the Creative Commons Attribution 4.0 License.
the Creative Commons Attribution 4.0 License.
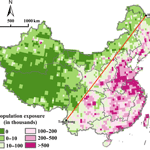
Population exposure to droughts in China under the 1.5 °C global warming target
Tao Pan
Yanhua Liu
Fubao Sun
Quansheng Ge
The Paris Agreement proposes a 1.5 ∘C target to limit the increase in global mean temperature (GMT). Studying the population exposure to droughts under this 1.5 ∘C target will be helpful in guiding new policies that mitigate and adapt to disaster risks under climate change. Based on simulations from the Inter-Sectoral Impact Model Intercomparison Project (ISI-MIP), the Standardized Precipitation Evapotranspiration Index (SPEI) was used to calculate drought frequencies in the reference period (1986–2005) and 1.5 ∘C global warming scenario (2020–2039 in RCP2.6). Then population exposure was evaluated by combining drought frequency with simulated population data from shared socioeconomic pathways (SSPs). In addition, the relative importance of climate and demographic change and the cumulative probability of exposure change were analyzed. Results revealed that population exposure to droughts in the east of China is higher than that in the west; exposure in the middle and lower reaches of the Yangtze River region is the highest, and it is lowest in the Qinghai-Tibet region. An additional 12.89 million people will be exposed to droughts under the 1.5 ∘C global warming scenario relative to the reference period. Demographic change is the primary contributor to exposure (79.95 %) in the 1.5 ∘C global warming scenario, more than climate change (29.93 %) or the interaction effect (−9.88 %). Of the three drought intensities – mild, moderate, and extreme – moderate droughts contribute the most to exposure (63.59 %). Probabilities of increasing or decreasing total drought frequency are roughly equal (49.86 % and 49.66 %, respectively), while the frequency of extreme drought is likely to decrease (71.83 % probability) in the 1.5 ∘C global warming scenario. The study suggested that reaching the 1.5 ∘C target is a potential way for mitigating the impact of climate change on both drought hazard and population exposure.
- Article
(2946 KB) - Full-text XML
-
Supplement
(759 KB) - BibTeX
- EndNote
The goal of the Paris Agreement is to pursue efforts to limit the increase in global mean temperature (GMT) to 1.5 ∘C above preindustrial levels, recognizing that this limit would significantly reduce the risks and impacts of climate change (UNFCCC, 2015). Studies quantifying climate extreme events and their socioeconomic impacts under the 1.5 ∘C target are urgently needed. These types of studies are key content for the IPCC special report on the 1.5 ∘C target, which will be published in 2018. Risk is often represented as the probability of occurrence of hazardous events or trends multiplied by the impacts if these events or trends occur, it results from the interaction of hazard, exposure, and vulnerability (Field et al., 2014). Therefore, exposure assessment is one of the most important aspect of disaster risk assessment. Exposure usually refers to the presence of people, livelihoods, species, or ecosystems; environmental functions, services, and resources; infrastructure; or economic, social, or cultural assets in places and settings that could be adversely affected (Field et al., 2014). As one of the most devastating natural disasters, droughts rank first in terms of globally affected populations (Mishra and Singh, 2010), and the frequency and intensity of droughts are likely to increase with global warming (Stocker et al., 2014; Field et al., 2012). Demographic growth in droughts-prone locations can increase the population exposed, and ultimately lead to increased risk (Forzieri et al., 2017; United Nations, 2013). Droughts have large impacts in China due to typical continental monsoon climate conditions and the large population (Qin et al., 2015). The losses caused by droughts accounted for 19.4 % of all meteorological disasters from 1985 to 2014 (CMA, 2015). Therefore, research on population exposure to droughts in China under the 1.5 ∘C target will be important for understanding future risk.
Several studies of the 1.5 ∘C target have been conducted recently (Donnelly et al., 2017; Henley and King, 2017; Huntingford et al., 2017; Guiot and Cramer, 2016). The objectives have been to evaluate the possible greenhouse gas (GHG) emission pathways to achieve the 1.5 ∘C target (CAT, 2016; Mitchell et al., 2017) or predict changes in extreme climate events under the 1.5 ∘C target (Karmalkar and Bradley, 2017; King et al., 2017; Wang et al., 2017). However, the influence of climate change on socioeconomic aspects, which also needs detailed assessment, has received less attention. The effects of droughts on human populations need to be quantified to identify the locations and intensity of disasters to which people will be exposed under the 1.5 ∘C target. Smirnov et al. (2016) assessed changing population exposure to extreme droughts in RCP8.5 using the Standardized Precipitation Evapotranspiration Index (SPEI); their results indicated that population exposure would increase 426.6 % compared to current conditions. RCP8.5 is a high emission scenario, which is far more than the 1.5 ∘C target, and the study did not account for mild and moderate droughts. Sun et al. (2017) analyzed population exposure to droughts under 1.5 and 2.0 ∘C global warming scenarios in the Haihe River Basin based on SPEI; their results indicated that population exposure under 1.5 ∘C conditions would be reduced 30.4 % relative to 1986–2005. However, population data used in this study was from the sixth national population census of China in 2010, in both reference period and global warming scenarios, ignoring the impact of demographic growth on population exposure change. In addition to climate change, the number, growth, and spatial distribution of population are important contributors to exposure risk, and should be taken into consideration.
