the Creative Commons Attribution 4.0 License.
the Creative Commons Attribution 4.0 License.
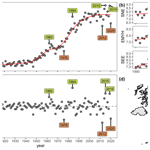
Resilience of UK crop yields to compound climate change
Louise J. Slater
Chris Huntingford
Richard F. Pywell
John W. Redhead
Elizabeth J. Kendon
Recent extreme weather events have had severe impacts on UK crop yields, and so there is concern that a greater frequency of extremes could affect crop production in a changing climate. Here we investigate the impacts of future climate change on wheat, the most widely grown cereal crop globally, in a temperate country with currently favourable wheat-growing conditions. Historically, following the plateau of UK wheat yields since the 1990s, we find there has been a recent significant increase in wheat yield volatility, which is only partially explained by seasonal metrics of temperature and precipitation across key wheat growth stages (foundation, construction and production). We find climate impacts on wheat yields are strongest in years with compound weather extremes across multiple growth stages (e.g. frost and heavy rainfall). To assess how these conditions might evolve in the future, we analyse the latest 2.2 km UK Climate Projections (UKCP Local): on average, the foundation growth stage (broadly 1 October to 9 April) is likely to become warmer and wetter, while the construction (10 April to 10 June) and production (11 June to 26 July) stages are likely to become warmer and slightly drier. Statistical wheat yield projections, obtained by driving the regression model with UKCP Local simulations of precipitation and temperature for the UK's three main wheat-growing regions, indicate continued growth of crop yields in the coming decades. Significantly warmer projected winter night temperatures offset the negative impacts of increasing rainfall during the foundation stage, while warmer day temperatures and drier conditions are generally beneficial to yields in the production stage. This work suggests that on average, at the regional scale, climate change is likely to have more positive impacts on UK wheat yields than previously considered. Against this background of positive change, however, our work illustrates that wheat farming in the UK is likely to move outside of the climatic envelope that it has previously experienced, increasing the risk of unseen weather conditions such as intense local thunderstorms or prolonged droughts, which are beyond the scope of this paper.
- Article
(5416 KB) - Full-text XML
- BibTeX
- EndNote
Globally, wheat is the most widely grown cereal crop by area, with more than 214 ×106 ha harvested and an annual production of about 730 ×106 t (FAO, 2018). In the UK, wheat is the most prevalent arable crop, with an annual planting of approximately 1.7 ×106 ha (DEFRA, 2018a). The UK climate has historically been well suited to growing wheat (Reynolds, 2010), partly due to technology and investment in the agricultural sector (as can be seen from the increasing trend in Fig. 1a as technological and agronomic innovations were introduced) but also due to the UK climate, which is suitable to temperate species when autumn-sown (Harkness et al., 2020; Reynolds, 2010). UK yields are of approximately 8 t ha−1 (Fig. 1a and b) compared to a global average of 3.5 t ha−1 (FAO, 2018). However, recent climate extremes such as the UK hot summer of 2018 and wet autumn of 2019 had substantial negative impacts on farm businesses, with significant reductions in crop yields. This climate-mediated reduction in yields is supported by evidence from the UK government (DEFRA, 2018b, 2019), the farming industry (AHDB, 2020), and real-time precision yield monitoring (Hunt et al., 2019).
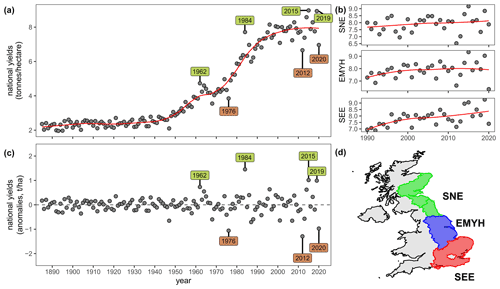
Figure 1UK national and regional wheat yields. (a) National wheat yields are shown as grey circles and locally weighted scatterplot smoothing (loess) curve as a red line. Green labels indicate examples of years with anomalously high yields; brown labels indicate examples of years with anomalously low yields. Panel (b) same as (a) for three main wheat-growing regions (data only available for 1990–2020 at the regional scale). (c) Anomalies of wheat yields computed by subtracting the Loess moving mean from the annual values. (d) Map of the three wheat-growing regions. Green indicates north-eastern Scotland, eastern Scotland, and the north-east English region (SNE); blue indicates the East Midlands, Yorkshire and the Humber region (EMYH); red indicates the south-east and eastern region (SEE).
Observed, direct impacts of climate change on crop yields are emerging globally (Brisson et al., 2010; Grassini et al., 2013; Hochman et al., 2017; Rigden et al., 2020), slowing the growth in global agricultural productivity (Ortiz-Bobea et al., 2021), and altering patterns of global food production (Ray et al., 2019). Rising temperatures under anthropogenic climate change are often detrimental to agricultural productivity (Ortiz-Bobea et al., 2021) and compound heat-drought impacts may directly affect crop growth: for instance, maize and soil yields are historically worse in places with strong associations between low rainfall and high temperature (Lesk et al., 2021). Cool and wet growth phases have also been linked to poor yields because it is hard to warm the surface when soils are wet and hard to dry wet soils during cooler periods. Thus it remains to be seen how warming and precipitation interact and whether future warming may help offset the increased precipitation by drying out waterlogged soils. This interaction depends on how the link between precipitation and soil moisture may evolve in the future (a topic drawing increasing attention in both climate and crop science, enabled by the rise in satellite-derived soil moisture observations). Combined with the nutrition demands of a rapidly growing global population, there is an urgent requirement to estimate these effects on future crop yields. Breeding and evaluating new wheat varieties tolerant of hotter, drier summers may take decades (Zheng et al., 2012) and it is unclear whether advances in agronomy are occurring fast enough to mitigate the impacts of any accelerating frequency of extreme climatic events (Chen et al., 2021). Changing climatic conditions may also affect yields indirectly by constraining the ability of farmers to undertake key management actions of tillage, sowing, and harvest or by causing damage to natural capital, such as soil erosion. These new constraints on yields may overtake any gains from physiological and phenological advances obtained through plant breeding.
In order to assess this risk to future food production, there is a critical need to understand how climate extremes are likely to evolve during the seasonal growth phases that are most relevant to the farming industry. Observational evidence has revealed changes in the intensity, frequency, duration, and extent of weather extremes, such as heavy rainfall events and hot days, across certain regions and continents (Rahmstorf and Coumou, 2011; Slater et al., 2021). There has been much research relating weather indices to potential crop variability or projected damage (Harkness et al., 2020; Iizumi and Ramankutty, 2016; Rosenzweig et al., 2001; Trnka et al., 2014), but most work has described weather extremes by using seasonal or annual metrics rather than focussing on the periods most relevant to crop growth (Frich et al., 2002; Zhang et al., 2011). There is also increasing research focus on compound weather extremes (Zscheischler et al., 2020) occurring simultaneously or in close succession, such as very warm temperatures in the late autumn followed by abnormally wet conditions in spring (Ben-Ari et al., 2018) and their impacts on crop yields. Of the total annual crop losses in world agriculture, many are due to direct weather and climatic effects such as drought, flash floods, heavy rainfall in otherwise dry periods, frost, hail, and storms (Ray et al., 2019; Sultan et al., 2019). High temperatures and heat stress lead to stomatal closure and therefore reduced photosynthesis due to restricted CO2 diffusion (Chaves et al., 2003), offsetting potential yield gains that might otherwise occur with greater fertilization in a CO2-enriched environment (Ainsworth and Long, 2021). In some regions of the mid- and high latitudes, water excess may prove more detrimental to wheat yields than drought (Zampieri et al., 2017). However, for crops such as maize and soy yields, it has also been shown that heavy rainfall of up to 20 mm h−1 may even prove beneficial, highlighting the benefits of rainfall intensification in a warming climate (Lesk et al., 2020). Overall, there is thus a need to investigate historical data to elucidate the linkage between extreme temperature and rainfall over the agricultural phases of relevance to crop growth. Climate models may then be employed to explore how such linkages might evolve as the climate warms.
This work thus investigates (1) whether statistically significant associations exist between observed temperature/precipitation metrics and historical wheat yields during the three crop growth stages, in the three main wheat-growing regions of the UK, and (2) the extent to which projections of compound temperature and precipitation extremes under a high-emission scenario may impact future crop yields. To assess future changes in precipitation and temperature extremes, we employ state-of-the-art UK Climate Projections Local (UKCP 2.2 km) convection-permitting simulations, which constitute a step change in resolving small-scale processes in the atmosphere. These climate projections are considered the most reliable simulations presently available in terms of their ability to project future changes in meteorological extremes over the UK.
2.1 Wheat yield data
Geographically, we focus on the three main wheat-growing regions outlined using the EU “NUTS” classification (European Commission, 2010). These three regions are (i) north-eastern Scotland, eastern Scotland, and the north-east English region (SNE); (ii) the East Midlands, Yorkshire, and the Humber region (EMYH); and (iii) the south-east and eastern region (SEE; Fig. 1d). These three regions account for over 80 % of total UK wheat production by tonnage (DEFRA, 2020) and correspond with the yield reporting boundaries of available data. The regional wheat yield data were obtained from the UK Department for Environment, Food and Rural Affairs (Defra; DEFRA, 2020). The data are drawn from the England Cereals and Oilseeds Production Survey and Scotland Cereal Production and Disposal Survey, part of an annual survey of the UK agricultural industry. For full details of the survey methodology, see DEFRA (2018b). The data were summarized by Defra to average yield at the national (1885–2020) and regional (1990–2020) levels, resulting in 136 and 31 years of data, respectively.
