the Creative Commons Attribution 4.0 License.
the Creative Commons Attribution 4.0 License.
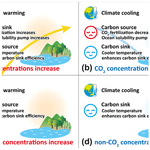
Carbon cycle and climate feedbacks under CO2 and non-CO2 overshoot pathways
Irina Melnikova
Philippe Ciais
Katsumasa Tanaka
Hideo Shiogama
Kaoru Tachiiri
Tokuta Yokohata
Olivier Boucher
Reducing emissions of non-carbon dioxide (CO2) greenhouse gases (GHGs), such as methane (CH4) and nitrous oxide (N2O), complements CO2 mitigation in limiting global warming. However, estimating carbon–climate feedback for these gases remains fraught with uncertainties, especially under overshoot scenarios. This study investigates the impact of CO2 and non-CO2 gases with nearly equal levels of effective radiative forcing on the climate and carbon cycle, using the Earth system model (ESM) IPSL-CM6A-LR. We first present a method to recalibrate methane and nitrous oxide concentrations to align with published radiative forcings, ensuring accurate model performance. Next, we carry out a series of idealised ramp-up and ramp-down concentration-driven experiments and show that, while the impacts of increasing and decreasing CO2 and non-CO2 gases on the surface climate are nearly equivalent (when their radiative forcing magnitudes are set to be the same), regional differences emerge. We further explore the carbon cycle feedbacks and demonstrate that they differ under CO2 and non-CO2 forcing. CO2 forcing leads to both carbon–climate and carbon–concentration feedbacks, whereas non-CO2 gases give rise to the carbon–climate feedback only. We introduce a framework, building on previous studies that addressed CO2 forcing, to separate the carbon–climate feedback into a temperature term and a temperature–CO2 cross-term. Our findings reveal that these feedback terms are comparable in magnitude for the global ocean. This underscores the importance of considering both terms in carbon cycle feedback framework and climate change mitigation strategies.
- Article
(12243 KB) - Full-text XML
-
Supplement
(6287 KB) - BibTeX
- EndNote
Increases in the atmospheric concentrations of carbon dioxide (CO2), methane (CH4), and nitrous oxide (N2O) have predominantly caused human-induced climate change since the preindustrial period. They contributed nearly 63 %, 11 %, and 6 %, respectively, to the total effective radiative forcing (ERF) over the 1960–2019 period (Canadell et al., 2021). Anthropogenic CO2 emissions are dominated by the combustion of fossil fuels (FFs) and land-use change (LUC), CH4 emissions by FF and the agricultural sector, and N2O emissions by the use of nitrogen fertiliser and manure. CH4 and N2O have atmospheric lifetimes of 11.8 ± 1.8 years and 109 ± 10 years and 100-year global warming potentials (GWP100) of 27.9 and 273, respectively (Forster et al., 2021; Myhre et al., 2013).
Mitigation of non-CO2 greenhouse gases (GHGs) is an essential strategy to limit global warming in the context of the Paris Agreement's temperature target (Abernethy et al., 2021; Jones et al., 2018; Rao and Riahi, 2006; de Richter et al., 2017; Tanaka et al., 2021). The reduction in CH4 emissions can lead to a rapid decrease in the radiative forcing and may limit the peak warming (Mengis and Matthews, 2020; Montzka et al., 2011). To facilitate the achievement of the Paris Agreement temperature target, the Global Methane Pledge was adopted to reduce anthropogenic CH4 emissions by 30 % over the 2020–2030 period (CCAC, 2021). Several existing studies have confirmed the technical and socioeconomic capacities to reduce the global methane emissions and benefits for reducing atmospheric pollution (Höglund-Isaksson et al., 2020; Jackson et al., 2021; Malley et al., 2023; Nisbet et al., 2020). Atmospheric methane removal methods have also been discussed in some studies (Boucher and Folberth, 2010; Jackson et al., 2021; Mundra and Lockley, 2023), but they are still in their infancy.
Numerous studies have investigated the impacts of changes in the emissions/concentrations of CO2 and non-CO2 GHGs on the Earth system. The Precipitation Driver and Response Model Intercomparison Project (PDRMIP) focused on the role of different climate change drivers on the mean and extreme precipitation changes using a set of idealised perturbed experiments (Myhre et al., 2017). Richardson et al. (2019) revealed spatial and temporal differences in the surface temperature response to different forcings, such as CO2 and CH4, in part due to the physiological CO2 warming over the densely vegetated regions that is absent under non-CO2 forcing. This physiological warming occurs because plants close their stomata under elevated CO2, reducing transpiration and decreasing latent heat loss, which causes a local surface warming. Nordling et al. (2021) further demonstrated that the change in long-wave clear-sky emissivity is the key driver of the differences in temperature response between GHG forcings. Using intermediate-complexity Earth system climate model simulations, Nzotungicimpaye et al. (2023) showed that delaying methane mitigation has implications both for meeting the stringent temperature targets and for the climate over many centuries. Using Earth system model (ESM) simulations, Tokarska et al. (2018) showed that non-CO2 forcing reduces the remaining carbon budget due to its direct radiative effect on surface temperature, causing additional warming. Fu et al. (2020) showed that non-CO2 GHGs, which have a shorter atmospheric lifetime than CO2, may have long-term consequences on climate through their impacts on the carbon cycle, namely, warming from non-CO2 GHGs weakening land and ocean carbon sinks.
The weakening of land and ocean carbon sinks due to non-CO2 GHGs underscores the importance of understanding the differences in carbon cycle feedbacks between CO2 and non-CO2 GHGs. Only the changes in CO2 concentrations give rise to the carbon–concentration feedback, which is the response of the land and ocean carbon uptake to the changes in CO2 concentration, mainly via the stimulation of photosynthesis by the CO2 fertilisation effect on land and the solubility pump in the ocean. The changes in both CO2 and non-CO2 concentrations lead to the carbon–climate feedback (γ), that is, the response of the land and ocean carbon uptake to climate change, mainly via the increased plant and soil respiration over land and reduction in the CO2 solubility in the ocean with warming (Arora et al., 2013; Schwinger et al., 2014; Zickfeld et al., 2011). Under changing CO2 concentrations, land and ocean carbon storages respond to both carbon–concentration and carbon–climate feedbacks. However, the interaction between these feedbacks can introduce a nonlinearity into the system, whereby the combined effect is not simply the sum of the individual feedbacks (Schwinger et al., 2014; Zickfeld et al., 2011). Thus, temperature-mediated feedback can differ under changing versus constant CO2 levels, an important distinction when comparing CO2 and non-CO2 GHG feedback mechanisms. Here, it is also important to recognise that other factors, such as time lags and potential irreversibilities in the climate system, may also contribute to these differences (Boucher et al., 2012; Chimuka et al., 2023; Schwinger et al., 2014).
Previous studies investigated the nonlinearities in the carbon cycle feedback, showing that the cross-term – arising from interactions between changing atmospheric CO2 and temperatures – can be comparable in size with γ (Schwinger et al., 2014; Zickfeld et al., 2011). They attributed the nonlinearity to the different responses of the land biosphere to the temperature changes, depending on the presence or absence of the CO2 fertilisation effect, and to the weakening of ocean circulation and mixing between water masses of different temperatures. However, these studies did not consider non-CO2 GHGs.
Previous studies have also examined the impact of declining atmospheric CO2 concentration on the climate and carbon cycle (Boucher et al., 2012; Chimuka et al., 2023; Jones et al., 2016; Koven et al., 2023; Melnikova et al., 2021; Schwinger and Tjiputra, 2018). During the period of decreasing atmospheric CO2 concentration and temperature (ramp-down), the β and γ feedbacks arise from both the reduction in CO2 levels and temperature and the inertia of the carbon cycle – specifically, the altered land and ocean carbon pools resulting from prior increases in the CO2 concentration and temperature (Chimuka et al., 2023; Zickfeld et al., 2016). Melnikova et al. (2021) showed that this leads to an amplification of the β and γ feedbacks under decreasing CO2 concentration and temperature. The effectiveness of non-CO2 mitigation has been explored and is an integral part of the integrated assessment models (Ou et al., 2021; Rao and Riahi, 2006; Tanaka et al., 2021). However, few studies have investigated the effects of declining non-CO2 GHG concentrations on the climate and carbon cycle using Earth system models (ESMs). Abernethy et al. (2021) used an ESM to demonstrate the effectiveness of methane removal in reducing global mean surface temperature, complementing negative CO2 emissions. Thus, the purpose of this study is 2-fold:
-
to clarify whether the climate and carbon cycle responses to declining CO2 and non-CO2 GHGs differ globally and regionally,
-
to investigate the nonlinearities of carbon cycle feedbacks under CO2 and non-CO2 GHG decrease and the implications for climate change mitigation.
Here, we conduct a series of idealised CO2 and non-CO2 (CH4 and N2O) concentration-driven ramp-up and ramp-down experiments using the IPSL-CM6A-LR ESM. We then compare the global and spatial impacts of CO2 and non-CO2 concentration changes on climate and the carbon cycle under overshoot pathways.
2.1 Recalibration of model's CH4 and N2O concentrations
We use Version 6 of the Institut Pierre-Simon Laplace (IPSL) low-resolution ESM, IPSL-CM6A-LR (Boucher et al., 2020), developed in the runup to the sixth phase of the Coupled Model Intercomparison Project (CMIP). It comprises the LMDZ atmospheric model Version 6A and the ORCHIDEE land surface model Version 2.0 with a 144×143 spatial resolution and the oceanic model NEMO Version 3 with a resolution of 1°.