In this study, population exposure to droughts under global warming was quantified, the relative importance of different factors, and the uncertainty in exposure change were evaluated. First, SPEI was used to calculate drought frequencies during the reference period and 1.5 ∘C global warming scenario based on simulations from the Inter-Sectoral Impact Model Intercomparison Project (ISI-MIP; Warszawski et al., 2014). Second, modeled population data from shared socioeconomic pathways (SSPs; O'Neill et al., 2014) were used to evaluate the spatial distribution and change in population exposure to droughts in China. Third, the relative importance of climate and demographic change was compared, and the uncertainty in exposure change was assessed using cumulative distribution functions (CDFs). This evaluation of population exposure to droughts in China under the 1.5 ∘C target is expected to provide a basis for adaptation and mitigation strategies.
2.1 Materials
Meteorological data, including precipitation, average maximum temperature, average minimum temperature, average wind speed, average relative humidity, and solar radiation, used in this study were obtained from ISI-MIP (Warszawski et al., 2014), which contains five global climate models (GCMs) simulation results in representative concentration pathways (RCPs). These five GCMs are GFDL-ESM2M, HadGEM2-ES, IPSL-CM5A-LR, MIROC-ESM-CHEM, and NorESM1-M. In this study, we synthesized the results of the five GCMs based on the separately calculated SPEI for each GCM, as combining results of multiple models has been shown to be superior to a single model (Zhou and Yu, 2006). The chosen reference period was 1986–2005, which is a common period to assess climate change effect, and is 0.61 ∘C warmer than preindustrial levels (Stocker et al., 2014). According to previous research (Schleussner et al., 2016; Sun et al., 2017), a stable increase of 1.5 ∘C GMT above the preindustrial level for 20 years will be in 2020–2039 under RCP2.6. As for population data, the United Nations (http://www.un.org, last access: 20 August 2018), World Bank (https://data.worldbank.org.cn, last access: 20 August 2018), and other organizations have proposed projections of population in the future. Considering the RCP scenario we chose in this study, projections of population data in SSP scenarios were used. These are reference pathways describing plausible alternative trends in the evolution of society and ecosystems over a century timescale, in the absence of climate change or climate policies. According to the correspondence between RCPs and SSPs provided by the IPCC, RCP2.6 generally corresponds to SSP1. SSP1 is a sustainable development scenario facing low mitigation and adaption challenges (O'Neill et al., 2014). Therefore, SSP1 was chosen in this study. Population data for SSP1 was obtained from the National Institute for Environmental Studies (NIES), Japan, which was downscaled from the International Institute for Applied Systems Analysis (IIASA) simulated results (Murakami and Yamagata, 2016). Populations in 2000 and 2030 were used to represent the populations in the reference period and 1.5 ∘C global warming scenario, respectively. The spatial resolution of meteorological and population data is given in degree latitude and longitude, i.e., .
2.2 Calculation of SPEI
Combining the characteristics of the Standardized Precipitation Index (SPI; McKee et al., 1993) at multiple scales and the Palmer Drought Severity Index (PDSI; Palmer, 1965), which is sensitive to warming, SPEI was proposed by Vicente-Serrano et al. (2010). The SPEI reflects the change in water deficit using the log-logistic probability distribution function, and obtains the drought index value by standardizing. SPEI is commonly applied as an indication of meteorological droughts and, to a lesser extent, hydrological droughts (Zargar et al., 2011; Hao et al., 2018). SPEI-12 was chosen in this study to reflect long-term trends and interannual changes in droughts. Differences between precipitation (P) and potential evapotranspiration (ET0), which reflect the water surplus or deficit in a region, were calculated to deduce the SPEI by using
The Thornthwaite (1948) equation for ET0 in SPEI only takes temperature into account, ignoring the effects other dynamic factors on droughts. Therefore, the Penman–Monteith equation (Allen et al., 1998) was replaced to calculate ET0 in this study. The Penman–Monteith equation comprehensively considers the impact of both thermal and dynamic factors on ET0, i.e., temperature, wind speed, relative humidity, and solar radiation. Therefore, results are more consistent with true reference crop evapotranspiration. The radiation coefficient used is based on the radiation calibration results in China provided by Yin et al. (2008):
Here, ET0 is the potential evapotranspiration; Rn is the net radiation; G is the soil heat flux density; T is the surface mean daily air temperature; u2 is the wind speed at 2 m height above the ground; es is the saturation vapor pressure; and ea is the actual vapor pressure. The SPEI was calculated by the R SPEI package (https://CRAN.R-project.org/package=SPEI, last access: 20 August 2018). The input data are monthly time series of D (differences between precipitation and potential evapotranspiration), where the set parameters are scale =12, kernel =“rectangular”, distribution = “log-logistic”, and fit = “ub-pwm”. The categorization of drought grade by SPEI and its probability as well as the definition of each grade of drought are shown in Table 1 (Liu and Jiang, 2015).
