and Rounsevell, M.: How modelling paradigms affect simulated future land use change, Earth Syst. Dynam., 12, 211–231, https://doi.org/10.5194/esd-12-211-2021, 2021. a, b
Buchhorn, M., Smets, B., Bertels, L., De Roo, B., Lesiv, M., Tsendbazar, N.-E, Li, L., and Tarko, A.: Copernicus Global Land Service: Land Cover 100m: Version 3 Globe 2015–2019: Product User Manual, Zenodo, https://doi.org/10.5281/zenodo.3938963, 2020. a, b, c
Chen, M. and Vernon, C. R.: GCAM-Demeter-LU, DataHub [data set], https://doi.org/10.25584/data.2020-04.1188/1614678, 2020. a
Chen, G., Li, X., and Liu, X.: Global land projection based on plant functional types with a 1-km resolution under socio-climatic scenarios, Scientific Data, 9, 125, https://doi.org/10.1038/s41597-022-01208-6, 2022. a, b
Chen, H., Zeng, Z., Wu, J., Peng, L., Lakshmi, V., Yang, H., and Liu, J.: Large uncertainty on forest area change in the early 21st century among widely used global land cover datasets, Remote Sens.-Basel, 12, 1–18, https://doi.org/10.3390/rs12213502, 2020. a, b
Chen, M., Vernon, C. R., Huang, M., Calvin, K. V., and Kraucunas, I. P.: Calibration and analysis of the uncertainty in downscaling global land use and land cover projections from GCAM using Demeter (v1.0.0), Geosci. Model Dev., 12, 1753–1764, https://doi.org/10.5194/gmd-12-1753-2019, 2019. a, b
Clarke, H., Nolan, R. H., Dios, V. R. D., Bradstock, R., Griebel, A., Khanal, S., and Boer, M. M.: Forest fire threatens global carbon sinks and population centres under rising atmospheric water demand, Nat. Commun., 13, 7161, https://doi.org/10.1038/s41467-022-34966-3, 2022. a
Dale, V. H., Joyce, L. A., McNulty, S., Neilson, R. P., Ayres, M. P., Flannigan, M. D., Hanson, P. J., Irland, L. C., Lugo, A. E., Peterson, C. J., Simberloff, D., Swanson, F. J., Stocks, B. J., and Wotton, B. M.: Climate Change and Forest Disturbances: Climate change can affect forests by altering the frequency, intensity, duration, and timing of fire, drought, introduced species, insect and pathogen outbreaks, hurricanes, windstorms, ice storms, or landslides, BioScience, 51, 723–734, https://doi.org/10.1641/0006-3568(2001)051[0723:CCAFD]2.0.CO;2, 2001. a
Davies-Barnard, T., Catto, J. L., Harper, A. B., Imron, M. A., and van Veen, F. J. F.: Future fire risk under climate change and deforestation scenarios in tropical Borneo, Environ. Res. Lett., 18, 024015, https://doi.org/10.1088/1748-9326/acb225, 2023. a
Davis, L. L. B.: ProPlot, Zenodo, https://doi.org/10.5281/zenodo.5602155, 2021. a
Doelman, J. C., Stehfest, E., van Vuuren, D. P., Tabeau, A., Hof, A. F., Braakhekke, M. C., Gernaat, D. E., van den Berg, M., van Zeist, W. J., Daioglou, V., van Meijl, H., and Lucas, P. L.: Afforestation for climate change mitigation: Potentials, risks and trade-offs, Glob. Change Biol., 26, 1576–1591, https://doi.org/10.1111/gcb.14887, 2020. a, b, c, d, e, f
Doelman, J. C., Beier, F. D., Stehfest, E., Bodirsky, B. L., Beusen, A. H. W., Humpenöder, F., Mishra, A., Popp, A., van Vuuren, D. P., de Vos, L., Weindl, I., van Zeist, W.-J., and Kram, T.: Quantifying synergies and trade-offs in the global water-land-food-climate nexus using a multi-model scenario approach, Environ. Res. Lett., 17, 045004, https://doi.org/10.1088/1748-9326/ac5766, 2022. a
Fan, L., Wigneron, J.-P., Ciais, P., Chave, J., Brandt, M., Sitch, S., Yue, C., Bastos, A., Li, X., Qin, Y., Yuan, W., Schepaschenko, D., Mukhortova, L., Li, X., Liu, X., Wang, M., Frappart, F., Xiao, X., Chen, J., Ma, M., Wen, J., Chen, X., Yang, H., van Wees, D., and Fensholt, R.: Siberian carbon sink reduced by forest disturbances, Nat. Geosci., 16, 56–62, https://doi.org/10.1038/s41561-022-01087-x, 2023. a
