the Creative Commons Attribution 4.0 License.
the Creative Commons Attribution 4.0 License.
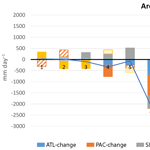
A new pattern of the moisture transport for precipitation related to the drastic decline in Arctic sea ice extent
Luis Gimeno-Sotelo
Raquel Nieto
Marta Vázquez
Luis Gimeno
In this study we use the term moisture transport for precipitation for a target region as the moisture coming to this region from its major moisture sources resulting in precipitation over the target region (MTP). We have identified changes in the pattern of moisture transport for precipitation over the Arctic region, the Arctic Ocean, and its 13 main subdomains concurrent with the major sea ice decline that occurred in 2003. The pattern consists of a general decrease in moisture transport in summer and enhanced moisture transport in autumn and early winter, with different contributions depending on the moisture source and ocean subregion. The pattern is statistically significant and consistent with changes in the vertically integrated moisture fluxes and frequency of circulation types. The results of this paper also reveal that the assumed and partially documented enhanced poleward moisture transport from lower latitudes as a consequence of increased moisture from climate change seems to be less simple and constant than typically recognised in relation to enhanced Arctic precipitation throughout the year in the present climate.
- Article
(3139 KB) - Full-text XML
- Companion paper
-
Supplement
(756 KB) - BibTeX
- EndNote
The shrinking of the cryosphere since the 1970s is among the most robust signals of climate change identified in the last IPCC Assessment Report (IPCC, 2013). There is little doubt that this change is a result of global warming caused by increased anthropogenic greenhouse gas emissions (AR5; IPCC, 2013). The Arctic is of particular scientific and environmental interest; the rise in Arctic near-surface temperature doubles the global average in almost all months (e.g. Screen and Simmonds, 2010; Tang et al., 2014; Cohen et al., 2014). Without doubt, the most important indicator of Arctic climate change is sea ice extent, which is characterised by a very significant decline since the 1970s (Tang et al., 2014; IPCC, 2013). This decline has accelerated in recent decades in terms of both extent and thickness to the point at which a summer ice-free Arctic Ocean is expected to occur within the next few decades (IPCC, 2013). Changes in atmospheric and oceanic circulation with implications for Arctic mid-latitude climate or reductions and shifts in the distribution of oceanic and terrestrial fauna are among the most concerning and already apparent impacts of this decline in sea ice (Screen and Simmonds, 2010; Post et al., 2013). The scientific mechanisms involved in Arctic sea ice extent (SIE) are multiple and varied; atmospheric processes (Ogi and Wallace, 2007; Rigor et al., 2002) interact closely and nonlinearly with hydrological and oceanographic processes (Zhang et al., 1999, 2012; Årthun et al., 2012). Changes in atmospheric circulation can affect SIE via dynamical (e.g. changes of surface winds) or thermodynamic (i.e. changes in heat and moisture fluxes in the Arctic; Tjernström et al., 2015; Ding et al., 2017) factors. Among these effects, change in moisture transport has emerged as one of the most important with respect to the greenhouse effect (Koenigk et al., 2013; Graversen and Burtu, 2016; Vihma et al., 2016), and is related to SIE decline through hydrological mechanisms such as changes in Arctic river discharges (Zhang et al., 2012), radiative mechanisms such as anomalous downward longwave radiation at the surface (Woods et al., 2013; Park et al., 2015a; Mortin et al., 2016; Woods and Caballero, 2016; Lee et al., 2017), or meteorological mechanisms such as changes in the frequency and intensity of cyclones crossing the Arctic (Rinke et al., 2017). Because the effects of enhanced moisture transport on Arctic ice are diverse, there is no direct relationship between enhanced transport and SIE decline. Anomalously high moisture transport into the Arctic is associated with intense surface winds, and increment in moisture content and induced radiative warming, which lead to decreased SIE (Kapsch et al., 2013; Park et al., 2015b). However, anomalous moisture transport can result in anomalous precipitation, and in this case, the relation between enhanced moisture transport and diminished SIE is unclear because changes in precipitation are not always related to SIE in the same way, depending on the type of precipitation and the season. Snowfall on sea ice enhances thermal insulation and thus reduces sea ice growth in winter (Leppäranta, 1993), but increases the surface albedo and thus reduces melt in spring and summer (Cheng et al., 2008). In contrast, rainfall is generally related to sea ice melt, and for both snowfall and rainfall, flooding over the ice favours the formation of superimposed ice and potentially increases in the Arctic sea ice thickness.
