the Creative Commons Attribution 4.0 License.
the Creative Commons Attribution 4.0 License.
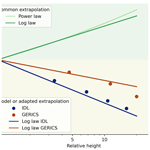
Extrapolation is not enough: impacts of extreme land use change on wind profiles and wind energy according to regional climate models
Peter Hoffmann
Daniela C. A. Lima
Marcus Breil
Olivier Asselin
Diana Rechid
Humans change climate in many ways. In addition to greenhouse gases, climate models must therefore incorporate a range of other forcings, such as land use change. While studies typically investigate the joint effects of all forcings, here we isolate the impact of afforestation and deforestation on winds in the lowermost 350 m of the atmosphere to assess the relevance of land use change for large-scale wind energy assessments. We use vertically resolved sub-daily output from two regional climate models instead of extrapolating near-surface winds with simplified profiles. Comparing two extreme scenarios, we report that afforestation reduces wind speeds by more than 1 m s−1 in many locations across Europe, even 300 m above ground, underscoring its relevance at hub heights of current and future wind turbines. We show that standard extrapolation with modified parameters approximates long-term means well but fails to capture essential spatio-temporal details, such as changes in the daily cycle, and it is thus insufficient to estimate wind energy potentials. Using adjacent climate model levels to account for spatio-temporal wind profile complexity, we report that wind energy capacity factors are strongly impacted by afforestation and deforestation: they differ by more than 0.1 in absolute terms and up to 50 % in relative terms. Our results confirm earlier studies showing that land use change impacts on wind energy can be severe and that they are generally misrepresented with common extrapolation techniques.
- Article
(9229 KB) - Full-text XML
-
Supplement
(13151 KB) - BibTeX
- EndNote
The last 2 decades have seen substantial progress in the deployment of renewable energy, in terms of both technological advances and reduced costs (IRENA, 2022). However, ambitious climate change mitigation requires further deep and rapid emission reductions, ultimately reaching net-zero or net-negative emissions (IPCC, 2022). This objective drives numerous countries to pursue a significant expansion of their renewable energy capacity. Ambitious goals are widespread across Europe: the European Union strives for carbon neutrality by 2050 (Commission, 2021), Switzerland targets climate neutrality by 2050 (Confederation, 2022), and the United Kingdom aims for net-zero emissions by 2050 (UK, 2008). The European power system will therefore dominantly rely on weather-dependent power generation from wind and solar energy in just a few years. This reliance means that we need to better understand renewable resources, including how they might be affected by human activity, to guarantee the reliability of our future power systems.
Concern about adverse impacts of climate change on wind energy potentials has motivated a range of studies (e.g., Hueging et al., 2013; Tobin et al., 2016; Reyers et al., 2016; Moemken et al., 2018; Karnauskas et al., 2018; Schlott et al., 2018; Soares et al., 2019; Lima et al., 2021; Hahmann et al., 2022). Drawing from those papers and other published peer-reviewed literature, a recent review (Pryor et al., 2020) and the Sixth Assessment Report of the IPCC (IPCC, 2022) concludes that climate change will only weakly impact the wind resource and that it remains unclear in most locations whether winds will be amplified or weakened. However, there are many possible interpretations of this conclusion, including (i) that climate change will indeed only have limited impacts on wind energy, (ii) that climate models are deficient in capturing dynamical changes and therefore underestimate the real risks, (iii) that methodological flaws in the conversion of climate model output to wind power generation hinder signal detection, and (iv) that the effects of different aspects of human activity happen to cancel out.
It is worthwhile to consider option (ii) because strong reliance on structurally similar models can be problematic if inter-model agreement is mistakenly interpreted as evidence for reliability (e.g., Thompson, 2022). Current climate models are structurally similar. On the one hand, this is because they all approximate the same climate system obeying the same physical laws, providing a good reason for structural similarity. On the other hand, coupled climate models also share sub-components, such as the same atmospheric or ocean model, or they are derived from a common older predecessors (Knutti et al., 2013), which introduces structural similarity that has practical justification but lacks physical justification. More importantly, however, current models have documented deficiencies in capturing important aspects of atmospheric dynamics. For instance, the storm track is generally positioned too close to the Equator and is oriented too much along the east–west direction, which might be an artifact of the current resolution in global climate models (Schemm, 2023). The observed late-winter jet stream variability on multidecadal scales is also not realistically captured in current climate models, likely due to issues in the ocean–atmosphere coupling (Simpson et al., 2018). Moreover, climate models underestimate the importance of multidecadal variability in the mid-latitudes (O’Reilly et al., 2021), potentially implying that important processes are not well captured and calling into question the fitness for purpose of current climate models for wind energy assessments.
Given these issues, it makes sense to unpack the problem step by step. In this study, we therefore ignore dynamical changes caused by altered greenhouse gas and aerosol concentrations and instead explore options (iii) and (iv). A potential methodological flaw is the conversion of winds from near-surface to hub height using extrapolation techniques. Indeed, Soares et al. (2020) report that extrapolation introduces sizable errors, with discrepancies of up to 30 % during the historical period compared to using model level information from ERA5. Despite this, most of the above-cited climate change literature extrapolates with a wind profile that even remains constant over the 21st century, with two notable exceptions. First, Hahmann et al. (2022) derive North Sea hub height winds from model levels and report that the power law performs poorly in comparison. This result is striking because unlike over land, the lower boundary condition of the North Sea does not change directly as a function of the prescribed scenario but only indirectly via changes in sea state. Over land, the Climate Model Intercomparison Project (CMIP; Taylor et al., 2012; Eyring et al., 2016) scenarios include substantial changes in land use during the 21st century (Hurtt et al., 2011, 2020). Onshore wind profiles may thus change more drastically, suggesting that the power law is even more ill-suited than offshore. Second, Wohland (2022) restricted his analysis to surface winds and consequently did not require a conversion to hub height. However, he found land use change to cause systematic large-scale differences between future winds projected by regional climate models from EURO-CORDEX (Jacob et al., 2014) and driving global climate models from CMIP5 (Taylor et al., 2012) that are caused by land use change.
The effects of land use change and dynamical changes on winds indeed cancel out in some scenarios. Analyzing the MPI Grand Ensemble and estimating the dynamical change from idealized simulations without land use change, Wohland et al. (2021b) show that global mean onshore wind speed would drop by about −0.25 m s−1 due to dynamical changes, whereas it would increase by about 0.25 m s−1 because of land use change, yielding zero net change. These numbers compare the end of the 21st century under the RCP8.5 scenario with the beginning of the historical period. It remains unclear, however, how this balance of effects evolves with height, although it appears plausible that the dynamical effects would dominate since the land use effects are expected to decay with height.
In this study, we isolate the effect of afforestation and deforestation on wind energy potentials. We do so by analyzing high-resolution regional climate model output, which enables us to compute hub height wind speeds without relying on constant parameter extrapolations. Our approach thus avoids the highlighted potential methodological flaw in published literature. Using experiments that only differ in the lower boundary condition allows us to investigate the effect of land use independently from the effects of other climate forcings, thereby enabling better understanding of the different effects that may cancel each other out in combined scenarios.
We use data from the coordinated regional climate model inter-comparison project “Land Use and Climate Across Scales” (LUCAS; see Davin et al., 2020). LUCAS is a World Climate Research Programme (WCRP) flagship pilot study that investigates the biogeophysical effects of land use changes in Europe (e.g., Breil et al., 2020; Sofiadis et al., 2022; Mooney et al., 2022; Daloz et al., 2022) and North America (Asselin et al., 2022). Within LUCAS, experiments with extreme land use change scenarios were conducted from which we quantify the maximum effects that land use change may have on future wind energy resources. In the GRASS scenario, all suitable areas are covered by grass, whereas in the FOREST scenario, all suitable areas are covered by forests.