2.3 Population exposure to drought
Our measure of population exposure is the number of people exposed to mild, moderate, and extreme droughts. That is, the annual average percentage of mild, moderate, and extreme droughts multiplied by the number of people exposed to that outcome (Jones et al., 2015). In this study, population exposures to mild, moderate, and extreme droughts were calculated in the 1.5 ∘C global warming scenario (2020–2039 in RCP2.6) and compared to the results of the reference period (1986–2005). The spatial distribution and change in exposure were analyzed based on the regional separation of China's population into eight major demographic regions (Hu, 1990; Fig. S1 in the Supplement).
2.4 Relative importance and cumulative probability analysis
Population exposure change was decomposed into climate change, demographic change, and interaction effects to evaluate the relative importance using the techniques from a previous study (Jones et al., 2015). The impact of population was calculated by holding climate constant; that is, the frequency of mild, moderate, and extreme droughts in the reference period multiplied by the population in the SSP1 scenario. Similarly, when calculating the impact of climate, the population was held constant; that is, the frequency of mild, moderate, and extreme droughts in the RCP2.6 scenario was multiplied by the population in the reference period. The interaction effect was also evaluated to assess whether the area with continued population growth is experiencing more drought events under climate change.
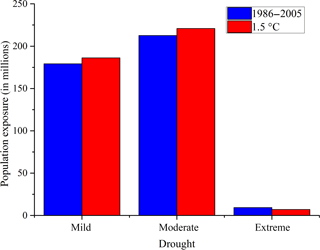
Figure 1Population exposure to mild, moderate, and extreme droughts for the reference period (1986–2005) and 1.5 ∘C global warming scenario (2020–2039 in RCP2.6).
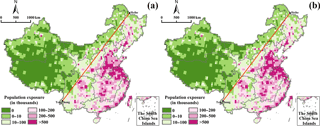
Figure 2Spatial distribution of population exposure to droughts in (a) the reference period (1986–2005) and (b) the 1.5 ∘C global warming scenario (2020–2039 in RCP2.6). (Red line is the Hu line, an imaginary line that diagonally divides the area of China into two parts, stretching from the city of Heihe in Heilongjiang Province to Tengchong in Yunnan Province, which is also called the “geo-demographic demarcation line”.) Please note that the above figure contains disputed territories.
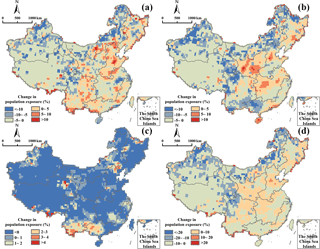
Figure 3Change in population exposure to droughts between the reference period (1986–2005) and the 1.5 ∘C global warming scenario (2020–2039 in RCP2.6), (a) change in exposure to mild droughts, (b) change in exposure to moderate droughts, (c) change in exposure to extreme droughts, and (d) change in exposure to all droughts. Please note that the above figure contains disputed territories.
The CDF of a random variable X is the function representing the probability that the random variable X takes on a value less than or equal to x. The uncertainty in drought frequency and exposure change were analyzed based on CDFs to evaluate the possible impact of climate change. First, the change in frequency and population exposure to mild, moderate, extreme, and all droughts were separately calculated in the 1.5 ∘C global warming scenario relative to the reference scenario. Then, the probability distribution of change was calculated using CDFs.
