the Creative Commons Attribution 4.0 License.
the Creative Commons Attribution 4.0 License.
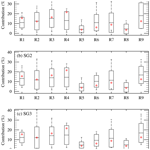
Impacts of land use change and elevated CO2 on the interannual variations and seasonal cycles of gross primary productivity in China
Xin Luo
Ximing Cai
Atul Jain
Deborah N. Huntzinger
Zhenghui Xie
Ning Zeng
Jiafu Mao
Xiaoying Shi
Akihiko Ito
Yaxing Wei
Hanqin Tian
Benjamin Poulter
Dan Hayes
Kevin Schaefer
Climate change, rising CO2 concentration, and land use and land cover change (LULCC) are primary driving forces for terrestrial gross primary productivity (GPP), but their impacts on the temporal changes in GPP are uncertain. In this study, the effects of the three main factors on the interannual variation (IAV) and seasonal cycle amplitude (SCA) of GPP in China were investigated using 12 terrestrial biosphere models from the Multi-scale Synthesis and Terrestrial Model Intercomparison Project. The simulated ensemble mean value of China's GPP between 1981 and 2010, driven by common climate forcing, LULCC and CO2 data, was found to be 7.4±1.8 Pg C yr−1. In general, climate was the dominant control factor of the annual trends, IAV and seasonality of China's GPP. The overall rising CO2 led to enhanced plant photosynthesis, thus increasing annual mean and IAV of China's total GPP, especially in northeastern and southern China, where vegetation is dense. LULCC decreased the IAV of China's total GPP by ∼7 %, whereas rising CO2 induced an increase of 8 %. Compared to climate change and elevated CO2, LULCC showed less contributions to GPP's temporal variation, and its impact acted locally, mainly in southwestern China. Furthermore, this study also examined subregional contributions to the temporal changes in China's total GPP. Southern and southeastern China showed higher contributions to China's annual GPP, whereas southwestern and central parts of China explained larger fractions of the IAV in China's GPP.
- Article
(6338 KB) -
Supplement
(2120 KB) - BibTeX
- EndNote
Terrestrial ecosystems can function as a major sink in the global carbon cycle, potentially offsetting a significant amount of anthropogenic carbon emissions (Le Quéré et al., 2018). Gross primary productivity (GPP) is the major driver of terrestrial ecosystem carbon storage and plays a key role in terrestrial carbon cycle (Yuan et al., 2010; Mao et al., 2012; Piao et al., 2013; Anav et al., 2015; Zhou et al., 2016; Ito et al., 2017). Therefore, understanding the spatiotemporal patterns of terrestrial ecosystem GPP has been a research focus in quantifying the global carbon cycle (Anav et al., 2015; Zhou et al., 2016; Chen et al., 2017). However, GPP is susceptible to CO2 concentration and human interference (primarily land use and land cover change, hereafter LULCC) besides climate change (Friedlingstein et al., 2010; Ciais et al., 2013; Li et al., 2015), which complicates the quantification of the impacts.
Atmospheric CO2 concentration has increased by ∼40 % from 1750 to 2011 (IPCC, 2013). Several studies have examined the effect of rising CO2 concentration on global terrestrial carbon uptake (Piao et al., 2013; Schimel et al., 2014; Ito et al., 2016). Schimel et al. (2014) found that up to 60 % of the present-day terrestrial sinks was caused by increasing atmospheric CO2. Simulations from a coupled earth system indicated that CO2 fertilization increased the global net primary productivity by ∼2.3 Pg C yr−1 between 1850 and 2005 (Devaraju et al., 2016). It suggests that the CO2 effect on land carbon storage may be a key potential negative feedback to future climate (Schimel et al., 2014). However, the extent to which CO2 fertilization is responsible for current and future terrestrial carbon storage is still unclear (Zaehle et al., 2010; IPCC, 2013).
Anthropogenic LULCC also has a large effect on terrestrial carbon cycles, including the “net effect” of CO2 sources (e.g., deforestation, logging, harvesting, and other direct human activities) and CO2 sinks (e.g., afforestation and vegetation regrowth following land disturbance) (Brovkin et al., 2004; Boysen et al., 2014; Pongratz et al., 2014; Houghton and Nassikas, 2017). IPCC (2013) pointed out that LULCC-associated CO2 emissions have contributed Pg C to cumulative anthropogenic CO2 emissions (one-third of total anthropogenic CO2 emissions) since 1750. As indicated by Le Quéré et al. (2018), CO2 emissions from LULCC at the global scale have remained relatively constant, at around 1.3±0.7 Pg C yr−1, over the past half-century. However, regional CO2 emissions showed different characteristics (Houghton and Nassikas, 2017).
During the past decades, China has experienced tremendous LULCC as a result of continued population growth and intensified human development against a broad background of climate change (Piao et al., 2009; Liu and Tian, 2010; Xiao et al., 2015; Li et al., 2015; Zhang et al., 2016). These massive LULCCs have made a significant contribution to regional and global carbon sinks during the past few decades (Guo et al., 2013; Fang et al., 2014; Xiao et al., 2015; Li et al., 2015). Hence, studies on the impacts of LULCC on GPP in China have important theoretical and practical value for understanding the spatiotemporal patterns of terrestrial carbon cycle and forecasting their response to future global and regional changes and human activities (Tian et al., 2011a, b).
However, few studies have adequately explored the impacts of climate change, atmospheric CO2 concentration, and LULCC to interannual and seasonal variations of GPP in China (Piao et al., 2013; Yao et al., 2018). These studies mainly focused on the climatic driver (temperature, precipitation, and solar radiation) of GPP interannual variations (Yao et al., 2018) and responses of GPP to climate variations and atmospheric CO2 concentration (Piao et al., 2013). But the quantitative contributions of these three factors to GPP in China are still unclear, which urgently needs to be addressed. Although continuous improvements have been achieved for the development of terrestrial biosphere models (TBMs) alongside our deepening understanding of terrestrial carbon cycle process, current TBMs still have large uncertainties in GPP simulation (Piao et al., 2013; Devaraju et al., 2016; Ito et al., 2016). Multi-model ensemble simulation has been an effective method to reduce the uncertainties induced by TBMs (Schwalm et al., 2015; Liu et al., 2016). Therefore, in the present study, 12 progress-based TBMs from the Multi-scale Synthesis and Terrestrial Model Intercomparison Project (MsTMIP) (Huntzinger et al., 2013; Wei et al., 2014a) were used to investigate the effects of climate change, increasing CO2 concentration and LULCC on the interannual variation and seasonal cycle of GPP in China. The goals of this work were to (1) investigate the interannual and seasonal variations of GPP in China between 1981 and 2010, (2) quantify the individual influences of climate change, CO2 concentration, and LULCC, and (3) examine the relative contributions of major subregions to China's total GPP.
2.1 Model description and experimental design
Twelve TBMs that participated in the MsTMIP were used in this study: CLM4, CLM4VIC, DLEM, GTEC, ISAM, LPJ-wsl, ORCHIDEE-LSCE, SiB3-JPL, SiB3CASA, TEM6, VEGAS2.1 and VISIT (Huntzinger et al., 2013; Wei et al., 2014a, b). These model simulations all followed the same experimental design. Three sensitivity model simulations were used in this study: SG1, driven by time-varying climate data; SG2, considering the effect of LULCC based on SG1; and SG3, similar to SG2, but using time-varying atmospheric CO2 concentration. In this way, these three experiments can be used to assess the relative contributions of climate change, LULCC and rising CO2 concentration to temporal changes in GPP (Sect. S1 of the Supplement). All the simulated results have a spatial resolution of and are available at https://doi.org/10.3334/ORNLDAAC/1225 (Huntzinger et al., 2018). More detailed descriptions of the experimental design and forcing datasets can be found in the Supplement and Huntzinger et al. (2013) and Wei et al. (2014a, b). The simulated monthly GPP from these 12 models for the period of 1981–2010 was used in this work. The mean values calculated from these models (hereafter “ENSEMBLE”) were also calculated.
2.2 Evaluation data
This study used an observation-driven global monthly gridded GPP product derived from FLUXNET measurements by statistical upscaling with the machine-learning algorithm, model tree ensembles (Jung et al., 2009, 2011) (hereafter referred to as MTEs). The MTE statistical model consisting of a set of regression trees was first trained using site-level explanatory variables and GPP estimations from eddy flux tower measurements. These explanatory variables covered climate and biophysical variables such as vegetation types, temperature, precipitation, radiation, and satellite-derived fraction of absorbed photosynthetic active radiation. Then the MTE GPP product was generated through applying the trained regression trees for global upscaling using gridded datasets of the same explanatory variables. It has a spatial resolution of and is available between 1982 and 2011. The uncertainty of the MTE data is ∼46 g C m−2 yr−1 (5 %), which was calculated using the standard deviation of the 25 model tree ensembles (Jung et al., 2011).
