the Creative Commons Attribution 4.0 License.
the Creative Commons Attribution 4.0 License.
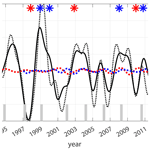
CO2 surface variability: from the stratosphere or not?
Fluctuations in atmospheric CO2 can be measured with great precision and are used to identify human-driven sources as well as natural cycles of ocean and land carbon. One source of variability is the stratosphere, where the influx of aged CO2-depleted air can produce fluctuations at the surface. This process has been speculated to be a potential source of interannual variability (IAV) in CO2 that might obscure the quantification of other sources of IAV. Given the recent success in demonstrating that the stratospheric influx of N2O- and chlorofluorocarbon-depleted air is a dominant source of their surface IAV in the Southern Hemisphere, I apply the same model and measurement analysis here to CO2. Using chemistry-transport modeling or scaling of the observed N2O variability, I find that the stratosphere-driven surface variability in CO2 is at most 10 % of the observed IAV and is not an important source. Diagnosing the amplitude of the CO2 annual cycle and its increase from 1985 to 2021 through the annual variance gives rates similar to traditional methods in the Northern Hemisphere (BRW, MLO) but can identify the emergence of small trends (0.08 ppm per decade) in the Southern Hemisphere (SMO, CGO).
The surface abundance of CO2, also called the Keeling curve (Fig. 1a), is used as the prime example of the human-driven increases in greenhouse gases. It is also used to demonstrate control of CO2 by the land biosphere and the oceans through its annual cycles and interannual variations (Le Quéré et al., 2016, 2018). The inverse modeling of surface sources based on these CO2 observations is used to infer regional sources of fossil fuel emissions as well as year-to-year changes in primary productivity of the biosphere or oceanic degassing (e.g., Gurney et al., 2002; Baker et al., 2006; Engelen et al., 2006; Nassar et al., 2011; Peylin et al., 2013; Frankenberg et al., 2016; Pandey et al., 2016; Nakazawa, 2020). There is concern that atmospheric variations in CO2, and hence the net sources derived from them, may be affected by interannual variations (IAVs) in tropospheric mixing or stratosphere–troposphere exchange (STE) (Gaubert et al., 2019), but there are no definitive studies. For example, Nazakawa's (2020) review of greenhouse gas studies mentions the stratosphere only in connection with CH4 and N2O, not with CO2.
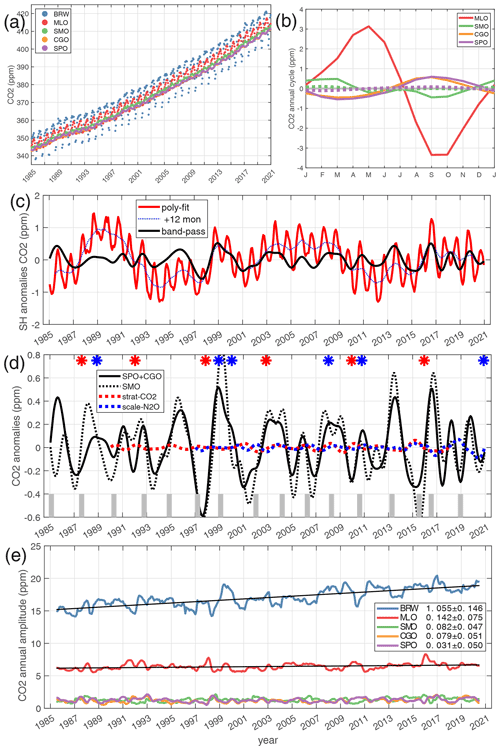
Figure 1(a) NOAA surface CO2 monthly data (ppm, mole fraction) from Dlugokencky et al. (2021b). The five sites are as follows: BRW – Barrow, AK (now Utqiaġvik), at 71∘ N, 156∘ W; MLO – Mauna Loa, HI, at 20∘ N, 156∘ W; SMO – Tutuila, Am. Samoa, at 14∘ S, 171∘ W; CGO – Cape Grim, Tasmania, Australia, at 41∘ S, 144∘ E; SPO – South Pole at 90∘ S. Monthly average in situ observations are used with gaps filled by flask data at the same site. Only one point is interpolated (SMO, May 2015). CGO is flask only. (b) The mean annual cycle in surface CO2 (ppm) at four sites (BRW not shown) using 1985 through 2021 calculated from the residuals after the polynomial fit was removed. The eCO2 model results are shown as dotted lines for SMO and SPO with the same color coding; eCO2 has similar phasing at SPO and is double-peaked at SMO, but the amplitudes (∼0.18 ppm) are much smaller than observed. The sCO2 amplitude is even smaller (∼0.06 ppm) and not shown. (c) Observed CO2 variability (ppm) derived from the monthly averages of two SH extratropical stations (SPO and CGO) for the period 1985–2021. The poly-fit (solid red curve) shows the residuals after removal of a polynomial fit (average of third- and fourth-order in time). A 12-month running mean (thin dashed blue curve) is derived from the poly-fit residuals and removes the annual cycle. The interannual variability (IAV, solid black curve) is derived from bandpass filtering described in the text. The bandpass limits [0.20 0.80] are set to truncate periods longer than 5 years and shorter than 1.25 years. (d) Surface CO2 IAV (ppm) for SH extratropics. The SPO + CGO IAV (black solid line) is compared with the SMO IAV (thin dotted black line). Other IAVs shown are (1) model-calculated sCO2 (dashed red line) from the stratosphere-driven influx of aged, low-CO2 air and (2) N2O observed IAV (dashed blue line) scaled to match flux of low-CO2 air. The timing of the QBO phase change in equatorial zonal wind at 40 hPa from negative (easterlies) to positive (westerlies) is denoted with thick vertical gray bars. The timing of moderate to extreme El Niños (red stars) and La Niñas (blue stars) is also shown. (e) CO2 annual amplitude (ppm, max–min) derived from the variance across 12-monthly values. Each monthly point (centered on the beginning of each month) is the standard deviation of the surrounding ±6-monthly means, scaled by 2×2.5 to give the max–min amplitude as if it were a sine curve. The line fits for BRW and MLO are shown. The slope and standard error (SE) in units of parts per million (ppm) per decade are given in the legend. The SE is calculated conservatively based on the number of years rather than the number of months.