The dates for the foundation, construction, and production growth stages are taken from benchmarks in the UK's “Wheat growth guide”, in Table 1 (AHDB, 2018). Prior knowledge on the effects of climate in different growth stages guides our choice of climate variables in the study (Table 1). Absolute anomalies of wheat yields were computed by fitting a locally weighted scatterplot smoothing curve (LOESS) to obtain the running mean (red lines shown in Fig. 1a and b), and subtracting this running mean from each annual value (resulting anomalies shown in Fig. 1c). We perform this calculation to remove the trend and thereby isolate annual anomalies, which we expect to be related to inter-annual climate variability rather than other factors such as long-term technological improvements, increasing atmospheric carbon dioxide, or climate warming.
2.2 Historical precipitation and temperature reference data
For historical climate data we employ the HadUK gridded 5 km observational data from the National Climate Information Centre (NCIC; Hollis et al., 2019). Provisional HadUK data were employed for the year 2020, produced as per previous years (Hollis et al., 2019); provisional data may have very small differences at regional scales compared with the final published dataset, available later in the year. Observed precipitation and temperature data were checked for completeness: any incomplete climate data during each of the crop growth stages (i.e. a foundation phase with less than 187 d of data; a construction phase with less than 60 d, or a production phase with less than 46 d) were removed, to ensure consistency and comparability across years.
To investigate the association with crop yields, we computed climate metrics within each geographical region and wheat growth stage (Table 2) using region-averaged values of temperature (∘C) and precipitation (mm). Specifically, for temperature, we derived the maximum, mean, and minimum of the region-averaged maximum daily temperature (max_maxT, mean_maxT, min_maxT), of the mean daily temperature (max_meanT, mean_meanT, min_meanT) and of the minimum daily temperature (max_minT, mean_minT, min_minT). For example, max_maxT indicates the day with the hottest (maximum hourly) temperature, and max_minT indicates the day with the warmest nighttime (minimum hourly) temperature, during a given growth stage. We also create metrics representing the daily variability in temperature (var_dailyT) and its seasonal variability (var_maxT, var_meanT, var_minT). For instance, var_maxT indicates the difference between the highest/lowest daily values of maximum hourly temperature in a season.
Table 2Association between observed climate metrics and wheat yields in each crop growth stage and region. Table indicates Pearson's correlation coefficients and their p values ( indicates p<0.01, , ). National data are tailored to the same time period as regional data here (31 years between 1990–2020) for comparability. Note: total_P and mean_ dailyP are equivalent. Some of the most relevant metrics with relatively consistent sign are indicated in bold font (see Fig. 7 for trends in these metrics).
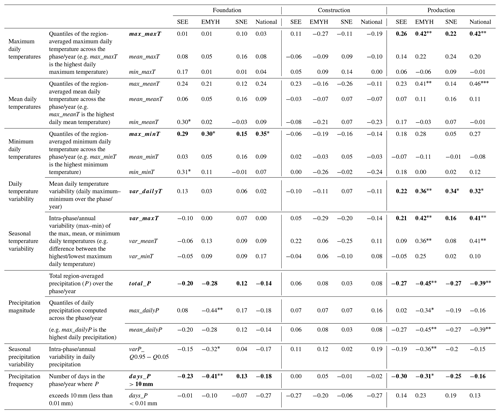
For precipitation, we computed metrics representing the total region-averaged daily precipitation within a growth stage (total_P) and its quantiles (max_dailyP or mean_dailyP), where max_dailyP is the maximum total daily precipitation within a growth stage. We also considered the variability in daily precipitation across a growth stage (varP_Q0.95−Q0.05); the number of heavy rainfall days where precipitation exceeds 10 mm (days_P>10 mm); and the number of dry days where precipitation is less than 0.01 mm (days_P<0.01 mm; Table 2). The heavy precipitation threshold is chosen based on the historical wheat yield literature for the UK (Thomas et al., 1989); other thresholds may be more relevant elsewhere. For instance, Lesk et al. (2020) found extreme rainfall impacts only at especially high intensities >50 mm h−1 for US maize and soy; others have used more holistic distributional measures like the wet-day Gini coefficient (Shortridge, 2019), a measure of daily rainfall variability.
2.3 UKCP Local (2.2 km) projections
The UKCP Local simulations have a spatial resolution of just 2.2 km – providing exceptional detail in local rainfall changes. Importantly, such high resolution allows the climate model to explicitly represent convective precipitation events on the model grid (see Kendon et al., 2019, 2020, for details), thus providing credible projections of future changes in short-duration precipitation extremes and in particular for summer months. The UKCP Local simulations were initially released in September 2019 (Kendon et al., 2019) but were then updated in July 2021 after correction of an error in the representation of graupel (soft ice pellets; Kendon et al., 2021). Here we use the new updated Local 2.2 km projections. The local 2.2 km model (HadREM3-RA11M) spans the UK and is nested within the 12 km regional model (HadREM3-GA705), which is in turn driven by the 60 km global model (HadGEM3-GC3.05; Andrews et al., 2019; Williams et al., 2018). The 2.2 km projections are available for three 20-year periods of 1981–2000, 2021–2040, and 2061–2080. Known atmospheric greenhouse gas (GHG) concentrations are prescribed as forcings to the historical 20-year period. For the second and third periods, the projections employed follow the RCP8.5 scenario, which assumes substantial on-going human burning of fossil fuels. The 2.2 km projections consist of an ensemble of 12 members (Table 3), each of which can be regarded as a plausible realization of the climatic response to rising GHG levels. The local members are driven by different members of the global coupled model ensemble and corresponding regional model ensemble, created by perturbing uncertain parameters in the model physics within their bounds of uncertainty. Thus, the range of the 2.2 km projections provides an estimate of the uncertainty in future changes due to natural variability while additionally accounting for uncertainty in the physics of the driving global climate model. We computed regionally averaged UKCP temperature and precipitation projections for each of the three regions shown in Fig. 1d and for each of the crop growth stages indicated in Table 1. For a detailed discussion of modelling assumptions and limitations see Sect. 2.6.
Table 3Bias correction factors for region-averaged total daily precipitation and minimum/mean/maximum daily temperature for each of the three regions (columns) and each of the 12 UKCP ensemble members (rows) relative to HadUK observed data. These are the complete data (ensembles 02, 03, and 14 do not exist in the UKCP Local dataset). Bias correction is performed using daily data over the common historical period 1 December 1980 to 30 November 2000. The bias correction factors are multiplicative for precipitation and additive for temperature.
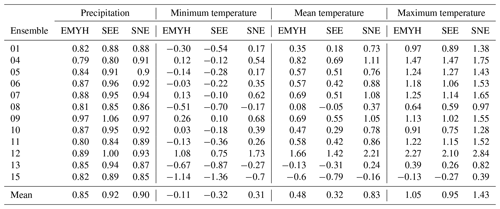
2.4 Bias correction
Given that the driving parent model of each UKCP Local simulation comes from a perturbed physics ensemble, each ensemble member is typically regarded as a different model and therefore is independently bias-corrected. UKCP Local simulations of area-averaged precipitation and temperature were bias-corrected against the 5 km area-averaged observed daily HadUK data (Hollis et al., 2019) for each geographical region, using the entire the historical period of December 1980 to November 2000 (Table 3). The bias correction scaling factors were identified and applied with the “hyfo” (Xu, 2020) package written in the software R. This bias correction approach is a simple scaling method which is additive for temperature and multiplicative for precipitation (one correction factor per ensemble, per region), so it preserves an absolute or relative trend, respectively. The UKCP data have 30 d in each month; therefore, to perform the bias correction we added calendar days for each of the three 20-year periods (e.g. from 1 December 1980 to 30 November 2000 with only 30 d in each month) and merged the historical period with observed data, removing any non-matched days (e.g. dropping the 31st of the month from the observed data, or dropping 29–30 February from the projections). This produced two overlapping time series of equal length over the period of December 1980 to November 2000 to perform the bias correction. We make the assumption these present-day biases are likely to extend into the future periods, a key caveat of any bias correction method. The bias correction factors are relatively small, which suggests the simulations are well-aligned with the historical observations: ×0.89 on average for precipitation for the three regions (individual factors for each member and region are shown in Table 3); −0.04 ∘C for minimum daily temperature, +0.54 ∘C for mean daily temperature, and +1.14 ∘C for maximum daily temperature. We apply the bias corrections to the two future UKCP periods (December 2020 to November 2040 and December 2060 to November 2080, recalling these are for the RCP8.5 scenario). The bias correction performs well at the annual scale (Fig. 2) but may differ across specific growth stages and regions (e.g. in the foundation phase, median precipitation is slightly overestimated in EMYH and SEE regions; Fig. 3). Bias-corrected projections inevitably contain some uncertainty and should be regarded as providing general directions of change.
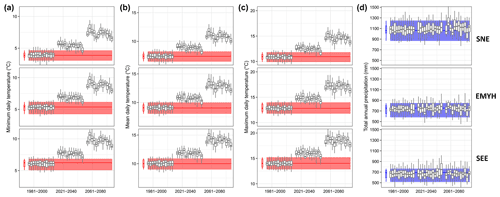
Figure 2Bias correction of each UKCP 2.2 km ensemble member, for (a–c) the minimum, mean, and maximum daily temperature (mean_minT, mean_meanT, and mean_maxT), respectively, and (d) total precipitation (total_P), in each year, for each of the three regions (top row: SNE; middle row: EMYH; bottom row: SEE). Red (blue) boxplots and rectangles indicate the range of observed temperature (precipitation) over the first period (1981–2000), based on the HadUK dataset. Grey boxplots indicate projections (1 for each of the 12 UKCP Local ensembles) for three periods (historical – 1981–2000; future – 2021–2040; 2061–2080) using RCP8.5. Boxplot hinges represent 25th and 75th percentiles, and the horizontal bar indicates the median. Whiskers extend to the largest value no further than 1.5 times the interquartile range (distance between 25th–75th percentiles) from the hinge.