Previous studies showed that IPSL-CM6A-LR can adequately estimate the ERF of CO2 (Lurton et al., 2020). However, it underestimates the CH4 radiative forcing for the historical period due to known limitations in the parameterisation of gaseous optical properties in the Rapid Radiative Transfer Model (see Fig. 8 in Hogan and Matricardi, 2020). CH4 also absorbs in the short-wave spectrum, an effect that is not accounted for in the radiative transfer code used in IPSL-CM6A-LR and many other climate models. The Intergovernmental Panel on Climate Change (IPCC) Sixth Assessment Report (AR6) (Forster et al., 2021) estimated the CH4 ERF to be 0.54 (0.43 to 0.65) W m−2 for the 1750–2019 period. However, the estimated ERF of CH4 at the top of the atmosphere (TOA) in IPSL-CM6A-LR is only 0.27 W m−2 for the 1850–2014 period. Note that, in the above estimates, the ERF is defined as the difference in the net TOA flux between a model experiment with perturbed GHG concentration but fixed sea surface and ice temperatures and a control simulation with preindustrial GHG concentrations. Thus, the estimates include (minimal) effects on the ERF from changes in land surface temperature because, unlike sea surface temperature (SST), the land surface temperature is not prescribed (see Thornhill et al., 2021). Likewise, the ERF of N2O may not be accurate in the model. This problem may not be specific to IPSL-CM6A-LR: other climate models (e.g. CNRM-CM6) share the same radiative transfer code, and most radiative transfer models used in some climate models have some degree of inaccuracy because they are designed to be computationally efficient (Collins et al., 2006; Fyfe et al., 2021; Pincus et al., 2016). Thus, there is a need to represent the ERF of CH4 and N2O more accurately in order to better understand the effects of non-CO2 GHG mitigation on the Earth system. As developing better parameterisations of the gaseous optical properties is beyond the scope of this study, we have developed an approach that adjusts CH4 and N2O concentrations to “effective” concentrations that generate CH4 and N2O ERFs consistent with the reference estimates of IPCC AR6 (see Appendix A). The effective concentrations of CH4 and N2O are used as input to the radiative transfer scheme of the climate model throughout the rest of this study. In the text and figures, these are presented as the actual (equivalent) concentrations.
2.2 Experiment design
We perform and analyse a series of idealised global mean CO2 and non-CO2 concentration-driven ensemble experiments as summarised in Table 1 and Fig. 1. Including 50-year ramp-up, ramp-down, and stabilisation periods allows the exploration of responses to increasing or decreasing CO2 and non-CO2 (CH4 and N2O) concentrations and of the long-term consequences and reversibility of their impacts on the climate and carbon cycle. The inclusion of CO2 and non-CO2 concentration-driven experiments ([CO2] and [nonCO2] with comparable ERF levels), a combined CO2 and non-CO2 concentration-driven experiment [CO2+ nonCO2], a biogeochemically coupled (BGC) experiment where CO2 forcing affects only the carbon cycle of land and ocean [CO2bgc] (with minor temperature effects from CO2 physiological forcing), and a radiatively coupled (RAD) experiment that includes only CO2 radiative forcing [CO2rad] (where CO2 change does not affect the carbon cycle) enables us to explore the impacts of different forcing components on the climate and carbon cycle and potential nonlinearities of feedbacks. Additionally, an experiment that combines nonCO2 radiative forcing with CO2 physiological forcing [CO2bgc + nonCO2] allows the comparison of nonlinearities arising from combined carbon–concentration feedback and CO2- and non-CO2-driven carbon–climate feedback. It serves as the non-CO2 counterpart of the [CO2] experiment.
Table 1Description of experiments. Note that all experiments are analysed relative to their [piControl] counterparts.
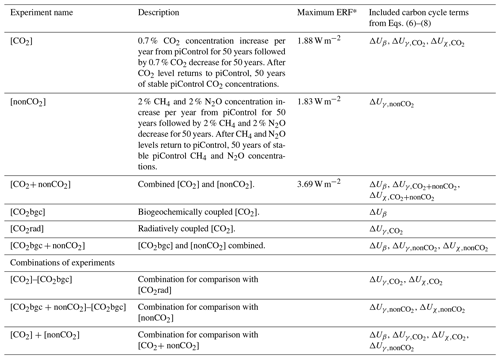
* According to equations by Etminan et al. (2016), warming from the physiological CO2 forcing is assumed to be negligible.
The experiment design uses a fixed land cover and constant (other than CO2, CH4, and N2O) GHG and aerosol forcings that might otherwise interfere with the interpretation of the results. The maximum ERF in our experiments is 3.69 W m−2, as estimated from the equations by Etminan et al. (2016) (see also Appendix A), corresponding to an (actual) CO2 concentration of 403 ppm, a CH4 concentration of 2175 ppb, and an N2O concentration of 735 ppb. This ERF level (very much in line with the current CO2 concentration level of ca. 420 ppm) makes our experiments and results relevant to mitigation efforts in the near future. The small differences in ERF between the [CO2] and [nonCO2] experiments are not significant when considering ramp-up, ramp-down, and full periods at p<0.05.
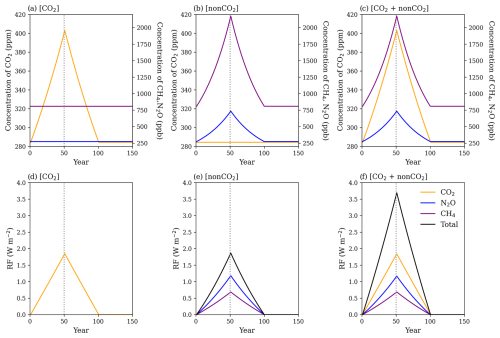
Figure 1Time series of input (a)–(c) CO2, CH4, and N2O concentrations and (d)–(f) their respective radiative ERFs according to the equations of Etminan et al. (2016) for (a, d) [CO2], (b, e) [nonCO2], and (c, f) [CO2+ nonCO2] experiments. Note the different scales on the y axes in panels (a)–(c) for CO2 concentrations in ppm (left) and other GHG concentrations in ppb (right).
We investigate CO2 and non-CO2 impacts on the climate by looking at the differences between [CO2] and [nonCO2] experiments and [CO2rad] and [nonCO2] experiments, hereafter referred to as [CO2]–[nonCO2] and [CO2rad]–[nonCO2], respectively. The experiments manipulate CH4 and N2O concentrations simultaneously because our primary focus is to compare the effects of CO2 with those of non-CO2 gases (i.e. CH4 and N2O combined) in this study.
For each experiment, three ensemble members are branched from the years 1870, 2020, and 2170 of the CMIP6 piControl experiment, hereafter [piControl]. We note that the use of three members is not ideal, but it is a common compromise between computational cost and sampling the uncertainty due to climate variability. We estimate the changes relative to the corresponding [piControl] periods in order to avoid the effects of low-frequency internal climate variability from the piControl (Fig. S1 in the Supplement), as discussed in Bonnet et al. (2021). When reporting carbon sink/source in the following sections, we refer to the fluxes relative to the [piControl]. For diagnosing Atlantic Meridional Overturning Circulation (AMOC), we utilised the ocean overturning mass streamfunction in depth space (msftyz variable in CMIP6). Specifically, we calculated the maximum annual mean value of the streamfunction in the Atlantic basin north of 20° N through all of the model's depth layers (up to ca. 5800 m).
2.3 Carbon cycle feedback attribution
Traditionally, carbon cycle feedback analysis relies on fully coupled [CO2], biogeochemically coupled [CO2bgc], and radiatively coupled [CO2rad] simulations (Arora et al., 2013, 2020; Friedlingstein et al., 2006; Gregory et al., 2009; Schwinger et al., 2014; Schwinger and Tjiputra, 2018; Williams et al., 2019; Zickfeld et al., 2011). The carbon uptake (ΔU) can then be derived using the well-established carbon cycle feedback framework as a sum of the carbon–concentration β parameter (GtC ppm−1) multiplied by the changes in the atmospheric CO2 concentration (ppm) and the carbon–climate γ feedback parameter (GtC K−1) multiplied by the changes in surface temperature ΔT (K), using Eq. (1):
Here, the term ε refers to a residual term.
The β parameter can be estimated from the [CO2bgc]–[piControl], using Eq. (2):
where ΔUBGC is the carbon uptake in the BGC experiment [CO2bgc]. The β feedback reflects the changes in land and ocean carbon pools driven by the changes in CO2 concentrations.
The γ parameter can be estimated from the [CO2rad]–[piControl], using Eq. (3):
where ΔURAD is the carbon uptake in the RAD experiment [CO2rad]. The γ feedback reflects the changes in the land and ocean carbon pools due to the changes in climate.
Many existing studies have estimated γ using the difference between the fully coupled (COU) and BGC experiments as a proxy for the RAD experiment (Arora et al., 2013, 2020; Asaadi et al., 2024; Friedlingstein et al., 2003, 2006; Melnikova et al., 2021). However, Zickfeld et al. (2011) and Schwinger et al. (2014) showed that this substitution introduces a residual term ε, which can be derived from the difference between [CO2]–[CO2bgc] and [CO2rad]–[piControl] using Eq. (4):
These studies indicate that the residual “nonlinearity” term depends on both CO2 concentration and climate change, and it can be of the same order of magnitude as the γ term. Here, we propose that this residual nonlinearity be attributed to a cross-term, χ. Although recent studies continue to subsume χ under the γ feedback – partly due to the absence of the [CO2rad] experiment in some experimental designs and also because this approach has been widely established in earlier research (Friedlingstein et al., 2003, 2006) – we show that these metrics become less well defined when examining the effects of both CO2 and non-CO2 GHGs on the carbon cycle.