2.3 Analysis method
The land area of China was divided into nine regions (Fig. 1a) through a consideration of their climate characteristics, plant vegetation types and geopolitical boundaries (Piao et al., 2009, 2010). For the whole of China and each subregion, interannual variations (IAVs), seasonal cycle amplitude (SCA) and GPP trends were analyzed and compared across MsTMIP models and MTE data. The IAV of GPP was defined using the standard deviations of each region's detrended annual time-series data. The SCA of GPP was defined as the difference between the largest and smallest values, indicating the maximum range of oscillation between peak and trough within a calendar year (Ito et al., 2016).
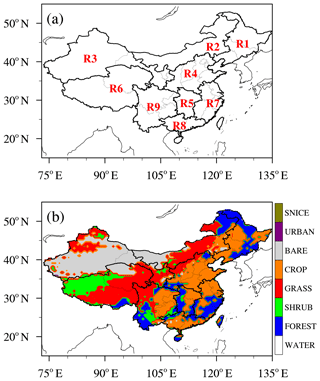
Figure 1Spatial distributions of (a) nine subregions (R1–R9) in the study region; and (b) present plant functional types (PFTs) used in SG1 simulations. R1: northeastern China (Heilongjiang, Jilin, Liaoning); R2: Inner Mongolia; R3: northwestern China (Gansu, Ningxia, Xinjiang); R4: northern China (Beijing, Hebei, Henan, Shandong, Shanxi, Shaanxi, Tianjin); R5: central China (Hubei, Hunan); R6: Tibetan Plateau (Qinghai, Tibet); R7: southeastern China (Anhui, Fujian, Jiangsu, Jiangxi, Shanghai, Zhejiang) and Taiwan; R8: southern China (Guangdong, Guangxi, Hainan, Hong Kong, Macao); and R9: southwestern China (Guizhou, Sichuan, Yunnan, Chongqing). SNICE is snow and ice; BARE is bare soil.
The nonparametric Mann–Kendall method was used to determine the statistical significance of trends in Chinese and regional GPP (area-weighted), where the Sen median slope (Sen, 1968) was considered as the trend value in this paper. Trend analysis was based on annual values averaged from monthly values. The relative contribution of each subregion to the IAV and SCA of China's GPP was also calculated based on the method proposed by Ahlström et al. (2015) and Chen et al. (2017). Please see the Supplement for more information.
3.1 Spatial patterns of GPP over China
In general, the spatial distributions of GPP from MsTMIP models (SG3) agreed well with the MTE (Fig. 2), with spatial correlation coefficients for most models higher than 0.9. The highest GPP values were observed in southeastern (R7) and southern China (R8) due to the wet climate and high solar radiation, and the smallest GPP values were mainly in arid regions of China (e.g., northwestern China, R3) and the Tibetan Plateau (R6) due to adverse conditions for plant photosynthetic activities. But the 12 models still have some differences in the spatial variations of GPP. VISIT showed a lower spatial correlation with the MTE (0.88) due to its higher GPP in R7 and lower values in R1. Compared to the MTE, three models (DLEM, TEM6, and VEGAS2.1) produced lower GPP in R1, and VEGAS2.1 produced higher GPP in R3 and the western parts of R6. The multi-model ensemble mean (ENSEMBLE) showed the highest spatial correlation with the MTE, suggesting that the ensemble mean best captured MTE spatial variability.
Figure 3 shows the annual mean GPP over China and each subregion. The 12 models' estimates of total China GPP were found to diverge, ranging from 4.9 (DLEM) to 10.5 (GTEC) Pg C yr−1 (Fig. 3a), with a standard deviation of 1.8 Pg C yr−1. The total China GPP from the multi-model ensemble mean was 7.4 Pg C yr−1, which was slightly higher than the MTE (7.0 Pg C yr−1, Fig. 3a). The regional sum of GPP in southwestern China from the ENSEMBLE (Fig. 3b) was the highest among all nine regions (1.5 Pg C yr−1, R9), followed by southeastern China (1.3 Pg C yr−1, R7) and southern China (1.0 Pg C yr−1, R8). These top three regions together contributed about 50 % of China's GPP (Fig. 3c). However, southern China (R8) showed the highest GPP estimates per unit area, at >2000 g C m−2 yr−1 (Fig. 3d). The relative contributions of each region to total China GPP from the MTE showed results similar to the MsTMIP. To understand more thoroughly the underlying mechanisms of GPP changes during 1981–2010, the effects of LULCC and atmospheric CO2 concentration on GPP changes were quantified based on the ensemble mean of the 12 MsTMIP models (Table 1). In general, LULCC (SG2, 7.1 Pg C yr−1) decreased annual mean GPP by ∼0.2 Pg C yr−1 (3 % of SG1) compared to SG1 (6.9 Pg C yr−1). In contrast, elevated atmospheric CO2 increased GPP by ∼0.7 Pg C yr−1 (10 % of SG1), although this response varied among different subregions (Table 1a). These results suggested that rising atmospheric CO2 concentration seems to have a greater effect on annual mean GPP over China than LULCC.
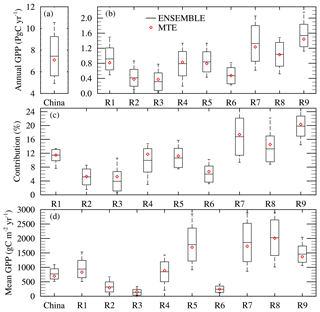
Figure 3Annual mean GPP from (a) China and (b) each subregion; (c) regional contributions to China GPP; (d) annual mean GPP per unit square meters. Horizontal lines at top, middle and bottom in the boxplots represent the maximum, ensemble mean, and minimum of multi-model simulations, respectively, whereas the box indicates 1 standard deviation. All the results in this figure are averaged for the period of 1981–2010 for the MsTMIP SG3 simulation and 1982–2010 for the MTE. Regional abbreviations used on the x axes are defined in Fig. 1a.
Table 1China and regional GPP from the MTE and the ensemble mean of the 12 MsTMIP models for three configurations (SG1, SG2 and SG3): (a) mean values (MEAN), (b) interannual variability (IAV), (c) seasonal-cycle amplitude (SCA).
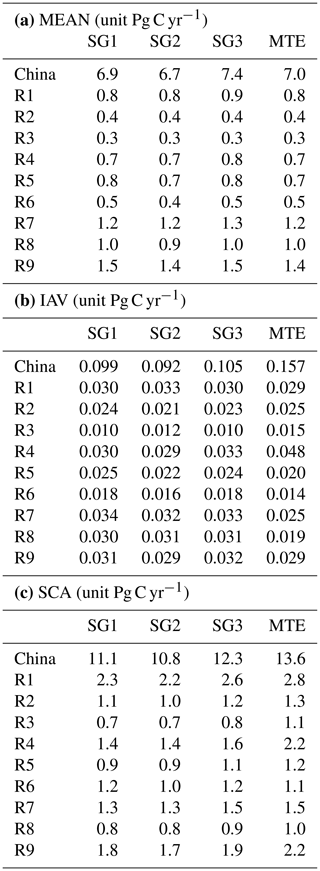
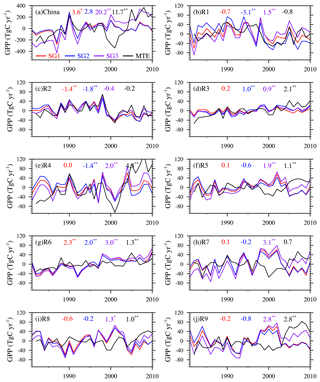
Figure 4Interannual variations in GPP of China and each subregion from the MTE (black) and the ensemble mean of the 12 MsTMIP models: SG1 (blue), SG2 (red), SG3 (purple). The anomalies of GPP were calculated as the difference between annual GPP and the long-term mean between 1981 and 2010 (MTE is 1982–2010). The numbers located at the top of each figure indicate the linear trends of SG1 (blue), SG2 (red), SG3 (purple) and MTE (black) with units of Tg C yr−2 (1 Tg C = 0.001 Pg C). Single (∗) and double () asterisks indicate that the trend is significant with p<0.1 and p<0.05, respectively.