3.1 Spatial and temporal patterns of drought frequency and population
The frequency of mild, moderate, and extreme droughts, and their relationship with population were calculated for the reference period and the 1.5 ∘C global warming scenario to evaluate the spatial and temporal variation in frequency (Fig. S2) and population (Fig. S3). Generally, mild and moderate droughts will occur more frequently than extreme droughts. The frequency of mild and moderate droughts in most areas is in the range of 5 %–20 %, while the frequency of extreme droughts is less than 5 % in both the reference period and 1.5 ∘C global warming scenario (Fig. S2). As for the spatial pattern of frequency, areas with a high frequency of mild droughts are scattered, while moderate droughts are more spatially concentrated. In the reference period, moderate droughts are concentrated in southern China and the lower reaches of the Yellow River region, i.e., Beijing, Tianjin, Hebei, Henan, and Shandong province. In the 1.5 ∘C global warming scenario, the Shanxi-Shaanxi-Gansu-Ningxia region and Inner Mongolia-Xinjiang region also have more frequent moderate droughts. Extreme droughts occur primarily in inland areas. For example, the Qinghai-Tibet region has the highest frequency of extreme droughts in both scenarios. However, the spatial pattern of extreme droughts changed between the two scenarios. In the 1.5 ∘C global warming scenario, the frequencies in the northeast region, i.e., Heilongjiang, Jilin, and Liaoning provinces; the Shanxi-Shaanxi-Gansu-Ningxia region; and the middle and lower reaches of the Yangtze River region, i.e., Shanghai, Jiangsu, Anhui, Jiangxi Hunan, and Hubei province, decrease. In contrast, in the southwest region, i.e., Sichuan, Chongqing, Guizhou, and Yunnan provinces and the southeast coastal region, i.e., Zhejiang, Fujian, Guangdong, Guangxi, Hainan, Hong Kong, Macao, and Taiwan, the frequency increases relative to the reference period.
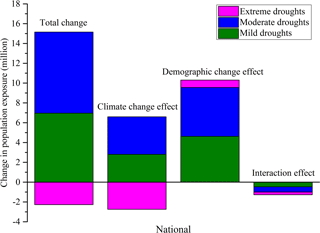
Figure 4Decomposition of population exposure based on different effects – climate change, demographic change, and their interaction, as well as mild, moderate, and extreme droughts.
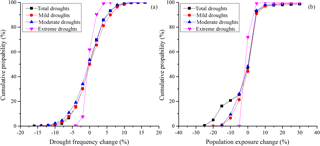
Figure 5Cumulative probability projected change drought frequency (a) and population exposure (b) to mild, moderate, extreme, and total droughts.
The population of China increases by 32.56 million, from 1.26 billion in the reference period to 1.29 billion in the 1.5 ∘C global warming scenario. However, areas of increasing population do not expand in size, and most areas decrease. The variation in demographic change is clear when comparing the two sides of the Hu line (Hu, 1935), which is an imaginary line that diagonally divides the area of China into two parts, stretching from the city of Heihe in Heilongjiang Province to Tengchong in Yunnan Province. It is also called the “geo-demographic demarcation line”; the west of the line occupies 56.2 % of the area of China, but only 5.9 % of the population, while the east of the line occupies 43.8 % of the area, but 94.1 % of the population (Fig. S3). However, the spatial pattern of demographic change in number and percentage is different (Fig. S4). The number of people decreases significantly on the east side of the Hu line, especially in the lower reaches of the Yellow River region and middle and lower reaches of the Yangtze River region, while the decrease in population by percentage is clear on the west side of the Hu line, such as the Inner Mongolia-Xinjiang region and east of the Qinghai-Tibet region. The reason for this dichotomy is the differing demographic distribution on both sides of the Hu line. The population density of the west side is so small that changes in percentage are clearer.
3.2 Spatial distribution and change in population exposure to droughts
The average annual aggregate population exposure in the reference period is 401.02 million and increases to 413.91 million in the 1.5 ∘C global warming scenario. Comparing the population exposure to different droughts in the reference period and the 1.5 ∘C global warming scenario (Fig. 1), the exposure to mild and moderate droughts increases while that to extreme droughts decreases. Moderate droughts account for 53.01 % of total exposure in the reference period and 53.34 % in the 1.5 ∘C global warming scenario, accounting for the most exposure. In comparison, mild droughts rank second and extreme droughts rank third accounting for 2.31 % and 1.69 % in the two scenarios. The spatial pattern of population exposure to droughts is similar to the population demographic distributions in China, i.e., divided by the Hu line. Exposure on the east side is much greater than on the west side (Fig. 2). Exposure in the Yellow River region and middle and lower reaches of the Yangtze River region is the highest, and it is lowest in the Inner Mongolia-Xinjiang region and the Qinghai-Tibet region.
Comparing the changes in exposure to mild (Fig. 3a), moderate (Fig. 3b), extreme (Fig. 3c), and total droughts (Fig. 3d), we found that, except for extreme droughts, they show similar spatial patterns. The exposure in southeast China increases, while that in the northwest part decreases. For mild droughts, exposure increases more clearly in the lower reaches of the Yellow River region, southeast region, and southeast coastal region. For moderate droughts, increases in the northeast region, Shanxi-Shaanxi-Gansu-Ningxia region, and southeast coastal region are apparent. In these regions, the combination of mild and moderate droughts dominates the overall pattern for total exposure. As for extreme droughts, the exposure for most of China decreases, except for the south of the southwest region and west of the southeast coastal region.