In order to investigate the associated carbon–climate feedback nonlinearities, we propose the following theoretical framework. The changes in carbon uptake (GtC yr−1) in the COU simulation can be defined as a function of changes in CO2 concentration () and temperature (ΔT). Following Schwinger et al. (2014), the formulation can be expanded to a Taylor series up to the second-order terms:
where and ΔTforc. are the respective increments of CO2 concentration and temperature relative to [piControl]. The third- and higher-order terms are defined as a residual (Res.). We found them to be negligible in our case. We can disentangle the first- and second-order terms of the right-hand side of the Eq. (5) into terms that are purely dependent on CO2 (ΔUβ), on temperature (ΔUγ), and on the cross-term (ΔUχ) as follows:
For simplicity, the second-order terms of Eqs. (6) and (7) are included in Res. Combining Eqs. (4) and (8), the χ feedback parameter may be quantified from
The carbon–concentration β feedback term ΔUβ may be estimated from [CO2bgc]–[piControl], under the assumption that the physiological CO2 warming and its impacts on the carbon cycle are negligible, consistent with findings of Asaadi et al. (2024). The γ feedback terms for CO2 () and non-CO2 () gases are estimated from [CO2rad]–[piControl] and [nonCO2]–[piControl], respectively. The difference between these terms (–) yields the difference between impacts of CO2 and non-CO2 forcing on the carbon–climate feedback. Finally, the cross-term ΔUχ, previously referred to as a nonlinearity term (Arora et al., 2013, 2020; Gregory et al., 2009; Schwinger et al., 2014; Schwinger and Tjiputra, 2018; Williams et al., 2019; Zickfeld et al., 2011), may be estimated by utilising a combination of experiments. The combination [CO2]–[CO2bgc]–[CO2rad] gives , and the combination [CO2bgc + nonCO2]–[CO2bgc]–[nonCO2] gives . Analogously, the difference between these cross-terms () yields the difference between the CO2 and non-CO2 forcings on the χ.
3.1 Climate impacts
The analysis of global climate variables as a function of CO2 concentration and GSAT shown in Fig. 2 follows a previous study by Boucher et al. (2012). Consistent with their findings, our results show that GSAT change lags behind GHG forcing by up to 1 decade. The lag increases with the increase in the forcing magnitude so that the largest lag is in the [CO2+ nonCO2] experiment. Even after a ramp-down period and 50 years of constant GHG forcing at [piControl] levels, GSAT does not return to preindustrial values in all experiments (Fig. 2a). This can be explained by the inertia of the climate system, apparent in the changes in the ocean heat uptake (OHU). The OHU increases during the ramp-up and decreases during the ramp-down period, being positive, i.e. taking up energy away from the atmosphere, during the ramp-up and the first half of the ramp-down period. OHU turns negative by the end of the ramp-down and stays negative during the 50 years of the stabilisation period, releasing energy back into the atmosphere (Fig. 2c–e). The hysteresis of the climate system is evident from the nearly linear relationship between maximum GSAT and mean GSAT during the stabilisation period (Fig. S2). The thermosteric (unrelated to ice sheet melting) sea level increases in all experiments except for [CO2bgc] and is closely related to the OHU. It does not recover (i.e. it is irreversible) within the time horizon considered here (Fig. 2i–k). The AMOC decreases with GSAT (with the strongest decrease reached for GSAT of 2 °C under [CO2+ nonCO2]) but fully recovers (Fig. S1b).
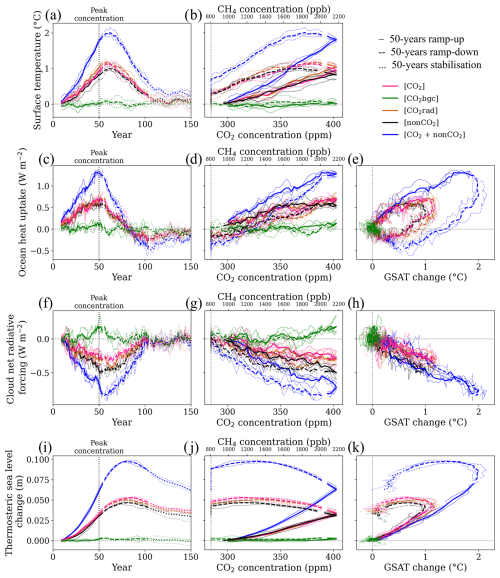
Figure 2Global annual mean changes in model climate variables as a function of (a, c, f, i) time (year), (b, d, g, j) CO2 concentration concentration (ppb; only for [nonCO2]), and (c, e, h, k) GSAT (°C) for (a, b) GSAT (°C), (c–e) ocean heat uptake (W m−2), (f–h) cloud net radiative forcing (W m−2), and (i–k) thermosteric sea level change (m) under selected scenarios. The ramp-up, ramp-down, and stabilisation periods are indicated by different line styles in all panels. Thick lines indicate the ensemble means, and thin lines correspond to three ensemble members.
The CO2 physiological warming that can be quantified by comparing [CO2bgc] with [piControl] is small (green line in Fig. 2). Spatially, some differences are ubiquitous over land, e.g. CO2 physiological warming persists over Eurasia during the ramp-up period, and over the high latitudes of both land and ocean during the stabilisation period (Fig. S3a). A larger ensemble size of model simulations would be required to investigate these differences more thoroughly. In our following analysis on carbon cycle feedbacks, we assume the CO2 physiological warming to be negligible, consistent with previous findings of Asaadi et al. (2024).
When comparing CO2- and non-CO2-induced forcing ([CO2] and [nonCO2] experiments) at a global scale, our results are consistent with Richardson et al. (2019), who show the higher surface temperature response of CO2 when compared to CH4. When comparing CO2- and non-CO2-induced radiative forcing ([CO2rad] and [nonCO2] experiments) at a global scale, the non-CO2 forcing still leads to a lower GSAT peak and a slightly lower peak of thermosteric sea level rise compared to the CO2 radiative forcing (brown and black lines of Fig. 2a, significant difference at p<0.05). This cannot be explained just by a slightly higher ERF of the [CO2rad] compared to the [nonCO2] experiment (Table 1, Figs. 2a and S4). Our results are consistent with Nordling et al. (2021), who show the higher effective temperature response for CO2 forcing compared to non-CO2 forcing, attributing it to the changes in clear-sky planetary emissivity.
Radiative forcing alone ([CO2rad] experiment) leads to a slightly higher global temperature increase compared to the coupled [CO2] experiment, which includes the combined effect of CO2 physiology and radiative forcing (Fig. 2a, b). This temperature difference is particularly evident in the Arctic region (Fig. S3a). Our findings differ from those of a CMIP5 intercomparison study, which reported that CO2 physiological warming amplifies the Arctic warming (Park et al., 2020). The study showed that the CO2 physiological effect contributes to high-latitude warming by reducing evaporative cooling due to stomatal closure under elevated CO2 levels. In contrast, we observe higher evapotranspiration in the [CO2rad] compared to the [CO2] experiment (Fig. S5), which is probably a consequence of the lower warming in the [CO2] experiment. In our study, the greater warming in the [CO2rad] experiment may be driven by increased surface albedo, especially in the Arctic Ocean (Fig. S3b). While the underlying causes remain unclear, this pattern appears consistent in other experiments conducted with IPSL-CM6A-LR under moderate CO2 levels (not shown). Because the ensemble size in our study is limited and the effects of the model's internal variability should be considerable, future research should validate the robustness of our findings with larger ensemble simulations.
3.2 Carbon cycle feedback
3.2.1 Carbon–concentration feedback
The term corresponds to the flux arising from variations in CO2 concentration ([CO2bgc] experiment). The dominates the land and ocean carbon uptake changes through all three considered periods (Fig. 3, Tables 2 and S1). Since the considered maximum surface warming levels are below 2 °C, land and ocean carbon fluxes are primarily controlled by the CO2-induced effects during the ramp-up period, resulting in positive β (Fig. 3a–d). Nearly two-thirds of land and half of ocean carbon accumulated during the ramp-up period due to the atmospheric CO2 increase are being released during the latter periods, consistent with previous studies (Asaadi et al., 2024; Chimuka et al., 2023). In the ocean, β is positive (carbon sink) in all regions during the ramp-up period. However, a decrease in CO2 concentration induces a carbon source over all ocean regions, except for the Atlantic and Southern oceans (Fig. 4). All regions are carbon sources during the stabilisation period of the [CO2bgc] experiment.
Table 2Cumulative CO2 and climate-change-driven changes in the land and ocean carbon fluxes (GtC), shown as a three-member ensemble mean. The ± indicates 1 standard deviation among the three members. Note that all experiments are analysed relative to their [piControl] counterparts.
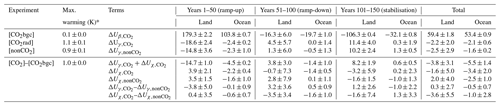
* Defined as the mean ΔGSAT during years 41–60 in the experiments.