3.2 Interannual variations and trends
During 1981–2010, the MTE estimates suggested that the IAV of China's GPP was 0.157 Pg C yr−1, but the multi-model ensemble mean values of MsTMIP for the three simulations all showed a slight underestimation (Table 1b). Compared to SG1 (0.099 Pg C yr−1), LULCC decreased the IAV by ∼0.007 Pg C yr−1 (7 % of SG1), whereas rising CO2 (SG3) led to an increase (∼0.008 Pg C yr−1, 8 % of SG1). The GPP from SG3 with a consideration of LULCC and elevated CO2 increased from 7.1 Pg C yr−1 in 1981 to 7.6 Pg C yr−1 in 2010, with a significant temporal trend of 0.02 Pg C yr−2 (p<0.05). The annual mean GPP values from SG3 exhibited significant increasing trends between 1981–2010 over all regions except for Inner Mongolia (R2, Fig. 4c), with the highest rates of increase over the Tibetan Plateau (R6, Fig. 4g) and southeastern China (R7, Fig. 4h), which were both more than 3.0 Tg C yr−2 (p < 0.05, 1 Tg C = 0.001 Pg C). Compared to SG1 (red line) with prescribed land cover, LULCC (blue line) decreased GPP trends over all regions, which was mainly related to land conversion including forest-to-crop and shrub-to-crop (Tao et al., 2013). On the contrary, elevated CO2 concentration significantly increased plant growth and thus led to more strongly increasing GPP trends (SG3, purple line). Compared to the SG3 simulations of MsTMIP, the MTE estimates appeared to show similar interannual variations (Table 1b). Figure S1 in the Supplement shows the spatial patterns of the correlation coefficients between annual GPP from MsTMIP and MTE. It is found that, compared to SG1 and SG2, SG3 captures the interannual variations in GPP of MTE best, with significantly positive correlations over most areas of China, except over the west of R2 and parts of R5 and R1. The highest correlations mainly occur over the middle of R2 and northeast of R6. In addition, SG3 has the same trends in GPP (significantly increasing) with MTE for R3, R4, R5, R6, R8, and R9 (Fig. 4d, e, f, g, i, j), except for some differences in the magnitude. For example, the SG3 is found to show a weaker increasing trend (2.0 Tg C yr−2) for R4 and a larger one for R6 than the MTE (4.3 Tg C yr−2). For R2 (Fig. 4c), SG1 and SG2 show a significant decreasing trend, while trends for SG3 and MTE are not significant. A similar increasing trend can be found for SG3 and MTE over R7 (Fig. 4h) except that the trend of SG3 is significant. Large differences in the trend of GPP can be observed over R1 (Fig. 4b): SG3 shows a significant increasing trend, while the GPP of MTE is decreasing. However, the mean values and IAV of GPP over R1 are close in SG3 and MTE (Table 1a). For all of China (Fig. 4a), the trend in GPP from the MsTMIP is lower than that of the MTE due to large discrepancies between 1999 and 2002. To further validate the trends in GPP from MsTMIP, we compare their spatial distributions with that from MTE (Fig. S2). Compared to SG1 (Fig. S2a), LULCC leads to a decrease in annual mean GPP (e.g., many areas with stronger negative trend; Fig. S2b). In contrast, rising atmospheric CO2 concentration significantly strengthens the ascending trend in GPP by increasing the rate of photosynthesis (Fig. S2c). Moreover, SG3 captures the trend in GPP of MTE better than SG1 and SG2, with significantly increasing trends over most areas of China and decreasing trends over the east of R2. However, some discrepancies between SG3 and MTE can be observed over R1, east parts of R7 and R9. We then compared them with another GPP product from Yao et al. (2018) (hereafter YAO, Fig. 4a in that paper). It is found that SG3 from MsTMIP shows similar trends with YAO over R1 and east parts of R7. In contrast, MTE shows the same increasing trends with YAO over east parts of R9. It suggests that both model simulations from MsTMIP and the MTE GPP product show certain uncertainties in the GPP trend over some areas of China, which needs more observations to evaluate the GPP trend in future work.
Figure 5 shows the regional contributions to the IAV of China's GPP for the three MsTMIP simulations (SG1, SG2 and SG3). The ensemble mean GPP of SG3 over R9 was found to explain the largest fraction (17 %) of the IAV for China's GPP, followed by R5 (15 %) and R4 (14 %). In contrast, the contributions of southeastern and southern China (R7, R8) to the IAV of China's overall GPP were relatively lower (4 % and 11 %), even with higher contributions to China's annual mean GPP (Fig. 3c). The relative contributions of each subregion to the IAV of China's GPP from the ENSEMBLE agreed well with the MTE (within 1 standard deviation), except for a slight overestimation over southwestern China and an underestimation over R5. The contributions from R4, R5 and R9 were all high for all three MsMTIP simulations and MTE, except for a few differences in magnitude. Note the significant uncertainties with large standard deviations among the estimated relative contributions of each subregion from the 12 MsTMIP models, especially over R4 and R9. Compared to SG1, SG2 and SG3 showed similar contributions for each subregion, suggesting that rising atmospheric CO2 and LULCC have little effect on the relative contribution of each region to the IAV of China's GPP. However, they modulated the magnitude of the IAV and the annual mean values of China and regional GPP (Table 1).
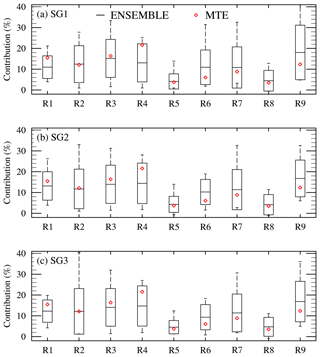
Figure 5The relative contributions of each subregion to the interannual variability (IAV) of China's GPP. Boxplots indicate the distributions of the 12 MsTMIP models. Horizontal lines at top, middle and bottom in the boxplots represent the maximum, ensemble mean and minimum of multi-model simulations, respectively, whereas the box indicates 1 standard deviation. All the results in this figure are averaged between 1981–2010 for the MsTMIP models and 1982–2010 for the MTE.
3.3 Seasonal variations and regional contributions
Figure 6 shows the seasonal variations in GPP of over all of China and each subregion from the MsTMIP and MTE. In general, the MsTMIP ensemble mean showed seasonal cycles similar to the MTE data over China and all subregions, with strong correlations (r>0.97), except for R4 (Fig. 6e), where large discrepancies in summer (July and August) could be observed. SG1 and SG2 showed almost the same seasonal variations except for a few differences in summer over R9 (Fig. 6j), suggesting that LULCC had few effects on seasonal GPP variation in China. In contrast, elevated CO2 concentrations produced higher GPP during the growing season through enhancing the plant growth rate and thus modulated seasonal GPP variations. Table 1c shows that human activities (e.g., LULCC and elevated CO2 concentration) exerted influences on the SCA of GPP. The difference between the SG2 and SG3 was mainly caused by rising atmospheric CO2 concentrations, whereas LULCC led to a small discrepancy between the SG1 and SG2. For example, compared to SG1, LULCC decreased the SCA by only ∼0.3 Pg C yr−1 (3 % of SG1), whereas elevated CO2 produced an increase of 1.5 Pg C yr−1 (14 % of SG1). Meanwhile, the SCAs of China's GPP (11.1–12.3 Pg C yr−1, Table 1c) from ENSEMBLE were detected with only a slight underestimation compared to the MTE data (13.6 Pg C yr−1).
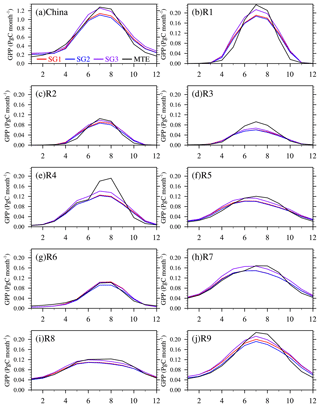
Figure 6Seasonal variations in GPP of China and each subregion from the ensemble mean of the 12 MsTMIP models for the three simulations (SG1, SG2 and SG3) and the MTE. All the results in this figure are averaged between 1981–2010 for the MsTMIP and 1982–2010 for the MTE.