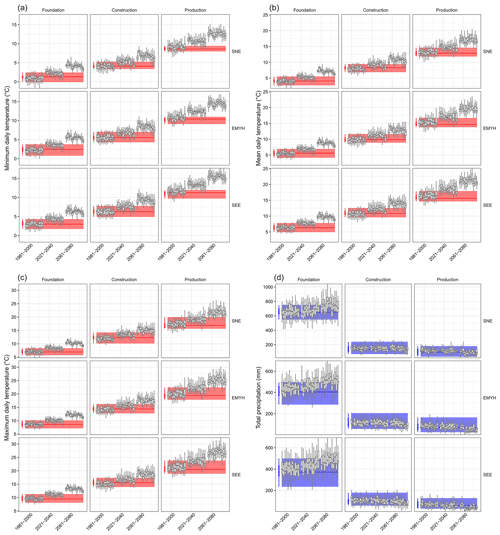
Figure 3Bias correction of each UKCP 2.2 km ensemble member, for (a–c) the minimum, mean, and maximum daily temperature (mean_minT, mean_meanT, and mean_maxT), respectively, and (d) total precipitation (total_P), within each phase, for each of the three regions (SNE, EMYH, SEE). Columns indicate the foundation, construction, and production phase, respectively. Red (blue) boxplots and rectangles indicate the range of observed temperature (precipitation) over the first period (1981–2000), based on the HadUK dataset. Grey boxplots indicate projections (1 for each of 12 UKCP Local ensembles) for three periods (historical – 1981–2000; future – 2021–2040; 2061–2080) using RCP8.5. Boxplot hinges represent 25th and 75th percentiles, and the horizontal bar indicates the median. Whiskers extend to the largest value no further than 1.5 times the interquartile range (distance between 25th–75th percentiles) from the hinge.
2.5 Statistical approach
We first assess the association between climate metrics and crop yield by using pairwise two-variable Pearson correlations (expressed as annual crop yield versus each individual seasonal climate variable). The magnitudes of the correlation coefficients and their p values are provided in Table 2.
Second, to assess the additive or offsetting effects of different climate conditions across crop growth stages, we develop a multiple linear regression model between regional crop yields and climate (Eq. 1). We develop one model per region, with different observed temperature and precipitation variables for each region. Using Table 2, we purposely select just two continuous variables per growth stage to develop the model (one temperature-based metric and one precipitation-based metric), thereby avoiding correlated metrics. The equation used to fit the observed data for a given region is formulated as
where y represents the wheat yields (t ha−1); x1 is foundationmax_minT (∘C), x2 is foundationtotal_P (mm), x3 is productionmax_maxT (∘C), and x4 is productiontotal_P (mm); β0 is the intercept; β1 to β4 are the regression slope coefficients for each of the explanatory climate variables; and ϵ is the error. The model statistics and coefficients are provided in Table 4 for each region. Although the model is significant (p<0.05) in EMYH, SEE, and NAT (national scale), the predictability is relatively low (Predicted R2 of 0.09 for NAT). Alternative metrics could also be selected, such as var_dailyT or var_maxT in the production phase, days_P>10 mm in either phase, or additional variables reflecting, e.g., precipitation intensity. These variables have not been tested and should be evaluated in future research, further developing the statistical crop model. Our model is a proof of concept that could be refined to improve the predictive skill if further data become available. The construction phase is not included in the regression model as it shows no consistent associations with wheat yields (Table 2). The multiple regression describes the “extremeness” of climate independently for each crop growth stage and so may account for compound positive and negative climate impacts on wheat yield across a year. For instance, detrimental climate conditions may have a cumulative impact on wheat yields if occurring across multiple growth stages, such as heavy precipitation events during the foundation phase (foundationtotal_P), followed by meteorological drought during the production phase (productiontotal_P). Conversely, poor conditions in one stage may be mitigated by good conditions or agronomic interventions in another stage (e.g. wet weather leading to increased incidence of fungal disease can be mitigated by subsequent increased use of fungicides), and this would be reflected by the regression model.
Table 4Statistics of the multiple linear regression model (Eq. 1) for each region and nationally (historical observed data, 1990–2020). The low R2 values can be explained by the fact that climate is only one of the mechanisms driving crop yields alongside agronomic management, as discussed in Sect. 3.3. Significance of the coefficients is indicated with stars ( indicates p<0.01, , ). We use these model fits to drive climate-based projections of future crop yields using the UKCP Local ensemble simulations (Fig. 6), assuming no future changes in agronomic management practices. The predictions issued by the regional models are similar to those issued by the national model (Fig. 6).
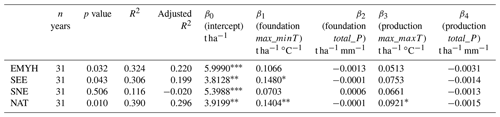
Third, to assess future changes in crop yields, we drive the same multiple regression model with the bias-corrected projections of the same variables, computed from the 12 members of the UKCP Local simulations (i.e. we employ a hybrid approach; see Slater et al., 2022). This approach allows us to fuse together the data-driven regression model with the meteorological simulations for higher greenhouse gas emissions. We use the model results to understand how multivariate climate change could lead to compensating or compounding impacts on future crop yields.
2.6 Assumptions and limitations
One of the advantages of the empirical data-driven approach herein is that there are fewer assumptions than in a process-based model approach. However, such an approach makes some key assumptions nonetheless, listed here.
-
To assess the impact of extreme weather on crop yields, we assume that the crop yields are affected by weather within the pre-defined crop growth stages described in Table 1. We employ fixed-in-time growth stages for practicality, but in reality these growth stages may be weather dependent from year to year, as plant vulnerabilities to extreme temperatures or precipitation may differ, e.g. from one July to another July. We did not use the 99 detailed physiological growth stages (AHDB, 2022) but rather the high-level growth stages which are defined over long time periods to split the year into key stages of wheat growth.
-
A major assumption in our regression-based approach is that wheat responses to climatic variables in the past are a reliable predictor of responses in the future. One important uncertainty that we do not consider is how wheat growth and water use might respond to increases in atmospheric CO2 (Ewert et al., 2002; Swann et al., 2016).
-
Spatially, we average the climate metrics over the three regions. This aggregation to regional scales may mask variation in the weather conditions occurring in individual grid cells (or farms) – for instance the regional average could show little change, but this could hide large local changes (such as less frequent but more intense bursts of rain), or contrasting directions of change within the region. Other spatial metrics, such as extracting the highest rainfall event within each region, may be worth testing in future work.
-
The multiple regression model describes the impact of compound climate effects in different growth stages on wheat yields but not that of antecedent conditions (memory effects). Compound effects are captured well by our model, e.g. frost conditions during the foundation phase and heavy waterlogging during the production phase might combine to produce poor conditions across the whole year. However, the model cannot assess whether the climatic impacts during the production phase are the same irrespective of “memory” impacts from the climatic conditions in the earlier plant development stages (for example, the antecedent effects of a warm winter and wet spring in leading to a crop failure, e.g. Ben-Ari et al., 2018).
-
For the future projections of altered meteorological conditions, the UKCP18 HadGEM3 climate model simulations (in which the UKCP Local 2.2 km simulations are nested) were only performed for the RCP8.5 pathway for atmospheric greenhouse gas concentrations, and we do not address emissions uncertainty from other scenarios. While the likelihood of such high on-going emissions is now considered low (Chen et al., 2021; Hausfather and Peters, 2020), the RCP8.5 scenario is commonly used to facilitate detection of climate signals in future projections above natural variations in the climate (due to the large changes projected) and was deliberately chosen as the configuration for UKCP Local simulations to maximize the signal to noise. Using a high-emission scenario also has the advantage that one can make estimates of climate changes for lower-emission scenarios using scaling approaches.
-
Our analysis employs one single model, the UKCP Local (2.2 km) climate projections. As described in Sect. 2.3, the UKCP Local simulations are driven by a perturbed physics ensemble (PPE) of a single forcing Earth system model (ESM), i.e. the parameters within the physics of the driving ESM are perturbed within their bounds of uncertainty. Thus, the 12 members of the high-resolution ensemble describe both internal climate variability and the climate modelling uncertainty in the driving model (i.e. they have wider uncertainty than is typically represented in one single climate model). The trends of the UKCP Local simulations therefore at least partially cover the range of uncertainty and trends that would occur in the ESMs developed by other climate research centres. However, the climate modelling range of uncertainty is likely to be underestimated since the UKCP Local ensemble lacks information from other international climate models. In winter, the UKCP Local simulations show some higher-precipitation responses compared to the full CMIP5 ensemble due to the improved representation of winter-time convective showers in the Local 2.2 km model (Kendon et al., 2020). UKCP Local projections also project relatively high temperature changes compared with other climate models (see, e.g., https://interactive-atlas.ipcc.ch/regional-information, last access: 22 September 2022). Changes in summer precipitation show a considerable drying in the UKCP Local projections, whereas the CMIP5 (and hence likely also CMIP6) simulations indicate that outcomes with more modest reductions or small increases should also be considered (Kendon et al., 2021).
-
The UKCP Local projections provide high spatial-resolution (2.2 km) downscaling of global climate model projections specifically for the UK. Such high-resolution simulations are able to at least partially resolve convective storms and do not require a parameterization scheme to provide a representation of convection, which is a simplification of the real world and a known source of model deficiencies. These simulations are therefore considered more reliable for projecting future changes in rainfall characteristics. However, there is still uncertainty in the parameterization of UKCP Local, and so it can be expected that as future research groups also build convective-permitting models, differences will emerge that we are presently unable to account for.
3.1 Historical increases in wheat yields and inter-annual yield volatility
Since the late 1800s, and especially since the 1950s, there has been exceptional growth in UK wheat yields due to rapid advances in crop breeding, increasing farm mechanization, and the availability of agrochemical inputs, such as fertilizers (Fig. 1a). Sustained increases throughout the 1980s–90s reflect the development of farming technologies, varieties, improved nutrient use efficiency and effective pesticides and growth regulators. Available time series of crop yields are much shorter when disaggregated to the regional level (Fig. 1b) than at the national level (Fig. 1a). Of particular note, though, is that the EMYH and SNE regions exhibit some levelling of wheat yields since 1990, mirroring the national trend, while the southernmost region, SEE, has seen some continued increases (Fig. 1b).