We report wind speed differences between the GRASS and FOREST simulations as
That is, positive differences correspond to stronger winds in the GRASS scenario as compared to the FOREST scenario. We refer to Δs as the effect of deforestation. Since the effect of deforestation is the opposite effect of afforestation and both share the same absolute values, we use the terms interchangeably when discussing the magnitude of change.
2.1 Model subset
To accurately evaluate the effect of deforestation and afforestation on wind energy, we need resolved model output with high temporal and vertical resolution. Temporal resolution is crucial because wind energy potential depends nonlinearly on wind speeds, and vertical resolution is imperative as wind turbines roughly populate the lowermost 300 m of the atmosphere. In particular, we use models that
-
provide sub-daily winds,
-
make model level information available,
-
have at least three atmospheric model levels in the lowermost 350 m,
and we discard the rest.
Based on these criteria, we identify two suitable models out of six models that were provided by LUCAS member groups. The suitable models are REMO-iMOVE run by the Climate Service Center Germany (GERICS) and WRFa-NoahMP run by Institute Dom Luiz (IDL); see Table 1 for an overview. Throughout this paper, we refer to the model output by naming the institution (i.e., GERICS or IDL). Specifics of the model setups like the lateral boundary formulation or the boundary layer scheme are documented in Table 1 in Davin et al. (2020). We acknowledge that an ensemble size of two is limited. However, it is the best currently available database for this analysis. Even with only two models, the results of this study are relevant because we are the first to systematically investigate land-use-driven changes in winds, wind profiles, and wind energy potentials using regional climate models.
2.2 Resolution
Both models have a horizontal resolution of 0.44° covering Europe on a grid with a rotated pole, following the standard CORDEX domain definitions (see EUR-44 in CORDEX, 2015). This resolution corresponds to about 50 km. Temporal resolution is sub-daily for both models. IDL provides hourly output while GERICS produces output every 6 h.
We use the lowermost three (four) model levels for GERICS (IDL), corresponding to approximate heights at 30, 140, and 340 m (28, 95, 190, 300 m). The approximate heights are computed by subtracting orography from the geopotential height fields averaged over the entire domain and a full year. The exact height above ground of a model level varies in time and space due to the use of terrain-following hybrid vertical coordinates. These variations are taken into account in the wind power conversion (see Sect. 2.6). We ensured, however, that the approximate heights can be used as a reasonable proxy for the exact heights in the context of long-term changes because the variations are relatively small. For example, the lowest GERICS model level sits 28.1 m above ground on average. The temporal standard deviation averaged over all locations is 0.8 m, and the spatial standard deviation averaged over all time steps is 0.7 m. That is, the variations in the level height are on the order of a few percent, as expected, since they originate from atmospheric pressure and density differences, which are also small compared to their means. The total number of vertical levels is 50 in the IDL simulations, which is substantially more than the 27 levels in the GERICS simulations (see Table 1 in Davin et al., 2020, for additional details).
Vegetation is parameterized in the IDL and GERICS models, and it impacts the atmosphere via changes in surface parameters like roughness length and albedo. Parameterization is needed because explicit physical modeling of individual trees would require substantially finer grid resolution than the 50 km grid spacing available from LUCAS. One implication of the parameterization and large grid box sizes is that trees cannot directly impact atmospheric flow in higher atmospheric levels even if very tall real trees exceed 30 m and would thus reach into the second model level. As shown in this paper, however, trees do impact atmospheric flow further up via surface changes that are mediated upwards.
2.3 Area and period of interest
To compute onshore winds, we use data over the European continent extending east up to approximately Ankara, Türkiye (see Fig. S10 for details). We only use grid boxes that are contained in both datasets. Since the GERICS simulations contain a larger sponge zone, we use only 106×103 of the available 129×121 grid boxes.
We compute mean wind speeds by averaging over model years from 1986 to the end of 2015 as suggested by the LUCAS consortium, addressing changes in the annual means and the seasonal and daily cycles. When comparing the daily cycles, we follow a downsampling rather than an averaging approach: to compare the GERICS output (00:00, 06:00, 12:00, 18:00; all times UTC) with the IDL output (00:00, 01:00, 02:00, etc.; all times UTC), we only consider time steps that are available in both datasets and ignore the additional information contained in IDL at the other output times. We argue that this approach is most meaningful because wind speeds are reported as instantaneous values and the choice of output frequency does not affect the output. By contrast, we use data at the highest available frequency to calculate wind power, namely hourly in IDL and 6-hourly in GERICS.
2.4 Preprocessing the horizontal and vertical coordinates
GERICS reports wind components u and v at the horizontal grid box edges, and we interpolate them linearly to obtain wind components at the grid box centers. Moreover, geopotential and horizontal wind components are reported at different vertical levels that are separated by half a grid box in the vertical. After consultation with the modelers, we decided to interpolate the geopotential linearly to the vertical position of the winds. These two steps allow us to compute wind speeds at the same horizontal locations as in the IDL simulations and with known elevation above ground. The implementation is documented in preprocess_GERICS.py, which is part of the GitHub repository belonging to this publication (Wohland, 2024).
The IDL model output was already post-processed to provide wind components and geopotential height at the same vertical levels and horizontal coordinates before the data were handed over to us. We therefore did not perform a postprocessing as outlined above, but we instead used the data as provided.
2.5 Comparison with the log and power laws
We do not rely on heuristics to extrapolate from near-surface winds to hub heights because we build on models that provide winds at multiple heights in the relevant domain. However, we compare the results obtained from the LUCAS models with standard extrapolations to gain insights into the errors made by the extrapolation. Specifically, we compare against the log and power law, which are dominantly used in the literature (e.g., Bloomfield et al., 2016; Tobin et al., 2016; Wohland et al., 2017; Schlott et al., 2018; Soares et al., 2019; van der Wiel et al., 2019; Pryor et al., 2020).
The log law relates winds s at heights z and z′ and location x via the roughness length z0 and the displacement height d as
The power law only depends on the power law exponent α:
The log law can be formally derived as a special case of the general equations of motion under neutral stratification, while the power law is empirically motivated (e.g., Emeis, 2013). We highlight the location and time dependency in Eqs. (2) and (3) to illustrate two assumptions of these approaches that limit their applicability. First, both approaches assume that the wind profile is constant in time because the right-hand side has no time dependency. Second, the power law generally does not include a spatial dependency of the wind profile, although some studies use different values for α over land and over ocean to partially address this issue (e.g., Hueging et al., 2013).
When comparing model level results with extrapolations (in Fig. 3), we use the widely adopted power law exponent . To compute the log law profiles, we use the roughness lengths reported in Breil et al. (2020) for IDL, assuming a split between coniferous and deciduous trees. The GERICS values from that paper, however, cannot be directly used because the contribution from subgrid-scale orography is missing. We therefore computed onshore mean effective roughness lengths from the climate model (FOREST: zo=1.686 m; GRASS: zo=0.693 m) and use those values. We set displacement height d=0 m in the log law plots in Fig. 3.
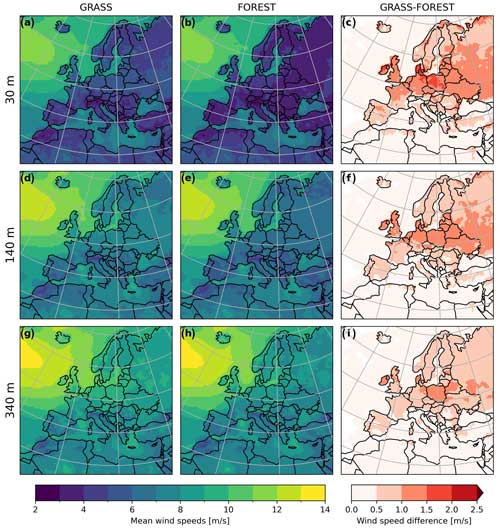
Figure 1Mean wind speeds in the GRASS (a, d, g) and FOREST (b, e, h) scenario, as well as their difference (c, f, i), based on the GERICS simulations. Data are plotted for the lowermost three model levels, which have approximate heights of 30, 140, and 340 m, respectively.