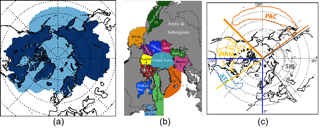
Figure 1(a) The Arctic region (AR) included in the present work defined following the definition of Roberts et al. (2010). (b) The Arctic Ocean (OA) and its 13 subregions as described by Boisvert et al. (2016). (c) Major moisture sources for the Arctic as detected by Vázquez et al. (2016). The coloured longitudinal lines mark the areas used for the types of circulation analysis in Fig. 11.
In this study we use the term moisture transport for precipitation (MTP) for a target region as the moisture coming to this region from its major moisture sources that then results in precipitation over it. We focus on identifying the patterns of moisture transport for precipitation in the Arctic region (AR), the Arctic Ocean (AO) as a whole, and its 13 main subdomains, which fit better with sea ice decline. For this purpose, we have studied the different patterns of moisture transport for the cases of high and low SIE linked to periods before and after the main change point (CP) in the SIE. The study differs significantly from our previous work, Vázquez et al. (2017), in which we analysed the influence of the transport of moisture on the two most important sea ice minimum events (2007 and 2012) based mostly on an analysis of anomalies. In this paper we analysed the long-term changes in the moisture transport concurrent with long-term changes in sea ice (sea ice decline).
2.1 Data
The target region in this study comprises the AR (Fig. 1a) as defined by Roberts et al. (2010) and used by Vázquez et al. (2016), the AO as a whole, and its 13 main subdomains as defined in Fig. 1b (Boisvert et al., 2015). The analysed period was from 1 January 1980 to 31 December 2016. For Arctic SIE, we used daily, monthly, and annual data from the US National Snow and Ice Data Center (Fetterer et al., 2016). To implement the Lagrangian approach, by which moisture transport is estimated to approximate vertical integrated moisture fluxes and circulation types, we used the European Centre for Medium-Range Weather Forecasts (ECMWF) ERA-Interim reanalysis (Dee et al., 2011). Data from this reanalysis cover the period from January 1979 to the present and extending forward continuously in near-real time. These data are available at 6 h intervals at a 1∘ × 1∘ spatial resolution in latitude and longitude for 61 vertical levels (1000 to 0.1 hPa). The ERA-Interim reanalysis is typically considered to have the highest quality relative to other reanalysis data for the water cycle (Lorenz and Kunstmann, 2012) and is especially appropriate over the AR (Jakobson et al., 2012), with better representation of mass fluxes including water vapour (Graversen et al., 2011).
2.2 Methods
2.2.1 Calculation of sea ice extent anomalies
Daily, monthly, and annual data of SIE from 1980 to 2016 taken from the National Snow and Ice Data Center (Fetterer et al., 2016) were used to build four different series of ice extent anomalies for the AO and its 13 subregions. These four series were constructed as follows.
-
DS (in millions of square kilometres): 365 daily anomaly series (size of each series: 37 data points); for each daily value, the average for the same calendar day over the 37-year period is subtracted (for instance, the series for 21 September comprises the anomaly of each 21 September vs. the average of the 37 data points from this date).
-
MS (in millions of square kilometres): 12 monthly anomaly series (size of each series: 37 data points); for each monthly value, the average for the same month over the 37-year period is subtracted (for instance the series for September results of the anomaly of each September vs. the average of the 37 September data points).
-
ADS (in millions of square kilometres): one series of all daily anomalies (size of the series: 13 505 data points) built by ordering the daily anomalies in DS from 1 January 1980 to 31 December 2016.
-
AMS (in millions of square kilometres): one series of all monthly anomalies (size of the series: 444 data points) built by ordering the monthly anomalies in MS from January 1980 to December 2016.
2.2.2 Detection of change points in Arctic sea ice extent
We have used several methods to estimate CPs in Arctic SIE. As usual in time series analysis a CP detection tries to identify times when the time series in mean or variance changed; in this case we were interested mainly in changes in mean. Changes in mean for the Arctic SIE are equivalent to a decrease, higher Arctic SIE values before the CP and lower after it.
CPs of each of these series for the AO were calculated using three different methods, one detecting single CPs (at most one change, AMOC) and two detecting multiple CPs (binary segmentation, BinSeg; and pruned exact linear time, PELT).