The transient change in the β feedback over land can be better explained by analysing the gross primary production (GPP) and the autotrophic and heterotrophic respiration (Ra and Rh) fluxes. Land GPP, representing photosynthetic uptake, increases during the ramp-up period under elevated CO2 concentration and decreases almost linearly with decreasing CO2, showing only a small hysteresis (Figs. S6–S7). In contrast, both Ra and Rh exhibit a larger hysteresis, which leads to an extended period of the carbon release to the atmosphere. This suggests that, while there may be initial carbon sequestration benefits gained during elevated CO2 periods, these benefits are susceptible to being lost as CO2 concentrations decline due to decreased photosynthesis and increased respiration, albeit at a reduced rate.
The spatial variation in cumulative net carbon uptake provides further details on the feedback changes (Figs. 4, S8–S10). During the CO2 ramp-up phase, CO2 increase triggers a land carbon sink in all regions. However, during the ramp-down phase, it induces a net carbon source over subtropical regions while still driving a land carbon sink in northern high latitudes so that global land becomes a carbon source in the middle of the ramp-down phase. Finally, during the stabilisation period, all land regions become net carbon sources.
Subtropical and southern land regions exhibit a shorter hysteresis in response to decreasing CO2 concentrations. This disparity arises from the larger proportion of carbon accumulated in aboveground vegetation biomass in southern regions, contrasting with the greater fraction stored in soils within northern latitudes (Figs. S9–S11). The extended period of high positive β in northern mid- to high latitudes can be attributed to the longer carbon turnover time, particularly in soils, compared to tropical regions (Fig. S8).
3.2.2 Carbon–climate feedback
The term ΔUγ corresponds to the carbon flux arising from variations in radiative forcing (such as in the [nonCO2] and [CO2rad] experiments). The ΔUγ for CO2 and non-CO2 ( and , respectively) are equivalent (within 1 standard deviation uncertainty range) under nearly equivalent levels of ERF (compare panels b and c of Fig. 4; see also – in Table 2 and Table S1). The γ is negative on a global scale in the land and ocean, with greater magnitude but also faster reversibility over land. Spatially, land γ is positive in the mid- to high latitudes and negative in the tropical regions (Figs. 4, S9–10; see also Melnikova et al., 2021). During the ramp-up, climate change drives carbon sink in the northern mid- to high latitudes and carbon source in the subtropical regions and the Southern Hemisphere, with a larger magnitude of changes in the experiments, in which CO2 concentration change is present (Figs. 4, S9–11).
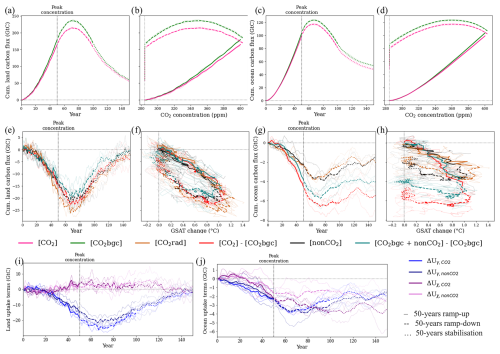
Figure 3Global cumulative carbon fluxes (GtC) over (a, b, e, f, i) land and (c, d, g, h, j) ocean as a function of (a, c, e, g, i, j) time (year), (b, d) CO2 concentration (ppm), and (f, h) GSAT changes (°C) under selected scenarios. The ramp-up, ramp-down, and stabilisation periods are indicated by different line styles. Thick lines indicate the ensemble means, and thin lines correspond to other ensemble members. Note that vertical axes differ between panels (e) and (g) and panels (i) and (j).
3.2.3 Nonlinearity in carbon cycle feedback
The cross-term ΔUχ induces non-negligible differences between climate-change-induced carbon flux when comparing experiments with the presence or absence of atmospheric CO2 concentration change. It reaches ca. 20 %–23 % of the land ΔUγ and ca. 70 %–90 % of the ocean ΔUγ, cumulative over the ramp-up period (Table 2). During the ramp-up phase, the ΔUχ corresponds to a decrease in the climate-driven land carbon source and an increase in the climate-driven ocean carbon source (Fig. 3). The χ feedback is positive (larger carbon sink) on land and negative (larger carbon source) in the ocean (Table S1). There is no significant difference between CO2 and non-CO2 χ feedback at similar ERF levels (Figs. 3e–j and 4f–g, Tables 2 and S1). In the ocean, the cross-term differences for CO2 and non-CO2 forcing already arise during the ramp-up and propagate during the ramp-down and stabilisation phases, spatially concentrating in the deep-mixing region of the Southern Ocean.
On land, the positive χ reflects more biomass at high latitudes available for climate change effects, leading to a larger carbon sink (positive γ). During the ramp-down, climate warming through temperature change (lagged after GHG concentrations change) increases the carbon sink over high latitudes and weakens the carbon source in the tropics. Here, the ΔUχ reflects more biomass available globally for the climate change effects, leading to a larger carbon source (negative γ). The differences (in the presence/absence of the cross-term ΔUχ) diminish for land but not for the ocean during the ramp-down and stabilisation periods.
In the ocean, the contribution from the nonlinearity of carbon cycle feedbacks leads to a greater reduction in the CO2-driven carbon sink (Fig. 3). The contribution of the cross-term ΔUχ to the total ΔU increases during the ramp-down phases of considered GHG concentration scenarios. Previously, Schwinger and Tjiputra (2018), who considered nonlinearity of carbon cycle feedback, warned that RAD experiments may underestimate the carbon–climate feedback (when compared to COU–BGC experiments) because “the reduction of sequestration of preformed dissolved inorganic carbon under high atmospheric CO2 is not taken into account.” In this study, the nonlinearity effects nearly double climate-change-driven carbon loss (compared to the RAD experiment, in which atmospheric CO2 is constant) relative to the total 150-year net ocean carbon uptake under the [CO2] experiment. Spatially, while the Southern Ocean remains the largest ocean carbon sink in all considered experiments involving atmospheric CO2 changes, it, along with the Atlantic Ocean, undergoes the largest climate-change-driven reduction in carbon sink (Fig. 4).
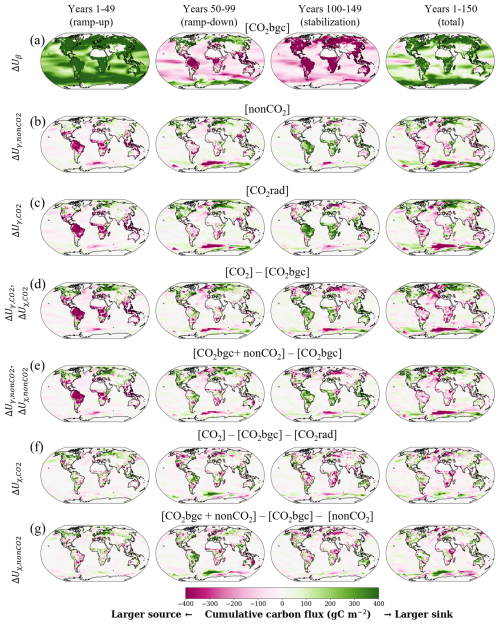
Figure 4Spatial variation in land and ocean carbon fluxes (GtC, negative to the atmosphere) cumulative over 50 years of (first column) ramp-up (second column) and ramp-down (third column) stabilisation phases and (last column) the full 150-year period. The data for the three-member ensemble mean are used.
Our findings provide evidence on the effectiveness of non-CO2 GHG mitigation. While it can effectively reduce GSAT peak, non-CO2 GHG mitigation may also lead to smaller climate-change-driven losses in the ocean carbon sink. In the real world, the presence/absence of ΔUχ suggests disparities between CO2 mitigation efforts and non-CO2 mitigation efforts. The CO2- and non-CO2-driven climate change leads to an unequal decrease in carbon uptake, especially apparent for the ocean on a global scale (compare red and black lines in Fig. 3, corresponding to [CO2]–[CO2bgc] and [nonCO2] experiments). Reducing CO2 concentrations for climate mitigation implies alteration of all three terms of the proposed carbon cycle feedback attribution framework, namely ΔUβ, ΔUγ, and ΔUχ. Reducing non-CO2 GHG concentrations, such as CH4 and N2O, implies alteration of ΔUγ and ΔUχ terms. Reducing both CO2 and non-CO2 concentrations implies alteration of all ΔUβ, ΔUγ, and ΔUχ terms but with larger change in ΔUγ and ΔUχ terms. From this point, combining CO2 and non-CO2 reduction measures may be more effective for climate change mitigation compared to the CO2 reduction measures alone. This finding should be confirmed with emission-driven experiments that consider GHG atmospheric lifetimes.
3.3 Radiative forcing and carbon cycle feedback additivity
In order to overcome the small signal-to-noise ratio of the considered experiments and the regional differences in the radiative forcing between [CO2] and [nonCO2] experiments, we compare (1) the [CO2+ nonCO2] experiment that includes both CO2 and non-CO2 effects with (2) the sum of two [CO2] and [nonCO2] experiments that include CO2 and non-CO2 effects, accordingly (Figs. 5 and S12–13). The climate effects, defined via temperature change, differ during the ramp-up and ramp-down periods (Fig. S12). These differences imply non-additivity of radiative forcing and can also be attributed to biophysical feedback.