2.6 Wind power conversion
Despite using the model level output, we need to perform some corrections as the model layers do not perfectly match the turbine hub height. We interpolate rather than extrapolate, allowing for spatio-temporal variations of the wind profile. Specifically, we compute a temporally and spatially evolving coefficient based on Eq. (2) from the adjacent levels as
where zabove and zbelow are the height of the layer above and below hub height, respectively. Those heights are also a function of time and location, but we do not highlight this dependency in the equation to ease legibility.
We then apply the obtained coefficient to interpolate to hub height using Eq. (2). In Supplement Fig. S1, we show an illustration of the approach for a few locations, highlighting that α′ is far from constant and instead varies vastly between bounds of about −0.2 to 0.6. This range includes the widely used value of . However, the large scatter around that value indicates that a constant value does not capture the complexity of wind profiles.
In a last step, we multiply hub height wind speeds with the power curve of a SWT120-3600 turbine to obtain capacity factor (CF) timeseries. The power curve is taken from the windpowerlib database (Haas et al., 2019). This turbine has a hub height of 90 m and was chosen following Wohland et al. (2021a) as it represents the median current wind turbine in terms of its capacity factor at 7 m s−1. Please note that we ignore turbine-to-turbine and park-to-park interactions like wake effects, wind farm blockage, and resource depletion by upstream wind parks in this study. We also do not model turbine damage and aging caused by changes in the wind resource, such as changes in gusts. While we acknowledge that these effects matter for wind energy yield assessments, capturing them is beyond the scope of the current study and would require a different and more highly resolved model setup.
In line with physical expectation, the effect of forests is strongest near the surface where momentum is removed from the atmospheric flow and weakens with height, ultimately becoming irrelevant in the free troposphere. Our core finding is that afforestation or deforestation matters for winds around hub height according to both models. For instance, wind speeds drop by more than 1 m s−1, even 340 m above ground in large parts of Poland, Ireland, and Belarus in the GERICS simulations, and they drop by at least 0.5 m s−1 virtually everywhere else on the continent (Fig. 1c, f, and i). In fact, wind speed drops greater than 1 m s−1 occur in almost half of the onshore grid cells at 30 m and in 10 % of grid cells at 340 m above ground (Table 2). IDL features reductions of a similar magnitude, even exceeding 1.5 m s−1 in large parts of Scandinavia and Iceland near the surface (Fig. 2c). These wind speed reductions slowly decay with height (Fig. 2c, f, i, and l). About 25 % of onshore grid cells still experience reductions greater than 1 m s−1 at 190 m above ground, dropping to 14 % at 300 m (Table 2).
Interestingly, the models also agree that deforestation has non-local effects on wind speeds, namely by impacting offshore winds. While a clear land–sea divide exist in both models and at all levels (e.g., Figs. 1c and 2c), mean offshore winds decrease by 0.34 m s−1 at 190 m and by 0.26 m s−1 at 140 m according to IDL and GERICS, respectively (Table 2).
While the models broadly agree on the magnitude of wind speed changes, they disagree considerably about where maximum wind speed occurs. For example, GERICS positions the offshore wind speed maximum northwest of the UK, while IDL locates it much further east. The models also disagree with respect to the location of maximum wind changes. While GERICS winds decline most strongly in a band ranging from the UK to Poland via the Benelux and Germany, IDL positions the center of change around Scandinavia, highlighting significant model uncertainty. Among the numerous factors influencing model uncertainty, two are of particular relevance here, namely (i) how the models (or modelers) translate the scenario into actual boundary conditions and (ii) how the models respond to those boundary conditions. For instance, in the FOREST scenario, forests grow in all suitable locations, but models can disagree as to whether an area is suitable and whether to include any subgrid-scale information. In particular, the GERICS simulations follow a tile approach that allows for combinations of different land uses within a grid box, whereas IDL adopts a majority approach where each grid box has exactly one land use type (Davin et al., 2020). The inter-model difference manifests, for example, in the lowest model level over the Alps, where the signal in GERICS is much weaker than in IDL (cf. Figs. 1c and 2c). This is because alpine land cover changes are small in the GERICS simulations due to the high bare-ground fraction. Additionally, disagreement can stem from the allocation of land surface parameters (e.g., roughness length and leaf area index) and whether and how those parameters evolve throughout the year.
To test the robustness of change in different parts of the year, we analyzed the changes per season (see Supplement Figs. S2–S9). Overall, we find qualitatively similar changes in all seasons, but the amplitude of change is modulated. In particular, the summer signal (June–July–August) is weaker than the winter signal (December–January–February).
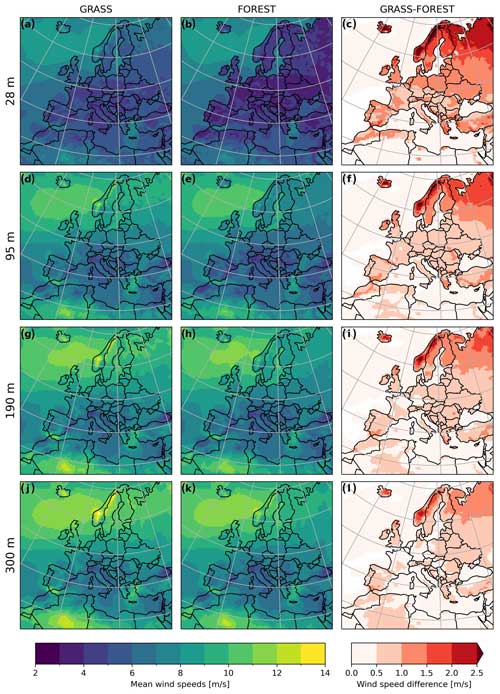
Figure 2Mean wind speeds in the GRASS and FOREST scenario, as well as their difference, based on the IDL simulations. Data are plotted for the four lowermost model levels, which have approximate heights of 28, 95, 190, and 300 m, respectively.
3.1 Onshore mean signal decay with height
Given that extrapolation from 10 m winds is the most common approach in wind-energy-related climate research, we now address the discrepancy between extrapolation and explicit modeling. Figure 3 shows the evolution of near-surface wind speed change with height. It documents qualitatively different results, where some approaches show that the surface perturbation grows with height, while others show the opposite.
Surface change grows with height when using the power or log law with the same profile in the GRASS and FOREST scenarios (i.e., following Eqs. 2 and 3 with constant , z0=0.05 m corresponding to grass, and d=0 m). The reason is simple: with the same profile, the near-surface winds in both scenarios are scaled with the same monotonically increasing profile, and it follows that the difference between the two also increases monotonically with height.
By contrast, the model output suggests that the surface change decays with height, in line with physical expectation. The seeming contradiction between extrapolation and model output can be reconciled by using modified extrapolation parameters for the GRASS and FOREST experiments. Using the model specific roughness lengths (see Sect. 2.5), we find good agreement between the explicitly modeled decay and the extrapolated decay.
These results have practical implications because CMIP scenarios for the 21st century contain substantial land use change. Our results mean that land use change impacts on hub height winds are exaggerated when using the same scaling with different lower boundary conditions. For instance, 10 m wind speeds are projected to decrease by up to 0.5 m s−1 in eastern Europe in the RCP4.5 climate change scenario by the end of the 21st century due to changes in the lower boundary condition (Wohland, 2022). Following Fig. 3, this near-surface reduction is amplified by about 40 % to 0.7 m s−1 at 100 m above ground using the unmodified log or power law. By contrast, it is reduced by about a third according to the GERICS and IDL simulations to 0.35 m s−1. In other words, ignoring the change in the wind profile leads to an overestimation by a factor of 2 at hub height. Since dynamical changes, e.g., from modified storm tracks or altered temperature gradients, are not scaled in a similar fashion, it follows that the detection of climate change signals for hub height winds might be hampered, as the relative role of dynamical changes is underestimated.