The possibility of a true STE-driven IAV CO2 signal, raised by Gaubert et al. (2019), has not been seriously investigated. For the most part, when studies investigate the stratospheric influence on CO2 source inversions, they are not concerned about STE fluxes directly but other factors that degrade the results: e.g., gradients across the tropopause, the effective tropospheric air mass diluting surface emissions, or the inclusion of CO2-depleted stratospheric air in column CO2 calculations (Nassar et al., 2011; Deng et al., 2015; Frankenberg et al., 2016; Pandey et al., 2016). For example, Le Quéré et al. (2018) are concerned with how emissions will mix throughout the troposphere and the stratosphere, but not how stratospheric air will come back down to the surface. Only studies of the CO2 triple-oxygen isotope signature (Δ17O) are concerned with accurate STE fluxes, recognizing its importance in the seasonal isotopic signals (Liang et al., 2017; Koren et al., 2019; Laskar et al., 2019).
Both models and observations have shown that the stratospheric quasi-biennial oscillation (QBO) modulates the STE and drives much of the IAV observed in surface N2O through the stratospheric influx of N2O-depleted air (Hamilton and Fan, 2000; Nevison et al., 2004, 2011; Ray et al., 2020; Ruiz et al., 2021; Ruiz and Prather, 2022). Here, I use the N2O studies of Ruiz et al. (2021) with parallel model simulations of CO2 to place constraints on the CO2 IAV caused by atmospheric circulation, finding that this effect is a clear but minor perturbation in driving the observed IAV of CO2.
I investigate the CO2 IAV and its causes using surface CO2 observations from 1985 through 2020, surface N2O observations from 1997 through 2020, and tracer simulations from the UC Irvine chemistry-transport model (CTM) simulations for the historical period 1990–2017.
To study the circulation-driven IAV of CO2, including STE, I focus on the Southern Hemisphere (SH) because fluctuations in the large biosphere-driven seasonality in the Northern Hemisphere (NH) (Fig. 1a and b) will obscure any stratosphere-driven IAV there. The UCI CTM uses ECMWF integrated forecast fields at 1.1∘ horizontal resolution and has proven quite successful in simulating the historical IAV of surface N2O, ozone columns, and the Antarctic ozone hole (Ruiz et al., 2021; Ruiz and Prather, 2022; Tang et al., 2021). For CO2, I develop two model scenarios to highlight the impacts of atmospheric transport. First, I define a surface-emissions-driven eCO2 scenario, in which the total atmosphere increases at a constant rate of 2 ppm yr−1 (parts per million, dry-air mole fraction), driving a flux of about 2 Pg C yr−1 into the SH. This eCO2 scenario is a simple experiment with area-uniform (20–60∘ N) and time-constant emissions to test how atmospheric circulation driving a NH–SH gradient might affect the seasonal and interannual variability of SH surface CO2. It is obviously not realistic, lacking the large biospheric and oceanic seasonality. A second stratospheric-driven sCO2 scenario is forced with a net stratospheric flux of CO2-depleted air being transported into the troposphere and down to the surface. This STE flux is calculated as the equivalent of the aging of stratospheric CO2 relative to the troposphere (2 ppm yr−1), yielding an apparent negative CO2 flux of about 0.4 Pg C yr−1 into each hemisphere. This forcing flux is placed in the uppermost model layer (∼80 km altitude) and transported to the surface. In both of these cases, CO2 changes linearly with a known trend, and I subtract that trend to get the modeled anomalies. The eCO2 scenario effectively forces the stratosphere with a negative flux of 2 ppm yr−1, but most of the SH signal comes from the much larger interhemispheric flux. A third independent method for deriving CO2 IAV uses the observed SH surface N2O signal, driven by stratospheric photochemical loss of 13 Tg N (as N2O) yr−1, as a measure of STE influence. In this case I scale the results to CO2 using the ratio of the STE fluxes, i.e., 0.15 ppm CO2 per ppb N2O. Ruiz et al. (2021, Figs. 3 and S3) show that the tropospheric QBO patterns for N2O and CFCl3 are nearly identical despite the different vertical locations and QBO patterns in their stratospheric loss. A species STE flux pattern (i) scales with the total flux out of the stratosphere and (ii) is determined by the dynamics of the lowermost midlatitude stratosphere (Ruiz and Prather, 2022, Fig. 1).