As for the carbon cycle feedback, the [CO2+ nonCO2] experiment that has all feedback is different from the sum of two experiments [CO2] + [nonCO2] both on land and in the ocean (Fig. 5). The differences are larger and stay longer in the ocean. This implies non-additivity of carbon cycle feedback. From the proposed carbon cycle feedback attribution framework, the non-additivity arises from non-equality of () and (). The significant difference (p<0.1) on land is in the high-latitude region, where the “all-effects together” [CO2+ nonCO2] experiment yields a larger carbon sink during the ramp-up phase. The largest difference in the ocean is in the Southern Ocean, followed by the North Atlantic Ocean. The [CO2+ nonCO2] experiment has a large carbon sink in the Southern Ocean compared to the sum of two experiments. This might be related to the saturation of the decrease in the mixed-layer depth with more warming, but a more thorough study is needed to confirm such phenomena.
To our knowledge, this is the first study of its kind to compare idealised CO2 and non-CO2 ramp-up and ramp-down scenarios for their effects on global temperature change and the carbon cycle feedbacks. Below we draw attention to the caveats and limitations that should be addressed in future studies.
Firstly, since IPSL-CM6A-LR, like all other ESMs participating in CMIP6, does not have interactive modules of the CH4 and N2O cycles, the changes in stratospheric water vapour, aerosols, and tropospheric ozone due to atmospheric CH4 changes and the effects of nitrogen deposition on the carbon cycle are not considered in this study. Future studies could separately consider simulations for CH4 and N2O. However, for this study, the use of the model is justified because current changes in CH4 and N2O concentrations are primarily driven by anthropogenic sources, suggesting that the absence of interactive modules of natural sink/source processes does not significantly affect the representation of natural variability trends for the CH4 and N2O concentration (Nakazawa, 2020; Palazzo Corner et al., 2023; Zhu et al., 2013).
Secondly, when interpreting the results, it should be kept in mind that some carbon cycle processes in IPSL-CM6A-LR, such as permafrost and fire, are not considered. However, these should have only a limited impact on our results, given the (relatively) small warming levels in the considered experiments. Previous studies have shown that IPSL-CM6A-LR estimates one of the smallest soil carbon pools among CMIP6 models, which may lead to an underestimation of the carbon–climate feedback (Arora et al., 2020; Melnikova et al., 2021).
Thirdly, the results of the present study are limited by the use of a single ESM and a small number of ensemble members. Conducting similar experiments with other ESMs and using larger ensemble runs, which are particularly valuable in the low-warming scenarios, and complementing the findings of our study with emission-driven experiments could contribute to validating and extending our findings.
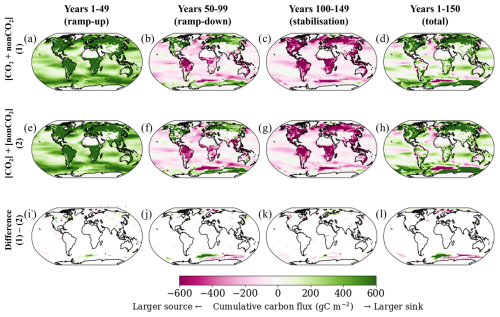
Figure 5Spatial variation in three-member ensemble mean land and ocean carbon fluxes (GtC, negative to the atmosphere) cumulative over 50 years of (a, e, i) ramp-up, (b, f, j) ramp-down, (c, g, k) stabilisation phases, and (d, h, l) the full 150-year period. We draw only significantly different grids between (i–l) [CO2+ nonCO2] and [CO2] + [nonCO2] experiments using three ensemble members (p<0.1 based on t-test, N=60).
This study first presents a novel approach to recalibrate the ERF of CH4 and N2O in ESMs without changing the radiative scheme of the model. We then discuss the effects of increases and decreases in the concentrations of the CO2 and non-CO2 GHGs on the surface climate and carbon cycle. We find only small differences between CO2 and non-CO2 ramp-up and ramp-down forcing on global and regional climate.
The differences in climate responses can be linked to differences in the carbon cycle feedbacks. We show that CO2- and non-CO2-driven carbon–climate feedback are nearly equivalent at a global scale. However, increasing atmospheric CO2 amplifies the reduction in the climate-change-driven carbon sink, especially in the ocean. We propose a novel framework to disentangle the carbon–climate feedback into a component that is purely driven by climate change, i.e. expressed as a temperature term, and a component driven by climate change and rising atmospheric CO2 at the same time, i.e. a cross-term. Since the cross-term can be quantified from the difference between COU, BGC, and RAD simulations, we advocate for continuing to carry out all three types of experiments in the future phases of CMIP. We further warn that the cross-term and non-additivity of feedback should be considered in the simple climate models (emulators).
Finally, this study showcases the additional benefits of non-CO2 GHG mitigation on a smaller reduction in the ocean carbon sinks under overshoot scenarios. We stress that our findings do not imply that non-CO2 GHG mitigation should be given a priority over other means to mitigate climate change but that they provide insights on the intricate interplay between the carbon–concentration and CO2- and non-CO2-driven carbon–climate feedbacks to inform comprehensive mitigation strategies.
A set of 40-year idealised IPSL-CM6A-LR simulations (840 years in total) has been carried out. In these experiments, the sea surface temperature (SST) and sea ice fractions were fixed to their preindustrial levels. CH4 concentration levels were kept at 2× CH4, 3× CH4, 4× CH4, 5× CH4, 8× CH4, and 12× CH4, and N2O concentration levels were kept at 1× N2O, 1.25× N2O, 1.5× N2O, and 1.75× N2O (Table A1).
Figure A1 shows the set of 40-year time series of global mean radiative forcing based on 21 idealised experiments. The piClim experiment holds preindustrial levels of CO2, CH4, and N2O concentrations. The mean interannual variation in the radiative forcings (1 standard deviation) is 0.15 W m−2. The first 10 years of the experiments were dropped to allow the climate to adjust to the new radiative equilibrium after an abrupt change from the preindustrial levels. The last 30 years were used to obtain 18 data points of mean global ERF by IPSL-CM6A-LR relative to the levels obtained from the piClim experiment. The ERF is estimated as a TOA imbalance difference between each experiment and the piClim.
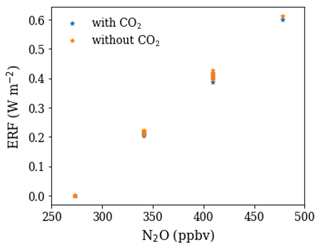
Figure A2The ERF estimated from E16 with and without accounting for CO2 impact on the N2O forcing (including/excluding the term in the equation) using the concentration values of the idealised experiments described in Table 1 with preindustrial CO2 concentration (284.32 ppm) and the same set of the experiments with 3 × CO2 concentration (852.96 ppm).
There are two frequently used sets of equations to derive radiative forcing of well-mixed greenhouse gases, e.g. CO2, CH4, and N2O, based on their concentrations. The first set from Myhre et al. (1998; hereafter, M98) was used in IPCC AR3:
Here, the CO2, CH4, and N2O indicate their concentrations, where the units are ppm, ppb, and ppb, respectively.
Etminan et al. (2016; hereafter, E16) improved the IPCC AR3 equations by inclusion of the short-wave (near-infrared) bands of CH4. Their set of equations was used in IPCC AR6. The radiative forcing of N2O in the M98 equation depends on the CH4 and N2O concentrations, while the radiative forcing of N2O in the equation by E16 depends on the CO2, CH4, and N2O concentrations:
In the sets of Eq. (A2), the radiative forcing due to CH4 depends not only on the CH4 concentration but also on that of N2O (and conversely) because CH4 and N2O absorption bands overlap to some extent. These simplified equations are therefore additive: the radiative forcing due to a change in CH4 and N2O concentrations (CH4, N2O) relative to reference (preindustrial) values (, ) is equal to
The effect of CO2 on the radiative forcing of N2O is small (< 5 %) and is thus, for simplicity, neglected in the rest of this study (Fig. A2).
Figure A3 shows the ERFs simulated by IPSL-CM6A-LR and those estimated from the two equations from the IPCC report and revised by E16. IPSL-CM6A-LR underestimates CH4 ERF and overestimates N2O ERF, relative to both equations. Considering the improvements introduced by E16 to include the short-wave bands of CH4, we use their equations (and not of IPCC AR3) to recalibrate the IPSL-CM6A-LR model.
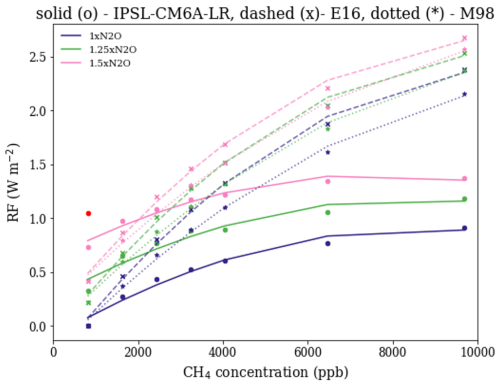
Figure A3The ERF of CH4 and N2O from IPSL-CM6A-LR idealised experiments (points and fitted solid lines) and estimated from M98 (* and fitted dotted lines) and E16 (x and fitted dashed lines) equations, fitted to polynomial regressions for three levels of N2O concentrations.