Nevertheless, the results show that the decay modeled by GERICS and IDL is fairly linear in log space and can thus be approximated well by the log and power law on average over all seasons, 30 years, and an entire continent. We will explore in the rest of the paper whether the modified extrapolations are also suitable at specific locations and seasons, and whether it correctly modifies quantities that depend nonlinearly on wind speeds.
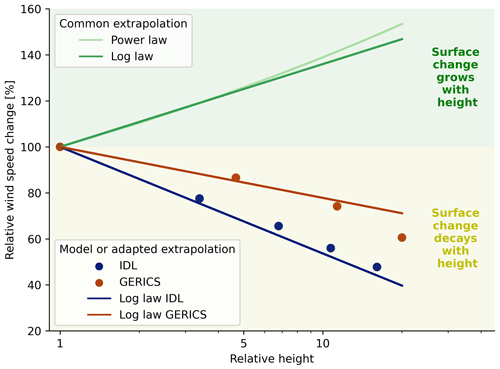
Figure 3Evolution of relative wind speed change with height according to common extrapolations (green lines), explicit regional climate models (circles), and adapted extrapolation (brown and blue lines). Wind speed change between FOREST and GRASS is computed as an average over all onshore locations and 30 years. It is expressed relative to the change observed at the lowermost model level (ca. 30 m). The upward-sloping green lines with changes greater than 100 % mean that the wind speed drop grows relative to the surface drop, while the downward-sloping lines indicate wind recovery with height. The common extrapolations use fixed values for the power and log law (z0=0.05 m, α=0.143), mimicking current common practice. By contrast, the adapted extrapolations use different roughness lengths in FOREST and GRASS (see Sect. 2.5 for details).
3.2 Wind change profiles per season at strongly, normally, and weakly impacted locations
Figure 4 shows wind speed changes per height for different seasons and locations. We choose locations based on the amplitude of surface wind speed change, focusing on the 90th, 50th (median), and 10th percentiles. That is, we analyze three locations where winds are changed strongly, normally, and weakly by afforestation or deforestation for each model.
To ensure that our results are representative of a range of similar locations rather than artifacts of a single location, we draw random 10-member samples from a centered percentile range with a width of 10 percentiles. For instance, the 90th percentile corresponds to a 10-member draw from the percentile range between the 85th and 95th percentile, and the same approach is used for the other cases (i.e., 50th percentile: 45th–55th percentile; 10th percentile: 5th–15th percentile). We report the mean change per season and model together with error bars representing the standard error of the mean (i.e., , where σ is the standard deviation of wind speed change across the 10 locations from each percentile band and N=10 is the sample size).
Both models agree that winter changes are strongest and summer changes weakest (even though some exceptions exist). The models also agree that wind speed changes decay monotonically with height, although exceptions exist during the summer, where IDL projects a local minimum followed by increased change (Fig. 4b and c). Error bars are small compared to the difference between seasons, vertical levels, and models, indicating the robustness of the presented results to sampling different yet similar locations. In many cases, the error bars do not even extend beyond the marker for the mean value.
While agreement on the amplitudes of wind speed changes 30 m above ground is high in the 50th percentile (Fig. 4b and e) and in the 90th percentile in summer (Fig. 4a and d), the models disagree strongly on other aspects. For instance, winter changes in the 90th percentile are twice as strong in IDL compared with GERICS (Fig. 4a and d), and summer changes in the 10th percentile in IDL drop below zero at 300 m, whereas they only marginally reduce from the near-surface value in GERICS (Fig. 4c and f). In other words, model uncertainty is high at individual locations.
Comparing seasons reveals a strong seasonal dependency in IDL and a weaker but noticeable one in GERICS. The strongly impacted location features convergence from vastly different values near the surface to a similar range further up (Fig. 4a). Surface changes are very high in winter (ca. 3 m s−1), high in the annual mean and spring (ca. 2 m s−1), medium in fall (ca. 1.75 m s−1), and comparably low in summer (ca. 1.2 m s−1). By contrast, wind speed change is approximately 0.8 m s−1 at the highest displayed level (around 750 m) in all seasons.
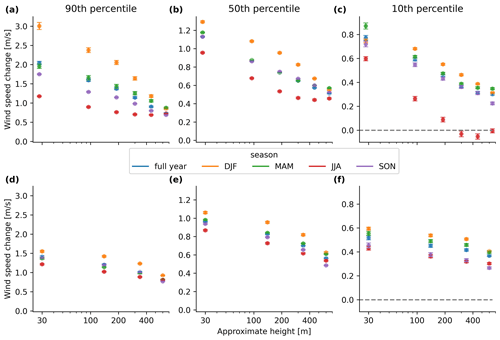
Figure 4Wind profile changes between the GRASS and FOREST simulations over land according to IDL (upper row, a–c) and GERICS (lower row, d–f). Results are given for the full year, and individual seasons, respectively. The first (a, d) and last column (c, f) show wind speed change at a location that is strongly or weakly impacted by afforestation. The second column shows changes the median (50th percentile) location (b, e). Points denote the mean over 10 randomly sampled locations in a centered percentile band with a width of 10 percentiles, and error bars denote the standard error of the mean.
3.3 Case study: daily cycle of wind change profiles during different months
Besides the seasonal cycle, we expect changes in the daily cycle because sub-daily alterations in atmospheric stability and boundary layer height modulate the importance of the surface for upper-level winds. As an example, we analyze the daily cycle near Bielefeld in central Germany (see Fig. S11 for a map) during different months and report that the change signal indeed depends on the time of day and season (see Fig. 5).
In particular, we find that the surface perturbation decays slowly around noon and decays relatively quickly at night during all months in IDL (Fig. 5d, h, l, and p) and during all months but October in GERICS (Fig. 5b, f, j, and n). This daily cycle is consistent with physical expectation: increased mixing around noon implies that the near-surface wind reductions impact higher level winds more strongly. Similarly, more stable nighttime conditions imply that the near-surface wind reductions are more constrained to the surface. While models roughly agree on time of day dependence, they disagree somewhat on the seasonal evolution. For instance, April changes are strong for GERICS but weak for IDL.
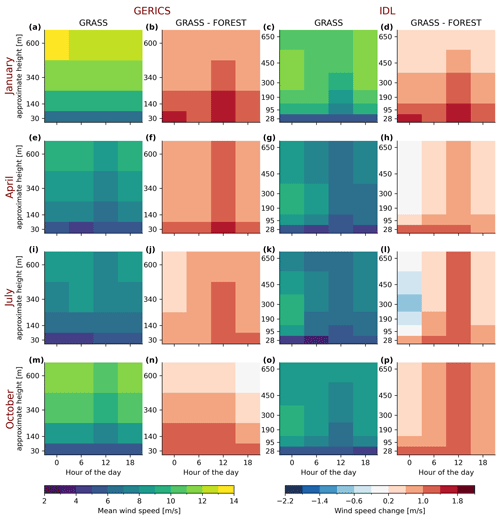
Figure 5Wind speed profiles per hour of the day and month averaged over 2×2 grid boxes near Bielefeld, Germany. The first (second) and third (fourth) column display the wind speed (change) at model grid levels for GERICS and IDL, respectively. For example, the yellow box in (a) means that winds in January at 00:00 UTC and 600 m above ground are between 13 and 14 m s−1 in the GERICS GRASS simulations. Absolute wind speeds are shown according to the color bar displayed on the lower left, while changes in wind speeds follow the color bar shown on the lower right. IDL data, which have hourly resolution, is only sampled every 6 h to match the temporal resolution of GERICS.