AMOC uses a test to detect a hypothesised single CP. The null hypothesis refers to no CP, and its maximum log likelihood is given by , where p(⋅) is the probability density function associated with the distribution of the data and is the maximum likelihood estimate of the parameters. For the alternative hypothesis, a CP at τ1 is considered, with . The expression for the maximum log likelihood for a given τ1 is ML.
Taking into account that the CP location is discrete in nature, maxτ1ML(τ1) is the maximum log-likelihood value under the alternative hypothesis, where the maximum is taken over all possible CP locations. Consequently, the test statistic is . The null hypothesis is rejected if λ > C, where C is a threshold of our choice. When detecting a CP, its position is estimated as , which is the value of that maximises ML(τ1).
BinSeg and PELT function as follows: by summing the likelihood for each of the m segments, the likelihood test statistic can be extended to multiple changes. However, there is a problem in identifying the maximum of ML(τ1:m) over all possible combinations of τ1:m. The most common approach to resolve this problem is to minimise
where C is a cost function for a segment, such as the negative log likelihood, and βf(m) is a penalty to guard against over-fitting. The BinSeg method starts with applying a single CP test statistic to the entire data set. If a CP is identified, the data are then split into two at the CP location. The single CP process is repeated for the two new data sets, before and after the change. If CPs are identified in either of the new data sets, they are split further. This procedure continues until no CPs are found in any parts of the data. This process is an approximate minimisation of Eq. (1) with f(m)=m, as any CP locations are conditional on CPs identified previously. The segment neighbourhood algorithm precisely minimises the expression given by Eq. (1) using a dynamic programming technique to obtain the optimal segmentation for (m+1) CPs reusing the information calculated for m CPs. The PELT method is similar to that of the segment neighbourhood algorithm in that it provides an exact segmentation. We have used the PELT algorithm instead of the segment neighbourhood method as it has proven to be more computationally efficient. The reason for this greater efficiency is the use of dynamic programming and pruning in the algorithm's construction.
A full description of these methods and the subroutines in R used in this study can be found in Killick and Eckley (2014).
The three different CP detection methods were used for the four different Arctic SIE time series defined in Sect. 2.2.1. Figure 2 illustrates the detection of the CP in mean for two series (ADS and AMS) using the AMOC method. The top plot represents the 13 505-value Arctic ice extent anomaly series consisting of all days from 1 January 1980 to 31 December 2016 (ADS series). There are two horizontal lines representing the mean of the values before and after the CP identified by the AMOC method (the 8660th day that corresponds to 22 September 2003). Those means are 0.27 and −0.91 million km2, respectively. The graphic at the bottom portrays the 444-value Arctic ice extent anomaly series consisting of all months from January 1980 to December 2016 (AMS series). As in the previous one, there are two horizontal lines, which correspond to the SIE mean before and after the AMOC CP (the 286th month of the series, that is October 2003). Those means are 0.41 and −0.74 million km2, respectively.
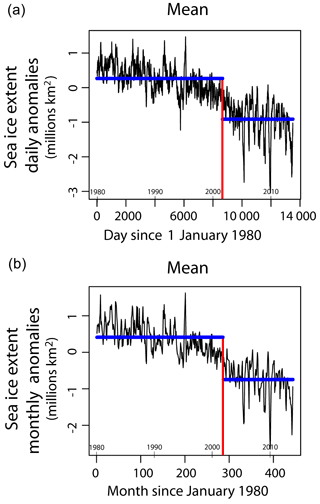
Figure 2Example of change point detection in mean for two series and one method, AMOC. Panel (a) represents the change points in the ADS series. The two horizontal lines represent the mean of the values before and after the change point identified with the AMOC method (8660th day – 22 September 2003). Those means are 0.27 and −0.91 million km2, respectively. Panel (b) represents the change points in the AMS series. The two horizontal lines represent the mean of the values before and after the change point identified with the AMOC method (286th month – October 2003). Those means are 0.41 and −0.74 million km2, respectively.