FAO: Global forest resources assessment 2015: how are the world's forests changing?, Forest Ecol. Manag., 352, 3–8, https://doi.org/10.1016/j.foreco.2015.02.006, 2016. a
FAO: Global Forest Resources Assessment 2020, FAO, https://doi.org/10.4060/ca9825en, 2020. a, b
Forkel, M., Drüke, M., Thurner, M., Dorigo, W., Schaphoff, S., Thonicke, K., von Bloh, W., and Carvalhais, N.: Constraining modelled global vegetation dynamics and carbon turnover using multiple satellite observations, Sci. Rep.-UK, 9, 18757, https://doi.org/10.1038/s41598-019-55187-7, 2019. a
Frank, S., Gusti, M., Havlík, P., Lauri, P., DiFulvio, F., Forsell, N., Hasegawa, T., Krisztin, T., Palazzo, A., and Valin, H.: Land-based climate change mitigation potentials within the agenda for sustainable development, Environ. Res. Lett., 16, 024006, https://doi.org/10.1088/1748-9326/abc58a, 2021. a, b, c, d
Friedlingstein, P., O'Sullivan, M., Jones, M. W., Andrew, R. M., Bakker, D. C. E., Hauck, J., Landschützer, P., Le Quéré, C., Luijkx, I. T., Peters, G. P., Peters, W., Pongratz, J., Schwingshackl, C., Sitch, S., Canadell, J. G., Ciais, P., Jackson, R. B., Alin, S. R., Anthoni, P., Barbero, L., Bates, N. R., Becker, M., Bellouin, N., Decharme, B., Bopp, L., Brasika, I. B. M., Cadule, P., Chamberlain, M. A., Chandra, N., Chau, T.-T.-T., Chevallier, F., Chini, L. P., Cronin, M., Dou, X., Enyo, K., Evans, W., Falk, S., Feely, R. A., Feng, L., Ford, D. J., Gasser, T., Ghattas, J., Gkritzalis, T., Grassi, G., Gregor, L., Gruber, N., Gürses, Ö., Harris, I., Hefner, M., Heinke, J., Houghton, R. A., Hurtt, G. C., Iida, Y., Ilyina, T., Jacobson, A. R., Jain, A., Jarníková, T., Jersild, A., Jiang, F., Jin, Z., Joos, F., Kato, E., Keeling, R. F., Kennedy, D., Klein Goldewijk, K., Knauer, J., Korsbakken, J. I., Körtzinger, A., Lan, X., Lefèvre, N., Li, H., Liu, J., Liu, Z., Ma, L., Marland, G., Mayot, N., McGuire, P. C., McKinley, G. A., Meyer, G., Morgan, E. J., Munro, D. R., Nakaoka, S.-I., Niwa, Y., O'Brien, K. M., Olsen, A., Omar, A. M., Ono, T., Paulsen, M., Pierrot, D., Pocock, K., Poulter, B., Powis, C. M., Rehder, G., Resplandy, L., Robertson, E., Rödenbeck, C., Rosan, T. M., Schwinger, J., Séférian, R., Smallman, T. L., Smith, S. M., Sospedra-Alfonso, R., Sun, Q., Sutton, A. J., Sweeney, C., Takao, S., Tans, P. P., Tian, H., Tilbrook, B., Tsujino, H., Tubiello, F., van der Werf, G. R., van Ooijen, E., Wanninkhof, R., Watanabe, M., Wimart-Rousseau, C., Yang, D., Yang, X., Yuan, W., Yue, X., Zaehle, S., Zeng, J., and Zheng, B.: Global Carbon Budget 2023, Earth Syst. Sci. Data, 15, 5301–5369, https://doi.org/10.5194/essd-15-5301-2023, 2023. a
Fujimori, S., Hasegawa, T., Masui, T., Takahashi, K., Herran, D. S., Dai, H., Hijioka, Y., and Kainuma, M.: SSP3: AIM implementation of Shared Socioeconomic Pathways, Global Environ. Chang., 42, 268–283, https://doi.org/10.1016/j.gloenvcha.2016.06.009, 2017. a
Fuss, S., Lamb, W. F., Callaghan, M. W., Hilaire, J., Creutzig, F., Amann, T., Beringer, T., de Oliveira Garcia, W., Hartmann, J., Khanna, T., Luderer, G., Nemet, G. F., Rogelj, J., Smith, P., Vicente, J. L. V., Wilcox, J., del Mar Zamora Dominguez, M., and Minx, J. C.: Negative emissions—Part 2: Costs, potentials and side effects, Environ. Res. Lett., 13, 063002, https://doi.org/10.1088/1748-9326/aabf9f, 2018. a
Gallo, C., Eden, J. M., Dieppois, B., Drobyshev, I., Fulé, P. Z., San-Miguel-Ayanz, J., and Blackett, M.: Evaluation of CMIP6 model performances in simulating fire weather spatiotemporal variability on global and regional scales, Geosci. Model Dev., 16, 3103–3122, https://doi.org/10.5194/gmd-16-3103-2023, 2023. a