In addition to increases in mean yields, the national yield time series exhibits a visible increase in the variance of yields in the last few decades (Fig. 1c). This increase in volatility is not solely driven by increases in the mean of the time series. A comparison of the variance of crop yields between the periods 1885–1989 (105 years) and 1990–2020 (31 years) using both Levene's test (p=0.022) and the non-parametric Fligner–Killeen's test (p=0.093) indicates that there is a significant difference in the variance. The results are even more significant when comparing periods of similar length, 1960–1989 and 1990–2020 (30–31 years), for both Levene (p=0.002) and Fligner–Killeen (p=0.003) or focussing on the last 2 decades, 1970–1999 and 2000–2020 (30–21 years); p<0.001 for both tests. A question of notable interest, therefore, is understanding why the variance of yields has significantly increased and whether it might be associated with more frequent or intense weather extremes.
3.2 Association between climate extremes and wheat yields in each crop growth stage
We assess the association between seasonal climate and crop yields by using precipitation and temperature metrics during the three crop growth stages. We expect the association between climate anomalies and wheat yields to differ regionally due to a range of factors, including the resilience of the wheat plant, husbandry practices of farmers and agronomists, biophysical conditions (e.g. soils, day length), and climatic differences (e.g. rainfall tends to be more frontal in the north, with orographic rainfall over high ground, and more convective in the south-eastern UK). Although only some of the associations between the seasonal climate metrics and annual crop yields are statistically significant, we show all the associations and their relative strength for full transparency (Table 2). In Figs. 4 and 5, we focus on total_P, max_minT, and max_maxT in each growth stage, as these are some of the most consistent metrics in the historical data (Table 2); figures produced using max_maxT give very similar patterns to max_meanT (not shown). These figures reveal the climatic “space” generated by the interaction between precipitation and temperature in each growth stage: some of the worst UK wheat yields in recent decades have occurred during years with anomalously high/low seasonal rainfall or prolonged heat, an important indicator of crop heat stress (Arnell and Freeman, 2021). The figures also indicate that temperature and precipitation are not independent from one another, since the wet years with poor yields also tend to be relatively cool (e.g. 2001, 2020 in the foundation phase) and the dry years can sometimes be particularly hot (e.g. 1976, 2018 in the production phase; Fig. 5).
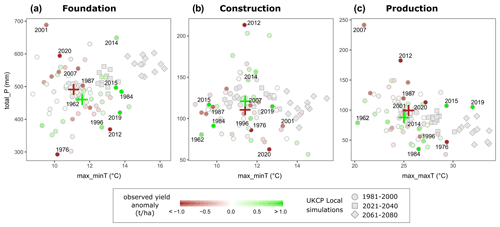
Figure 4Association between observed wheat yields and climate during the three wheat-growing phases. Anomalies of observed UK wheat yields are shown for total area-averaged precipitation (total_P) and the maximum of area-averaged minimum/maximum daily temperature within each phase (i.e. the metrics total_P, max_minT, and max_maxT, chosen for their associations with crop yields; Table 2), alongside UKCP projections. (a) Foundation phase (1 October to 9 April); (b) construction phase (10 April to 10 June); (c) production phase (11 June to 26 July). Yield time series are shown for the national scale here (longer than regional time series; see Fig. 1a vs. Fig. 1b) and are the same in the three panels. Small green circles indicate positive yield anomalies for individual years; small brown circles indicate negative yield anomalies for individual years. Large green crosses indicate the mean for all the years with positive wheat yield anomalies; large brown crosses indicate the mean for all the years with negative wheat yield anomalies. Grey diamonds indicate UKCP Local projections of temperature and precipitation for the historical (circle: 1981–2000) and future (square: 2021–2040; diamond: 2061–2080) periods, where each symbol indicates 1 of the 12 ensemble members. Specific years mentioned in the main text are labelled.
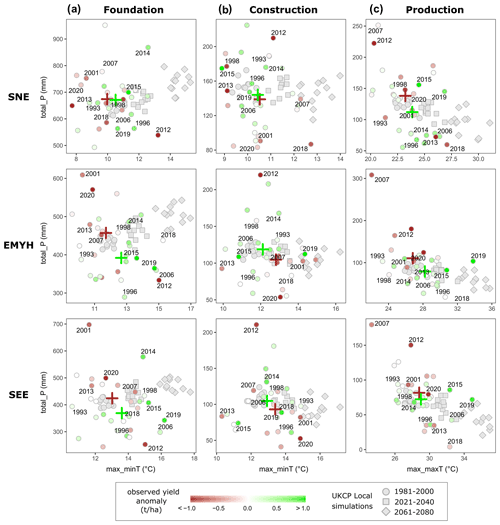
Figure 5Association between wheat yields and climate during the three wheat-growing phases and in each of the three UK wheat-growing regions. Anomalies of observed UK wheat yields are shown for total area-averaged precipitation (total_P) and the area-averaged minimum/maximum daily temperature within each phase (i.e. the metrics total_P, max_minT, and max_maxT, chosen for their associations with crop yields; Table 2), alongside UKCP projections. Columns (a–c): same as Fig. 4. Rows: SNE, EMYH, SEE. Yield time series are shorter at the regional scale than national (see Fig. 1b). Small green circles indicate positive yield anomalies for individual years; small brown circles indicate negative yield anomalies for individual years. Symbology is the same as Fig. 4.
From a crop physiology perspective, in the foundation phase (October to early April; Table 1), prolonged waterlogging of the soil may suppress wheat yields by restricting root development and plant growth (AHDB, 2018). We find a significant negative association between crop yields and the number of heavy rainfall days in the EMYH region (Table 2, days_P>10 mm), as can be seen in Fig. 5 (years 2001, 2020; Fig. 4a). The association between yields and total_P days_P>10 mm and yields is also negative in SEE and at the national scale but not significantly so. In the winter of 2000/01, for instance, wet autumn and winter conditions resulted in delayed sowing and poor seedbed conditions. Additionally, colder-than-usual conditions in the foundation stage (e.g. year 2013, not shown) may delay or prevent crop tillering: frost can damage early drilled and fast-growing varieties, while frost heave can kill seedlings. We find significant positive associations between yield and max_minT at the national scale and in the EMYH region and with min_meanT and min_minT in the SEE region (Table 2). The positive associations indicate that warming temperatures may benefit UK wheat yields in a warming climate.
While crops are growing rapidly during the construction phase (April to early June), both late frosts and dry weather can reduce crop growth (Table 1). For this period in each year, we find no significant associations between climate characteristics and crop yields (Table 2). This is not necessarily a contradiction, as reduced growth does not always carry through to reduced yield. Both low yields (e.g. years 1976, 2001, 2020; Fig. 4b) and some high yields (1962, 1984) have occurred during drier-than-average construction phases. Overall, wheat yields seem to be more sensitive to climate conditions during the foundation or production phases.
The clearest association between climate extremes and crop yields seems to be in the production phase, which is the time from post-flowering to harvest (summer: June and July). It is during this phase that yields may be susceptible to both drought and water logging (Table 1). We find a consistently negative association between heavy rainfall (both total_P and days_P>10 mm) and crop yield in all three regions. For total_P the association is significant in EMYH and at the national scale and for days_P>10 mm in EMYH (Table 2). The association between low wheat yields and high summer rainfall is apparent in specific years such as 2007 and 2012 (top left corner of Figs. 4c and 5c). For example, the year 2012 witnessed exceptionally poor yields due to high spring and summer rain, a high incidence of fungal disease (e.g. Septoria tritici; DEFRA, 2012), and low sunlight during the grain-filling period (i.e. the first part of the production period, when the grain is swelling and requires sunlight for photosynthesis). In contrast, good yield years are often associated with warm summer temperatures and moderate to low rainfall: this can be seen in the positive associations between wheat yields and max_maxT or max_meanT which are significant both nationally and in EMYH (Table 2). Examples are the years 2015 and 2019 (Figs. 4c and 5c). During the production phase, meteorological drought conditions may also have negative impacts. Hot, dry weather shortens the growth period, resulting in early canopy senescence and reduced grain weight (Table 1). Indeed, some of the UK's poorest crop yields occurred during warm, dry summers (e.g. years 2013, 2018 in SNE and SEE; Fig. 5c). The benchmark grain-filling period is 45 d from flowering until maximum dry weight in late July, but it can be as short as 28 d during severe droughts (AHDB, 2018).
3.3 Explaining the association between crop yields and climate extremes: compound impacts across growth stages
It can be challenging to systematically identify the weather conditions to which wheat yields are most vulnerable within individual growth stages. Most of the correlations in the historical data are not statistically significant (Table 2). The often relatively weak association between climate anomalies and wheat yields at the level of individual growth stages can be explained partly by the shortness of observational records, the combined resilience of the wheat plant (i.e. physiological reproductive mechanisms), and the husbandry skills of farmers and agronomists in mitigating these impacts by adjusting to climatic extremes. There is thus a role for agronomic management in mitigating apparent relationships with climate: this role might not be as direct as irrigating in response to drought, but farmers can dampen the effects of climatic variation through crop management, for example, by changing fungicide regimes to respond to increased fungal disease brought about by wetter conditions, changing the timing or amount of inputs of nutrients, pesticides, and growth regulators (Knight et al., 2012). The relatively intensive nature of UK wheat production (Hillocks, 2012; Wesseler et al., 2015) may thus be sufficient to dampen crop responses to climatic variation (Gagic et al., 2017). Farmers can also change many other aspects of management, including wheat variety, tillage, sowing date, sowing rate, or harvest date, in response to forecast or current conditions. Wheat cultivars are bred with a measure of resistance to certain climatic variables, so a farmer can select a cultivar appropriate to local climatic conditions (Kahiluoto et al., 2019).