3.3 Relative importance analysis
The relative importance of different factors, i.e., climate change, demographic change, and interaction effects, as well as different droughts were analyzed (Fig. 4). For different factors, climate change and demographic change have positive impacts on the total exposure change (29.93 %, 79.95 %), while the interaction effect has a negative impact (−9.88 %). These results imply that the areas experiencing more droughts have decreasing populations in the 1.5 ∘C global warming scenario. For different droughts, the effect of mild and moderate droughts is positive and of similar magnitude, whereas extreme droughts have a lesser effect. Except for the constant climate scenario for analyzing the demographic change effect, the effect of extreme droughts is negative. In total change in exposure, the contributions from mild and moderate droughts are 54.03 % and 63.59 %, respectively, leaving −17.62 % for the effect of extreme droughts. In summary, the demographic change and moderate droughts are the dominant contributors to exposure change in the two scenarios.
3.4 Cumulative probability analysis
Figure 5 shows CDFs for drought frequency and population exposure for changes in the 1.5 ∘C global warming scenario relative to the reference period. For the change in drought frequency (Fig. 5a), extreme droughts are in the minimum range, with changes of −5 % to 5 %, whereas total droughts are in the maximum range, −18 % to 16 %. The cumulative probabilities of an increase in drought frequency under the 1.5 ∘C global warming scenario for mild, moderate, extreme, and total droughts are 50.14 %, 46.48 %, 38.23 %, and 49.86 %, respectively. Apart from extreme droughts, which show a clear downward trend, the probabilities of an increase or decrease in mild, moderate, and total droughts are roughly equal. In terms of change in population exposure (Fig. 5b), extreme droughts show a minimum, at −5 % to 5 %, and total droughts show a maximum, −25 % to 25 %, probability. Extreme droughts decrease, with a cumulative probability of 71.83 %, while mild, moderate, and total droughts increase, with cumulative probabilities of 55.17 %, 51.71 %, and 53.01 %, respectively. The probability of an increase in mild droughts is the highest, while the probability of an increase in extreme droughts is the lowest in both drought frequency and exposure under the 1.5 ∘C global warming scenario.
Extensive studies have focused on changes in extreme climate events under global climate change (Hirabayashi et al., 2013; Huang et al., 2017; Kharin et al., 2013). Currently, with the 1.5 ∘C target, the socioeconomic impacts of 1.5 ∘C global warming on factors, such as population exposed to disasters, need to be further studied. In this study, the population exposure to droughts was calculated for the 1.5 ∘C global warming scenario and reference period by combining drought frequency and population simulations. The relative importance of different factors as well as evaluating the cumulative probability of exposure change were analyzed.
Our results indicate that average annual population exposure to droughts in the 1.5 ∘C global warming scenario would increase by 12.89 million compared to the reference period, roughly 0.94 % of the projected Chinese population under the SSP1 scenario in 2030. The increase in exposure is rather unremarkable, suggesting that achieving the 1.5 ∘C target may limit the potential damage incurred by climate change. Among the three different droughts, exposure to moderate droughts will be the largest because the areas with a high frequency of moderate droughts coincide with high population density. Drought frequency and population are two important factors that contribute to exposure. To determine which one has a larger impact, the relative importance of these two factors, and the interaction effect, were analyzed. Results revealed that exposure change is mainly due to demographic change (79.95 %), and climate change is responsible for 29.93 % of the change; the interaction effects explain the remaining −9.88 %. These results are different from previous studies applying contribution analysis. Jones et al. (2015) calculated future population exposure to US heat extremes under the Special Report on Emission Scenarios (SRES) A2 scenario; they found that the growth in exposure is mainly due to climate change. Smirnov et al. (2016) analyzed the relative importance of climate change and demographic growth for exposure to future extreme droughts in RCP4.5 and RCP8.5, and their results indicated that climate change is more responsible for exposure change than demographic change in both scenarios. The contradiction may be due to the different scenarios used in the studies. SRES A2, RCP4.5, and RCP8.5 are scenarios with higher GHG emissions relative to RCP2.6, which corresponds to the 1.5 ∘C target used in our study. This 1.5 ∘C target is an important constraint because it is relevant to the requirements for large GHG emission reductions. The difference of GHG emissions also explains the cumulative probability analysis result for drought frequency. Drought frequency is not likely to increase in the 1.5 ∘C global warming scenario compared to the reference period. Therefore, the effect of climate change to exposure is reduced compared to higher emission pathways, which results in demographic change acting as the primary contributor to exposure. In future studies, we would like to evaluate population exposure for high GHG emission pathways, i.e., RCP4.5/SSP2 and RCP8.5/SSP3, and compare with the results from RCP2.6/SSP1 to illustrate the impacts of achieving the 1.5 ∘C target. Furthermore, studies accounting for more demographic characteristics in addition to growth, i.e., age, sex, education, and income would be carried out, which are likely to be stronger factors for demographic change in the 1.5 ∘C target. However, we currently lack the required sophisticated data.