Golub, A., Sohngen, B., Cai, Y., Kim, J., and Hertel, T.: Costs of forest carbon sequestration in the presence of climate change impacts, Environ. Res. Lett., 17, 104011, https://doi.org/10.1088/1748-9326/ac8ec5, 2022. a
Grillakis, M., Voulgarakis, A., Rovithakis, A., Seiradakis, K. D., Koutroulis, A., Field, R. D., Kasoar, M., Papadopoulos, A., and Lazaridis, M.: Climate drivers of global wildfire burned area, Environ. Res. Lett., 17, 045021, https://doi.org/10.1088/1748-9326/ac5fa1, 2022. a
Griscom, B. W., Adams, J., Ellis, P. W., Houghton, R. A., Lomax, G., Miteva, D. A., Schlesinger, W. H., Shoch, D., Siikamäki, J. V., Smith, P., Woodbury, P., Zganjar, C., Blackman, A., Campari, J., Conant, R. T., Delgado, C., Elias, P., Gopalakrishna, T., Hamsik, M. R., Herrero, M., Kiesecker, J., Landis, E., Laestadius, L., Leavitt, S. M., Minnemeyer, S., Polasky, S., Potapov, P., Putz, F. E., Sanderman, J., Silvius, M., Wollenberg, E., and Fargione, J.: Natural climate solutions, P. Natl. Acad. Sci. USA, 114, 11645–11650, https://doi.org/10.1073/pnas.1710465114, 2017. a
Hammond, W. M., Williams, A. P., Abatzoglou, J. T., Adams, H. D., Klein, T., López, R., Sáenz-Romero, C., Hartmann, H., Breshears, D. D., and Allen, C. D.: Global field observations of tree die-off reveal hotter-drought fingerprint for Earth's forests, Nat. Commun., 13, 1761, https://doi.org/10.1038/s41467-022-29289-2, 2022. a
Hansen, A. J., Neilson, R. P., Dale, V. H., Flather, C. H., Iverson, L. R., Currie, D. J., Shafer, S., Cook, R., and Bartlein, P. J.: Global Change in Forests: Responses of Species, Communities, and Biomes: Interactions between climate change and land use are projected to cause large shifts in biodiversity, BioScience, 51, 765–779, https://doi.org/10.1641/0006-3568(2001)051[0765:GCIFRO]2.0.CO;2, 2001. a
Hantson, S., Kelley, D. I., Arneth, A., Harrison, S. P., Archibald, S., Bachelet, D., Forrest, M., Hickler, T., Lasslop, G., Li, F., Mangeon, S., Melton, J. R., Nieradzik, L., Rabin, S. S., Prentice, I. C., Sheehan, T., Sitch, S., Teckentrup, L., Voulgarakis, A., and Yue, C.: Quantitative assessment of fire and vegetation properties in simulations with fire-enabled vegetation models from the Fire Model Intercomparison Project, Geosci. Model Dev., 13, 3299–3318, https://doi.org/10.5194/gmd-13-3299-2020, 2020. a, b, c
Harper, K. L., Lamarche, C., Hartley, A., Peylin, P., Ottlé, C., Bastrikov, V., San Martín, R., Bohnenstengel, S. I., Kirches, G., Boettcher, M., Shevchuk, R., Brockmann, C., and Defourny, P.: A 29-year time series of annual 300 m resolution plant-functional-type maps for climate models, Earth Syst. Sci. Data, 15, 1465–1499, https://doi.org/10.5194/essd-15-1465-2023, 2023 (data available at http://maps.elie.ucl.ac.be/CCI/viewer/download.php, last access: 27 July 2022). a, b, c, d, e
Harris, C. R., Millman, K. J., van der Walt, S. J., Gommers, R., Virtanen, P., Cournapeau, D., Wieser, E., Taylor, J., Berg, S., Smith, N. J., Kern, R., Picus, M., Hoyer, S., van Kerkwijk, M. H., Brett, M., Haldane, A., del Río, J. F., Wiebe, M., Peterson, P., Gérard-Marchant, P., Sheppard, K., Reddy, T., Weckesser, W., Abbasi, H., Gohlke, C., and Oliphant, T. E.: Array programming with NumPy, Nature, 585, 357–362, https://doi.org/10.1038/s41586-020-2649-2, 2020. a
Hasegawa, T., Fujimori, S., Ito, A., Takahashi, K., and Masui, T.: Global land-use allocation model linked to an integrated assessment model, Sci. Total Environ., 580, 787–796, https://doi.org/10.1016/j.scitotenv.2016.12.025, 2017. a
Hermoso, V., Regos, A., Morán-Ordóñez, A., Duane, A., and Brotons, L.: Tree planting: A double-edged sword to fight climate change in an era of megafires, Glob. Change Biol., 27, 3001–3003, https://doi.org/10.1111/gcb.15625, 2021. a