Next, the regional contributions to the seasonality of China's GPP were examined for MsTMIP (SG1, SG2 and SG3) and MTE (Fig. 7). The ensemble mean GPP of SG3 (Fig. 7c) over R1 explained the largest fraction (20 %) of the seasonality of China's GPP, followed by R6 (16 %) and R9 (15 %). This could be explained because the GPP in these regions had strong seasonal cycles (Fig. 6b, g and j). In contrast, the contributions of R7 and R8 to the seasonal cycle of China's GPP were relatively low (3 % and 8 %, respectively). The relative regional contributions to the seasonal dynamics of China's GPP from MsTMIP agreed well with MTE (within 1 standard deviation). The contributions from R4, R5 and R9 were all high for all three MsTMIP simulations and MTE, except for a few differences in magnitude. Note the significant uncertainties, with large standard deviations, in R3 and R4 among the estimated relative regional contributions from the 12 MsTMIP models. Compared to SG1, SG2 and SG3 showed similar contributions for each subregion, suggesting that atmospheric CO2 and LULCC had little effect on the relative subregional contributions to the seasonal cycle of China's GPP.
4.1 Understanding the contribution of LULCC
The TBMs used in this study relied on LULCC data by combining a static satellite-based land cover product (Jung et al., 2006) with time-varying land use harmonization version 1 (LUH1) data (Hurtt et al., 2011). Based on this dataset, time series of different vegetation cover types over China and the nine subregions were developed and are presented in Fig. 8 (solid lines). Crop areas showed a persistent increase during the past 3 decades (from 13 % to 18 %), whereas forest areas were shrinking (from 25 % to 20 %). Grassland areas showed a slight increase in the 1990s and then changed little during the past 2 decades. These changes induced a decrease in the mean values of China's GPP (Table 1a). LULCC in China showed significant spatial variations. For example, changes in grassland occurred mostly in Inner Mongolia (R2, Fig. 8c). Cropland expansion was widely distributed across China, but at different rates in each subregion. As for forest land, the largest loss occurred over northern China (Fig. 8e) and parts of southern China (Fig. 8f, h, i and j).
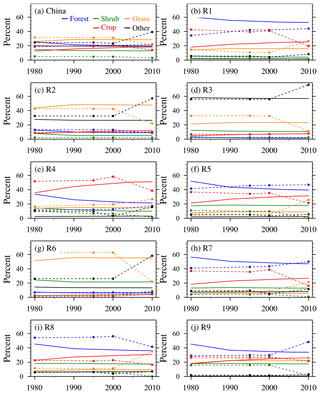
Figure 8Interannual changes of vegetation types over China and nine subregions between 1981 and 2010 from the MsTMIP (solid lines) and the China Land Use/Cover Dataset (dashed lines with dots). Other includes SNICE (snow and ice), water and bare soil.
LULCC in China from the LUH1 product used in this study showed some differences from previous studies. For example, Liu and Tian (2010) reconstructed an LULCC dataset for China using high-resolution satellite and historical survey data and found that LULCC in China during 1980–2005 was characterized by shrinking cropland and expanding urban and forest areas. Chen (2007) also reported a similar trend of shrinking cropland in China during 1977–2003 and attributed it to urbanization. Several studies have reported an increase in forest area after 1980 (Fang et al., 2001; Houghton and Hackler, 2003; Song and Deng, 2017), which was mainly due to new plantings to protect the environment (Wang et al., 2004). To assess the reliability of LULCC data used in this study, we compared them with the China Land Use/Cover Dataset (CLUD) (Liu, et al., 2003, 2005, 2010, 2014; Kuang et al., 2016), which was generated using two satellite datasets: the LandsatTM/ETM+ and HJ-1A/1B images from the China Centre for Resources Satellite Data and Application (http://www.cresda.com/; last access: 1 December 2017). The CLUD is a national high-resolution database (1 km) and contains the longest time-series dataset available for LULCC in China (Kuang et al., 2016). Its classification system includes 6 classes (woodland, cultivated land, grassland, water bodies, built-up land and unused land) and 25 subclasses (Liu et al., 2005; Zhang et al., 2014). The accuracy assessments for the CLUD have been addressed in previous studies (Liu et al., 2003, 2005, 2010, 2014; Kuang et al., 2013, 2016). Based on the CLUD, the maps of main vegetation types in 1990, 1995, 2000 and 2010 were used here, and their temporal changes in China and nine subregions are shown in Fig. 8 (dashed lines with dots). It is noted that CLUD is not available before 1990. In general, the LULCC data used in the MsTMIP agree well with the CLUD between 1990 and 2005, except for some discrepancies in 2010. Compared to that in 2000, the CLUD showed a slight increase in forest (from 20 % to 22 %) and shrinking cropland (from 31 % to 21 %) and grassland (from 20 % to 14 %) in 2010 for all of China. The decrease in cropland was mainly from R1 (Fig. 8b), R4 (Fig. 8e), R5 (Fig. 8f) and R7 (Fig. 8h), while the changes in grassland occurred mostly in R2 (Fig. 8c), R3 (Fig. 8d) and R6 (Fig. 8g).
The uncertainties in the LULCC dataset could influence its contribution to terrestrial carbon fluxes. For example, the MTE GPP product shows a significantly increasing trend after 2005 over R4 (Fig. 4e), R5 (Fig. 4f) and R7 (Fig. 4h), while some underestimations can be found for model-simulated GPP. This may be related with the discrepancies in the LULCC datasets over these areas. In upcoming revisions to LUH1, the new LUH2 product (http://luh.umd.edu/data.shtml; last access: 15 December 2018) will include updated inputs, higher spatial resolution, more detailed land use transitions and the addition of important agricultural management layers. Moreover, forest cover gross transitions are now constrained by remote-sensing information and have generally been re-estimated. Therefore, future studies are expected to compare the potential effect on GPP with the new product.
4.2 Uncertainties in simulating GPP in China
Despite growing efforts to quantify GPP, current TBM simulations still have large uncertainties. Each TBM has different parameterizations, which leads to its own bias, and the ensemble mean of multi-model simulations may reduce the bias in GPP (Ito et al., 2016; Chen et al., 2017). Therefore, this study did not focus on comparisons among the 12 model simulations.
The multi-model mean of the 12 MsTMIP models (SG3) for all of China GPP was 7.4 Pg C yr−1, which was slightly higher than the MTE estimate (7.0 Pg C yr−1). The results in this study also showed some differences with previous studies. For example, China GPP estimates based on the Eddy-Covariance Light Use Efficiency model were 5.38 (Yuan et al., 2010), 5.55 (Cai et al., 2014) and 6.04 Pg C yr−1 (Li et al., 2014), respectively, which was more than 20 % lower than in this study. Yao et al. (2018) developed a new GPP product for China with higher spatial resolution (0.1∘) based on a machine-learning algorithm using more eddy flux observations than the MTE. They found that the annual GPP of China was 6.62±0.23 Pg C yr−1 during 1982–2015. In contrast, the ensemble mean of nine TBMs produced a higher estimate of 7.85 Pg C yr−1 (Yao et al., 2018). In addition, two newly published studies also generated high estimates of total annual GPP: 7.85 Pg C yr−1 for 2001–2010 by multiple regression (Zhu et al., 2014) and 7.81 Pg C yr−1 for 2000–2015 using support vector regression (Ichii et al., 2017). Unlike the discrepancies in the magnitude of annual mean China GPP, the trend in this study is very similar to that of Yao et al. (2018), with a positive value of 0.02 Pg C yr−2 (p<0.05).
In this study, MsTMIP and MTE were found to show some discrepancies in the IAV and trends of GPP. For example, the trend of MsTMIP is about twice that derived from the MTE data (Fig. 4). The reason for the differences can be explained through the following two aspects. First of all, uncertainties in meteorological forcing dataset, model structure and parameterization can lead to large biases in simulating the spatiotemporal patterns of GPP, although this could be reduced by ensemble simulations from MsTMIP. Secondly, although a data-oriented GPP product (e.g., the MTE) has been used as the reference data to evaluate the TBM simulations (Piao et al., 2012, 2013; Jia et al., 2018; Yao et al., 2018), previous studies found that MTE data may underestimate the IAV and trends (Jung et al., 2011; Piao et al., 2013; Yao et al., 2018). Yao et al. (2018) pointed out that it may be due to the potential biases caused by “spatial gradients extrapolation to temporal interannual gradients” (Reichstein et al., 2007; Jung et al., 2009; Piao et al., 2013), and leaving out some cumulative effects like soil moisture (Jung et al., 2007). In addition, most of the stations used by the MTE data only had a short measurement period (Yao et al., 2018), which may affect the estimations of long-term temporal variations in GPP (e.g., IAV, trend). It is noted that the latest version of the MTE data agreed well with TBM simulations (Jung et al., 2017; Yao et al., 2018), which will be compared with the GPP estimations over China from MsTMIP in our future work.