There are many kinds of droughts: meteorological, agricultural, hydrological, and socioeconomic. In this study, based on simulated climate data, we assessed population exposure to meteorological droughts under the 1.5 ∘C global warming target using the SPEI; however, the results do not necessarily coincide with agricultural, hydrological, or socioeconomic droughts. Therefore, we would like to assess population exposure to different kinds of droughts to determine their impacts on populations. In addition, there are some uncertainties in estimating population exposure under climate change. The main sources include GHG emission scenarios (Maurer, 2007), GCMs (Kirono et al., 2011), calculating potential evapotranspiration, population prediction, and selection of the drought index (Burke and Brown, 2008). For instance, SPEI was chosen in this study because it combines the characteristics of SPI and PDSI; however, it is limited by providing a measure of dryness in a relative rather than absolute sense. Selecting different drought indexes may lead to differences in drought hazard and population exposure results. Therefore, future studies could evaluate different drought indexes based on more advanced and higher resolution GCMs and RCMs (regional climate models), determine importance of sources of uncertainty, and generate assessment results that are more accurate and reasonable.
This study leads to four key findings. First, population exposure to droughts on the east side of the Hu line is higher than that on the west side, which corresponds to general demographic distributions in China. Among the eight demographic regions, exposure in the middle and lower reaches of the Yangtze River region is the highest, and the lowest occurs in the Qinghai-Tibet region. Second, in the 1.5 ∘C global warming scenario, population exposure to droughts has a slight increase, 12.89 million more residents exposed, relative to the reference period. Third, variations in both population and climate are important factors in this change in exposure, but demographic change is the primary contributor (79.95 %) in the 1.5 ∘C global warming scenario. Moderate droughts contribute most among three droughts (63.59 %). Fourth, probabilities of increasing or decreasing total drought frequency are approximately equal (49.86 % and 49.66%, respectively), while the frequency of extreme drought is likely to decrease (71.83 % probability) in the 1.5 ∘C global warming scenario. To conclude, in the 1.5 ∘C global warming scenario, the contribution of climate change is significantly less than demographic change and drought frequency will not increase distinctly compared to the reference period, which indicates that reaching the 1.5 ∘C target is a potential mechanism for mitigating the impact of climate change on both droughts and population exposure. In addition, demographic change should be regarded as a significant component to control the growth in exposure to droughts.
Data supporting this study are available at the following location: ISI-MIP data archive (Warszawski et al., 2014), https://www.isimip.org/ (last access: 29 August 2018). Global dataset of gridded population and GDP scenarios (Murakami and Yamagata, 2016), http://www.cger.nies.go.jp/gcp/population-and-gdp.html (last access: 29 August 2018).
The supplement related to this article is available online at: https://doi.org/10.5194/esd-9-1097-2018-supplement.
JC and YL conceived the study. JC performed all analysis and wrote the initial manuscript with support from YL and TP. All authors contributed to interpreting results, discussion, and improvement of the manuscript.
The authors declare that they have no conflict of interest.
This article is part of the special issue “The Earth system at a global warming of 1.5 ∘C and 2.0 ∘C”. It is not associated with a conference.
This study was supported by the National Key Research and Development
Program of China (grant no. 2016YFA0602402); the National Natural Science
Foundation of China (grant nos. 41671037, 41301091); the Key Research Program
of Frontier Sciences, CAS (QYZDB-SSW-DQC005); and the Youth Innovation
Promotion Association, CAS (grant no. 2016049). We also thank ISI-MIP and
NIES for data support.
Edited by: Axel Kleidon
Reviewed by: four anonymous referees
Allen, R. G., Pereira, L. S., Raes, D., and Smith, M.: Crop evapotranspiration -guidelines for computing crop requirements, FAO Irrigation and drainage paper 56, Food and Agriculture Organization of the United Nations, Rome, Italy, 1998.
Burke, E. J. and Brown, S. J.: Evaluating Uncertainties in the Projection of Future Drought, J. Hydrometeorol., 9, 292–299, 2008.
China Meteorological Administration (CMA): China meteorological Disaster Yearbook, Meteorological Press of China, Beijing, 2015.
Climate Action Tracker (CAT): The Ten Most Important Short Term Steps to Limit Warming to 1.5 ∘C, available at: http://climateactiontracker.org/news/268/The-ten-most-important-short-term-steps-to-limit-warming-to-1.5C.html (last access: 20 August 2018), 2016.