Hollnaicher, S.: On economic modeling of carbon dioxide removal: values, bias, and norms for good policy-advising modeling, Global Sustainability, 5, e18, https://doi.org/10.1017/sus.2022.16, 2022. a
Hoyer, S. and Hamman, J.: xarray: N-D labeled arrays and datasets in Python, J. Open Res. Softw., 5, 10, https://doi.org/10.5334/jors.148, 2017. a
Humpenöder, F., Popp, A., Dietrich, J. P., Klein, D., Lotze-Campen, H., Bonsch, M., Bodirsky, B. L., Weindl, I., Stevanovic, M., and Müller, C.: Investigating afforestation and bioenergy CCS as climate change mitigation strategies, Environ. Res. Lett., 9, 064029, https://doi.org/10.1088/1748-9326/9/6/064029, 2014. a, b, c
Humpenöder, F., Popp, A., Stevanovic, M., Müller, C., Bodirsky, B. L., Bonsch, M., Dietrich, J. P., Lotze-Campen, H., Weindl, I., Biewald, A., and Rolinski, S.: Land-use and carbon cycle responses to moderate climate change: Implications for land-based mitigation?, Environ. Sci. Technol., 49, 6731–6739, https://doi.org/10.1021/es506201r, 2015. a, b
Humpenöder, F., Popp, A., Schleussner, C.-F., Orlov, A., Windisch, M. G., Menke, I., Pongratz, J., Havermann, F., Thiery, W., Luo, F., v. Jeetze, P., Dietrich, J. P., Lotze-Campen, H., Weindl, I., and Lejeune, Q.: Overcoming global inequality is critical for land-based mitigation in line with the Paris Agreement, Nat. Commun., 13, 7453, https://doi.org/10.1038/s41467-022-35114-7, 2022. a, b, c
Hunter, J. D.: Matplotlib: A 2D graphics environment, Comput. Sci. Eng., 9, 90–95, https://doi.org/10.1109/MCSE.2007.55, 2007. a
Hurtt, G. C., Chini, L. P., Frolking, S., Betts, R. A., Feddema, J., Fischer, G., Fisk, J. P., Hibbard, K., Houghton, R. A., Janetos, A., Jones, C. D., Kindermann, G., Kinoshita, T., Klein Goldewijk, K., Riahi, K., Shevliakova, E., Smith, S., Stehfest, E., Thomson, A., Thornton, P., van Vuuren, D. P., and Wang, Y. P.: Harmonization of land-use scenarios for the period 1500–2100: 600 years of global gridded annual land-use transitions, wood harvest, and resulting secondary lands, Climatic Change, 109, 117, https://doi.org/10.1007/s10584-011-0153-2, 2011. a
Hurtt, G., Chini, L., Sahajpal, R., Frolking, S., Bodirsky, B. L., Calvin, K., Doelman, J., Fisk, J., Fujimori, S., Goldewijk, K., K., Hasegawa, T., Havlik, P., Heinimann, A., Humpenöder, F., Jungclaus, J., Kaplan, J., Krisztin, T., Lawrence, D., Lawrence, P., Mertz, O., Pongratz, J., Popp, A., Riahi, K., Shevliakova, E., Stehfest, E., Thornton, P., van Vuuren, D., and Zhang, X.: Harmonization of Global Land Use Change and Management for the Period 2015–2300, Earth System Grid Federation [data set], https://doi.org/10.22033/ESGF/input4MIPs.10468, 2019. a
Hurtt, G. C., Chini, L., Sahajpal, R., Frolking, S., Bodirsky, B. L., Calvin, K., Doelman, J. C., Fisk, J., Fujimori, S., Klein Goldewijk, K., Hasegawa, T., Havlik, P., Heinimann, A., Humpenöder, F., Jungclaus, J., Kaplan, J. O., Kennedy, J., Krisztin, T., Lawrence, D., Lawrence, P., Ma, L., Mertz, O., Pongratz, J., Popp, A., Poulter, B., Riahi, K., Shevliakova, E., Stehfest, E., Thornton, P., Tubiello, F. N., van Vuuren, D. P., and Zhang, X.: Harmonization of global land use change and management for the period 850–2100 (LUH2) for CMIP6, Geosci. Model Dev., 13, 5425–5464, https://doi.org/10.5194/gmd-13-5425-2020, 2020. a, b