A system of equations can convert the input CH4 and N2O concentrations to the effective concentrations so that, if used in the climate model, they would yield actual forcing from the equations by E16. Among several linear and nonlinear functions to relate the actual concentrations of CH4 and N2O with the concentrations seen by the IPSL-CM6A model (effective concentrations) that have been tested, the following set of equations yielded the best fit:
The initial values of CH4 and N2O concentrations are fixed at the preindustrial levels (t=0). Those of the effective CH4 and N2O concentrations are also assumed to take the same respective preindustrial levels. Eq. (A1) is used to relate the effective concentrations of CH4 and N2O of IPSL-CM6A-LR to the ERF estimated by E16:
We optimise the four parameters of Eq. (A4) by minimising the sum of squared residuals between ERF estimated by E16 equations and simulated by IPSL-CM6A-LR using a Python implementation of the Limited-memory Broyden–Fletcher–Goldfarb–Shanno algorithm with bound constraints (L-BFGS-B), which is an algorithm for solving large nonlinear optimisation problems with simple bounds (Byrd et al., 1995). The cost function is defined as
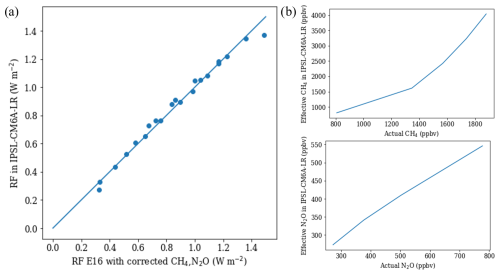
Figure A4(a) Scatterplot of ERF simulated by IPSL-CM6A and estimated by E16 equations using the corrected effective concentrations from Eq. (A3) and (b) the effective IPSL-CM6A-LR concentrations of CH4 and N2O as a function of the actual concentrations derived via a system of nonlinear functions.
The residual error after fit equals 0.03 (W m−2)2 (Fig. A4). The solution of the cost function provides the estimates of four uncertain parameters for Eq. (3), indicated in Table A2. The effective IPSL-CM6A-LR concentrations of CH4 and N2O are functions of the actual concentrations (Fig. A4b). Higher effective CH4 and lower N2O effective concentrations are needed for IPSL-CM6A-LR to reproduce the ERF in agreement with IPCC estimates.
The estimated parameters were applied to derive the effective IPSL-CM6A-LR concentrations for a target scenario (Fig. A5). Higher CH4 and lower N2O concentrations are required to reproduce the ERF correctly.
The piControl output data from the CMIP6 simulations are available from the CMIP6 archive: https://esgf-node.llnl.gov/search/cmip6 (WCRP, 2022). Additional IPSL-CM6A-LR outputs are available upon request. The Jupyter notebook and data to reproduce the figures are stored in the Zenodo archive: https://doi.org/10.5281/zenodo.12200056 (Melnikova, 2024).
The supplement related to this article is available online at: https://doi.org/10.5194/esd-16-257-2025-supplement.
IM and OB initiated the study. OB, KATT, and IM developed methodology and study ideas. IM and OB performed IPSL-CM6A-LR simulations. IM performed data analysis and wrote the initial draft. All authors contributed to the discussions and to writing and revising the paper.
The contact author has declared that none of the authors has any competing interests.
Publisher’s note: Copernicus Publications remains neutral with regard to jurisdictional claims made in the text, published maps, institutional affiliations, or any other geographical representation in this paper. While Copernicus Publications makes every effort to include appropriate place names, the final responsibility lies with the authors.
The IPSL-CM6 simulations were performed using the HPC resources of TGCC under the allocation 2022-A0120107732 and 2023-A0140107732 (project gencmip6) provided by the Grand Equipement National de Calcul Intensif (GENCI). This work benefited from the technical support of and discussions with Adriana Sima and Nicolas Lebas at IPSL, Arnaud Caubel and Sébastien Nguyen at LSCE (France), and Michio Kawamiya at JAMSTEC (Japan).
This work benefited from state assistance managed by the National Research Agency in France under the Programme d'Investissements d'Avenir (grant no. ANR-19-MPGA-0008). We further acknowledge the Program for the Advanced Studies of Climate Change Projection (SENTAN; grant no. JPMXD0722681344) from the Ministry of Education, Culture, Sports, Science and Technology, Japan, and KAKENHI (grant no. JP24K20979) of the Japan Society for the Promotion of Science. We acknowledge the European Union's Horizon Europe research and innovation programme (grant nos. 101056939) (RESCUE – Response of the Earth System to overshoot, Climate neUtrality and negative Emissions) and (grant no. 101081193) (OptimESM – Optimal High Resolution Earth System Models for Exploring Future Climate Changes). Philippe Ciais acknowledges support from the CArbon Losses in Plants, Soils and Ocean (CALIPSO) project funded through the generosity of Eric and Wendy Schmidt by recommendation of the Schmidt Futures programme.
This paper was edited by Kirsten Zickfeld and reviewed by Jörg Schwinger and one anonymous referee.
Abernethy, S., O'Connor, F. M., Jones, C. D., and Jackson, R. B.: Methane removal and the proportional reductions in surface temperature and ozone, Philos. T. R. Soc. A, 379, 20210104, https://doi.org/10.1098/rsta.2021.0104, 2021.
Arora, V. K., Boer, G. J., Friedlingstein, P., Eby, M., Jones, C. D., Christian, J. R., Bonan, G., Bopp, L., Brovkin, V., Cadule, P., Hajima, T., Ilyina, T., Lindsay, K., Tjiputra, J. F., and Wu, T.: Carbon–Concentration and Carbon–Climate Feedbacks in CMIP5 Earth System Models, J. Climate, 26, 5289–5314, https://doi.org/10.1175/JCLI-D-12-00494.1, 2013.
Arora, V. K., Katavouta, A., Williams, R. G., Jones, C. D., Brovkin, V., Friedlingstein, P., Schwinger, J., Bopp, L., Boucher, O., Cadule, P., Chamberlain, M. A., Christian, J. R., Delire, C., Fisher, R. A., Hajima, T., Ilyina, T., Joetzjer, E., Kawamiya, M., Koven, C. D., Krasting, J. P., Law, R. M., Lawrence, D. M., Lenton, A., Lindsay, K., Pongratz, J., Raddatz, T., Séférian, R., Tachiiri, K., Tjiputra, J. F., Wiltshire, A., Wu, T., and Ziehn, T.: Carbon–concentration and carbon–climate feedbacks in CMIP6 models and their comparison to CMIP5 models, Biogeosciences, 17, 4173–4222, https://doi.org/10.5194/bg-17-4173-2020, 2020.
Asaadi, A., Schwinger, J., Lee, H., Tjiputra, J., Arora, V., Séférian, R., Liddicoat, S., Hajima, T., Santana-Falcón, Y., and Jones, C. D.: Carbon cycle feedbacks in an idealized simulation and a scenario simulation of negative emissions in CMIP6 Earth system models, Biogeosciences, 21, 411–435, https://doi.org/10.5194/bg-21-411-2024, 2024.
Bonnet, R., Swingedouw, D., Gastineau, G., Boucher, O., Deshayes, J., Hourdin, F., Mignot, J., Servonnat, J., and Sima, A.: Increased risk of near term global warming due to a recent AMOC weakening, Nat. Commun., 12, 6108, https://doi.org/10.1038/s41467-021-26370-0, 2021.
Boucher, O. and Folberth, G. A.: New Directions: Atmospheric methane removal as a way to mitigate climate change?, Atmos. Environ., 44, 3343–3345, https://doi.org/10.1016/j.atmosenv.2010.04.032, 2010.
Boucher, O., Halloran, P. R., Burke, E. J., Doutriaux-Boucher, M., Jones, C. D., Lowe, J., Ringer, M. A., Robertson, E., and Wu, P.: Reversibility in an Earth System model in response to CO2 concentration changes, Environ. Res. Lett., 7, 024013, https://doi.org/10.1088/1748-9326/7/2/024013, 2012.
Boucher, O., Servonnat, J., Albright, A. L., Aumont, O., Balkanski, Y., Bastrikov, V., Bekki, S., Bonnet, R., Bony, S., Bopp, L., Braconnot, P., Brockmann, P., Cadule, P., Caubel, A., Cheruy, F., Codron, F., Cozic, A., Cugnet, D., D'Andrea, F., Davini, P., Lavergne, C. de, Denvil, S., Deshayes, J., Devilliers, M., Ducharne, A., Dufresne, J.-L., Dupont, E., Éthé, C., Fairhead, L., Falletti, L., Flavoni, S., Foujols, M.-A., Gardoll, S., Gastineau, G., Ghattas, J., Grandpeix, J.-Y., Guenet, B., Guez, L., E., Guilyardi, E., Guimberteau, M., Hauglustaine, D., Hourdin, F., Idelkadi, A., Joussaume, S., Kageyama, M., Khodri, M., Krinner, G., Lebas, N., Levavasseur, G., Lévy, C., Li, L., Lott, F., Lurton, T., Luyssaert, S., Madec, G., Madeleine, J.-B., Maignan, F., Marchand, M., Marti, O., Mellul, L., Meurdesoif, Y., Mignot, J., Musat, I., Ottlé, C., Peylin, P., Planton, Y., Polcher, J., Rio, C., Rochetin, N., Rousset, C., Sepulchre, P., Sima, A., Swingedouw, D., Thiéblemont, R., Traore, A. K., Vancoppenolle, M., Vial, J., Vialard, J., Viovy, N., and Vuichard, N.: Presentation and Evaluation of the IPSL-CM6A-LR Climate Model, J. Adv. Model. Earth Syst., 12, e2019MS002010, https://doi.org/10.1029/2019MS002010, 2020.