Low correlations between climate and yield anomalies over seasonal wheat growth stages may also reflect compensatory effects between growing phases. For instance, a less than ideal foundation phase might be offset by a favourable production phase or vice versa. It is equally important to note that growing phases in real plants are determined by their growth rather than calendar days. Thus a phase may last longer, resulting in delayed crop growth but maintaining the expected yield. Our calendar-fixed phases are a simplification of this process.
Conversely, cumulative detrimental impacts of climate across stages (e.g. accumulated rainfall and subsequent waterlogging) may be one of the most damaging factors affecting overall annual crop yields. In other words, the flexibility and techniques farmers have at their disposal to adapt to climate variability are bounded. For instance, low yields in the year 2018 were due to very dry conditions in the foundation stage, followed by very hot and dry conditions in the construction and production stage (DEFRA, 2018a). In contrast, very low yields in the years 2001 and 2007 were caused by a combination of high rainfall in the foundation and production stages (Fig. 4). The exceptionally wet winter of 2019 (affecting the 2020 harvest in Fig. 4) also imposed severe constraints on farming operations and resulted in a reduction in the areas of autumn-sown crops. These examples illustrate why a full understanding of projected changes to temperature and precipitation across wheat growth stages is required.
To try to assess the offsetting or additive effects across growth stages, we develop a simple multiple regression model relating the observed wheat yields in each region to just two metrics reflecting temperature and precipitation conditions in the most important stages based on the outcomes of Table 2: foundationmax_minT, foundationtotal_P, productionmax_maxT, and productiontotal_P. We find this model is significant at the 95 % level (p<0.05) for EMYH, SEE, and the national scale but not SNE (Fig. 6, Table 4). The lack of significance in SNE can be easily explained, since the association between yields and foundationmax_minT is weak there (R=0.15) but good elsewhere ( in SEE and EMYH). Similarly, the association between foundationtotal_P and annual yields is negative in other regions ( in SEE and EMYH) but weakly positive in SNE (Table 2). The multiple regression model displays the best fit in the EMYH region, where the climate metrics display the strongest correlations with yields (significant for foundationmax_minT, productionmax_maxT, and productiontotal_P). As expected, these model fits only explain a portion of yield variation (R2 ranges from 0.12 for SNE to 0.32 for EMYH), since crop yields are only partially explained by climate, as discussed above. However, the models allow us to capture the multivariate impacts of temperature (foundationmax_minT and productionmax_maxT exhibit a positive association with crop yields) and precipitation (foundationtotal_P and productiontotal_P exhibit a negative association with yields). Thus, the strongest associations between climate and yield anomalies may occur during years with cumulative climate impacts across growth stages. Cumulative impacts can be seen in the improved R2 in the multiple regression compared to the pairwise correlations. In other words, the model is capturing something individual variable correlations do not, and this could be that compensation across phases. That said, whether this added explanatory power is from inter-stage compensation or compensation between variables within a single stage is not clear from the regression results alone.
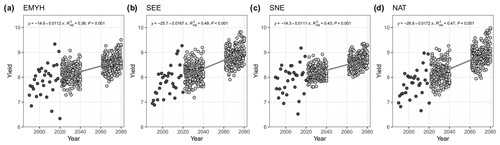
Figure 6Temporal trends in wheat yields (t ha−1): observations and future projections. The observations (black circles, 1990–2020) are the same as in Fig. 1. The projections (grey circles; 12 members per year) are obtained by forcing a multiple linear regression model (Eq. 1; Table 4) obtained for each region with the UKCP Local projections of the same climate variables in each growth stage (see Sect. 2.5). Grey lines indicate the linear regressions between the ensemble of projected values and time (the regression equation, adjusted R2, and p value are indicated in each panel).
3.4 Annual projections of future climate conditions and implications for crop yields
At the annual scale, projections of future maximum hourly temperature are available for the periods 2021–2040 and 2061–2080 from the UKCP Local simulations. The interquartile range of projected temperature for 2021–2040 lies well above the median of historical extremes (Fig. 2a–c). Future high-temperature conditions generally fall beyond the bounds of annual variability experienced in the contemporary period for all three wheat-growing regions (Fig. 2c). As expected, changes are largest for the later modelled period 2061–2080, corresponding to higher atmospheric greenhouse gas concentrations. This exceedance of historical thresholds by temperature projections is true for all 12 UKCP Local ensemble members, independent of uncertainty in changes in the large-scale conditions sampled by perturbing parameters in the Hadley Centre global climate model. However, it is important to note that the 12 climate model members (Table 3) do not sample the full range of uncertainty, evident in differences between all available global climate models (Kendon et al., 2021); see Sect. 2.6 for a discussion.
For total annual precipitation (Fig. 2d), the projections do not indicate a very obvious increase or decrease in any of the three regions relative to the historical period, although SNE may seem very slightly wetter and SEE very slightly drier on average (comparing medians) in the later period (2061–2080). This lack of trend in yearly data may be explained by the opposing changes in the different seasons: in general the winter season is projected to become wetter and the summer drier (Kendon et al., 2021). Importantly, there are also changes in the underlying intensity and frequency of precipitation (e.g. significant increases in days_P>10 mm and var_P in the foundation phase, Fig. 7), which are not evident from simply looking at trends in annual mean precipitation.
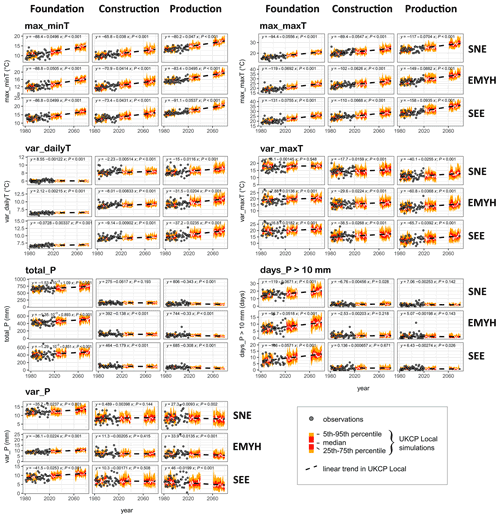
Figure 7Trends in key climate metrics for the three growth stages (columns) and three regions (rows). Metrics are selected from (and defined in) Table 2: max_minT, max_maxT, var_dailyT, var_maxT, total_P, days_P>10 mm, and var_P. Dark grey circles indicate observations; colour ribbons are the 12 UKCP Local members (5th–95th percentile in orange, 25th–75th percentile in red, and the median as a white line). Linear trend lines for the UKCP simulations are shown as dashed black lines, along with the regression equation and p value in each panel.
3.5 Seasonal projections of future wheat-growing conditions and crop yields
When considering UKCP Local projections by wheat growth stages (instead of at the annual scale), clearer patterns become apparent (Fig. 3). We expect to find spatial differences in the climate projections, as they exhibit north–south gradients in changes across the UK. Even in a single ensemble, there are gradients in the future changes in rainfall which differ from present-day climatology and relate to regional differences in increases in moisture availability as well as changes in circulation patterns. The question of compound climate change – i.e. the joint impacts of temperature and rainfall or moisture availability – is important for future crop yields.
Contrary to global expectations of declining yields under climate change, the multiple regression model indicates that projections of future temperature and precipitation change are likely to contribute to a continued growth of future wheat yields in the UK (Fig. 6). These projections rely on broad estimates of changing night/day temperature extremes as well as total rainfall in the foundation and production stages. It is possible that more data may provide greater information on changing water availability, atmospheric vapour demand, and plant stress; however with the existing observations our data-driven approach highlights that a changing climate may not be entirely negative for wheat yields. This can be explained as follows.
For the foundation phase (October to early April), all regions can expect to see progressively warmer, wetter conditions in the coming decades according to the UKCP simulations. Significant projected increases in max_minT, max_maxT, and total_P are evident in all three regions (Fig. 7). Such conditions might not necessarily adversely affect wheat production (Fig. 4) and are likely to be beneficial in decreasing the risk of frost damage (Table 2). When considering max_minT and total_P, the projections indicate that there is a good chance of seeing more temperate winters similar to the one preceding year 2015, where foundation conditions were warm and not too wet, resulting in high crop yields (Fig. 4a); however, the significant projected increases in total rain, heavy rain, and rainfall variability (total_P, days_P>10 mm and var_P; Fig. 7) in all three regions may equally prove problematic beyond certain thresholds. In very wet years, the UK may also experience winters more like those of 2001 and 2020, which led to low yields across the UK (Fig. 4a), especially in EMYH/SEE (Fig. 5a).
Projections for the construction phase (mid-April to mid-June) are not included in the multiple regression model, due to the lack of significant associations between climate and wheat yields (Table 2). During this phase, the projections indicate significant decreases in total_P in EMYH and SEE but not SNE (Fig. 7). There are no evident changes in heavy rain (days_P>10 mm; Fig. 7), and we find considerable overlap with both good and poor yields in the historical data (Fig. 4b). These findings suggest that the construction phase may not necessarily be the most at-risk in terms of the impacts of changing UK climate on crop yields. Although precipitation may not change much, there is still warming, which will increase atmospheric vapour demand (all else being equal). Thus, understanding the effects of compound change such as heat waves and drought (Zampieri et al., 2017) or the evaporative role of temperature (Lobell et al., 2013) is important to help provide more robust conclusions about the future.