In this study, a multi-model analysis using 12 MsTMIP-based models was used to investigate the relative contributions of climate change and anthropogenic activities to interannual and seasonal variations in China's GPP. In addition, this study examined subregional contributions to temporal changes in China's total GPP. Ensemble simulations from MsTMIP were compared with an independent upscaling GPP product (Jung et al., 2011) and with flux-tower-based GPP observations in China.
The simulated GPP for China from the 12 MsTMIP models, driven by common climate forcing, LULCC, and CO2 data, was 7.4±1.8 Pg C yr−1, which agreed well with independent MTE dataset (7.1 Pg C yr−1). In general, climate was the dominant control factor for the trends, interannual variation and seasonality of China's GPP. When only constrained by climatic driver, mean annual GPP over China from 1981 to 2010 is 6.9±1.7 Pg C yr−1, with a trend of 0.0036 Pg C yr−2. The overall rise in CO2 enhanced plant photosynthesis and thus increased total China GPP, with increasing annual mean and interannual variability, especially in northeastern and southern China, where vegetation is dense. LULCC decreased the IAV of China's total GPP by ∼7 %, whereas rising CO2 induced an increase of 8 %. Our research examined the joint effects of the three factors and their quantitative contributions to the interannual variations and seasonal cycles of GPP. Given the important role of GPP in regulating terrestrial carbon cycling, this work is expected to help us better understand the interactions of the carbon cycle, climate change and human activity. Furthermore, it will also be interesting for policy makers to make public decisions on how to achieve the balance between an optimized economy and minimized carbon loss.
Note that existing model estimates of GPP from state-of-the-art TBMs vary widely and still have large uncertainties driven by biases in environmental driver data and unrealistic assumptions in model parameterizations and parameters (Friedlingstein et al., 2006; Huntzinger et al., 2012). The multi-model ensemble strategy is a means to address model structural uncertainty by synthesizing outcomes from multiple models representing different parameterizations of underlying biogeophysical and biogeochemical processes, and it has been demonstrated to offer better predictability (Hagedorn et al., 2005). However, there are some missing factors that are not considered in this study. One is that the interaction between LULCC and elevated CO2 was not completely separated in this study. For example, deforestation against the background of rising CO2 induces higher emissions because CO2 fertilization leads to an increase in terrestrial carbon storage, but higher CO2 concentrations also cause a stronger regrowth (Houghton et al., 2012). Moreover, the uncertainty in LULCC datasets remains a serious challenge today. More satellite data with higher spatial resolution are expected to reduce this uncertainty.
The data used in this paper are available upon request. Please contact Binghao Jia at bhjia@mail.iap.ac.cn.
The supplement related to this article is available online at: https://doi.org/10.5194/esd-11-235-2020-supplement.
BJ designed the research, analyzed the data and drafted the paper with contributions from XL, XC, AJ, DNH and ZX. AJ, DNH, NZ, JM, XS, AI, YW, HT, BP, DH and KS contributed model runs to the MsTMIP products, which were used in the paper.
The authors declare that they have no conflict of interest.
This research was supported by the National Key R&D Program of China (2016YFA0600203), the Key Research Program of Frontier Sciences, CAS (QYZDY-SSW-DQC012), the National Natural Science Foundation of China (41575096, 41830967), and the Key Lab of Guangdong for Utilization of Remote Sensing and Geographical Information System, Guangzhou Institute of Geography (2017B030314138). We acknowledge the MsTMIP modelers, including Maoyi Huang from the Pacific Northwest National Laboratory, Shushi Peng from Peking University, Joshus B. Fisher from the California Institute of Technology and Daniel M. Ricciuto from the Oak Ridge National Laboratory, for contributing model output used in this work. We also thank Wenhui Kuang for providing us with the China Land Use/Cover Dataset (CLUD). Finalized MsTMIP data products are archived at the ORNL DAAC (https://daac.ornl.gov/NACP/guides/NACP_MsTMIP_TBMO.html, last access: 24 January 2016). The MTE data were downloaded freely from the Max Planck Institute for Biogeochemistry (https://www.bgc-jena.mpg.de/geodb/projects/Data.php, last access: 20 November 2017). We thank Somnath Baidya Roy and two anonymous reviewers for the helpful comments that improved the paper.
This research has been supported by the National Key R&D Program of China (grant no. 2016YFA0600203), the Key Research Program of Frontier Sciences, CAS (grant no. QYZDY-SSW-DQC012), the National Natural Science Foundation of China (grant nos. 41575096, 41830967), and the Key Lab of Guangdong for Utilization of Remote Sensing and Geographical Information System, Guangzhou Institute of Geography (grant no. 2017B030314138).
This paper was edited by Somnath Baidya Roy and reviewed by two anonymous referees.
Ahlström, A., Raupach, M., Schurgers, G., Smith, B., Arneth, A., Jung, M., Reichstein, M., Canadell, J., Friedlingstein, P., Jain, A., Kato, E., Poulter, B., Sitch, S., Stocker, B., Viovy, N., Wang, Y., Wiltshire, A., Zaehle, S., and Zeng, N.: The dominant role of semi-arid ecosystems in the trend and variability of the land CO2 sink, Science, 348, 895–899, 2015.
Anav, A., Friedlingstein, P., Beer, C., Ciais, P., Harper, A., Jones, C., Murray-Tortarolo, G., Papale, D., Parazoo, N. C., Peylin, P., Piao, S. L., Sitch, S., Viovy, N., Wiltshire, A., and Zhao, M.: Spatiotemporal patterns of terrestrial gross primary production: A review, Rev. Geophys., 53, 785–818, https://doi.org/10.1002/2015RG000483, 2015.
Boysen, L. R., Brovkin, V., Arora, V. K., Cadule, P., de Noblet-Ducoudré, N., Kato, E., Pongratz, J., and Gayler, V.: Global and regional effects of land-use change on climate in 21st century simulations with interactive carbon cycle, Earth Syst. Dynam., 5, 309–319, https://doi.org/10.5194/esd-5-309-2014, 2014.
Brovkin, V., Sitch, S., von Bloh, W., Claussen, M., Bauer, E., and Cramer, W.: Role of land cover changes for atmospheric CO2 increase and climate change during the last 150 years, Glob. Change Biol., 10, 1253–1266, 2004.
Cai, W., Yuan, W., Liang, S., Zhang, X., Dong, W., Xia, J., Fu, Y., Chen, Y., Liu, D., and Zhang, Q.: Improved estimations of gross primary production using satellite-derived photosynthetically active radiation, J. Geophys. Res.-Biogeo., 119, 110–123, https://doi.org/10.1002/2013JG002456, 2014.
Chen, J.: Rapid urbanization in China: A real challenge to soil protection and food security, Catena, 69, 1–15, https://doi.org/10.1016/j.catena.2006.04.019, 2007.
Chen, M., Rafique, R., Asrar, G. R., Bond-Lamberty, B., Ciais, P., Zhao, F., Reyer, C. P. O., Ostberg, S., Chang, J., Ito, A., Yang, J., Zeng, N., Kalnay, E., West, T., Leng, G., Francois, L., Munhoven, G., Henrot, A., Tian, H., Pan, S., Nishina, K., Viovy, N., Morfopoulos, C., Betts, R., Schaphoff, S., Steinkamp, J., and Hickler, T.: Regional contribution to variability and trends of global gross primary productivity, Environ. Res. Lett., 12, 105005, https://doi.org/10.1088/1748-9326/aa8978, 2017.
Ciais, P., Bala, G., Bopp, L., Brovkin, V., Canadell, J., Chhabra, A., DeFries, R., Galloway, J., Heimann, M., Jones, C., Le Quéré, C., Myneni, R. B., Piao, S. L., and Thornton, P.: Carbon and other biogeochemical cycles, in: Climate change 2013: The Physical Science Basis. Contribution of Working Group I to the Fifth Assessment Report of the Intergovernmental Panel on Climate Change, edited by: Stocker, T. F., Qin, D., Plattner, G.-K., Tignor, M., Allen, S. K., Boschung, J., Nauels, A., Xia, Y., Bex, V., and Midgley, P. M., Cambridge University Press, Cambridge, UK and New York, NY, USA, 465–570, 2013.
Devaraju, N., Bala, G., Caldeira, K., and Nemani, R.: A model based investigation of the relative importance of CO2-fertilization, climate warming, nitrogen deposition and land use change on the global terrestrial carbon uptake in the historical period, Clim. Dynam., 47, 173–190, https://doi.org/10.1007/s00382-015-2830-8, 2016.