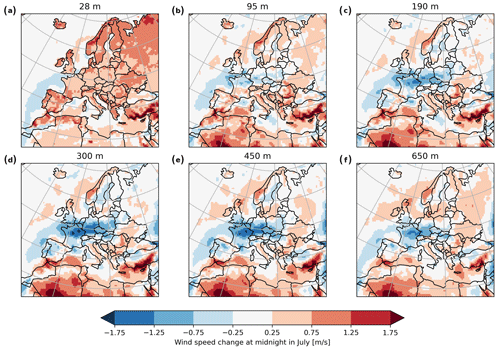
Figure 6Wind speed change at the IDL model levels evaluated at midnight (00:00 UTC) in July. Change is computed as GRASS − FOREST. Red values correspond to higher wind speeds in GRASS compared to FOREST, and blue values correspond to higher winds in FOREST compared to GRASS. Subplot titles give the approximate height per model level, increasing from (a) to (f).
Moreover, changes in the daily cycle are more varied in IDL than in GERICS. For example, GERICS April changes are indistinguishable at 0, 6, and 18 h (Fig. 5f), while there are clear differences at those hours in IDL (Fig. 5h). Similarly, October GERICS changes are identical at 0, 6, and 12 h, while IDL shows quick decay with height at 0 h, less decay at 6 h, and almost no decay at 12 h.
In addition, IDL features a local wind speed maximum at around 190 to 300 m at midnight in April, July, and October in the GRASS simulations (Fig. 5g, k, and o) that does not exist in GERICS (Fig. 5e, i, and m). This local maximum even gains additional strength in July from afforestation: the wind speed difference is between −0.4 and −0.8 m s−1 (Fig. 5l). In other words, IDL projects that wind speeds increase in the FOREST as compared the GRASS simulations at midnight in July. We will explore this unexpected feature and evaluate whether it is a singularity at this location in Sect. 3.4.
Comparing the climatologies, we find that higher-altitude winds generally blow stronger in GERICS than IDL (e.g., Fig. 5a vs. c). This is consistent with Breil et al. (2020), who found that IDL is warmer than GERICS and is therefore expected to feature more mixing and reduce wind speeds further aloft.
We analyzed additional locations in Spain and Sweden and report that those also feature complex responses of the wind profile (see Supplement Figs. S12 and S13), strengthening the conclusions drawn from the German case study. While some effects co-occur at all three locations, such as particularly strong upper-level changes in IDL at noon, other effects are unique for the respective sites, suggesting that explicit modeling of the vertical wind field structure is needed.
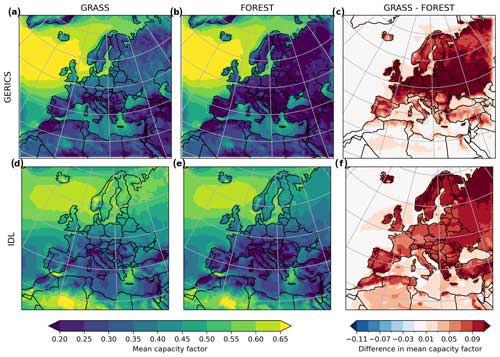
Figure 7Mean capacity factors for the SWT120-3600 turbine in the GRASS (a, d) and FOREST(b, e) simulations, as well as the GRASS–FOREST difference(c, f) in capacity factors. Note that the domain sizes are slightly different because the GERICS simulation provides a few additional grid boxes outside the core domain.
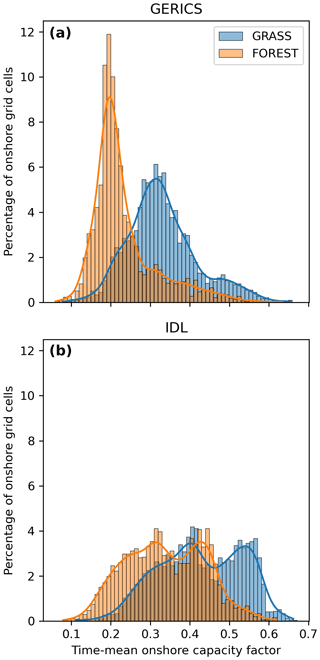
Figure 8Distribution of European onshore capacity factors by model (a vs. b) and experiment (blue vs. orange). Values are normalized such that the sum over all bins equals 1.
Overall, we conclude that changes in the wind profile caused by afforestation or deforestation are highly complex. Constant extrapolation from the surface values would miss essential features of changes in the daily cycle and is therefore not well suited to compute sub-daily hub height wind speeds needed for wind power conversion.
3.4 Formation and extent of the summer nocturnal low-level jet in the IDL response
The higher wind speeds in FOREST as compared to GRASS are a large-scale phenomenon rather than an artifact of the selected case study location (see Fig. 6). While the wind speed difference is always positive at the lowest level at 28 m, a band of negative values begins to emerge at 95 m, strengthens up to 450 m, and decays aloft. The band covers large parts of central Europe, including France, the Benelux, Germany, the Czech Republic, and Poland. It reaches values of up to −1.75 m s−1, which is considerable given that it occurs in summer when mean wind speeds are low (around 7 m s−1 at 95 m and 8 m s−1 at 190 m; see Fig. S8d and g).
The vertical extent of this anomaly and its occurrence at nighttime in summer are in line with observed nocturnal low-level jets (e.g., Weide Luiz and Fiedler, 2022) that occur as a consequence of decoupling of winds from the surface layer due to a temperature inversion. This nocturnal jet sits at a height that is relevant for wind energy. Crucially, it would be missed using the simple log or power law scaling, calling for explicit dynamical atmospheric modeling.
We can only speculate why the jet only occurs in IDL and not in GERICS. In addition to differences in the boundary layer schemes, one potential explanation is related to the temporal resolution that controls which processes can be explicitly modeled. The integration time step is 90 s for IDL vs. 240 s for GERICS, which could mean that important processes are not resolved in the coarser GERICS simulations.
Moreover, the difference could also be related to the output frequency because nocturnal low-level jets are rather short-lived, usually existing for a few hours. We cannot tell from the available output whether GERICS captured the jet with a small temporal offset of a few hours. For example, if a jet occurred in the GERICS simulation at 02:00 and lasted until 05:00 UTC, we would not be able to observe it from the 00:00 and 06:00 UTC time steps.
We propose a potential process-based explanation related to atmospheric stability. During the day, the sun heats the surface, which releases some of the heat into the atmosphere as sensible heat fluxes, thereby warming the boundary layer. These fluxes are significantly higher in IDL than in GERICS (Davin et al., 2020), resulting in a stronger daytime boundary layer heating. During the night, temperatures generally decrease more at the surface than in the boundary layer, due to the outgoing longwave radiation at the surface. The surface therefore cools the boundary layer from below, and temperatures increase with height, and thus a stable stratification evolves near the ground. As the boundary layer in IDL has warmed up more during the day, the nocturnal temperature gradient between the cooling surface and the atmosphere is greater than in GERICS, and the atmospheric stratification is therefore more stable, favoring the development of a nocturnal low-level jet. While this explanation is physically plausible and in line with previous results (e.g., Breil et al., 2020), a full analysis of the vertical column would be necessary to confirm this hypothesis, which is beyond the scope of the current study.
While we cannot robustly pinpoint a single explanation for the jet occurrence, our results emphasize the need for explicit modeling rather than extrapolation of surface winds, as low-level jets would generally not be captured using the log and power laws.
3.5 Afforestation impacts on wind power generation
Figure 7 provides maps of mean capacity factors (CFs). Both models feature a clear land–sea divide in both scenarios, but the absolute CFs differ between the models. While GERICS has a large area of very high offshore CFs, exceeding 0.65 northwest of the UK, IDL shows largest CF further to the east, and CFs almost never exceed 0.65 (see Fig. 7a and d). Over land, the models swap roles and GERICS CFs are generally lower than those from IDL.