In the production phase (mid-June to end of July), UKCP simulations project both much warmer and drier conditions (Figs. 3 and 7). The drying signal is relatively similar across the three regions and becomes more apparent in the later simulations towards the end of the century. It is important to note that the UKCP Local projects stronger drying than CMIP5-6 models. Projected trends also indicate significant, strong increases in max_minT, max_maxT, and equally in temperature variability (var_dailyT and var_maxT; Fig. 7). A simple analogue approach suggests we may see more production phases similar to the years 2006, 2015, and 2019 in the EMYH/SEE regions, conducive to high yields (Fig. 5c). Both the national and the regional data suggest all regions may benefit from a warmer and drier production phase (Figs. 4c and 5c). The projected trends reveal significant decreases in rainfall total and variability (total_P and var_P) in all three regions but no apparent decreases in heavy rain (Fig. 7). However, individual anomalous years with poor yields and warm dry conditions remain plausible, such as the year 1976 at the national scale (Fig. 4c) and 2013 in the SNE and SEE areas (Fig. 5c). Because the projected high-temperature conditions are outside those experienced in the historic period, there is also a risk that the positive association between hotter, drier production phases and enhanced yield found in the historical observations will no longer hold. This is especially true since temperature could have non-linear impacts (e.g. sterility or abortion of formed grains) through the physiological effects of frost and heat shock (Barlow et al., 2015). Droughts and heatwaves severe enough to have a substantial impact on yield are rare in the historic data (Knight et al., 2012), and so we have few data by which to determine at what thresholds temperature and dryness cease to be beneficial for wheat and begin to have negative impacts. However, the anomalous years (e.g. 1976 and 2013) suggest that this can occur, and recent research indicates that days exceeding heat stress temperatures for wheat are likely to increase under climate change (Arnell et al., 2021).
Overall, projections of future temperature and precipitation conditions suggest a continued increase in future wheat yields when relying on max_minT, max_maxT, and total_P (Fig. 6). The higher yields are found in the far-future period (2061–2080) partly due to the effect of warming conditions and thus reduced frost risk in the foundation phase. These beneficial impacts may however be offset by the significant increases in heavy rainfall (and rainfall variability) projected in the foundation phase and enhanced meteorological drought conditions in the production phase (Fig. 7). The offsetting between climate effects, e.g. the interactions between temperature and precipitation, is an important mechanism and uncertainty both in the climate and in terms of their implications for crops. For instance, very hot conditions in the UK can often only be reached with a dry land surface (visible as apparent negative temperature–precipitation correlations during the production stage, Figs. 4 and 5). Drought and heatwaves are believed to self-intensify and propagate due to feedbacks between the land and atmosphere (Miralles et al., 2019). Cool and wet conditions could also be linked physically (e.g. production phase in 2007 and 2012), with implications for crop yields. This raises questions about joint heat and moisture impacts and how their interdependence might evolve into the future as greenhouse gases rise.
Lastly, the impact of rising atmospheric CO2 on crop water use is an important uncertainty which is hard to model via our statistical approach and likely to impact future crop growth (Ewert et al., 2002; Swann et al., 2016) but is not considered herein. Overall, our approach suggests that, on average, climate change is likely to have more positive impacts on UK wheat yields than previously considered. However, against this background of average positive change, our work illustrates that we are likely to move outside of the climatic envelope which wheat farming in the UK has previously adapted to. Thus, the new weather conditions generated by the effects of rising temperatures (including intense local thunderstorms) are only likely to increase the degree to which farmers may struggle to mitigate against climate impacts.
Mean UK crop yields saw a rapid growth in the 1950s followed by a plateau in the 1990s and then substantial increases in the inter-annual variability in yields. This acceleration has been challenging for UK wheat farmers, since crop yields over the past 2 decades (2000–2020) have been significantly more volatile than over the previous century (Fig. 1).
A first question is thus our ability to explain such changes, and assess whether statistically significant associations exist between observed temperature/precipitation metrics and historical wheat yields during the three crop growth stages, in the three main wheat-growing regions of the UK. While the plateau in yields can be explained by a variety of technological and agronomic factors (Knight et al., 2012), we find some evidence that yields over the last 30 years can be partially explained by climate metrics such as warm night temperatures and heavy rainfall days in the foundation phase (principally in the EMYH region) or maximum daily temperatures, daily temperature variability, and total precipitation in the production phase (Table 2; with correlation strength and significance varying regionally). Significant statistical associations are found principally in the foundation and production phases and for regions EMYH and NAT. Yields are more fully explained when considering a multiple regression model characterizing additive and offsetting impacts of climate across growth phases (e.g. detrimental impact of very cold temperatures in foundation phase followed by very high precipitation in the production phase). However, it is unclear whether the added explanatory power of the regression model is from inter-stage compensation or compensation between variables within a single growth stage. This would be an area for further research. The data-driven regression could additionally be refined by including various thresholds (e.g. considering the beneficial impacts of a warm and dry production phase only up to certain limits relevant to plant stress). We find the association between historical climate and crop yields is most evident in years which saw compound extremes (Zscheischler et al., 2020), i.e. climate anomalies across multiple growth stages (e.g. 2007, 2012, 2020, Figs. 4 and 5), “escaping” the ability of farmers to adapt through agronomic means. Outside these combined extremes, the data indicate a strong inter-annual resilience of wheat production, implying that at present farmers can, and do, successfully utilize crop husbandry to maintain yield levels.
Our second question seeks to understand how projections of compound temperature and precipitation extremes might impact future crop yields under a high-emission climate scenario. Overall, the data provide a surprisingly favourable outlook of climate conditions for future crop yields. During the foundation phase, high seasonal values of night temperatures (max_minT) are correlated positively and significantly with crop yields in EMYH and nationally (Table 2), suggesting that the significant future increases projected by the UKCP Local simulations (Fig. 7) are likely to provide more beneficial growing conditions during the winter. These positive temperature impacts may be offset by the significant projected increases in rainfall total, heavy rainfall, and rainfall variability in all three regions (total_P, days_P>10 mm, and var_P, Fig. 7), since heavy rainfall is detrimental to wheat yields (in EMYH especially; Table 2). Later in the year during the production phase, when high day temperatures are significantly and positively associated with wheat yields in EMYH and nationally (Table 2), the UKCP local simulations also project significantly warmer and drier mean conditions (Fig. 7), which may be conducive to positive yields, similar to the years 2015 and 2019 (Fig. 4). Since high rainfall totals in the production phase adversely affect growing and production conditions (total_P is negatively and significantly associated with crop yields in EMYH and nationally, Table 2), the projected significant decreases in future rainfall (which are stronger in UKCP Local than in CMIP5 and 6) could equally be beneficial to wheat yields (total_P, Fig. 7). Future anomalous years similar to 2020, with a wet crop foundation phase and a much drier construction phase that significantly suppressed yields (Fig. 4), are a possibility (Fig. 7). It seems plausible that the farming community may also face increased inter-annual variability in the future, e.g. a sequence of dry years (similar to 2019) followed by very wet years (2001, 2012) against a backdrop of warmer and wetter/drier conditions. Further analyses could equally assess whether the optimal time and place to grow wheat is changing or the effects of changes in rainfall patterns at the local (rather than regional) scale.
In summary, this work provides evidence that wheat yields over the last 30 years are associated with combined temperature and precipitation extremes, especially across the crop foundation and production phases, in the EMYH region and nationally (Table 2). Although the climate projections provide a generally positive outlook for future yields across the UK, it is important to note that the relationships between past wheat yields and historic climatic conditions may not be adequate guides to the risks associated with projected future conditions, as future temperature extremes and rainfall lie outside the range of conditions that UK agriculture has so far experienced. Further, this work studies climate extremes at the regional scale but not local changes in rainfall intensity and variability, which are beyond the scope of the paper (e.g. drier average regional conditions may hide less frequent but more intense local thunderstorms). Out of caution, therefore, a priority is to continue developing agricultural systems resilient to emerging climate patterns, as the global demand for wheat and other crops has been projected to double from 2005 to 2050 (Tilman et al., 2011). As higher-resolution crop yield data become available, further research into robust process-based or AI-informed crop models, alongside improved collaboration across spatial, governance, and supply-chain scales (Holman et al., 2021), will be required to help farmers adapt to evolving climate conditions and maintain the security of wheat production.
All data employed in the paper are publicly available as described in the “Methods” section. (1) HadUK gridded 5 km observational temperature and precipitation data were obtained from the National Climate Information Centre (https://dap.ceda.ac.uk/badc/ukmo-hadobs/data/insitu/MOHC/HadOBS/HadUK-Grid/, Hollis et al., 2019). (2) UKCP 2.2 km Local temperature and precipitation projections were obtained from the UK Met Office (https://www.metoffice.gov.uk/research/approach/collaboration/ukcp/data/index, Kendon et al., 2019). (3) UK wheat yields were obtained from the UK Department for Environment, Food and Rural Affairs (Defra; DEFRA, 2020). The code to produce the analyses can be obtained from the corresponding author upon reasonable request.
LJS led the coding, analysis and visualization. CH extracted the regional UKCP Local projections. All authors (LJS, CH, RFP, JWR, EJK) contributed to designing the experiments and writing the paper.
The contact author has declared that none of the authors has any competing interests.
Publisher’s note: Copernicus Publications remains neutral with regard to jurisdictional claims in published maps and institutional affiliations.
We gratefully thank the editor and reviewers (Corey Lesk and two other anonymous reviewers) for their helpful comments, which greatly improved the paper. Louise J. Slater gratefully acknowledges funding from the UK Research and Innovation FLF scheme (grant no. MR/V022008/1). Chris Huntingford, Richard F. Pywell, and John W. Redhead gratefully acknowledge the Agland project. Chris Huntingford also acknowledges the NERC CEH National Capability Fund. Richard F. Pywell, Chris Huntingford, and John W. Redhead were supported by research programme LTS-M ASSIST – Achieving Sustainable Agricultural Systems (grant no. NE/N018125/1), funded by NERC and BBSRC. Elizabeth J. Kendon gratefully acknowledges funding from the Joint UK BEIS/Defra Met Office Hadley Centre Climate Programme (grant no. GA01101).