Fang, J., Chen, A., Peng, C., Zhao, S., and Ci, L.: Changes in forest biomass carbon storage in China between 1949 and 1998, Science, 292, 2320–2322, https://doi.org/10.1126/science.1058629, 2001.
Fang, J., Guo, Z., Hu, H., Kato, T., Muraoka, H., and Son, Y.: Forest biomass carbon sinks in East Asia, with special reference to the relative contributions of forest expansion and forest growth, Glob. Change Biol., 20, 2019–2030, 2014.
Friedlingstein, P., Cox, P., Betts, R., Bopp, L., von Bloh, W., Brovkin, V., Cadule, P., Doney, S., Eby, M., Fung, I., Bala, G., John, J., Jones, C., Joos, F., Kato, T., Kawamiya, M., Knorr, W., Lindsay,K., Matthews, H. D., Raddatz, T., Rayner, P., Reick, C., Roeckner, E., Schnitzler, K.G., Schnur, R., Strassmann, K., Weaver, A. J., Yoshikawa, C., and Zeng, N.: Climate-carbon cycle feedback analysis: results from the C4MIP model intercomparison, J. Climate, 19, 3337–3353, 2006.
Friedlingstein, P., Houghton, R. A., Marland, G., Hackler, J., Boden, T. A., Conway, T. J., Canadell, J. G., Raupach, M. R., Ciais, P., and Le Quéré, C.: Update on CO2 emissions. Nat. Geosci., 3, 811–812, https://doi.org/10.1038/ngeo1022, 2010.
Guo, Z., Hu, H., Li, P., Li, N., and Fang, J.: Spatio-temporal changes in biomass carbon sinks in China's forests from 1977 to 2008, Sci. China Life Sci., 56, 661–671, 2013.
Hagedorn, R., Doblas-Reyes, F. J., and Palmer, T. N.: The rationale behind the success of multi-model ensembles in seasonal forecasting – I Basic concept, Tellus, 57A, 219–233, 2005.
Houghton, R. A. and Hackler, J. L.: Sources and sinks of carbon from land-use change in China, Global Biogeochem. Cy., 17, 1034, https://doi.org/10.1029/2002GB001970, 2003.
Houghton, R. A. and Nassikas, A. A.: Global and regional fluxes of carbon from land use and land cover change 1850–2015, Global Biogeochem. Cy., 31, 456–472, https://doi.org/10.1002/2016GB005546, 2017.
Houghton, R. A., House, J. I., Pongratz, J., van der Werf, G. R., DeFries, R. S., Hansen, M. C., Le Quéré, C., and Ramankutty, N.: Carbon emissions from land use and land-cover change, Biogeosciences, 9, 5125–5142, https://doi.org/10.5194/bg-9-5125-2012, 2012.
Huntzinger, D. N., Post, W. M., Wei, Y., Michalak, A. M., West, T. O., Jacobson, A. R., Baker, I. T., Chen, J. M., Davis, K. J., Hayes, D. J., Hoffman, F. M., Jain, A. K., Liu, S., McGuire, A. D., Neilson, R. P., Potter, C., Poulter, B., Price, D., Raczka, B. M., Tian, H. Q., Thornton, P., Tomelleri, E., Viovy, N., Xiao, J., Yuan, W., Zeng, N., Zhao, M., and Cook, R.: North American Carbon Project (NACP) Regional Interim Synthesis: Terrestrial Biospheric Model Intercomparison, Ecol. Model., 224, 144–157, 2012.
Huntzinger, D. N., Schwalm, C., Michalak, A. M., Schaefer, K., King, A. W., Wei, Y., Jacobson, A., Liu, S., Cook, R. B., Post, W. M., Berthier, G., Hayes, D., Huang, M., Ito, A., Lei, H., Lu, C., Mao, J., Peng, C. H., Peng, S., Poulter, B., Riccuito, D., Shi, X., Tian, H., Wang, W., Zeng, N., Zhao, F., and Zhu, Q.: The North American Carbon Program Multi-Scale Synthesis and Terrestrial Model Intercomparison Project – Part 1: Overview and experimental design, Geosci. Model Dev., 6, 2121–2133, https://doi.org/10.5194/gmd-6-2121-2013, 2013.
Huntzinger, D. N., Schwalm, C. R., Wei, Y., Cook, R. B., Michalak, A. M., Schaefer, K., Jacobson, A. R., Arain, M. A., Ciais, P., Fisher, J. B., Hayes, D. J., Huang, M., Huang, S., Ito, A., Jain, A., Lei, H., Lu, C., Maignan, F., Mao, J., Parazoo, N. C., Peng, C., Peng, S., Poulter, B., Ricciuto, D. M., Tian, H., Shi, X., Wang, W., Zeng, N., Zhao, F., Zhu, Q., Yang, J., and Tao, B.: NACP MsTMIP: Global 0.5-degree Model Outputs in Standard Format, Version 1.0. ORNL DAAC, Oak Ridge, Tennessee, USA, https://doi.org/10.3334/ORNLDAAC/1225, 2018.
Hurtt, G. C., Chini, L., Frolking, S., Betts, R., Edmonds, J., Feddema, J., Fisher, G., Goldewijk, K. K., Hibbard, K., Houghton, R., Janetos, A., Jones, C., Kinderman, G., Konoshita, T., Riahi, K., Shevliakova, E., Smith, S. J., Stefest, E., Thomson, A. M., Thornton, P., van Vuuren, D., and Wang, Y.: Harmonization of land-use scenarios for the period 1500–2100: 600 years of global gridded annual land-use transitions, wood harvest, and resulting secondary lands, Climatic Change, 109, 117–161, https://doi.org/10.1007/s10584-011-0153-2, 2011.
Ichii, K., Ueyama, M., Kondo, M., Saigusa, N., Kim, J., Alberto, M., Ardö, J., Euskirchen, E. S., Kang, M., Hirano, T., Joiner, J., Kobayashi, H., Marchesini, L., Merbold, L., Miyata, A., Saitoh, T., Takagi, K., Varlagin, A., Bret-Harte, M., Kitamura, K., Kosugi, Y., Kotani, A., Kumar, K., Li, S., Machimura, T., Matsuura, Y., Mizoguchi, Y., Ohta, T., Mukherjee, S., Yanagi, Y., Yasuda, Y., Zhang, Y., and Zhao, F.: New data-driven estimation of terrestrial CO2 fluxes in Asia using a standardized database of eddy covariance measurements, remote sensing data, and support vector regression, J. Geophys. Res.-Biogeo., 122, 767–795, https://doi.org/10.1002/2016JG003640, 2017.
IPCC: Climate change 2013: The physical science basis, in: Contribution of working group I to the fifth assessment report of the Intergovernmental Panel on Climate Change, edited by: Stocker, T. F., Qin, D., Plattner, G.-K., Tignor, M., Allen, S. K., Boschung, J., Nauels, A., Xia, Y., Bex, V., and Midgley, P. M., Cambridge University Press, Cambridge, UK, https://doi.org/10.1017/CBO9781107415324, 2013.
Ito, A., Inatomi, M., Huntzinger, D. N., Schwalm, C., Michalak, A. M., Cook, R., King, A. W., Mao, J., Wei, Y., Post, W. M., Wang, W., Arain, M. A., Huang, S., Hayes, D. J., Ricciuto, D. M., Shi, X., Huang, M., Lei, H., Tian, H., Lu, C., Yang, J., Tao, B., Jain, A., Poulter, B., Peng, S., Ciais, P., Fisher, J. B., Parazoo, N., Schaefer, K., Peng, C., Zeng, N., and Zhao, F.: Decadal trends in the seasonal-cycle amplitude of terrestrial CO2 exchange resulting from the ensemble of terrestrial biosphere models, Tellus B, 68, 28968, https://doi.org/10.3402/tellusb.v68.28968, 2016.
Ito, A., Nishina, K., Reyer, C. P. O., François, L., Henrot, A. J., Munhoven, G., Jacquemin, I., Tian, H., Yang, J., Pan, S., Morfopoulos, C., Betts, R., Hickler, T., Steinkamp, J., Ostberg, S., Schaphoff, S., Ciais, P., Chang, J., Rafique, R., Zeng, N., and Zhao, F.: Photosynthetic productivity and its efficiencies in ISIMIP2a biome models: benchmarking for impact assessment studies, Environ. Res. Lett., 12, 085001, https://doi.org/10.1088/1748-9326/aa7a19, 2017.