We tested whether this inter-model difference is caused by the different output frequencies in combination with the nonlinear power curve. Power generation could be underestimated in calm onshore locations and overestimated in windy offshore locations when using 6-hourly output because the tails of the distribution are less well sampled compared to hourly output. However, we found that the IDL mean capacity factors are not substantially impacted by downsampling to 6-hourly resolution (Fig. S15), and thus we conclude that the output frequency difference does not explain the strong inter-model differences.
Irrespective of those differences, both models agree that afforestation substantially reduces CFs by more than 0.05 in most locations (Figs. 7c and e and 8). CF reductions even exceed 0.11 over approximately half of the continent in GERICS. That is, a single 3.6 MW turbine would produce about 3.5 GWh yr−1 less in the FOREST simulation. The reduction is particularly striking when considered in relative terms: the GRASS CF is more than 50 % higher than the FOREST CF in most European onshore locations (Fig. S14). The reductions are weaker in most locations in the IDL simulation, typically ranging between 0.07 and 0.11. Exceptions are Norway, northwestern Russia, southern Spain, and Türkiye, where IDL projects stronger reduction that is potentially related to the different suitability criteria for tree growth (Fig. 7). The combination of higher mean CFs and weaker changes implies that the relative reduction is markedly smaller in IDL compared to GERICS, particularly in northern Europe. However, drops still reach values of 40 % in southern Europe in IDL, which is far from negligible.
The mean CF distribution is wider for IDL than for GERICS (Fig. 8). For example, in the GERICS FOREST simulation, the majority of grid cells feature CFs between 0.15 and 0.25, and an individual 0.01 CF increment occurs in more than 10 % of the grid cells. By contrast, the IDL FOREST simulations cover values from 0.1 to 0.6 more evenly, and no single 0.01 CF increment occurs in more than 5 % of grid cells. Moreover, while GERICS features a single distinct peak in both experiments, IDL is weakly bimodal. The single peak in the GERICS GRASS distribution means that values around 0.3 occur most frequently. By contrast, the bimodal shape of the IDL distributions implies that CF of around 0.4 or around 0.55 in the GRASS simulation occurs more often than other values. While we are not aware of any a priori reason to expect one, two, or more peaks in the distribution of capacity factors, this qualitative difference in shape is another example of model uncertainty.
Using two regional climate model simulations from the WCRP CORDEX Flagship Pilot Study LUCAS (Davin et al., 2020), we have shown that afforestation substantially impacts winds and wind energy capacity factors. The influence of land use change extends through the entire lowermost 350 m, even exceeding 1 m s−1 at around 300 m, and it should therefore not be ignored in wind energy assessments.
Different parts of the day and year are affected differently, with implications for power system design. Although afforestation has a minimal impact on higher-level winds during the night, its effects are markedly stronger around noon, coinciding with the typical peak in current electricity demand. Similarly, winter winds are more strongly affected than summer winds, implying that the benefits of using wind power to compensate generation shortfalls from solar photovoltaics in winter might be compromised. Using a 3.6 MW wind turbine with 90 m hub height, we find that capacity factors are up to 50 % higher in the GRASS as compared to the FOREST scenario according to one model (GERICS) and up to 40 % according to the other model (IDL).
As the reported changes are complex, non-local, location-dependent, and affect both daily and seasonal cycles, they are therefore not well captured by simple heuristics like the log law and power law even when using modified parameters. Using the log and power law in simulations with substantially different land use with the same parameters is particularly detrimental because it overestimates the effects of land use change on hub height winds and might mask dynamical changes. We therefore strongly advise against scaling 10 m climate model output to hub heights with constant coefficients in the log or power law.
Instead, model level information could be utilized as in Hahmann et al. (2022) or in the present study. However, using model level outputs is generally more challenging because it requires in-depth knowledge about the structure of climate models (e.g., terrain-following vs. absolute vertical coordinates) and is more data-heavy for impact modelers. The provision of wind speeds interpolated to multiple heights in the 100 to 200 m band by climate modeling groups would therefore enable better climate (change) assessments for wind energy and help to overcome the disconnect between energy and climate modeling (Craig et al., 2022).
While covering Europe completely in forest or grass represent clear edge cases that are unlikely to happen in reality, strong land use changes do exist in the widely used CMIP simulations, for example, over eastern Europe in the representative concentration pathway 4.5 (e.g., Wohland, 2022). The results of this study thus have implications for climate change impact assessments based on CMIP or CORDEX.
The results in this study are restricted to two models that provided data at the required granularity in the vertical and time dimensions. Since we also report substantial model uncertainty, the exact values provided here should be treated with caution, even though the overall results are consistent and physically plausible. Future work, however, should focus on including more models and scenarios. We intend to complement this study with different models and more realistic scenarios from the second phase of the LUCAS experiment.
The code used within this paper is written in Python and openly available (Wohland, 2024). The regional climate model data from REMO-iMOVE and WRFa-NoahMP are available upon request (peter.hoffmann@hereon.de or diana.rechid@hereon.de). The data used within this paper are planned to be made available on World Data Center for Climate (WDCC) within the next few months.
The supplement related to this article is available online at: https://doi.org/10.5194/esd-15-1385-2024-supplement.
JW developed the research idea, implemented it, produced all of the figures, wrote the code, and drafted the manuscript. PH and DR gave feedback about the REMO model. OA and DL provided data. MB helped to contextualize the results. All authors reviewed and edited the manuscript and attended progress discussions.
The contact author has declared that none of the authors has any competing interests.
Publisher’s note: Copernicus Publications remains neutral with regard to jurisdictional claims made in the text, published maps, institutional affiliations, or any other geographical representation in this paper. While Copernicus Publications makes every effort to include appropriate place names, the final responsibility lies with the authors.
We would like to thank the wider LUCAS consortium for providing discussions, in particular Merja Tölle, Edouard Davin, Priscilla Mooney, Pedro Soares, and Eleni Katragkou. The authors gratefully acknowledge the WCRP CORDEX Flagship pilot study LUCAS (Land use and Climate Across Scales) and the research data exchange infrastructure and services provided by the Jülich Supercomputing Centre, Germany, as part of the Helmholtz Data Federation initiative.
Jan Wohland is part of the ETH Zurich SPEED2ZERO initiative, which received support from the ETH Board under the Joint Initiatives scheme. During the early stages of this work, he was part of the KliWiSt project that received funding from the German Federal Ministry for Economic Affairs and Climate Action (grant no. 03EE3041B). Daniela C. A. Lima is supported by the Portuguese Foundation for Science and Technology (FCT) financed by national funds from the MCTES through grant no. https://doi.org/10.54499/2022.03183.CEECIND/CP1715/CT0004.
This paper was edited by Somnath Baidya Roy and reviewed by three anonymous referees.