IPCC: Summary for Policymakers, in: Global Warming of 1.5 °C: An IPCC Special Report on the impacts of global warming of 1.5 °C above pre-industrial levels and related global greenhouse gas emission pathways, in the context of strengthening the global response to the threat of climate change, sustainable development, and efforts to eradicate poverty, edited by: Masson-Delmotte, V., Zhai, P., Pörtner, H.-O., Roberts, D., Skea, J., Shukla, P. R., Pirani, A., Moufouma-Okia, W., Péan, C., Pidcock, R., Connors, S., Matthews, J. B. R., Chen, Y., Zhou, X., Gomis, M. I., Lonnoy, E., Maycock, T., Tignor, M., Waterfield, T., Pirani, A., Moufouma-Okia, W., Péan, C., Pidcock, R., Connors, S., Matthews, J. B. R., Chen, Y., Zhou, X., Gomis, M. I., Lonnoy, E., Maycock, T., Tignor, M., and Waterfield, T., book section SPM, World Meteorological Organization, Geneva, Switzerland, https://www.ipcc.ch/sr15/ (last access: 19 April 2023), 2018. a, b
IPCC: Climate Change 2021: The Physical Science Basis. Contribution of Working Group I to the Sixth Assessment Report of the Intergovernmental Panel on Climate Change, vol. in Press, Cambridge University Press, Cambridge, United Kingdom and New York, NY, USA, https://doi.org/10.1017/9781009157896, 2021. a
Jäger, F.: Data used in “Fire Weather Compromises Forestation-reliant Climate Mitigation Pathways” (Jaeger et al., 2024a), Zenodo [data set], https://doi.org/10.5281/zenodo.12627389, 2024. a
Jäger, F. M., Quilcaille, Y., Schwaab, J., Seneviratne, S. I., and Padron, R. S.: Code for data preprocessing, analysis and plotting in “Fire Weather Compromises Forestation-reliant Climate Mitigation Pathways” (Jaeger et al., 2024a), Zenodo [code], https://doi.org/10.5281/zenodo.11068957, 2024. a
Johnstone, J. F., Allen, C. D., Franklin, J. F., Frelich, L. E., Harvey, B. J., Higuera, P. E., Mack, M. C., Meentemeyer, R. K., Metz, M. R., Perry, G. L., Schoennagel, T., and Turner, M. G.: Changing disturbance regimes, ecological memory, and forest resilience, Front. Ecol. Environ., 14, 369–378, https://doi.org/10.1002/fee.1311, 2016. a
Jones, M. W., Abatzoglou, J. T., Veraverbeke, S., Andela, N., Lasslop, G., Forkel, M., Smith, A. J. P., Burton, C., Betts, R. A., van der Werf, G. R., Sitch, S., Canadell, J. G., Santín, C., Kolden, C., Doerr, S. H., and Le Quéré, C.: Global and Regional Trends and Drivers of Fire Under Climate Change, Rev. Geophys., 60, e2020RG000726, https://doi.org/10.1029/2020RG000726, 2022. a, b, c
Koch, A. and Kaplan, J. O.: Tropical forest restoration under future climate change, Nat. Clim. Change, 12, 279–283, https://doi.org/10.1038/s41558-022-01289-6, 2022. a, b
Krey, V., Havlik, P., Kishimoto, P. N., Fricko, O., Zilliacus, J., Gidden, M., Strubegger, M., Kartasasmita, G., Ermolieva, T., Forsell, N., Gusti, M., Johnson, N., Kikstra, J., Kindermann, G., Kolp, P., Lovat, F., McCollum, D. L., Min, J., Pachauri, S., C., P. S., Rao, S., Rogelj, J., H., Ünlü, Valin, G., Wagner, P., Zakeri, B., Obersteiner, M., and Riahi, K.: MESSAGEix-GLOBIOM Documentation – 2020 release, International Institute for Applied Systems Analysis, https://doi.org/10.22022/iacc/03-2021.17115, 2020. a
Lasslop, G., Hantson, S., Harrison, S. P., Bachelet, D., Burton, C., Forkel, M., Forrest, M., Li, F., Melton, J. R., Yue, C., Archibald, S., Scheiter, S., Arneth, A., Hickler, T., and Sitch, S.: Global ecosystems and fire: Multi-model assessment of fire-induced tree-cover and carbon storage reduction, Glob. Change Biol., 26, 5027–5041, https://doi.org/10.1111/gcb.15160, 2020. a, b
Li, Y., Brando, P. M., Morton, D. C., Lawrence, D. M., Yang, H., and Randerson, J. T.: Deforestation-induced climate change reduces carbon storage in remaining tropical forests, Nat. Commun., 13, 1964, https://doi.org/10.1038/s41467-022-29601-0, 2022. a
Moya, D., Certini, G., and Fulé, P. Z.: Fire regime and ecosystem responses: adaptive forest management in a changing world (Part 1), Int. J. Wildland Fire, 28, 327–328, https://doi.org/10.1071/WFv28n5_FO, 2019. a