The monthly CO2 surface observations are gathered from NOAA ESRL (Dlugokencky et al., 2021b). I use five sites: BRW – Barrow, AK (now Utqiaġvik), at 71∘ N, 156∘ W; MLO – Mauna Loa, HI, at 20∘ N, 156∘ W; SMO – Tutuila, Am. Samoa, at 14∘ S, 171∘ W; CGO – Cape Grim, Tasmania, Australia, at 41∘ S, 144∘ E; and SPO – South Pole at 90∘ S. Monthly average in situ observations are used, and gaps are filled by flask data at the same site. CGO is flask only. I have a continuous monthly record from 1985 through 2020 (Fig. 1a). I convert these to a stationary series of residuals by fitting polynomials, assuming the months are equally spaced. The second-, third-, fourth-, and fifth-order polynomials produce almost identical results for each site (not shown), and the average third- and fourth-order fits are subtracted to calculate the residuals. The CO2 residuals for the average of SPO+CGO are shown in Fig. 1c as the red line, which shows a clear annual cycle plus equally large variability on decadal scales. The annual cycle of CO2 and its rate of change is a critical metric used to evaluate the carbon cycle in Earth system models (Graven et al., 2013; Zhao and Zeng, 2014; Wenzel et al., 2016). Here, I calculate the cycle simply by averaging each calendar month of the year, with results shown in Fig. 1b. The annual amplitudes (max–min) are 16.4, 6.4, 0.92, 1.02, and 1.14 ppm for BRW, MLO, SMO, CGO, and SPO, respectively. These are consistent with those previous studies, although SH cycles remain understudied and not well evaluated. In some months SMO at 14∘ S can be north of the South Pacific Convergence Zone and thus influenced by NH air, explaining its non-sinusoidal cycle when compared with SPO and CGO. Also shown is the annual cycle for the modeled eCO2 scenario (∼0.18 ppm, dotted lines for SMO and SPO). That for the sCO2 scenario is even smaller (∼0.06 ppm) and is not shown. It is interesting that the SH annual cycles in eCO2 are similar in shape to those observed, even catching the double peak at SMO, but the magnitude is much smaller. There is no evidence in our direct modeling or analysis that stratosphere–troposphere exchange, which does drive an annual cycle in N2O, can produce a detectable annual cycle in CO2 above the large observed cycle.
The observed QBO signal in surface N2O (Ruiz et al., 2021, Fig. 3) has the largest amplitude in the SH extratropics, becoming weaker in the tropics and NH. Because this signal is nearly uniform across the SH extratropics in both observations and models, I combine the SPO and CGO CO2 data and focus our efforts on that time series. The challenge is to extract the CO2 IAV signal in the 2–5-year period range. A simple 12-month running mean is great for removing the annual cycle but leaves the large-amplitude decadal periods (blue dashed line in Fig. 1c). I select bandpass filtering, while recognizing that this method can produce spurious results, especially at the edges. After several false starts and with help from the reviewers, I chose the MATLAB bandpass filter. This function is well documented (https://www.mathworks.com/help/signal/ref/bandpass.html, last access: 8 March 2022), and the bandpass is defined by the lower and upper cut-off frequencies. After experimentation with the CO2 signal to reduce edge effects, I chose the following settings: bandpass frequency (yr−1) range [0.20 0.80]; bandpass applied forward and backward is averaged; ImpulseResponse=iir; Steepness=0.85. A wider filter, e.g., [0.16 0.95], produced similar results for the middle years but large swings for the beginning and end years. IAV signals derived from frequency filtering for the last 2–3 years of the record are not robust. The resulting bandpass IAV (thick black line in Fig. 1c) clearly shows the patterns seen in the 12-month running mean. The SMO IAV is calculated in the same way and plotted alongside the SPO+CGO IAV in Fig. 1d. The IAVs for N2O observations as well as the modeled eCO2 and sCO2 scenarios use the same processing.