This research has been supported by the Natural Environment Research Council (grant no. NE/N018125/1), the Department for Business, Energy and Industrial Strategy, UK Government (grant no. GA01101), and UK Research and Innovation (grant no. MR/V022008/1).
This paper was edited by Gabriele Messori and reviewed by Corey Lesk and two anonymous referees.
AHDB: Wheat growth guide, Kenilworth, Warwickshire, https://cereals.ahdb.org.uk/media/185687/g66-wheat-growth-guide.pdf (last access: 1 January 2021), 2018.
AHDB: AHDB Harvest Report, Report 6 – Week 13, Week ending 6 October, https://ahdb.org.uk/cereals-oilseeds/gb-harvest-progress (last access: 22 September 2022), 2020.
AHDB: The growth stages of cereals, Kenilworth, Warwicksh, https://ahdb.org.uk/knowledge-library/the-growth-stages-of-cereals, last access: 22 September 2022.
Ainsworth, E. A. and Long, S. P.: 30 years of free-air carbon dioxide enrichment (FACE): What have we learned about future crop productivity and its potential for adaptation?, Glob. Chang. Biol., 27, 27–49, https://doi.org/10.1111/gcb.15375, 2021.
Andrews, T., Andrews, M. B., Bodas-Salcedo, A., Jones, G. S., Kuhlbrodt, T., Manners, J., Menary, M. B., Ridley, J., Ringer, M. A., Sellar, A. A., Senior, C. A., and Tang, Y.: Forcings, Feedbacks, and Climate Sensitivity in HadGEM3-GC3.1 and UKESM1, J. Adv. Model. Earth Syst., 11, 4377–4394, https://doi.org/10.1029/2019MS001866, 2019.
Arnell, N. W. and Freeman, A.: The effect of climate change on agro-climatic indicators in the UK, Clim. Change, 165, 40, https://doi.org/10.1007/s10584-021-03054-8, 2021.
Arnell, N. W., Freeman, A., Kay, A. L., Rudd, A. C., and Lowe, J. A.: Indicators of climate risk in the UK at different levels of warming, Environ. Res. Commun., 3, 095005, https://doi.org/10.1088/2515-7620/ac24c0, 2021.
Barlow, K. M., Christy, B. P., O'Leary, G. J., Riffkin, P. A., and Nuttall, J. G.: Simulating the impact of extreme heat and frost events on wheat crop production: A review, F. Crop. Res., 171, 109–119, https://doi.org/10.1016/j.fcr.2014.11.010, 2015.
Ben-Ari, T., Boé, J., Ciais, P., Lecerf, R., Van Der Velde, M., and Makowski, D.: Causes and implications of the unforeseen 2016 extreme yield loss in the breadbasket of France, Nat. Commun., 9, 1–10, https://doi.org/10.1038/s41467-018-04087-x, 2018.
Brisson, N., Gate, P., Gouache, D., Charmet, G., Oury, F. X., and Huard, F.: Why are wheat yields stagnating in Europe? A comprehensive data analysis for France, F. Crop. Res., 119, 201–212, https://doi.org/10.1016/j.fcr.2010.07.012, 2010.
Chaves, M. M., Maroco, J. P., and Pereira, J. S.: Understanding plant responses to drought – From genes to the whole plant, Funct. Plant Biol., 30, 239–264, https://doi.org/10.1071/FP02076, 2003.
Chen, D., Rojas, M., Samset, B. H., Cobb, K., Diongue Niang, A., Edwards, P., Emori, S., Faria, S. H., Hawkins, E., Hope, P., Huybrechts, P., Meinshausen, M., Mustafa, S. K., Plattner, G. K., and Tréguier, A. M.: Framing, Context, and Methods, in: Climate Change 2021: The Physical Science Basis. Contribution of Working Group I to the Sixth Assessment Report of the Intergovernmental Panel on Climate Change, Cambridge University Press, Cambridge, United Kingdom and New York, NY, USA, 14–286, https://www.ipcc.ch/report/ar6/wg1/downloads/report/IPCC_AR6_WGI_FullReport.pdf (last access: 22 September 2022), 2021.
DEFRA: Farming Statistics – Final Crop Areas, Yields, Livestock Populations and Agricultural Workforce at 1 June 2012, United Kingdom, https://assets.publishing.service.gov.uk/file/183200/defra-stats-foodfarm (last access: 22 September 2022), 2012.
DEFRA: United Kingdom Cereal Yields, 2020.
DEFRA: Agriculture in the United Kingdom 2018, 1–111, https://assets.publishing.service.gov.uk/government/uploads/system/uploads/attachment_data/file/741062/AUK-2017-18sep18.pdf (last access: 22 September 2022), 2018a.
DEFRA: Farming Statistics – Final Crop Areas, Yields, Livestock Populations and Agricultural Workforce at 1 June 2018, United Kingdom, 1–24, https://assets.publishing.service.gov.uk/government/uploads/system/uploads/attachment_data/file/869062/structure-jun2018final-uk-28feb20.pdf (last access: 22 September 2022), 2018b.
DEFRA: Farming Statistics – Final Crop Areas, Yields, Livestock Populations and Agricultural Workforce at June 2019, United Kingdom, 1–22, https://assets.publishing.service.gov.uk/government/uploads/system/uploads/attachment_data/file/865769/structure-jun2019final-uk-22jan20-rev_v2.pdf (last access: 22 September 2022), 2019.
European Commission: Eurostat NUTS Administrative statistical units, https://ec.europa.eu/eurostat/web/gisco/geodata/reference-data/administrative-units-statistical-units/nuts (last access: 22 September 2022), 2010.
Ewert, F., Rodriguez, D., Jamieson, P., Semenov, M. A., Mitchell, R. A. C., Goudriaan, J., Porter, J. R., Kimball, B. A., Pinter, P. J., Manderscheid, R., Weigel, H. J., Fangmeier, A., Fereres, E., and Villalobos, F.: Effects of elevated CO2 and drought on wheat: Testing crop simulation models for different experimental and climatic conditions, Agric. Ecosyst. Environ., 93, 249–266, https://doi.org/10.1016/S0167-8809(01)00352-8, 2002.
FAO: FAOSTAT crops, http://www.fao.org/faostat/en/#data/QC (last access: 22 September 2022), 2018.
Frich, P., Alexander, L. V., Della-Marta, P., Gleason, B., Haylock, M., Tank Klein, A. M. G., and Peterson, T.: Observed coherent changes in climatic extremes during the second half of the twentieth century, Clim. Res., 19, 193–212, https://doi.org/10.3354/cr019193, 2002.
Gagic, V., Kleijn, D., Báldi, A., Boros, G., Jørgensen, H. B., Elek, Z., Garratt, M. P. D., de Groot, G. A., Hedlund, K., Kovács-Hostyánszki, A., Marini, L., Martin, E., Pevere, I., Potts, S. G., Redlich, S., Senapathi, D., Steffan-Dewenter, I., Świtek, S., Smith, H. G., Takács, V., Tryjanowski, P., van der Putten, W. H., van Gils, S., and Bommarco, R.: Combined effects of agrochemicals and ecosystem services on crop yield across Europe, Ecol. Lett., 20, 1427–1436, https://doi.org/10.1111/ele.12850, 2017.
Grassini, P., Eskridge, K. M., and Cassman, K. G.: Distinguishing between yield advances and yield plateaus in historical crop production trends, Nat. Commun., 4, 1–11, https://doi.org/10.1038/ncomms3918, 2013.
Harkness, C., Semenov, M. A., Areal, F., Senapati, N., Trnka, M., Balek, J., and Bishop, J.: Adverse weather conditions for UK wheat production under climate change, Agric. For. Meteorol., 107862, 282–283, https://doi.org/10.1016/j.agrformet.2019.107862, 2020.
Hausfather, Z. and Peters, G. P.: Emissions - the “business as usual” story is misleading, Nature, 577, 618–620, 2020.
Hillocks, R. J.: Farming with fewer pesticides: EU pesticide review and resulting challenges for UK agriculture, Crop Prot., 31, 85–93, https://doi.org/10.1016/j.cropro.2011.08.008, 2012.
Hochman, Z., Gobbett, D. L., and Horan, H.: Climate trends account for stalled wheat yields in Australia since 1990, Glob. Chang. Biol., 23, 2071–2081, https://doi.org/10.1111/gcb.13604, 2017.
Hollis, D., McCarthy, M., Kendon, M., Legg, T., and Simpson, I.: HadUK-Grid – A new UK dataset of gridded climate observations, Geosci. Data J., 6, 151–159, https://doi.org/10.1002/gdj3.78, 2019 (data available at: https://dap.ceda.ac.uk/badc/ukmo-hadobs/data/insitu/MOHC/HadOBS/HadUK-Grid/, last access: 22 September 2022).
Holman, I. P., Hess, T. M., Rey, D., and Knox, J. W.: A Multi-Level Framework for Adaptation to Drought Within Temperate Agriculture, Front. Environ. Sci., 8, 1–14, https://doi.org/10.3389/fenvs.2020.589871, 2021.
Hunt, M. L., Blackburn, G. A., Carrasco, L., Redhead, J. W., and Rowland, C. S.: High resolution wheat yield mapping using Sentinel-2, Remote Sens. Environ., 233, 111410, https://doi.org/10.1016/j.rse.2019.111410, 2019.
Iizumi, T. and Ramankutty, N.: Changes in yield variability of major crops for 1981–2010 explained by climate change, Environ. Res. Lett., 11, 034003, https://doi.org/10.1088/1748-9326/11/3/034003, 2016.
Kahiluoto, H., Kaseva, J., Balek, J., Olesen, J. E., Ruiz-Ramos, M., Gobin, A., Kersebaum, K. C., Takáč, J., Ruget, F., Ferrise, R., Bezak, P., Capellades, G., Dibari, C., Mäkinen, H., Nendel, C., Ventrella, D., Rodríguez, A., Bindi, M., and Trnka, M.: Decline in climate resilience of European wheat, Proc. Natl. Acad. Sci. USA, 116, 123–128, https://doi.org/10.1073/pnas.1804387115, 2019.