Murphy, B. P. and Bowman, D. M.: What controls the distribution of tropical forest and savanna?, Ecol. Lett., 15, 748–758, https://doi.org/10.1111/j.1461-0248.2012.01771.x, 2012. a
National Institute for Environmental Studies: AIM-SSP/RCP Gridded Emissions and Land-use data, National Institute for Environmental Studies [data set]. https://doi.org/10.18959/20180403.001, 2018. a
O'Neill, B. C., Tebaldi, C., van Vuuren, D. P., Eyring, V., Friedlingstein, P., Hurtt, G., Knutti, R., Kriegler, E., Lamarque, J.-F., Lowe, J., Meehl, G. A., Moss, R., Riahi, K., and Sanderson, B. M.: The Scenario Model Intercomparison Project (ScenarioMIP) for CMIP6, Geosci. Model Dev., 9, 3461–3482, https://doi.org/10.5194/gmd-9-3461-2016, 2016. a
Ostberg, S., Schaphoff, S., Lucht, W., and Gerten, D.: Three centuries of dual pressure from land use and climate change on the biosphere, Environ. Res. Lett., 10, 044011, https://doi.org/10.1088/1748-9326/10/4/044011, 2015. a
Pawson, S. M., Brin, A., Brockerhoff, E. G., Lamb, D., Payn, T. W., Paquette, A., and Parrotta, J. A.: Plantation forests, climate change and biodiversity, Biodivers. Conserv., 22, 1203–1227, https://doi.org/10.1007/s10531-013-0458-8, 2013. a
Pellegrini, A. F., Harden, J., Georgiou, K., Hemes, K. S., Malhotra, A., Nolan, C. J., and Jackson, R. B.: Fire effects on the persistence of soil organic matter and long-term carbon storage, Nat. Geosci., 15, 5–13, https://doi.org/10.1038/s41561-021-00867-1, 2022. a
Popp, A., Calvin, K., Fujimori, S., Havlik, P., Humpenöder, F., Stehfest, E., Bodirsky, B. L., Dietrich, J. P., Doelmann, J. C., Gusti, M., Hasegawa, T., Kyle, P., Obersteiner, M., Tabeau, A., Takahashi, K., Valin, H., Waldhoff, S., Weindl, I., Wise, M., Kriegler, E., Lotze-Campen, H., Fricko, O., Riahi, K., and Vuuren, D. P.: Land-use futures in the shared socio-economic pathways, Global Environ. Chang., 42, 331–345, https://doi.org/10.1016/j.gloenvcha.2016.10.002, 2017. a, b, c, d
Prestele, R., Alexander, P., Rounsevell, M. D., Arneth, A., Calvin, K., Doelman, J., Eitelberg, D. A., Engström, K., Fujimori, S., Hasegawa, T., Havlik, P., Humpenöder, F., Jain, A. K., Krisztin, T., Kyle, P., Meiyappan, P., Popp, A., Sands, R. D., Schaldach, R., Schüngel, J., Stehfest, E., Tabeau, A., Meijl, H. V., Vliet, J. V., and Verburg, P. H.: Hotspots of uncertainty in land-use and land-cover change projections: a global-scale model comparison, Glob. Change Biol., 22, 3967–3983, https://doi.org/10.1111/gcb.13337, 2016. a
Quilcaille, Y. and Batibeniz, F.: Fire weather index data under historical and SSP projections in CMIP6 from 1850 to 2100, ETH Zurich Research Collection [data set], https://doi.org/10.3929/ethz-b-000583391, 2022. a, b, c, d
Quilcaille, Y., Batibeniz, F., Ribeiro, A. F. S., Padrón, R. S., and Seneviratne, S. I.: Fire weather index data under historical and shared socioeconomic pathway projections in the 6th phase of the Coupled Model Intercomparison Project from 1850 to 2100, Earth Syst. Sci. Data, 15, 2153–2177, https://doi.org/10.5194/essd-15-2153-2023, 2023. a
Rogelj, J., Popp, A., Calvin, K. V., Luderer, G., Emmerling, J., Gernaat, D., Fujimori, S., Strefler, J., Hasegawa, T., Marangoni, G., Krey, V., Kriegler, E., Riahi, K., van Vuuren, D. P., Doelman, J., Drouet, L., Edmonds, J., Fricko, O., Harmsen, M., Havlík, P., Humpenöder, F., Stehfest, E., and Tavoni, M.: Scenarios towards limiting global mean temperature increase below 1.5 °C, Nat. Clim. Change, 8, 325–332, https://doi.org/10.1038/s41558-018-0091-3, 2018. a