For monthly N2O surface observations, also from NOAA ESRL (Dlugokencky et al., 2021a), I focus on SH extratropics using SPO and CGO, plus three other sites: S30 – western Pacific cruise at 30∘ S, 168∘ E; USH – Tierra del Fuego, Ushuaia, Argentina, at 55∘ S, 68∘ W; and PSA – Palmer Station, Antarctica, at 65∘ S, 64∘ W. All five sites have nearly identical N2O records, and I average them to get our SH IAV signal with the same processing as for CO2. The QBO circulation is known to reach throughout the stratosphere and into the troposphere (Tung and Yang, 1994; Hamilton and Fan, 2000), and multi-model studies have attributed the surface N2O IAV to the STE flux (Ruiz et al., 2021). I can thus scale the surface N2O IAV with the ratio of STE fluxes (CO2:N2O) to give an observational estimate of the STE-driven CO2 IAV in the SH extratropics (dashed blue line in Fig. 1d). The IAV in SH (40–90∘ S) surface CO2 calculated from the sCO2 model scenario is also shown (dashed red line in Fig. 1d). The modeled sCO2 and observed N2O-scaled IAVs are not always in phase, but they are in strong agreement in terms of amplitude: the STE IAV in CO2 is a small fraction of the observed IAV. In addition, the modeled eCO2 IAV shows that tropospheric circulation changes produce small IAV.
I compare CO2 with well-known interannual cycles in the Earth system in Fig. 1d by plotting (i) the QBO phase change (from easterly to westerly zonal equatorial wind at 40 hPa, see Newman, 2021) as thick gray vertical bars and (ii) the times of moderate to extreme El Niños (red stars) and La Niñas (blue stars) (Trenberth, 2021). From this analysis, I expect minimal contribution of the QBO–driven circulation to the CO2 IAV and find no obvious connection between the two in this figure. For the El Niño–Southern Oscillation (ENSO), this simple comparison is inadequate. At best it shows that some of the larger positive SH IAVs align with El Niños, whereas I know that ENSO affects ocean upwelling and continental rainfall, and the CO2 anomalies correlate very well with tropical ocean temperatures (Wang et al., 2021; Keeling and Graven, 2021).
I have shown that the STE fluxes of old stratospheric air with “depleted” CO2 have little influence on the IAV or annual cycles of CO2 at the surface. The IAV observed for SH stations has a standard deviation of 0.22 to 0.28 ppm, while that for sCO2 is at most 0.02 ppm in both hemispheres, that for eCO2 is less than 0.02 ppm for SPO to SMO, and that for scaled N2O is 0.03 ppm for SPO+CGO average. The standard deviation for NH CO2 IAV is larger at 0.4 to 0.6 ppm and thus even less influenced by stratospheric air. Thus, the speculations of Gaubert et al. (2019) regarding atmospheric transport can be dismissed.
The latitudinal pattern of N2O IAV provides evidence for causes: e.g., the STE-driven signal weakens in the tropics and changes phase in the NH, and QBO composites show a clear separation of hemispheric sources (Ruiz et al., 2021). The latitudinal pattern of CO2 IAV may similarly provide information on its cause. Comparing tropics to extratropics in the SH (SPO+CGO vs. SMO, solid and dotted black lines in Fig. 1d), I find remarkably similar patterns after 1990, with similar amplitudes and some phase shifts of at most 1 year. If I add the NH tropics MLO IAV (not shown), the pattern and amplitude are similar. When the sites are in sync, one can only presume that the CO2 perturbation is tied to changes in the growth and decay of tropical biomass transported equally to both hemispheres (Keeling and Graven, 2021). The challenge lies in the phasing and which region leads or lags in change. Unfortunately, the bandpass IAVs in this analysis do not seem able to accurately determine the phase at a level up to 1 year.
The Samoan site SMO provides a valuable but very challenging record of CO2 and other trace gases having dominant NH emissions, such as chlorofluorocarbons (Cunnold et al., 1994) and N2O (Nevison et al., 2007). Sometimes SMO is synchronous with the SH extratropics (CGO and SPO, which are almost always synchronous with each other) and at other times it links with MLO and the NH. Thus, to use SMO CO2 as a metric for carbon cycle models, one must recognize that SMO is not simply representative of the SH tropics. When evaluating carbon cycle models, one should test tracer transport using the IAV for SMO versus SPO+CGO. As shown in Fig. 1d, there are clear times when SMO is distinct from CGO+SPO (e.g., 1994, 1999, 2008 2012, 2015). At these times the SMO IAV matches that of MLO (not shown). These interannual shifts provide an excellent test for CO2 historical simulations using weather forecasting systems (e.g., McNorton et al., 2020) and realistic sources and sinks (e.g., Piao et al., 2018; Wang et al., 2020).