Asselin, O., Leduc, M., Paquin, D., Di Luca, A., Winger, K., Bukovsky, M., Music, B., and Giguère, M.: On the Intercontinental Transferability of Regional Climate Model Response to Severe Forestation, Climate, 10, 138, https://doi.org/10.3390/cli10100138, 2022. a
Bloomfield, H. C., Brayshaw, D. J., Shaffrey, L. C., Coker, P. J., and Thornton, H. E.: Quantifying the increasing sensitivity of power systems to climate variability, Environ. Res. Lett., 11, 124025, https://doi.org/10.1088/1748-9326/11/12/124025, 2016. a
Breil, M., Rechid, D., Davin, E. L., de Noblet-Ducoudré, N., Katragkou, E., Cardoso, R. M., Hoffmann, P., Jach, L. L., Soares, P. M. M., Sofiadis, G., Strada, S., Strandberg, G., Tölle, M. H., and Warrach-Sagi, K.: The Opposing Effects of Reforestation and Afforestation on the Diurnal Temperature Cycle at the Surface and in the Lowest Atmospheric Model Level in the European Summer, J. Clim., 33, 9159–9179, https://doi.org/10.1175/JCLI-D-19-0624.1, 2020. a, b, c, d
Commission, E.: Regulation (EU) 2021/1119 of the European Parliament and of the Council of 30 June 2021 establishing the framework for achieving climate neutrality and amending Regulations (EC) No 401/2009 and (EU) 2018/1999 (‘European Climate Law’), Official Journal of the European Union, https://eur-lex.europa.eu/legal-content/EN/TXT/?uri=CELEX:32021R1119 (last access: 28 October 2024), 2021. a
Confederation, S.: Bundesgesetz über die Ziele im Klimaschutz, die Innovation und die Stärkung der Energiesicherheit, Fedlex, https://www.fedlex.admin.ch/eli/fga/2022/2403/de (last access: 28 October 2024), 2022. a
CORDEX, W.: CORDEX domains for model integrations, https://cordex.org/wp-content/uploads/2012/11/CORDEX-domain-description_231015.pdf (last access: 28 October 2024), 2015. a
Craig, M. T., Wohland, J., Stoop, L. P., Kies, A., Pickering, B., Bloomfield, H. C., Browell, J., De Felice, M., Dent, C. J., Deroubaix, A., Frischmuth, F., Gonzalez, P. L., Grochowicz, A., Gruber, K., Härtel, P., Kittel, M., Kotzur, L., Labuhn, I., Lundquist, J. K., Pflugradt, N., van der Wiel, K., Zeyringer, M., and Brayshaw, D. J.: Overcoming the disconnect between energy system and climate modeling, Joule, 6, 1405–1417, https://doi.org/10.1016/j.joule.2022.05.010, 2022. a
Daloz, A. S., Schwingshackl, C., Mooney, P., Strada, S., Rechid, D., Davin, E. L., Katragkou, E., De Noblet-Ducoudré, N., Belda, M., Halenka, T., Breil, M., Cardoso, R. M., Hoffmann, P., Lima, D. C. A., Meier, R., Soares, P. M. M., Sofiadis, G., Strandberg, G., Toelle, M. H., and Lund, M. T.: Land–atmosphere interactions in sub-polar and alpine climates in the CORDEX flagship pilot study Land Use and Climate Across Scales (LUCAS) models – Part 1: Evaluation of the snow-albedo effect, The Cryosphere, 16, 2403–2419, https://doi.org/10.5194/tc-16-2403-2022, 2022. a
Davin, E. L., Rechid, D., Breil, M., Cardoso, R. M., Coppola, E., Hoffmann, P., Jach, L. L., Katragkou, E., de Noblet-Ducoudré, N., Radtke, K., Raffa, M., Soares, P. M. M., Sofiadis, G., Strada, S., Strandberg, G., Tölle, M. H., Warrach-Sagi, K., and Wulfmeyer, V.: Biogeophysical impacts of forestation in Europe: first results from the LUCAS (Land Use and Climate Across Scales) regional climate model intercomparison, Earth Syst. Dynam., 11, 183–200, https://doi.org/10.5194/esd-11-183-2020, 2020. a, b, c, d, e, f
Emeis, S.: Wind Energy Meteorology: Atmospheric Physics for Wind Power Generation, Green Energy and Technology, Springer Berlin Heidelberg, Berlin, Heidelberg, ISBN 978-3-642-30522-1, 978-3-642-30523-8, https://doi.org/10.1007/978-3-642-30523-8, 2013. a
Eyring, V., Bony, S., Meehl, G. A., Senior, C. A., Stevens, B., Stouffer, R. J., and Taylor, K. E.: Overview of the Coupled Model Intercomparison Project Phase 6 (CMIP6) experimental design and organization, Geosci. Model Dev., 9, 1937–1958, https://doi.org/10.5194/gmd-9-1937-2016, 2016. a
Haas, S., Schachler, B., and Krien, U.: Windpowerlib – a Python Library to Model Wind Power Plants – v.0.2.0, Zenodo, https://doi.org/10.5281/zenodo.3403360, 2019. a
Hahmann, A. N., García-Santiago, O., and Peña, A.: Current and future wind energy resources in the North Sea according to CMIP6, Wind Energ. Sci., 7, 2373–2391, https://doi.org/10.5194/wes-7-2373-2022, 2022. a, b, c
Hueging, H., Haas, R., Born, K., Jacob, D., and Pinto, J. G.: Regional Changes in Wind Energy Potential over Europe Using Regional Climate Model Ensemble Projections, J. Appl. Meteorol. Climatol., 52, 903–917, https://doi.org/10.1175/JAMC-D-12-086.1, 2013. a, b
Hurtt, G. C., Chini, L. P., Frolking, S., Betts, R. A., Feddema, J., Fischer, G., Fisk, J. P., Hibbard, K., Houghton, R. A., Janetos, A., Jones, C. D., Kindermann, G., Kinoshita, T., Klein Goldewijk, K., Riahi, K., Shevliakova, E., Smith, S., Stehfest, E., Thomson, A., Thornton, P., van Vuuren, D. P., and Wang, Y. P.: Harmonization of land-use scenarios for the period 1500–2100: 600 years of global gridded annual land-use transitions, wood harvest, and resulting secondary lands, Climatic Change, 109, 117–161, https://doi.org/10.1007/s10584-011-0153-2, 2011. a
Hurtt, G. C., Chini, L., Sahajpal, R., Frolking, S., Bodirsky, B. L., Calvin, K., Doelman, J. C., Fisk, J., Fujimori, S., Klein Goldewijk, K., Hasegawa, T., Havlik, P., Heinimann, A., Humpenöder, F., Jungclaus, J., Kaplan, J. O., Kennedy, J., Krisztin, T., Lawrence, D., Lawrence, P., Ma, L., Mertz, O., Pongratz, J., Popp, A., Poulter, B., Riahi, K., Shevliakova, E., Stehfest, E., Thornton, P., Tubiello, F. N., van Vuuren, D. P., and Zhang, X.: Harmonization of global land use change and management for the period 850–2100 (LUH2) for CMIP6, Geosci. Model Dev., 13, 5425–5464, https://doi.org/10.5194/gmd-13-5425-2020, 2020. a
IPCC: Climate Change 2022: Mitigation of Climate Change. Contribution of Working Group III to the Sixth Assessment Report of the Intergovernmental Panel on Climate Change, edited by: Shukla, P. R., Skea, J., Slade, R., Al Khourdajie, A., van Diemen, R., McCollum, D., Pathak, M., Some, S., Vyas, P., Fradera, R., Belkacemi, M., Hasija, A., Lisboa, G., Luz, S., and Malley, J., Cambridge University Press, Cambridge, UK and New York, NY, USA, https://doi.org/10.1017/9781009157926, 2022. a, b
IRENA: Renewable Power Generation Costs in 2021, International Renewable Energy Agency, Abu Dhabi, 2022, ISBN 978-92-9260-452-3, 2022. a