Schaphoff, S., von Bloh, W., Rammig, A., Thonicke, K., Biemans, H., Forkel, M., Gerten, D., Heinke, J., Jägermeyr, J., Knauer, J., Langerwisch, F., Lucht, W., Müller, C., Rolinski, S., and Waha, K.: LPJmL4 – a dynamic global vegetation model with managed land – Part 1: Model description, Geosci. Model Dev., 11, 1343–1375, https://doi.org/10.5194/gmd-11-1343-2018, 2018. a, b, c, d, e
Seidl, R., Thom, D., Kautz, M., Martin-Benito, D., Peltoniemi, M., Vacchiano, G., Wild, J., Ascoli, D., Petr, M., Honkaniemi, J., Lexer, M. J., Trotsiuk, V., Mairota, P., Svoboda, M., Fabrika, M., Nagel, T. A., and Reyer, C. P.: Forest disturbances under climate change, Nat. Clim. Change, 7, 395–402, https://doi.org/10.1038/nclimate3303, 2017. a
Seneviratne, S. I., Zhang, X., Adnan, M., Badi, W., Dereczynski, C., Luca, A. D., Ghosh, S., Iskandar, I., Kossin, J., Lewis, S., Otto, F., Pinto, I., Satoh, M., Vicente-Serrano, S., Wehner, M., and Zhou, B.: Weather and Climate Extreme Events in a Changing Climate, in: Climate Change 2021: The Physical Science Basis. Contribution of Working Group I to the Sixth Assessment Report of the Intergovernmental Panel on Climate Change, edited by: Masson-Delmotte, V., Zhai, P., Pirani, A., Connors, S. L., Péan, C., Berger, S., Caud, N., Chen, Y., Goldfarb, L., Gomis, M. I., Huang, M., Leitzell, K., Lonnoy, E., Matthews, J. B. R., Maycock, T. K., Waterfield, T., Yelekçi, O., Yu, R., and Zhou, B., book section 11, p. 1513–1766, Cambridge University Press, Cambridge, United Kingdom and New York, NY, USA, https://doi.org/10.1017/9781009157896.013, 2021. a, b, c
Son, R., Kim, H., Wang, S. Y., Jeong, J. H., Woo, S. H., Jeong, J. Y., Lee, B. D., Kim, S. H., LaPlante, M., Kwon, C. G., and Yoon, J. H.: Changes in fire weather climatology under 1.5 °C and 2.0 °C warming, Environ. Res. Lett., 16, https://doi.org/10.1088/1748-9326/abe675, 2021. a
Spafford, L. and MacDougall, A. H.: Validation of terrestrial biogeochemistry in CMIP6 Earth system models: a review, Geosci. Model Dev., 14, 5863–5889, https://doi.org/10.5194/gmd-14-5863-2021, 2021. a, b, c
Stehfest, E., van Vuuren, D., Kram, T., Bouwman, L., Alkemade, R., Bakkenes, M., Biemans, H., Bouwman, A., den Elzen, M., Janse, J., Lucas, P., van Minnen, J., Müller, C., and Prins, A. G.: Integrated Assessment of Global Environmental Change with IMAGE 3.0 – Model description and policy applications, https://www.pbl.nl/sites/default/files/downloads/pbl-2014-integrated_assessment_of_global_environmental_change_with_image30_735.pdf (last access: 30 October 2023), 2014. a
Thom, D. and Seidl, R.: Natural disturbance impacts on ecosystem services and biodiversity in temperate and boreal forests, Biol. Rev., 91, 760–781, https://doi.org/10.1111/brv.12193, 2016. a
UNFCCC: Paris Agreement, UN Treaty, Treaty No. XXVII-7-d, https://unfccc.int/sites/default/files/resource/parisagreement_publication.pdf (last access: 3 Fewbruary 2023), 2015. a
van Vuuren, D. P., Hof, A. F., Sluisveld, M. A. V., and Riahi, K.: Open discussion of negative emissions is urgently needed, Nature Energy, 2, 902–904, https://doi.org/10.1038/s41560-017-0055-2, 2017a. a
van Vuuren, D. P., Stehfest, E., Gernaat, D. E., Doelman, J. C., van den Berg, M., Harmsen, M., de Boer, H. S., Bouwman, L. F., Daioglou, V., Edelenbosch, O. Y., Girod, B., Kram, T., Lassaletta, L., Lucas, P. L., van Meijl, H., Müller, C., van Ruijven, B. J., van der Sluis, S., and Tabeau, A.: Energy, land-use and greenhouse gas emissions trajectories under a green growth paradigm, Global Environ. Chang., 42, 237–250, https://doi.org/10.1016/j.gloenvcha.2016.05.008, 2017b. a, b
Vaughan, N. E., Gough, C., Mander, S., Littleton, E. W., Welfle, A., Gernaat, D. E., and Vuuren, D. P. V.: Evaluating the use of biomass energy with carbon capture and storage in low emission scenarios, Environ. Res. Lett., 13, 044014, https://doi.org/10.1088/1748-9326/aaaa02, 2018. a