The rate of increase of the amplitude of the annual cycle of CO2 is a key measure of changes in the biospheric and oceanic carbon cycles. The amplitude can be measured from the variance across 12 months. If the cycle is sinusoidal, then the max–min amplitude is equal to twice the square root of 2 times the standard deviation as plotted in Fig. 1e. With this approximation, I calculate a mean amplitude of 17.0, 6.4, 1.3, 1.2, and 1.3 ppm for BRW, MLO, SMO, CGO, and SPO, respectively. These results are almost identical to those from the composited annual cycles (Fig. 1b) but disagree at SMO as might be expected because of its double-peaked cycle. A linear fit to the standard deviations gives trends for the period 1985–2020 of 1.06±0.15, 0.142±0.075, 0.082±0.047, 0.079±0.051, and 0.031±0.050 ppm per decade for BRW, MLO, SMO, CGO, and SPO, respectively. The standard errors quoted here come from a standard linear fit of the monthly values shown in Fig. 1e but are calculated more conservatively using 35 years as the degrees of freedom instead of 420 months. Our results for BRW and MLO agree well with other more extensive data analyses (Graven et al., 2013; Zhao and Zeng, 2014; Wenzel et al., 2016; Piao et al., 2018; Wang et al., 2020) but are able to identify emergent trends in the SH, which is not often used for model evaluation. A more serious uncertainty analysis focusing on the SH sources and sinks, the annual and IAV cycles, and their trends would help solidify our knowledge of the carbon cycle.
All data and code used in this analysis are placed in the archive at datadryad.org: https://doi.org/10.7280/D1N10J (Prather, 2022). All figures and their tabulated data are included.
The author has declared that there are no competing interests.
Publisher's note: Copernicus Publications remains neutral with regard to jurisdictional claims in published maps and institutional affiliations.
The author is grateful for the efforts of the reviewers and their insight, particularly in catching the problems and vagaries with bandpass filtering; the paper is much improved. I thank Ed Dlugokencky and his colleagues at the NOAA GML Carbon Cycle Cooperative Global Air Sampling Network for maintaining high-quality, readily accessible data. I also acknowledge the support and assistance of my research group at UC Irvine, particularly Xin Zhu for the CTM simulations and Daniel Ruiz for the N2O simulations.
The research at UCI has been supported by grants from the National Aeronautics and Space Administration's Atmospheric Chemistry Modeling and Analysis Program (ACMAP; grant no. 80NSSC21K1454) and the National Science Foundation's Atmospheric Chemistry Program (grant no. AGS-2135749).
This paper was edited by Ning Zeng and reviewed by two anonymous referees.
Baker, D. F., Law, R. M., Gurney, K. R., Rayner, P., Peylin, P., Denning, A. S., Bousquet, P., Bruhwiler, L., Chen, Y. H., Ciais, P., Fung, I. Y., Heimann, M., John, J., Maki, T., Maksyutov, S., Masarie, K., Prather, M., Pak, B., Taguchi, S., and Zhu, Z.: TransCom 3 inversion intercomparison: Impact of transport model errors on the interannual variability of regional CO2 fluxes, 1988–2003, Global Biogeochem. Cy., 20, Gb1002, https://doi.org/10.1029/2004gb002439, 2006.
Cunnold, D. M., Fraser, P. J., Weiss, R. F., Prinn, R. G., Simmonds, P. G., Miller, B. R., Alyea, F. N., and Crawford, A. J.: Global trends and annual releases of CCl3F and CCl2F2 estimated from ALE/GAGE and other measurements from July 1978 to June 1991, J. Geophys. Res., 99, 1107–1126, https://doi.org/10.1029/93JD02715, 1994.
Deng, F., Jones, D. B. A., Walker, T. W., Keller, M., Bowman, K. W., Henze, D. K., Nassar, R., Kort, E. A., Wofsy, S. C., Walker, K. A., Bourassa, A. E., and Degenstein, D. A.: Sensitivity analysis of the potential impact of discrepancies in stratosphere–troposphere exchange on inferred sources and sinks of CO2, Atmos. Chem. Phys., 15, 11773–11788, https://doi.org/10.5194/acp-15-11773-2015, 2015.
Dlugokencky, E. J., Crotwell, A. M., Mund, J. W., Crotwell, M. J., and Thoning, K. W.: Atmospheric Nitrous Oxide Dry Air Mole Fractions from the NOAA GML Carbon Cycle Cooperative Global Air Sampling Network, 1997–2020, Version: 2021-07-30, NOAA Global Monitoring Laboratory Data Repository [data set], https://doi.org/10.15138/53g1-x417, 2021a.
Dlugokencky, E. J., Mund, J. W., Crotwell, A. M., Crotwell, M. J., and Thoning, K. W.: Atmospheric Carbon Dioxide Dry Air Mole Fractions from the NOAA GML Carbon Cycle Cooperative Global Air Sampling Network, 1968–2020, Version: 2021-07-30, NOAA Global Monitoring Laboratory Data Repository [data set], https://doi.org/10.15138/wkgj-f215, 2021b.
Engelen, R. J., Denning, A. S., Gurney, K. R., Law, R. M., Denning, A. S., Rayner, P. J., Baker, D., Bousquet, P., Bruhwiler, L., Chen, Y. H., Ciais, P., Fan, S., Fung, I. Y., Gloor, M., Heimann, M., Higuchi, K., John, J., Maki, T., Maksyutov, S., Masarie, K., Peylin, P., Prather, M., Pak, B. C., Sarmiento, J., Taguchi, S., Takahashi, T., and Yuen, C. W.: On error estimation in atmospheric CO2 inversions, J. Geophys. Res.-Atmos., 111, D14199, https://doi.org/10.1029/2006jd007428, 2006.