Jacob, D., Petersen, J., Eggert, B., Alias, A., Christensen, O. B., Bouwer, L. M., Braun, A., Colette, A., Déqué, M., Georgievski, G., Georgopoulou, E., Gobiet, A., Menut, L., Nikulin, G., Haensler, A., Hempelmann, N., Jones, C., Keuler, K., Kovats, S., Kröner, N., Kotlarski, S., Kriegsmann, A., Martin, E., van Meijgaard, E., Moseley, C., Pfeifer, S., Preuschmann, S., Radermacher, C., Radtke, K., Rechid, D., Rounsevell, M., Samuelsson, P., Somot, S., Soussana, J.-F., Teichmann, C., Valentini, R., Vautard, R., Weber, B., and Yiou, P.: EURO-CORDEX: new high-resolution climate change projections for European impact research, Reg. Environ. Change, 14, 563–578, https://doi.org/10.1007/s10113-013-0499-2, 2014. a
Karnauskas, K. B., Lundquist, J. K., and Zhang, L.: Southward shift of the global wind energy resource under high carbon dioxide emissions, Nat. Geosci., 11, 38–43, https://doi.org/10.1038/s41561-017-0029-9, 2018. a
Knutti, R., Masson, D., and Gettelman, A.: Climate model genealogy: Generation CMIP5 and how we got there: CLIMATE MODEL GENEALOGY, Geophys. Res. Lett., 40, 1194–1199, https://doi.org/10.1002/grl.50256, 2013. a
Lima, D. C. A., Soares, P. M. M., Cardoso, R. M., Semedo, A., Cabos, W., and Sein, D. V.: The present and future offshore wind resource in the Southwestern African region, Clim. Dynam., 56, 1371–1388, https://doi.org/10.1007/s00382-020-05536-4, 2021. a
Moemken, J., Reyers, M., Feldmann, H., and Pinto, J. G.: Future Changes of Wind Speed and Wind Energy Potentials in EURO-CORDEX Ensemble Simulations, J. Geophys. Res.-Atmos., 123, 6373–6389, https://doi.org/10.1029/2018JD028473, 2018. a
Mooney, P. A., Rechid, D., Davin, E. L., Katragkou, E., De Noblet-Ducoudré, N., Breil, M., Cardoso, R. M., Daloz, A. S., Hoffmann, P., Lima, D. C. A., Meier, R., Soares, P. M. M., Sofiadis, G., Strada, S., Strandberg, G., Toelle, M. H., and Lund, M. T.: Land–atmosphere interactions in sub-polar and alpine climates in the CORDEX Flagship Pilot Study Land Use and Climate Across Scales (LUCAS) models – Part 2: The role of changing vegetation, The Cryosphere, 16, 1383–1397, https://doi.org/10.5194/tc-16-1383-2022, 2022. a
O’Reilly, C. H., Befort, D. J., Weisheimer, A., Woollings, T., Ballinger, A., and Hegerl, G.: Projections of northern hemisphere extratropical climate underestimate internal variability and associated uncertainty, Commun. Earth Environ., 2, 194, https://doi.org/10.1038/s43247-021-00268-7, 2021. a
Pryor, S. C., Barthelmie, R. J., Bukovsky, M. S., Leung, L. R., and Sakaguchi, K.: Climate change impacts on wind power generation, Nat. Rev. Earth Environ., 1, 627–643, https://doi.org/10.1038/s43017-020-0101-7, 2020. a, b
Reyers, M., Moemken, J., and Pinto, J. G.: Future changes of wind energy potentials over Europe in a large CMIP5 multi-model ensemble: FUTURE CHANGES OF WIND ENERGY OVER EUROPE IN A CMIP5 ENSEMBLE, Int. J. Climatol., 36, 783–796, https://doi.org/10.1002/joc.4382, 2016. a
Schemm, S.: Toward Eliminating the Decades‐Old “Too Zonal and Too Equatorward” Storm‐Track Bias in Climate Models, J. Adv. Model. Earth Syst., 15, e2022MS003482, https://doi.org/10.1029/2022MS003482, 2023. a
Schlott, M., Kies, A., Brown, T., Schramm, S., and Greiner, M.: The impact of climate change on a cost-optimal highly renewable European electricity network, Appl. Energ., 230, 1645–1659, https://doi.org/10.1016/j.apenergy.2018.09.084, 2018. a, b
Simpson, I. R., Deser, C., McKinnon, K. A., and Barnes, E. A.: Modeled and Observed Multidecadal Variability in the North Atlantic Jet Stream and Its Connection to Sea Surface Temperatures, J. Climate, 31, 8313–8338, https://doi.org/10.1175/JCLI-D-18-0168.1, 2018. a
Soares, P. M. M., Lima, D. C. A., Semedo, A., Cabos, W., and Sein, D. V.: Climate change impact on Northwestern African offshore wind energy resources, Environ. Res. Lett., 14, 124065, https://doi.org/10.1088/1748-9326/ab5731, 2019. a, b
Soares, P. M. M., Lima, D. C. A., and Nogueira, M.: Global offshore wind energy resources using the new ERA-5 reanalysis, Environ. Res. Lett., 15, 1040a2, https://doi.org/10.1088/1748-9326/abb10d, 2020. a
Sofiadis, G., Katragkou, E., Davin, E. L., Rechid, D., De Noblet-Ducoudre, N., Breil, M., Cardoso, R. M., Hoffmann, P., Jach, L., Meier, R., Mooney, P. A., Soares, P. M. M., Strada, S., Tölle, M. H., and Warrach Sagi, K.: Afforestation impact on soil temperature in regional climate model simulations over Europe, Geosci. Model Dev., 15, 595–616, https://doi.org/10.5194/gmd-15-595-2022, 2022. a
Taylor, K. E., Stouffer, R. J., and Meehl, G. A.: An Overview of CMIP5 and the Experiment Design, Bull. Am. Meteorol. Soc., 93, 485–498, https://doi.org/10.1175/BAMS-D-11-00094.1, 2012. a, b
Thompson, E.: Escape from model land : how mathematical models can lead us astray and what we can do about it, Hodder And Stoughton, New York, Basic Books, New York, NY, ISBN 978-1-5416-0098-0, 2022. a
Tobin, I., Jerez, S., Vautard, R., Thais, F., van Meijgaard, E., Prein, A., Déqué, M., Kotlarski, S., Maule, C. F., Nikulin, G., Noël, T., and Teichmann, C.: Climate change impacts on the power generation potential of a European mid-century wind farms scenario, Environ. Res. Lett., 11, 034013, https://doi.org/10.1088/1748-9326/11/3/034013, 2016. a, b
UK: Climate Change Act, https://www.legislation.gov.uk/ukpga/2008/27/section/1 (last access: 28 October 2024), 2008. a
van der Wiel, K., Stoop, L., van Zuijlen, B., Blackport, R., van den Broek, M., and Selten, F.: Meteorological conditions leading to extreme low variable renewable energy production and extreme high energy shortfall, Renew. Sustain. Energ. Rev., 111, 261–275, https://doi.org/10.1016/j.rser.2019.04.065, 2019. a
Weide Luiz, E. and Fiedler, S.: Spatiotemporal observations of nocturnal low-level jets and impacts on wind power production, Wind Energ. Sci., 7, 1575–1591, https://doi.org/10.5194/wes-7-1575-2022, 2022. a
Wohland, J.: Process-based climate change assessment for European winds using EURO-CORDEX and global models, Environ. Res. Lett., 17, 124047, https://doi.org/10.1088/1748-9326/aca77f, 2022. a, b, c
Wohland, J.: Land-use change impacts on wind profiles according to regional climate models, GitHup repository [code], https://github.com/jwohland/lucas_3dwinds (last access: 28 October 2024), 2024. a, b
Wohland, J., Reyers, M., Weber, J., and Witthaut, D.: More homogeneous wind conditions under strong climate change decrease the potential for inter-state balancing of electricity in Europe, Earth Syst. Dynam., 8, 1047–1060, https://doi.org/10.5194/esd-8-1047-2017, 2017. a
Wohland, J., Brayshaw, D., and Pfenninger, S.: Mitigating a century of European renewable variability with transmission and informed siting, Environ. Res. Lett., 16, 064026, https://doi.org/10.1088/1748-9326/abff89, 2021a. a
Wohland, J., Folini, D., and Pickering, B.: Wind speed stilling and its recovery due to internal climate variability, Earth Syst. Dynam., 12, 1239–1251, https://doi.org/10.5194/esd-12-1239-2021, 2021b. a