Donnelly, C., Greuell, W., Andersson, J., Gerten, D., Pisacane, G., Roudier, P., and Ludwig, F.: Erratum to: Impacts of climate change on European hydrology at 1.5, 2 and 3 degrees mean global warming above preindustrial level, Climatic Change, 143, 535–535, 2017.
Field, C. B., Barros, V., and Stocker, T. F.: Managing the risks of extreme events and disasters to advance climate change adaptation, Special report of the Intergovernmental Panel on Climate Change (IPCC), J. Clin. Endocr. Metab., 18, 586–599, 2012.
Field, C. B., Barros, V. R., Mach, K., and Mastrandrea, M.: Climate change 2014: impacts, adaptation, and vulnerability, Contribution of Working Group II to the Third Assessment Report, 19, 81–111, 2014.
Forzieri, G., Cescatti, A., Silva, F. B. E., and Feyen, L.: Increasing risk over time of weather-related hazards to the European population: a data-driven prognostic study, Lancet Planet Health, 1, e200–e208, 2017.
Guiot, J. and Cramer, W.: Climate change: The 2015 Paris Agreement thresholds and Mediterranean basin ecosystems, Science, 354, 465–468, 2016.
Hao, Z., Singh, V. P., and Xia, Y.: Seasonal drought prediction: advances, challenges, and future prospects, Rev. Geophys., 56, 1–34, 2018.
Hirabayashi, Y., Mahendran, R., Koirala, S., Konoshima, L., Dai, Y., Watanabe, S., Kim, H., and Kanae, S.: Global flood risk under climate change, Nat. Clim. Change, 3, 816–821, 2013.
Henley, B. J. and King, A. D.: Trajectories toward the 1.5 ∘C Paris target: Modulation by the Interdecadal Pacific Oscillation, Geophys. Res. Lett., 44, 4256–4262, 2017.
Hu, H. Y.: The distribution of China's Population, Acta Geol. Sin., 2, 33–74, 1935.
Hu, H. Y.: The distribution, regionalization and prospect of China's Population, Acta Geol. Sin., 2, 13–19, 1990.
Huang, J., Zhai, J., Jiang, T., Wang, Y., Li, X., Wang, R., Xiong, M., Su, B., and Fischer, T.: Analysis of future drought characteristics in China using the regional climate model CCLM, Clim. Dynam., 50, 1–19, 2017.
Huntingford, C., Yang, H., Harper, A., Cox, P. M., Gedney, N., Burke, E. J., Lowe, J. A., Hayman, G., Collins, W. J., Smith, S. M., and Comyn-Platt, E.: Flexible parameter-sparse global temperature time profiles that stabilise at 1.5 and 2.0∘ C, Earth Syst. Dynam., 8, 617–626, https://doi.org/10.5194/esd-8-617-2017, 2017.
Jones, B., O'Neill, B. C., Mcdaniel, L., Mcginnis, S., Mearns, L. O., and Tebaldi, C.: Future population exposure to US heat extremes, Nat. Clim. Change, 5, 592–597, 2015.
Karmalkar, A. V. and Bradley, R. S.: Consequences of Global Warming of 1.5 ∘C and 2 ∘C for Regional Temperature and Precipitation Changes in the Contiguous United States, Plos One, 12, e0168697, https://doi.org/10.1371/journal.pone.0168697, 2017.
Kharin, V. V., Zwiers, F. W., Zhang, X., and Wehner, M.: Changes in temperature and precipitation extremes in the CMIP5 ensemble, Climatic Change, 119, 345–357, 2013.
King, A. D., Karoly, D. J., and Henley, B. J.: Australian climate extremes at 1.5 ∘C and 2 ∘C of global warming, Nat. Clim. Change, 7, 412–416, 2017.
Kirono, D. G. C., Kent, D. M., Hennessy, K. J., and Mpelasoka, F.: Characteristics of Australian droughts under enhanced greenhouse conditions: Results from 14 global climate models, J. Arid Environ., 75, 566–575, 2011.
Liu, K. and Jiang, D.: Analysis of dryness/wetness over China using standardized precipitation evapotranspiration index based on two evapotranspiration algorithms, Chinese J. Atmos. Sci., 39, 23–36, 2015.
Maurer, E. P.: Uncertainty in hydrologic impacts of climate change in the Sierra Nevada, California, under two emissions scenarios, Climatic Change, 82, 309–325, 2007.
Mckee, T. B., Doesken, N. J., and Kleist, J.: The relationship of drought frequency and duration to time scales, in: Proceedings of the 8th Conference on Applied Climatology, American Meteorological Society, 17, 179–183, Boston, MA, 1993.
Mishra, A. K. and Singh, V. P.: A review of drought concepts, J. Hydrol., 391, 202–216, 2010.