Frankenberg, C., Kulawik, S. S., Wofsy, S. C., Chevallier, F., Daube, B., Kort, E. A., O'Dell, C., Olsen, E. T., and Osterman, G.: Using airborne HIAPER Pole-to-Pole Observations (HIPPO) to evaluate model and remote sensing estimates of atmospheric carbon dioxide, Atmos. Chem. Phys., 16, 7867–7878, https://doi.org/10.5194/acp-16-7867-2016, 2016.
Gaubert, B., Stephens, B. B., Basu, S., Chevallier, F., Deng, F., Kort, E. A., Patra, P. K., Peters, W., Rödenbeck, C., Saeki, T., Schimel, D., Van der Laan-Luijkx, I., Wofsy, S., and Yin, Y.: Global atmospheric CO2 inverse models converging on neutral tropical land exchange, but disagreeing on fossil fuel and atmospheric growth rate, Biogeosciences, 16, 117–134, https://doi.org/10.5194/bg-16-117-2019, 2019.
Graven, H. D., Keeling, R. F., Piper, S. C., Patra, P. K., Stephens, B. B., Wofsy, S. C., Welp, L. R., Sweeney, C., Tans, P. P., Kelley, J. J., Daube, B. C., Kort, E. A., Santoni, G. W., and Bent, J. D.: Enhanced Seasonal Exchange of CO2 by Northern Ecosystems Since 1960, Science, 341, 1085–1089, https://doi.org/10.1126/science.1239207, 2013.
Gurney, K. R., Law, R. M., Denning, A. S., Rayner, P. J., Baker, D., Bousquet, P., Bruhwiler, L., Chen, Y. H., Ciais, P., Fan, S., Fung, I. Y., Gloor, M., Heimann, M., Higuchi, K., John, J., Maki, T., Maksyutov, S., Masarie, K., Peylin, P., Prather, M., Pak, B. C., Randerson, J., Sarmiento, J., Taguchi, S., Takahashi, T., and Yuen, C. W.: Towards robust regional estimates of CO2 sources and sinks using atmospheric transport models, Nature, 415, 626–630, https://doi.org/10.1038/415626a, 2002.
Hamilton, K. and Fan, S. M.: Effects of the stratospheric quasi-biennial oscillation on long-lived greenhouse gases in the troposphere, J. Geophys. Res.-Atmos., 105, 20581–20587, https://doi.org/10.1029/2000jd900331, 2000.
Keeling, R. F. and Graven, H. D.: Insights from Time Series of Atmospheric Carbon Dioxide and Related Tracers, Annu. Rev. Env. Resour., 46, 85–110, https://doi.org/10.1146/annurev-environ-012220-125406, 2021.
Koren, G., Schneider, L., van der Velde, I. R., van Schaik, E., Gromov, S. S., Adnew, G. A., Martino, D. J. M., Hofmann, M. E. G., Liang, M. C., Mahata, S., Bergamaschi, P., van der Laan-Luijkx, I. T., Krol, M. C., Rockmann, T., and Peters, W.: Global 3-D Simulations of the triple oxygen isotope signature delta O-17 in atmospheric CO2, J. Geophys. Res.-Atmos., 124, 8808–8836, https://doi.org/10.1029/2019JD030387, 2019.
Laskar, A. H., Mahata, S., Bhattacharya, S. K., and Liang, M. C.: Triple oxygen and clumped isotope compositions of CO2 in the middle troposphere, Earth Space Sci., 6, 1205–1219, https://doi.org/10.1029/2019EA000573, 2019.
Le Quéré, C., Andrew, R. M., Canadell, J. G., Sitch, S., Korsbakken, J. I., Peters, G. P., Manning, A. C., Boden, T. A., Tans, P. P., Houghton, R. A., Keeling, R. F., Alin, S., Andrews, O. D., Anthoni, P., Barbero, L., Bopp, L., Chevallier, F., Chini, L. P., Ciais, P., Currie, K., Delire, C., Doney, S. C., Friedlingstein, P., Gkritzalis, T., Harris, I., Hauck, J., Haverd, V., Hoppema, M., Klein Goldewijk, K., Jain, A. K., Kato, E., Körtzinger, A., Landschützer, P., Lefèvre, N., Lenton, A., Lienert, S., Lombardozzi, D., Melton, J. R., Metzl, N., Millero, F., Monteiro, P. M. S., Munro, D. R., Nabel, J. E. M. S., Nakaoka, S., O'Brien, K., Olsen, A., Omar, A. M., Ono, T., Pierrot, D., Poulter, B., Rödenbeck, C., Salisbury, J., Schuster, U., Schwinger, J., Séférian, R., Skjelvan, I., Stocker, B. D., Sutton, A. J., Takahashi, T., Tian, H., Tilbrook, B., van der Laan-Luijkx, I. T., van der Werf, G. R., Viovy, N., Walker, A. P., Wiltshire, A. J., and Zaehle, S.: Global Carbon Budget 2016, Earth Syst. Sci. Data, 8, 605–649, https://doi.org/10.5194/essd-8-605-2016, 2016.