Virtanen, P., Gommers, R., Oliphant, T. E., Haberland, M., Reddy, T., Cournapeau, D., Burovski, E., Peterson, P., Weckesser, W., Bright, J., van der Walt, S. J., Brett, M., Wilson, J., Millman, K. J., Mayorov, N., Nelson, A. R. J., Jones, E., Kern, R., Larson, E., Carey, C. J., Polat, İ., Feng, Y., Moore, E. W., VanderPlas, J., Laxalde, D., Perktold, J., Cimrman, R., Henriksen, I., Quintero, E. A., Harris, C. R., Archibald, A. M., Ribeiro, A. H., Pedregosa, F., van Mulbregt, P., and SciPy 1.0 Contributors: SciPy 1.0: Fundamental Algorithms for Scientific Computing in Python, Nature Meth., 17, 261–272, https://doi.org/10.1038/s41592-019-0686-2, 2020. a
Wagner, C. E. V.: Structure of the Canadian forest fire weather index, https://cfs.nrcan.gc.ca/pubwarehouse/pdfs/19927.pdf (last access: 6 September 2023), 1987. a
Walker, X. J., Baltzer, J. L., Cumming, S. G., Day, N. J., Ebert, C., Goetz, S., Johnstone, J. F., Potter, S., Rogers, B. M., Schuur, E. A., Turetsky, M. R., and Mack, M. C.: Increasing wildfires threaten historic carbon sink of boreal forest soils, Nature, 572, 520–523, https://doi.org/10.1038/s41586-019-1474-y, 2019. a
Wang, Y., Anderson, K. R., and Suddaby, R. M.: Updated source code for calculating fire danger indices in the Canadian Forest Fire Weather Index System, https://cfs.nrcan.gc.ca/publications?id=36461 (last access: 6 September 2023), 2015. a
Wise, M., Calvin, K., Thomson, A., Clarke, L., Bond-Lamberty, B., Sands, R., Smith, S. J., Janetos, A., and Edmonds, J.: Implications of Limiting CO2 Concentrations for Land Use and Energy, Science, 324, 1183–1186, https://doi.org/10.1126/science.1168475, 2009. a
Wu, C., Venevsky, S., Sitch, S., Mercado, L. M., Huntingford, C., and Staver, A. C.: Historical and future global burned area with changing climate and human demography, One Earth, 4, 517–530, https://doi.org/10.1016/j.oneear.2021.03.002, 2021. a
Zheng, B., Ciais, P., Chevallier, F., Yang, H., Canadell, J. G., Chen, Y., van der Velde, I. R., Aben, I., Chuvieco, E., Davis, S. J., Deeter, M., Hong, C., Kong, Y., Li, H., Li, H., Lin, X., He, K., and Zhang, Q.: Record-high CO2 emissions from boreal fires in 2021, Science, 379, 912–917, https://doi.org/10.1126/science.ade0805, 2023. a
- Abstract
- Introduction
- Large-scale forestation in ambitious climate mitigation scenarios
- Forest fire danger under intensifying fire weather
- Forestation drives forest exposure to extreme fire hazard
- Climate impacts on forests in IAMs to date
- Ways forward for climate-related forest disturbances in IAMs
- Appendix A: Forest-area-weighted mean fire weather index ()
- Appendix B: Potentially burned forest area
- Appendix C: Forest area in land cover and land use datasets in the past and present
- Appendix D: Limitations
- Code and data availability
- Author contributions
- Competing interests
- Disclaimer
- Acknowledgements
- Financial support
- Review statement
- References
- Supplement
- Abstract
- Introduction
- Large-scale forestation in ambitious climate mitigation scenarios
- Forest fire danger under intensifying fire weather
- Forestation drives forest exposure to extreme fire hazard
- Climate impacts on forests in IAMs to date
- Ways forward for climate-related forest disturbances in IAMs
- Appendix A: Forest-area-weighted mean fire weather index ()
- Appendix B: Potentially burned forest area
- Appendix C: Forest area in land cover and land use datasets in the past and present
- Appendix D: Limitations
- Code and data availability
- Author contributions
- Competing interests
- Disclaimer
- Acknowledgements
- Financial support
- Review statement
- References
- Supplement