Byrd, R. H., Lu, P., Nocedal, J., and Zhu, C.: A Limited Memory Algorithm for Bound Constrained Optimization, SIAM J. Sci. Comput., 16, 1190–1208, https://doi.org/10.1137/0916069, 1995.
Canadell, J. G., Monteiro, P. M., Costa, M. H., Da Cunha, L. C., Cox, P. M., Alexey, V., Henson, S., Ishii, M., Jaccard, S., and Koven, C.: Global carbon and other biogeochemical cycles and feedbacks., in: Climate Change 2021: The Physical Science Basis. Contribution of Working Group I to the Sixth Assessment Report of the Intergovernmental Panel on Climate Change, Chap. 5, Cambridge University Press, https://doi.org/10.1017/9781009157896.007, 2021.
CCAC (Climate and Clean Air Coalition): Global Methane Pledge, https://www.ccacoalition.org/content/global-methane-pledge (last access: 29 January 2025), 2021.
Chimuka, V. R., Nzotungicimpaye, C.-M., and Zickfeld, K.: Quantifying land carbon cycle feedbacks under negative CO2 emissions, Biogeosciences, 20, 2283–2299, https://doi.org/10.5194/bg-20-2283-2023, 2023.
Collins, W. D., Ramaswamy, V., Schwarzkopf, M. D., Sun, Y., Portmann, R. W., Fu, Q., Casanova, S. E. B., Dufresne, J.-L., Fillmore, D. W., Forster, P. M. D., Galin, V. Y., Gohar, L. K., Ingram, W. J., Kratz, D. P., Lefebvre, M.-P., Li, J., Marquet, P., Oinas, V., Tsushima, Y., Uchiyama, T., and Zhong, W. Y.: Radiative forcing by well-mixed greenhouse gases: Estimates from climate models in the Intergovernmental Panel on Climate Change (IPCC) Fourth Assessment Report (AR4), J. Geophys. Res.-Atmos., 111, D14317, https://doi.org/10.1029/2005JD006713, 2006.
de Richter, R., Ming, T., Davies, P., Liu, W., and Caillol, S.: Removal of non-CO2 greenhouse gases by large-scale atmospheric solar photocatalysis, Prog. Energ. Combust., 60, 68–96, https://doi.org/10.1016/j.pecs.2017.01.001, 2017.
Etminan, M., Myhre, G., Highwood, E. J., and Shine, K. P.: Radiative forcing of carbon dioxide, methane, and nitrous oxide: A significant revision of the methane radiative forcing, Geophys. Res. Lett., 43, 12614–12623, https://doi.org/10.1002/2016GL071930, 2016.
Forster, P., Storelvmo, T., Armour, K., Collins, W., Dufresne, J.-L., Frame, D., Lunt, D. J., Mauritsen, T., Palmer, M. D., Watanabe, M., Wild, M., and Zhang, H.: The Earth's Energy Budget, Climate Feedbacks, and Climate Sensitivity, edited by: Masson-Delmotte, V., Zhai, P., Pirani, A., Connors, S. L., Péan, C., Berger, S., Caud, N., Chen, Y., Goldfarb, L., Gomis, M. I., Huang, M., Leitzell, K., Lonnoy, E., Matthews, J. B. R., Maycock, T. K., Waterfield, T., Yelekçi, O., Yu, R., and Zhou, B.: Climate Change 2021, The Physical Science Basis, Contribution of Working Group I to the Sixth Assessment Report of the Intergovernmental Panel on Climate Change, 923–1054, https://doi.org/10.1017/9781009157896.009, 2021.
Friedlingstein, P., Dufresne, J.-L., Cox, P. M., and Rayner, P.: How positive is the feedback between climate change and the carbon cycle?, Tellus B, 55, 692–700, https://doi.org/10.3402/tellusb.v55i2.16765, 2003.
Friedlingstein, P., Cox, P., Betts, R., Bopp, L., von Bloh, W., Brovkin, V., Cadule, P., Doney, S., Eby, M., Fung, I., Bala, G., John, J., Jones, C., Joos, F., Kato, T., Kawamiya, M., Knorr, W., Lindsay, K., Matthews, H. D., Raddatz, T., Rayner, P., Reick, C., Roeckner, E., Schnitzler, K.-G., Schnur, R., Strassmann, K., Weaver, A. J., Yoshikawa, C., and Zeng, N.: Climate–Carbon Cycle Feedback Analysis: Results from the C4MIP Model Intercomparison, J. Climate, 19, 3337–3353, https://doi.org/10.1175/JCLI3800.1, 2006.
Fu, B., Gasser, T., Li, B., Tao, S., Ciais, P., Piao, S., Balkanski, Y., Li, W., Yin, T., Han, L., Li, X., Han, Y., An, J., Peng, S., and Xu, J.: Short-lived climate forcers have long-term climate impacts via the carbon–climate feedback, Nat. Clim. Change, 10, 851–855, https://doi.org/10.1038/s41558-020-0841-x, 2020.
Fyfe, J. C., Kharin, V. V., Santer, B. D., Cole, J. N. S., and Gillett, N. P.: Significant impact of forcing uncertainty in a large ensemble of climate model simulations, P. Natl. Acad. Sci. USA, 118, e2016549118, https://doi.org/10.1073/pnas.2016549118, 2021.
Gregory, J. M., Jones, C. D., Cadule, P., and Friedlingstein, P.: Quantifying Carbon Cycle Feedbacks, J. Climate, 22, 5232–5250, https://doi.org/10.1175/2009JCLI2949.1, 2009.
Hogan, R. J. and Matricardi, M.: Evaluating and improving the treatment of gases in radiation schemes: the Correlated K-Distribution Model Intercomparison Project (CKDMIP), Geosci. Model Dev., 13, 6501–6521, https://doi.org/10.5194/gmd-13-6501-2020, 2020.
Höglund-Isaksson, L., Gómez-Sanabria, A., Klimont, Z., Rafaj, P., and Schöpp, W.: Technical potentials and costs for reducing global anthropogenic methane emissions in the 2050 timeframe – results from the GAINS model, Environ. Res. Commun., 2, 025004, https://doi.org/10.1088/2515-7620/ab7457, 2020.
Jackson, R. B., Abernethy, S., Canadell, J. G., Cargnello, M., Davis, S. J., Féron, S., Fuss, S., Heyer, A. J., Hong, C., and Jones, C. D.: Atmospheric methane removal: a research agenda, Philos. T. R. Soc. A, 379, 20200454, https://doi.org/10.1098/rsta.2020.0454, 2021.
Jones, A., Haywood, J. M., and Jones, C. D.: Can reducing black carbon and methane below RCP2.6 levels keep global warming below 1.5 °C?, Atmos. Sci. Lett., 19, e821, https://doi.org/10.1002/asl.821, 2018.
Jones, C. D., Ciais, P., Davis, S. J., Friedlingstein, P., Gasser, T., Peters, G. P., Rogelj, J., van Vuuren, D. P., Canadell, J. G., Cowie, A., Jackson, R. B., Jonas, M., Kriegler, E., Littleton, E., Lowe, J. A., Milne, J., Shrestha, G., Smith, P., Torvanger, A., and Wiltshire, A.: Simulating the Earth system response to negative emissions, Environ. Res. Lett., 11, 095012, https://doi.org/10.1088/1748-9326/11/9/095012, 2016.
Koven, C. D., Sanderson, B. M., and Swann, A. L. S.: Much of zero emissions commitment occurs before reaching net zero emissions, Environ. Res. Lett., 18, 014017, https://doi.org/10.1088/1748-9326/acab1a, 2023.
Lurton, T., Balkanski, Y., Bastrikov, V., Bekki, S., Bopp, L., Braconnot, P., Brockmann, P., Cadule, P., Contoux, C., Cozic, A., Cugnet, D., Dufresne, J.-L., Éthé, C., Foujols, M.-A., Ghattas, J., Hauglustaine, D., Hu, R.-M., Kageyama, M., Khodri, M., Lebas, N., Levavasseur, G., Marchand, M., Ottlé, C., Peylin, P., Sima, A., Szopa, S., Thiéblemont, R., Vuichard, N., and Boucher, O.: Implementation of the CMIP6 Forcing Data in the IPSL-CM6A-LR Model, J. Adv. Model. Earth Syst., 12, e2019MS001940, https://doi.org/10.1029/2019MS001940, 2020.
Malley, C. S., Borgford-Parnell, N., Haeussling, S., Howard, I. C., Lefèvre, E. N., and Kuylenstierna, J. C. I.: A roadmap to achieve the global methane pledge, Environ. Res.-Climate, 2, 011003, https://doi.org/10.1088/2752-5295/acb4b4, 2023.
Melnikova, I.: Code and data for “Carbon cycle and climate feedback under CO2 and non-CO2 overshoot pathways”, Zenodo [data set], https://doi.org/10.5281/zenodo.12200056, 2024.
Melnikova, I., Boucher, O., Cadule, P., Ciais, P., Gasser, T., Quilcaille, Y., Shiogama, H., Tachiiri, K., Yokohata, T., and Tanaka, K.: Carbon cycle response to temperature overshoot beyond 2 °C – an analysis of CMIP6 models, Earth's Fut., 9, e2020EF001967, https://doi.org/10.1029/2020EF001967, 2021.
Mengis, N. and Matthews, H. D.: Non-CO2 forcing changes will likely decrease the remaining carbon budget for 1.5 °C, Clim. Atmos. Sci., 3, 19, https://doi.org/10.1038/s41612-020-0123-3, 2020.