Le Quéré, C., Andrew, R. M., Friedlingstein, P., Sitch, S., Pongratz, J., Manning, A. C., Korsbakken, J. I., Peters, G. P., Canadell, J. G., Jackson, R. B., Boden, T. A., Tans, P. P., Andrews, O. D., Arora, V. K., Bakker, D. C. E., Barbero, L., Becker, M., Betts, R. A., Bopp, L., Chevallier, F., Chini, L. P., Ciais, P., Cosca, C. E., Cross, J., Currie, K., Gasser, T., Harris, I., Hauck, J., Haverd, V., Houghton, R. A., Hunt, C. W., Hurtt, G., Ilyina, T., Jain, A. K., Kato, E., Kautz, M., Keeling, R. F., Klein Goldewijk, K., Körtzinger, A., Landschützer, P., Lefèvre, N., Lenton, A., Lienert, S., Lima, I., Lombardozzi, D., Metzl, N., Millero, F., Monteiro, P. M. S., Munro, D. R., Nabel, J. E. M. S., Nakaoka, S., Nojiri, Y., Padin, X. A., Peregon, A., Pfeil, B., Pierrot, D., Poulter, B., Rehder, G., Reimer, J., Rödenbeck, C., Schwinger, J., Séférian, R., Skjelvan, I., Stocker, B. D., Tian, H., Tilbrook, B., Tubiello, F. N., van der Laan-Luijkx, I. T., van der Werf, G. R., van Heuven, S., Viovy, N., Vuichard, N., Walker, A. P., Watson, A. J., Wiltshire, A. J., Zaehle, S., and Zhu, D.: Global Carbon Budget 2017, Earth Syst. Sci. Data, 10, 405–448, https://doi.org/10.5194/essd-10-405-2018, 2018.
Liang, M. C., Mahata, S., Laskar, A. H., Thiemens, M. H., and Newman, S.: Oxygen isotope anomaly in tropospheric CO2 and implications for CO2 residence time in the atmosphere and gross primary productivity, Sci. Rep.-UK, 7, 13180, https://doi.org/10.1038/S41598-017-12774-W, 2017.
McNorton, J. R., Bousserez, N., Agustí-Panareda, A., Balsamo, G., Choulga, M., Dawson, A., Engelen, R., Kipling, Z., and Lang, S.: Representing model uncertainty for global atmospheric CO2 flux inversions using ECMWF-IFS-46R1, Geosci. Model Dev., 13, 2297–2313, https://doi.org/10.5194/gmd-13-2297-2020, 2020.
Nakazawa, T.: Current understanding of the global cycling of carbon dioxide, methane, and nitrous oxide, P. Jpn. Acad. B-Phys., 96, 394–419, https://doi.org/10.2183/pjab.96.030, 2020.
Nassar, R., Jones, D. B. A., Kulawik, S. S., Worden, J. R., Bowman, K. W., Andres, R. J., Suntharalingam, P., Chen, J. M., Brenninkmeijer, C. A. M., Schuck, T. J., Conway, T. J., and Worthy, D. E.: Inverse modeling of CO2 sources and sinks using satellite observations of CO2 from TES and surface flask measurements, Atmos. Chem. Phys., 11, 6029–6047, https://doi.org/10.5194/acp-11-6029-2011, 2011.
Nevison, C. D., Kinnison, D. E., and Weiss, R. F.: Stratospheric influences on the tropospheric seasonal cycles of nitrous oxide and chlorofluorocarbons, Geophys. Res. Lett., 31, L20103, https://doi.org/10.1029/2004gl020398, 2004.
Nevison, C. D., Mahowald, N. M., Weiss, R. F., and Prinn, R. G.: Interannual and seasonal variability in atmospheric N2O, Global Biogeochem. Cy., 21, GB3017, https://doi.org/10.1029/2006GB002755, 2007.
Nevison, C. D., Dlugokencky, E., Dutton, G., Elkins, J. W., Fraser, P., Hall, B., Krummel, P. B., Langenfelds, R. L., O'Doherty, S., Prinn, R. G., Steele, L. P., and Weiss, R. F.: Exploring causes of interannual variability in the seasonal cycles of tropospheric nitrous oxide, Atmos. Chem. Phys., 11, 3713–3730, https://doi.org/10.5194/acp-11-3713-2011, 2011.
Newman, P.: The quasi-biennial oscillation (QBO), NASA, Goddard Space Flight Center, https://acd-ext.gsfc.nasa.gov/Data_services/met/qbo/qbo.html, last access: 30 August 2021.
Pandey, S., Houweling, S., Krol, M., Aben, I., Chevallier, F., Dlugokencky, E. J., Gatti, L. V., Gloor, E., Miller, J. B., Detmers, R., Machida, T., and Röckmann, T.: Inverse modeling of GOSAT-retrieved ratios of total column CH4 and CO2 for 2009 and 2010, Atmos. Chem. Phys., 16, 5043–5062, https://doi.org/10.5194/acp-16-5043-2016, 2016.