Jia, B., Wang, Y., and Xie, Z.: Responses of the terrestrial carbon cycle to drought over China: modeling sensitivities of the interactive nitrogen and dynamic vegetation, Ecol. Model., 368, 52–68, 2018.
Jung, M., Henkel, K., Herold, M., and Churkina, G.: Exploiting synergies of global land cover products for carbon cycle modeling, Remote Sens. Environ., 101, 534–553, https://doi.org/10.1016/j.rse.2006.01.020, 2006.
Jung, M., Vetter, M., Herold, M., Churkina, G., Reichstein, M., Zaehle, S., Ciais, P., Viovy, N., Bondeau, A., Chen, Y., Trusilova, K., Feser, F., and Heimann, M.: Uncertainties of modeling gross primary productivity over Europe: A systematic study on the effects of using different drivers and terrestrial biosphere models, Global Biogeochem. Cy., 21, GB4021, https://doi.org/10.1029/2006gb002915, 2007.
Jung, M., Reichstein, M., and Bondeau, A.: Towards global empirical upscaling of FLUXNET eddy covariance observations: validation of a model tree ensemble approach using a biosphere model, Biogeosciences, 6, 2001–2013, https://doi.org/10.5194/bg-6-2001-2009, 2009.
Jung, M., Reichstein, M., Margolis, H., Cescatti, A., Richardson, A., Arain, M., Arneth, A., Bernhofer, C., Bonal, D., Chen, J., Gianelle, D., Gobron, N., Kiely, G., Kutsch, W., Lasslop, G., Law, B., Lindroth, A., Merbold, L., Montagnani, L., Moors, E., Papale, D., Sottocornola, M., Vaccari, F., and Williams, C.: Global patterns of land-atmosphere fluxes of carbon dioxide, latent heat, and sensible heat derived from eddy covariance, satellite, and meteorological observations, J. Geophys. Res., 116, G00J07, https://doi.org/10.1029/2010JG001566, 2011.
Jung, M., Reichstein, M., Schwalm, C. R., Huntingford, C., Sitch, S., Ahlström, A., Arneth, A., Camps-Valls, G., Ciais, P., Friedlingstein, P., Gans, F., Ichii, K., Jain, A. K., Kato, E., Papale, D., Poulter, B., Raduly, B., Rödenbeck, C., Tramontana, G., Viovy, N., Wang, Y. P., Weber, U., Zaehle, S., and Zeng, N.: Compensatory water effects link yearly global land CO2 sink changes to temperature, Nature, 541, 516–520, https://doi.org/10.1038/nature20780, 2017.
Kuang, W., Liu, J., Zhang, Z., Lu, D., and Xiang, B.: Spatiotemporal dynamics of impervious surface areas across China during the early 21st century, Chinese Sci. Bull., 58, 1691–1701, https://doi.org/10.1007/s11434-012-5568-2, 2013.
Kuang, W., Liu, J., Dong, J., Chi, W., and Zhang, C.: The rapid and massive urban and industrial land expansions in China between 1990 and 2010: A CLUD-based analysis of their trajectories, patterns, and drivers, Landscape Urban Plan., 145, 21–33, 2016.
Le Quéré, C., Andrew, R. M., Friedlingstein, P., Sitch, S., Pongratz, J., Manning, A. C., Korsbakken, J. I., Peters, G. P., Canadell, J. G., Jackson, R. B., Boden, T. A., Tans, P. P., Andrews, O. D., Arora, V. K., Bakker, D. C. E., Barbero, L., Becker, M., Betts, R. A., Bopp, L., Chevallier, F., Chini, L. P., Ciais, P., Cosca, C. E., Cross, J., Currie, K., Gasser, T., Harris, I., Hauck, J., Haverd, V., Houghton, R. A., Hunt, C. W., Hurtt, G., Ilyina, T., Jain, A. K., Kato, E., Kautz, M., Keeling, R. F., Klein Goldewijk, K., Körtzinger, A., Landschützer, P., Lefèvre, N., Lenton, A., Lienert, S., Lima, I., Lombardozzi, D., Metzl, N., Millero, F., Monteiro, P. M. S., Munro, D. R., Nabel, J. E. M. S., Nakaoka, S., Nojiri, Y., Padin, X. A., Peregon, A., Pfeil, B., Pierrot, D., Poulter, B., Rehder, G., Reimer, J., Rödenbeck, C., Schwinger, J., Séférian, R., Skjelvan, I., Stocker, B. D., Tian, H., Tilbrook, B., Tubiello, F. N., van der Laan-Luijkx, I. T., van der Werf, G. R., van Heuven, S., Viovy, N., Vuichard, N., Walker, A. P., Watson, A. J., Wiltshire, A. J., Zaehle, S., and Zhu, D.: Global Carbon Budget 2017, Earth Syst. Sci. Data, 10, 405–448, https://doi.org/10.5194/essd-10-405-2018, 2018.
Li, X., Liang, S., Yu, G., Yuan, W., Cheng, X., Xia, J., Zhao, T., Feng, J., Ma, Z., Ma, M., Liu, S., Chen, J., Shao, C., Li, S., Zhang, X., Zhang, Z., Chen, S., Ohta, T., Varlagin, A., Miyata, A., Takagi, K., Saiqusa, N., and Kato, T.: Estimation of gross primary production over the terrestrial ecosystems in China, Ecol. Model., 261, 80–92, 2014.
Li, Z., Chen, Y., Li, W., Deng, H., and Fang, G.: Potential impacts of climate change on vegetation dynamics in Central Asia, J. Geophys. Res.-Atmos., 120, 12345–12356, https://doi.org/10.1002/2015JD023618, 2015.
Liu, J., Liu, M., Zhuang, D., Zhang, Z., and Deng, X.: Study on spatial pattern of land-use change in China during 1995–2000, Sci. China Ser. D, 46, 373–384, 2003.
Liu, J., Liu, M., Tian, H., Zhuang, D., Zhang, Z., Zhang, W., Tang, X., and Deng, X.: Spatial and temporal patterns of China cropland during 1990–2000: An analysis based on Landsat TM data, Remote Sens. Environ., 98, 442–456, 2005.
Liu, J., Zhang, Z., Xu, X., Kuang, W., Zhou, W., Zhang, S., Li, R., Yan, C., Yu, D., Wu, S., and Jiang, N.: Spatial patterns and driving forces of land use change in China during the early 21st century, J. Geogr. Sci., 20, 483–494, https://doi.org/10.1007/s11442-010-0483-4, 2010.
Liu, J., Kuang, W., Zhang, Z., Xu, X., Qin, Y., Ning, J., Zhou, W., Zhang, S., Li, R., Yan, C., Wu, S., Shi, X., Jiang, N., Yu, D., Pan, X., and Chi, W.: Spatiotemporal characteristics, patterns, and causes of land-use changes in China since the late 1980s, J. Geogr. Sci., 24, 195–210, https://doi.org/10.1007/s11442-014-1082-6, 2014.
Liu, J., Jia, B., Xie, Z., and Shi, C.: Ensemble simulation of land evapotranspiration in China based on a multi-forcing and multi-model approach, Adv. Atmos. Sci., 33, 673–684, https://doi.org/10.1007/s00376-016-5213-0, 2016.
Liu, M. L. and Tian, H. Q.: China's land cover and land use change from 1700 to 2005: estimations from high-resolution satellite data and historical archives, Global Biogeochem. Cy., 24, GB3003, https://doi.org/10.1029/2009GB003687, 2010.
Mao, J., Thornton, P. E., Shi, X., Zhao, M., and Post, W. M.: Remote sensing evaluation of CLM4 GPP for the period 2000–09, J. Climate, 25, 5327–5342, https://doi.org/10.1175/JCLI-D-11-00401.1, 2012.
Piao, S. L., Fang, J., Ciais, P., Peylin, P., Huang, Y., Sitch, S., and Wang, T.: The carbon balance of terrestrial ecosystems in China, Nature, 458, 1009–1013, 2009.
Piao, S. L., Ciais, P., Huang, Y., Shen, Z., Peng, S., Li, J., Zhou, L., Liu, H., Ma, Y., Ding, Y., Friedlingstein, P., Liu, C., Tan, K., Yu, Y., Zhang, T., and Fang, J.: The impacts of climate change on water resources and agriculture in China, Nature, 467, 43–51, 2010.