Montzka, S. A., Dlugokencky, E. J., and Butler, J. H.: Non-CO2 greenhouse gases and climate change, Nature, 476, 43–50, https://doi.org/10.1038/nature10322, 2011.
Mundra, I. and Lockley, A.: Emergent methane mitigation and removal approaches: A review, Atmos. Environ., X, 100223, ISSN 2590-1621, https://doi.org/10.1016/j.aeaoa.2023.100223, 2023.
Myhre, G., Highwood, E. J., Shine, K. P., and Stordal, F.: New estimates of radiative forcing due to well mixed greenhouse gases, Geophys. Res. Lett., 25, 2715–2718, https://doi.org/10.1029/98GL01908, 1998.
Myhre, G., Shindell, D., Bréon, F. M., Collins, W., Fuglestvedt, J., Huang, J., Koch, D., Lamarque, J. F., Lee, D., and Mendoza, B.: Anthropogenic and natural radiative forcing, in: Climate change 2013: the physical science basis. Contribution of Working Group I to the Fifth Assessment Report of the Intergovernmental Panel on Climate Change, Chapter 8, Cambridge University Press Cambridge, https://doi.org/10.1017/CBO9781107415324.018, 2013.
Myhre, G., Forster, P. M., Samset, B. H., Hodnebrog, Ø., Sillmann, J., Aalbergsjø, S. G., Andrews, T., Boucher, O., Faluvegi, G., Fläschner, D., Iversen, T., Kasoar, M., Kharin, V., Kirkevåg, A., Lamarque, J.-F., Olivié, D., Richardson, T. B., Shindell, D., Shine, K. P., Stjern, C. W., Takemura, T., Voulgarakis, A., and Zwiers, F.: PDRMIP: A Precipitation Driver and Response Model Intercomparison Project – Protocol and Preliminary Results, B. Am. Meteorol. Soc., 98, 1185–1198, https://doi.org/10.1175/BAMS-D-16-0019.1, 2017.
Nakazawa, T.: Current understanding of the global cycling of carbon dioxide, methane, and nitrous oxide, P. Jpn. Acad. B-Phys., 96, 394–419, https://doi.org/10.2183/pjab.96.030, 2020.
Nisbet, E. G., Fisher, R. E., Lowry, D., France, J. L., Allen, G., Bakkaloglu, S., Broderick, T. J., Cain, M., Coleman, M., Fernandez, J., Forster, G., Griffiths, P. T., Iverach, C. P., Kelly, B. F. J., Manning, M. R., Nisbet-Jones, P. B. R., Pyle, J. A., Townsend-Small, A., al-Shalaan, A., Warwick, N., and Zazzeri, G.: Methane Mitigation: Methods to Reduce Emissions, on the Path to the Paris Agreement, Rev. Geophys., 58, e2019RG000675, https://doi.org/10.1029/2019RG000675, 2020.
Nordling, K., Korhonen, H., Räisänen, J., Partanen, A.-I., Samset, B. H., and Merikanto, J.: Understanding the surface temperature response and its uncertainty to CO2, CH4, black carbon, and sulfate, Atmos. Chem. Phys., 21, 14941–14958, https://doi.org/10.5194/acp-21-14941-2021, 2021.
Nzotungicimpaye, C.-M., MacIsaac, A. J., and Zickfeld, K.: Delaying methane mitigation increases the risk of breaching the 2 °C warming limit, Commun. Earth Environ., 4, 250, https://doi.org/10.1038/s43247-023-00898-z, 2023.
Ou, Y., Roney, C., Alsalam, J., Calvin, K., Creason, J., Edmonds, J., Fawcett, A. A., Kyle, P., Narayan, K., O'Rourke, P., Patel, P., Ragnauth, S., Smith, S. J., and McJeon, H.: Deep mitigation of CO2 and non-CO2 greenhouse gases toward 1.5 °C and 2 °C futures, Nat. Commun., 12, 6245, https://doi.org/10.1038/s41467-021-26509-z, 2021.
Palazzo Corner, S., Siegert, M., Ceppi, P., Fox-Kemper, B., Frölicher, T. L., Gallego-Sala, A., Haigh, J., Hegerl, G. C., Jones, C. D., Knutti, R., Koven, C. D., MacDougall, A. H., Meinshausen, M., Nicholls, Z., Sallée, J. B., Sanderson, B. M., Séférian, R., Turetsky, M., Williams, R. G., Zaehle, S., and Rogelj, J.: The Zero Emissions Commitment and climate stabilization, Front. Sci., 1, 1–26, https://doi.org/10.3389/fsci.2023.1170744, 2023.
Park, S.-W., Kim, J.-S., and Kug, J.-S.: The intensification of Arctic warming as a result of CO2 physiological forcing, Nat. Commun., 11, 2098, https://doi.org/10.1038/s41467-020-15924-3, 2020.
Pincus, R., Forster, P. M., and Stevens, B.: The Radiative Forcing Model Intercomparison Project (RFMIP): experimental protocol for CMIP6, Geosci. Model Dev., 9, 3447–3460, https://doi.org/10.5194/gmd-9-3447-2016, 2016.
Rao, S. and Riahi, K.: The Role of Non-CO3 Greenhouse Gases in Climate Change Mitigation: Long-term Scenarios for the 21st Century, The Energy J., 27, 177–200, 2006.
Richardson, T. B., Forster, P. M., Smith, C. J., Maycock, A. C., Wood, T., Andrews, T., Boucher, O., Faluvegi, G., Fläschner, D., Hodnebrog, Ø., Kasoar, M., Kirkevåg, A., Lamarque, J.-F., Mülmenstädt, J., Myhre, G., Olivié, D., Portmann, R. W., Samset, B. H., Shawki, D., Shindell, D., Stier, P., Takemura, T., Voulgarakis, A., and Watson-Parris, D.: Efficacy of Climate Forcings in PDRMIP Models, J. Geophys. Res.-Atmos., 124, 12824–12844, https://doi.org/10.1029/2019JD030581, 2019.
Schwinger, J. and Tjiputra, J.: Ocean Carbon Cycle Feedbacks Under Negative Emissions, Geophys. Res. Lett., 45, 5062–5070, https://doi.org/10.1029/2018GL077790, 2018.
Schwinger, J., Tjiputra, J. F., Heinze, C., Bopp, L., Christian, J. R., Gehlen, M., Ilyina, T., Jones, C. D., Salas-Mélia, D., Segschneider, J., Séférian, R., and Totterdell, I.: Nonlinearity of Ocean Carbon Cycle Feedbacks in CMIP5 Earth System Models, J. Climate, 27, 3869–3888, https://doi.org/10.1175/JCLI-D-13-00452.1, 2014.
Tanaka, K., Boucher, O., Ciais, P., Johansson, D. J. A., and Morfeldt, J.: Cost-effective implementation of the Paris Agreement using flexible greenhouse gas metrics, Sci. Adv., 7, eabf9020, https://doi.org/10.1126/sciadv.abf9020, 2021.
Thornhill, G. D., Collins, W. J., Kramer, R. J., Olivié, D., Skeie, R. B., O'Connor, F. M., Abraham, N. L., Checa-Garcia, R., Bauer, S. E., Deushi, M., Emmons, L. K., Forster, P. M., Horowitz, L. W., Johnson, B., Keeble, J., Lamarque, J.-F., Michou, M., Mills, M. J., Mulcahy, J. P., Myhre, G., Nabat, P., Naik, V., Oshima, N., Schulz, M., Smith, C. J., Takemura, T., Tilmes, S., Wu, T., Zeng, G., and Zhang, J.: Effective radiative forcing from emissions of reactive gases and aerosols – a multi-model comparison, Atmos. Chem. Phys., 21, 853–874, https://doi.org/10.5194/acp-21-853-2021, 2021.
Tokarska, K. B., Gillett, N. P., Arora, V. K., Lee, W. G., and Zickfeld, K.: The influence of non-CO2 forcings on cumulative carbon emissions budgets, Environ. Res. Lett., 13, 034039, https://doi.org/10.1088/1748-9326/aaafdd, 2018.
WCRP (World Climate Research Programme): Coupled Model Intercomparison Project (Phase 6), ESGF [data set], https://esgf-node.llnl.gov/search/cmip6/ (last access: 6 July 2022), 2022.
Williams, R. G., Katavouta, A., and Goodwin, P.: Carbon-Cycle Feedbacks Operating in the Climate System, Curr. Clim. Change Rep., 5, 282–295, https://doi.org/10.1007/s40641-019-00144-9, 2019.
Zhu, X., Zhuang, Q., Gao, X., Sokolov, A., and Schlosser, C. A.: Pan-Arctic land–atmospheric fluxes of methane and carbon dioxide in response to climate change over the 21st century, Environ. Res. Lett., 8, 045003, https://doi.org/10.1088/1748-9326/8/4/045003, 2013.
Zickfeld, K., Eby, M., Matthews, H. D., Schmittner, A., and Weaver, A. J.: Nonlinearity of Carbon Cycle Feedbacks, J. Climate, 24, 4255–4275, https://doi.org/10.1175/2011JCLI3898.1, 2011.
Zickfeld, K., MacDougall, A. H., and Matthews, H. D.: On the proportionality between global temperature change and cumulative CO2 emissions during periods of net negative CO2 emissions, Environ. Res. Lett., 11, 055006, https://doi.org/10.1088/1748-9326/11/5/055006, 2016.