Peylin, P., Law, R. M., Gurney, K. R., Chevallier, F., Jacobson, A. R., Maki, T., Niwa, Y., Patra, P. K., Peters, W., Rayner, P. J., Rödenbeck, C., van der Laan-Luijkx, I. T., and Zhang, X.: Global atmospheric carbon budget: results from an ensemble of atmospheric CO2 inversions, Biogeosciences, 10, 6699–6720, https://doi.org/10.5194/bg-10-6699-2013, 2013.
Piao, S. L., Liu, Z., Wang, Y. L., Ciais, P., Yao, Y. T., Peng, S., Chevallier, F., Friedlingstein, P., Janssens, I. A., Penuelas, J., Sitch, S., and Wang, T.: On the causes of trends in the seasonal amplitude of atmospheric CO2, Glob. Change Biol., 24, 608–616, https://doi.org/10.1111/gcb.13909, 2018.
Prather, M.: Surface CO2 variability from the stratosphere, or not, Dryad [data set], https://doi.org/10.7280/D1N10J, 2022.
Ray, E. A., Portmann, R. W., Yu, P. F., Daniel, J., Montzka, S. A., Dutton, G. S., Hall, B. D., Moore, F. L., and Rosenlof, K. H.: The influence of the stratospheric Quasi-Biennial Oscillation on trace gas levels at the Earth's surface, Nat. Geosci., 13, 22, https://doi.org/10.1038/s41561-019-0507-3, 2020.
Ruiz, D. J. and Prather, M. J.: From the middle stratosphere to the surface, using nitrous oxide to constrain the stratosphere–troposphere exchange of ozone, Atmos. Chem. Phys., 22, 2079–2093, https://doi.org/10.5194/acp-22-2079-2022, 2022.
Ruiz, D. J., Prather, M. J., Strahan, S. E., Thompson, R. L., Froidevaux, L., and Steenrod, S. D.: How atmospheric chemistry and transport drive surface variability of N2O and CFC-11, J. Geophys. Res.-Atmos., 126, e2020JD033979, https://doi.org/10.1029/2020JD033979, 2021.
Tang, Q., Prather, M. J., Hsu, J., Ruiz, D. J., Cameron-Smith, P. J., Xie, S., and Golaz, J.-C.: Evaluation of the interactive stratospheric ozone (O3v2) module in the E3SM version 1 Earth system model, Geosci. Model Dev., 14, 1219–1236, https://doi.org/10.5194/gmd-14-1219-2021, 2021.
Trenberth, K. and National Center for Atmospheric Research Staff (Eds.): The Climate Data Guide: Nino SST Indices (Nino 1+2, 3, 3.4, 4; ONI and TNI), last modified: 21 January 2020, https://climatedataguide.ucar.edu/climate-data/nino-sst-indices-nino-12-3-34-4-oni-and-tni (last access: 1 June 2021), 2020.
Tung, K. K. and Yang, H.: Global QBO in circulation and ozone 2. A simple mechanistic model, J. Atmos. Sci., 51, 2708–2721, https://doi.org/10.1175/1520-0469(1994)051<2708:Gqicao>2.0.Co;2, 1994.
Wang, K., Wang, Y. L., Wang, X. H., He, Y., Li, X. Y., Keeling, R. F., Ciais, P., Heimann, M., Peng, S. S., Chevallier, F., Friedlingstein, P., Sitch, S., Buermann, W., Arora, V. K., Haverd, V., Jain, A. K., Kato, E., Lienert, S., Lombardozzi, D., Nabel, J. E. M. S., Poulter, B., Vuichard, N., Wiltshire, A., Zeng, N., Zhu, D., and Piao, S. L.: Causes of slowing-down seasonal CO2 amplitude at Mauna Loa, Glob. Change Biol., 26, 4462–4477, https://doi.org/10.1111/gcb.15162, 2020.
Wang, K., Wang, X. H., Piao, S. L., Chevallier, F., Mao, J. F., Shi, X. Y., Huntingford, C., Bastos, A., Ciais, P., Xu, H., Keeling, R. F., Pacala, S. W., and Chen, A. P.: Unusual characteristics of the carbon cycle during the 2015–2016 El Nino, Glob. Change Biol., 27, 3798–3809, https://doi.org/10.1111/gcb.15669, 2021.
Wenzel, S., Cox, P. M., Eyring, V., and Friedlingstein, P.: Projected land photosynthesis constrained by changes in the seasonal cycle of atmospheric CO2, Nature, 538, 499–501, https://doi.org/10.1038/nature19772, 2016.
Zhao, F. and Zeng, N.: Continued increase in atmospheric CO2 seasonal amplitude in the 21st century projected by the CMIP5 Earth system models, Earth Syst. Dynam., 5, 423–439, https://doi.org/10.5194/esd-5-423-2014, 2014.