Piao, S. L., Ito, A., Li, S. G., Huang, Y., Ciais, P., Wang, X. H., Peng, S. S., Nan, H. J., Zhao, C., Ahlström, A., Andres, R. J., Chevallier, F., Fang, J. Y., Hartmann, J., Huntingford, C., Jeong, S., Levis, S., Levy, P. E., Li, J. S., Lomas, M. R., Mao, J. F., Mayorga, E., Mohammat, A., Muraoka, H., Peng, C. H., Peylin, P., Poulter, B., Shen, Z. H., Shi, X., Sitch, S., Tao, S., Tian, H. Q., Wu, X. P., Xu, M., Yu, G. R., Viovy, N., Zaehle, S., Zeng, N., and Zhu, B.: The carbon budget of terrestrial ecosystems in East Asia over the last two decades, Biogeosciences, 9, 3571–3586, https://doi.org/10.5194/bg-9-3571-2012, 2012.
Piao, S. L., Sitch, S., Ciais, P., Friedlingstein, P., Peylin, P., Wang, X., Ahlström, A., Anav, A., Canadell, J. G., Cong, N., Huntingford, C., Jung, M., Levis, M., Levy, P. E., Li, J., Lin, X., Lomas, M. R., Lu, M., Luo, Y., Ma, Y., Myneni, R. B., Poulter, B., Sun, Z. Z., Wang, T., Viovy, N., Zaehle, S., and Zeng, N.: Evaluation of terrestrial carbon cycle models for their response to climate variability and to CO2 trends, Glob. Change Biol., 19, 2117–2132, 2013.
Pongratz, J., Reick, C. H., Houghton, R. A., and House, J. I.: Terminology as a key uncertainty in net land use and land cover change carbon flux estimates, Earth Syst. Dynam., 5, 177–195, https://doi.org/10.5194/esd-5-177-2014, 2014.
Reichstein, M., Papale, D., Valentini, R., Aubinet, M., Bernhofer, C., Knohl, A., Laurila, T., Lindroth, A., Moors, E., Pilegaard, K., and Seufert, G.: Determinants of terrestrial ecosystem carbon balance inferred from European eddy covariance flux sites. Geophys. Res. Lett., 34, L01402. https://doi.org/10.1029/2006GL027880, 2007.
Schimel, D., Stephens, B. B., and Fisher, J. B.: Effect of increasing CO2 on the terrestrial carbon cycle, P. Natl. Acad. Sci. USA, 112, 436–441, 2014.
Schwalm, C. R., Huntzinger, D. N., Fisher, J. B., Michalak, A. M., Bowen, K., Ciais, P., Cook, R., El-Masri, B., Hayes, D., Huang, M., Ito, A., Jain, A., King, A.W., Lei, H., Liu, J., Lu, C., Mao, J., Peng, S., Poulter, B., Ricciuto, D., Schaefer, K., Shi, X., Tao, B., Tian, H., Wang, W., Wei, Y., Yang, J., and Zeng, N.: Toward “optimal” integration of terrestrial biosphere models, Geophys. Res. Lett., 42, 4418–4428, https://doi.org/10.1002/2015GL064002, 2015.
Sen, P. K.: Estimates of the regression coefficient based on Kendall's tau, J. Am. Stat. Assoc., 63, 1379–1389, 1968.
Song, W., and Deng, X.: Land-use/land-cover change and ecosystem service provision in China, Sci. Total Environ., 576, 705–719, 2017.
Tao, B., Tian, H., Chen, G., Ren, W., Lu, C., Alley, K. D., Xu, X., Liu, M., Pan, S., and Virji, H.: Terrestrial carbon balance in tropical Asia: Contribution from cropland expansion and land management, Global Planet. Change, 100, 85–98, 2013.
Tian, H., Melillo, J., Lu, C., Kicklighter, D., Liu, M., Ren, W., Xu, X., Chen, G., Zhang, C., Pan, S., Liu, J., and Running, S.: China's terrestrial carbon balance: Contribution of multiple global change factors. Global Biogeochem. Cy., 25, GB1007, https://doi.org/10.1029/2010GB003838, 2011a.
Tian, H., Xu, X., Lu, C., Liu, M., Ren, W., Chen, G., Melillo, J., and Liu, J.: Net exchanges of CO2, CH4, and N2O between China's terrestrial ecosystems and the atmosphere and their contributions to global climate warming, J. Geophys. Res. 116, G02011, https://doi.org/10.1029/2010JG001393, 2011b.
Wang, S., van Kooten, G. C., and Wilson, B.: Mosaic of reform: Forest policy in post-1978 China, Forest Policy Econ., 6, 71–83, 2004.
Wei, Y., Liu, S., Huntzinger, D. N., Michalak, A. M., Viovy, N., Post, W. M., Schwalm, C. R., Schaefer, K., Jacobson, A. R., Lu, C., Tian, H., Ricciuto, D. M., Cook, R. B., Mao, J., and Shi, X.: The North American Carbon Program Multi-scale Synthesis and Terrestrial Model Intercomparison Project – Part 2: Environmental driver data, Geosci. Model Dev., 7, 2875–2893, https://doi.org/10.5194/gmd-7-2875-2014, 2014a.
Wei, Y., Liu, S., Huntzinger, D. N., Michalak, A. M., Viovy, N., Post, W. M., Schwalm, C. R., Schaefer, K., Jacobson, A. R., Lu, C., Tian, H., Ricciuto, D.M., Cook, R.B., Mao, J., and Shi, X.: NACP MsTMIP: Global and North American Driver Data for Multi-Model Intercomparison, ORNL DAAC, Oak Ridge, Tennessee, USA, https://doi.org/10.3334/ORNLDAAC/1220, 2014b.
Xiao, J. F., Zhou, Y., and Zhang, L.: Contributions of natural and human factors to increases in vegetation productivity in China, Ecosphere, 6, 1–20, 2015.
Yao, Y., Wang, X., Li, Y., Wang, T., Shen, M., Du, M., He, H., Li, Y., Luo, W., Ma, M., Ma, Y., Tang, Y., Wang, H., Zhang, X., Zhang, Y., Zhao, L., Zhou, G., and Piao, S. L.: Spatiotemporal pattern of gross primary productivity and its covariation with climate in China over the last thirty years, Glob. Change Biol., 24, 184–196, 2018.
Yuan, W., Liu, S., Yu, G., Bonnefond, J., Chen, J., Davis, K., Desai, A. R., Goldstein, A. H., Damiano, Gianelle, D., Rossi, F., Suyker, A. E., and Verma, S. B.: Global estimates of evapotranspiration and gross primary production based on MODIS and global meteorology data, Remote Sens. Environ., 114, 1416–1431, 2010.
Zaehle, S., Friend, A. D., Friedlingstein, P., Dentener, F., Peylin, P., and Schulz, M.: Carbon and nitrogen cycle dynamics in the O-CN land surface model: 2. Role of the nitrogen cycle in the historical terrestrial carbon balance, Global Biogeochem. Cy., 24, GB1006, https://doi.org/10.1029/2009GB003522, 2010.
Zhang, Y., Peng, C., Li, W., Tian, L., Zhu, Q., Chen, H., Fang, X., Zhang, G., Liu, G., Mu, X., Li, Z., Li, S., Yang, Y., Wang, J., and Xiao, X.: Multiple afforestation programs accelerate the greenness in the “Three North” region of China from 1982 to 2013, Ecol. Indic., 61, 404–412, 2016.
Zhang, Z., Wang, X., Zhao, X., Liu, B., Yi, L., Zuo, L., Wen, Q., Liu, F., Xu, J., and Hu, S. A 2010 update of National Land Use/Cover Database of China at 1 : 100 000 scale using medium spatial resolution satellite images, Remote Sens. Environ., 149, 142–154, 2014.
Zhou, S., Zhang, Y., Kelly, K., Luo, Y., Xiao, X., Ciais, P., Huang, Y., and Wang, G.: Explaining inter-annual variability of gross primary productivity from plant phenology and physiology, Agr. Forest Meteorol., 226–227, 246–256, 2016.
Zhu, X., Yu, G., He, H., Wang, Q., Chen, Z., Gao, Y., Zhang, Y., Zhang, J., Yan, J., Wang, H., Zhou, G., Jia, B., Xiang, W., Li, Y., Zhao, L., Wang, Y., Shi, P., Chen, S., Xin, X., Zhao, F., Wang, Y., Tong, C., Fu, Y., Wen, X., Liu, Y., Zhang, L., Zhang, L., Su, W., Li, S., and Sun, X.: Geographical statistical assessments of carbon fluxes in terrestrial ecosystems of China: Results from upscaling network observations, Global Planet. Change, 118, 52–61, 2014.