Kendon, E., Short, C., Pope, J., Chan, S., Wilkinson, J., Tucker, S., Bett, P., and Harris, G.: Update to UKCP Local (2.2 km) projections, Science Report, Met Office Hadley Centre, Exeter, UK, https://www.metoffice.gov.uk/pub/data/weather/uk/ukcp18/science-reports/ukcp18_local_update_report_2021.pdf (last access: 22 September 2022), 2021.
Kendon, E., Fosser, G., Murphy, J., Chan, S., Clark, R., Harris, G., Lock, A., Lowe, J., Martin, G., Pirret, J., Roberts, N., Sanderson, M., and Tucker, S.: UKCP Convection-permitting model projections, Science report, Met Office Hadley Centre, https://www.metoffice.gov.uk/pub/data/weather/uk/ukcp18/science-reports/UKCP-Convection-permitting-model-projections-report.pdf (last access: 22 September 2022), 2019 (data available at: https://www.metoffice.gov.uk/research/approach/collaboration/ukcp/data/index, last access: 22 September 2022).
Kendon, E. J., Roberts, N. M., Fosser, G., Martin, G. M., Lock, A. P., Murphy, J. M., Senior, C. A., and Tucker, S. O.: Greater future U.K. winter precipitation increase in new convection-permitting scenarios, J. Clim., 33, 7303–7318, https://doi.org/10.1175/JCLI-D-20-0089.1, 2020.
Knight, S., Kightley, S., Bingham, I., Hoad, S., Lang, B., Philpott, H., Stobart, R., Thomas, J., Barnes, A., and Ball, B.: Desk study to evaluate contributory causes of the current “yield plateau” in wheat and oilseed rape, AHDB Project Report No. 502, 2012.
Lesk, C., Coffel, E., and Horton, R.: Net benefits to US soy and maize yields from intensifying hourly rainfall, Nat. Clim. Chang., 10, 819–822, https://doi.org/10.1038/s41558-020-0830-0, 2020.
Lesk, C., Coffel, E., Winter, J., Ray, D., Zscheischler, J., Seneviratne, S. I., and Horton, R.: Stronger temperature–moisture couplings exacerbate the impact of climate warming on global crop yields, Nat. Food, 2, 683–691, https://doi.org/10.1038/s43016-021-00341-6, 2021.
Lobell, D. B., Hammer, G. L., McLean, G., Messina, C., Roberts, M. J., and Schlenker, W.: The critical role of extreme heat for maize production in the United States, Nat. Clim. Chang., 3, 497–501, https://doi.org/10.1038/nclimate1832, 2013.
Miralles, D. G., Gentine, P., Seneviratne, S. I., and Teuling, A. J.: Land–atmospheric feedbacks during droughts and heatwaves: state of the science and current challenges, Ann. N.Y. Acad. Sci., 1436, 19–35, https://doi.org/10.1111/nyas.13912, 2019.
Ortiz-Bobea, A., Ault, T. R., Carrillo, C. M., Chambers, R. G., and Lobell, D. B.: Anthropogenic climate change has slowed global agricultural productivity growth, Nat. Clim. Chang., 11, 306–312, https://doi.org/10.1038/s41558-021-01000-1, 2021.
Rahmstorf, S. and Coumou, D.: Increase of extreme events in a warming world, P. Natl. Acad. Sci. USA, 108, 17905–17909, https://doi.org/10.1073/pnas.1101766108, 2011.
Ray, D. K., West, P. C., Clark, M., Gerber, J. S., Prishchepov, A. V., and Chatterjee, S.: Climate change has likely already affected global food production, PLoS One, 14, 1–18, https://doi.org/10.1371/journal.pone.0217148, 2019.
Reynolds, M. P.: Climate change and crop production, CABI Climate Change Series, CABI, ISBN: 9781845936341, 2010.
Rigden, A. J., Mueller, N. D., Holbrook, N. M., Pillai, N., and Huybers, P.: Combined influence of soil moisture and atmospheric evaporative demand is important for accurately predicting US maize yields, Nat. Food, 1, 127–133, https://doi.org/10.1038/s43016-020-0028-7, 2020.
Rosenzweig, C., Iglesias, A., Yang, X. B., Epstein, P. R., and Chivian, E.: Implications for food production, plant diseases, and pests, Glob. Chang. Hum. Heal., 2, 90–104, https://doi.org/10.1023/A:1015086831467, 2001.
Shortridge, J.: Observed trends in daily rainfall variability result in more severe climate change impacts to agriculture, Clim. Change, 157, 429–444, https://doi.org/10.1007/s10584-019-02555-x, 2019.
Slater, L., Arnal, L., Boucher, M.-A., Chang, A. Y.-Y., Moulds, S., Murphy, C., Nearing, G., Shalev, G., Shen, C., Speight, L., Villarini, G., Wilby, R. L., Wood, A., and Zappa, M.: Hybrid forecasting: using statistics and machine learning to integrate predictions from dynamical models, Hydrol. Earth Syst. Sci. Discuss. [preprint], https://doi.org/10.5194/hess-2022-334, in review, 2022.
Slater, L. J., Anderson, B., Buechel, M., Dadson, S., Han, S., Harrigan, S., Kelder, T., Kowal, K., Lees, T., Matthews, T., Murphy, C., and Wilby, R. L.: Nonstationary weather and water extremes: a review of methods for their detection, attribution, and management, Hydrol. Earth Syst. Sci., 25, 3897–3935, https://doi.org/10.5194/hess-25-3897-2021, 2021.
Sultan, B., Defrance, D., and Iizumi, T.: Evidence of crop production losses in West Africa due to historical global warming in two crop models, Sci. Rep., 9, 1–15, https://doi.org/10.1038/s41598-019-49167-0, 2019.
Swann, A. L. S., Hoffman, F. M., Koven, C. D., and Randerson, J. T.: Plant responses to increasing CO2 reduce estimates of climate impacts on drought severity, P. Natl. Acad. Sci. USA, 113, 10019–10024, https://doi.org/10.1073/pnas.1604581113, 2016.
Thomas, M. R., Cook, R. J., and King, J. E.: Factors affecting development of Septoria tritici in winter wheat and its effect on yield, Plant Pathol., 38, 246–257, https://doi.org/10.1111/j.1365-3059.1989.tb02140.x, 1989.
Tilman, D., Balzer, C., Hill, J., and Befort, B. L.: Global food demand and the sustainable intensification of agriculture, P. Natl. Acad. Sci. USA, 108, 20260–20264, https://doi.org/10.1073/pnas.1116437108, 2011.
Trnka, M., Rötter, R. P., Ruiz-Ramos, M., Kersebaum, K. C., Olesen, J. E., Žalud, Z., and Semenov, M. A.: Adverse weather conditions for European wheat production will become more frequent with climate change, Nat. Clim. Chang., 4, 637–643, https://doi.org/10.1038/nclimate2242, 2014.
Wesseler, J., Bonanno, A., Drabik, D., Materia, V. C., Malaguti, L., Meijer, M., and Venus, T. J.: Overview of the agricultural inputs sector in the EU, European Parliament, Policy Department B: Structural and Cohesion Policies European, Brussels, https://policycommons.net/artifacts/1336081/overview-of-the-agricultural-inputs-sector-in-the-eu/1942999/ (last access: 22 September 2022), 2015.
Williams, K. D., Copsey, D., Blockley, E. W., Bodas-Salcedo, A., Calvert, D., Comer, R., Davis, P., Graham, T., Hewitt, H. T., Hill, R., Hyder, P., Ineson, S., Johns, T. C., Keen, A. B., Lee, R. W., Megann, A., Milton, S. F., Rae, J. G. L., Roberts, M. J., Scaife, A. A., Schiemann, R., Storkey, D., Thorpe, L., Watterson, I. G., Walters, D. N., West, A., Wood, R. A., Woollings, T., and Xavier, P. K.: The Met Office Global Coupled Model 3.0 and 3.1 (GC3.0 and GC3.1) Configurations, J. Adv. Model. Earth Syst., 10, 357–380, https://doi.org/10.1002/2017MS001115, 2018.
Xu, Y.: hyfo: Hydrology and Climate Forecasting, R package version 1.4.2, https://cran.r-project.org/package=hyfo (last access: 22 September 2022), 2020.
Zampieri, M., Ceglar, A., Dentener, F., and Toreti, A.: Wheat yield loss attributable to heat waves, drought and water excess at the global, national and subnational scales, Environ. Res. Lett., 12, 064008, https://doi.org/10.1088/1748-9326/aa723b, 2017.
Zhang, X., Alexander, L., Hegerl, G. C., Jones, P., Tank, A. K., Peterson, T. C., Trewin, B., and Zwiers, F. W.: Indices for monitoring changes in extremes based on daily temperature and precipitation data, WIREs Clim. Change, 2, 851–870, https://doi.org/10.1002/wcc.147, 2011.
Zheng, B., Chenu, K., Fernanda Dreccer, M., and Chapman, S. C.: Breeding for the future: What are the potential impacts of future frost and heat events on sowing and flowering time requirements for Australian bread wheat (Triticum aestivium) varieties?, Glob. Chang. Biol., 18, 2899–2914, https://doi.org/10.1111/j.1365-2486.2012.02724.x, 2012.
Zscheischler, J., Martius, O., Westra, S., Bevacqua, E., Raymond, C., Horton, R. M., van den Hurk, B., AghaKouchak, A., Jézéquel, A., Mahecha, M. D., Maraun, D., Ramos, A. M., Ridder, N. N., Thiery, W., and Vignotto, E.: A typology of compound weather and climate events, Nat. Rev. Earth Environ., 1, 333–347, https://doi.org/10.1038/s43017-020-0060-z, 2020.