Mitchell, D., AchutaRao, K., Allen, M., Bethke, I., Beyerle, U., Ciavarella, A., Forster, P. M., Fuglestvedt, J., Gillett, N., Haustein, K., Ingram, W., Iversen, T., Kharin, V., Klingaman, N., Massey, N., Fischer, E., Schleussner, C.-F., Scinocca, J., Seland, Ø., Shiogama, H., Shuckburgh, E., Sparrow, S., Stone, D., Uhe, P., Wallom, D., Wehner, M., and Zaaboul, R.: Half a degree additional warming, prognosis and projected impacts (HAPPI): background and experimental design, Geosci. Model Dev., 10, 571–583, https://doi.org/10.5194/gmd-10-571-2017, 2017.
Murakami, D. and Yamagata, Y.: Estimation of gridded population and GDP scenarios with spatially explicit statistical downscaling, available at: https://arxiv.org/abs/1610.09041 (last access: 24 August 2018), 2016.
O'Neill, B. C., Kriegler, E., Riahi, K., Ebi, K. L., Hallegatte, S., Carter, T. R., Mathur, R., and Vuuren, D. P. V.: A new scenario framework for climate change research: the concept of shared socioeconomic pathways, Climatic Change, 122, 401–414, 2014.
Palmer, W.: Meteorological drought, U.S. Department of Commerce Weather Bureau Research Paper, Washington D.C, USA, 1965.
Qin, D., Editor, C., and Edi, D. C.: China national assessment report on risk management and adaptation of climate extremes and disasters, Science Press, Beijing, China, 2015.
Schleussner, C.-F., Lissner, T. K., Fischer, E. M., Wohland, J., Perrette, M., Golly, A., Rogelj, J., Childers, K., Schewe, J., Frieler, K., Mengel, M., Hare, W., and Schaeffer, M.: Differential climate impacts for policy-relevant limits to global warming: the case of 1.5 ∘C and 2 ∘C, Earth Syst. Dynam., 7, 327–351, https://doi.org/10.5194/esd-7-327-2016, 2016.
Smirnov, O., Zhang, M., Xiao, T., Orbell, J., Lobben, A., and Gordon, J.: The relative importance of climate change and population growth for exposure to future extreme droughts, Climatic Change, 138, 1–13, 2016.
Stocker, T. (Ed.): Climate change 2013: the physical science basis: Working Group I contribution to the Fifth assessment report of the Intergovernmental Panel on Climate Change, Cambridge University Press, Cambridge, United Kingdom and New York, USA, 2014.
Sun, H., Wang, Y., Chen, J., Zhai, J., Jing, C., Zeng, X., Ju, H., Zhao, N., Zhan, M., and Luo, L.: Exposure of population to droughts in the Haihe River Basin under global warming of 1.5 and 2.0 ∘C scenarios, Quatern. Int., 453, 74–84, 2017.
Thornthwaite, C. W.: An approach toward a rational classification of climate, Geogr. Rev., 38, 55–94, 1948.
UNFCCC Conference of the Parties (COP): Adoption of the Paris Agreement, Paris, France, 2015.
United Nations, D. O. E. A. S. A.: Population Division World Population Prospects: The 2012 Revision, available at: http://esa.un.org/wpp/Excel-Data/population.htm (last access: 30 October 2017), 2013.
Vicente-Serrano, S. M., Beguería, S., and Lópezmoreno, J. I.: A multiscalar drought index sensitive to global warming: the standardized precipitation evapotranspiration index, J. Climate, 23, 1696–1718, 2010.
Wang, G., Cai, W., Gan, B., Wu, L., Santoso, A., Lin, X., Chen, Z., and Mcphaden, M. J.: Continued increase of extreme El Niño frequency long after 1.5 ∘C warming stabilization, Nat. Clim. Change, 7, 568–574, 2017.
Warszawski, L., Frieler, K., Huber, V., Piontek, F., Serdeczny, O., and Schewe, J.: The Inter-Sectoral Impact Model Intercomparison Project (ISI-MIP): project framework, P. Natl. Acad. Sci. USA, 111, 3228–3232, https://doi.org/10.1073/pnas.1312330110, 2014.
Yin, Y., Wu, S., Du, Z., and Yang, Q.: Radiation calibration of FAO56 Penman-Monteith model to estimate reference crop evapotranspiration in China, Agr. Water Manage., 95, 77–84, 2008.
Zargar, A., Sadiq, R., Naser, B., and Khan, F. I.: A review of drought indices, Environ. Rev., 19, 333–349, 2011.
Zhou, T. and Yu, R.: Twentieth-Century Surface Air Temperature over China and the Globe Simulated by Coupled Climate Models, J. Climate, 19, 5843–5858, 2006.