2.2.3 Estimation of the Lagrangian moisture transport from the main sources
In this study, we used a Lagrangian approach to calculate moisture transport from the main moisture sources as detected by Vázquez et al. (2016) (Fig. 1c) for the AR, AO, and its 13 subregions. This approach is based on the particle dispersion model FLEXPART v9.0 (i.e. the FLEXible PARTicle dispersion model of Stohl and James, 2004, 2005) forced by ERA-Interim data from the ECMWF. This approach has been used extensively in moisture transport analysis (e.g. Gimeno et al., 2010, 2013), and a review of its advantages and disadvantages versus other approaches for tracking water vapour was summarised by Gimeno et al. (2012 and 2016). To briefly summarise this method, the atmosphere is divided into so-called particles (finite elements of volume with equal mass) and individual three-dimensional trajectories are tracked backward or forward in time for 10 days, the average residence time of water vapour in the atmosphere (Numaguti, 1999). Then, taking into account the changes in (q) for each particle along its trajectory, the net rate of change of water vapour (e−p) for every particle, , is estimated, with e and p representing evaporation and precipitation, respectively. The total atmospheric moisture budget (E−P) is estimated by adding up (e−p) for all particles over a given area at each time step used in the analysis. If we follow the particles backward in time for a target region, positive (E−P) values identify the main moisture sources for this target region; if we follow the particles forward in time from a source region, negative (E−P) values identify the main moisture sinks of the source region. In this study we have used four predefined moisture sources, those identified as the major sources of moisture for the AR in Vázquez et al. (2016) following all the particles reaching the AR for the period 1980–2012 backward in time and taking the regions showing positive (E−P) values greater than the 90th percentile (taking into account global values of positive E−P). Then, to compute MTP from each of these four sources to each sink for the AO, the trajectories of particles from the moisture sources for the AR were followed forward in time from every source region detected by Vázquez et al. (2016) (Fig. 1c). This was carried out for 6 h in the period 1980–2016. Then, we selected all particles losing moisture, (e−p) < 0, at the sinks (the whole Arctic or any of the subregions), and by adding e−p for all of these particles, we estimated moisture transport for precipitation from the source to the sink ((E−P) < 0) at daily, monthly, or yearly scales. A schematic illustration of this approach is displayed in Fig. 3. A couple of clarifications on this approach are necessary to avoid misunderstandings of the results. The first one is that particles can gain moisture in the regions placed between those defined as the major moisture sources and the target region, even in the target region itself. However as our defined moisture regions were identified as the major moisture sources in the backward analysis (Vázquez et al., 2016) the contribution of the intermediate regions is much lower. A look at Fig. 2 from Vázquez et al. (2016) shows that intermediate regions are not net sources (particles reaching the Arctic region lost (not gained) moisture in these regions on average). In any case not all the precipitated water comes from the major sources; those particles that were not within those major source regions 10 days before precipitation are responsible for the rest of precipitation, including the particles coming from the AR itself, which account for the important contribution of local moisture recirculation. The second clarification is concerned with the size of the target regions and the number of particles reaching them. As commented on in the seminal description of the approach (Stohl and James, 2004, 2005), it works better for large regions. The size of the target regions in this study (Arctic region and subregions) is bigger than in many of the regions where the same methodology was used in previous studies (e.g. Ramos et al., 2016 or Wegmann et al., 2015) and the average number of particles by source that reach the target regions high enough daily (see Table S1 in the Supplement).
2.2.4 Identification of circulation types
The patterns of (E−P) < 0 can change daily in association with variations in atmospheric circulation. To evaluate the relationship between this variability and the moisture supply generated from each source of moisture, we identified different circulation types (CTCs) over the areas of interest using a methodology developed by Fettweis et al. (2011). This approach consists of an automated circulation type classification based on a correlation analysis whereby atmospheric circulation is categorised into a convenient number of four discrete circulation types in the present study. For each pair of days, a similarity index based on the correlation coefficient was calculated for the purpose of grouping days that showed similar circulation patterns (Belleflamme et al., 2012). The first category contains the greatest number of similar days, where similarity is defined by a particular threshold (0.95 for the first class). After establishing the first class, the same procedure was applied for the remaining days using a lower similarity threshold to find the second and then all other classes. The complete procedure was repeated for different thresholds to optimise the percentage of variance explained (Philipp et al., 2010). This method is termed a “leader” algorithm because each class is represented by a leader pattern considered to be the reference day (Philipp et al., 2010). In this study, we used the geopotential height field at 850 hPa from ERA-Interim. Because our aim was to find and analyse different circulation patterns affecting the Arctic system linked to each source of moisture, the Northern Hemisphere from 20 to 90∘ N was divided into sections according to the positions of the major sources of moisture (Fig. 1c). The size of the sections was 70∘ latitude × 90∘ longitude. Thus, the circulation type classification was obtained seasonally for each of these sections. The use of a regional domain centred in the moisture source is justified to account for regional modes instead of annular ones, which could not catch details in regional circulation. As changes in the size of the sections can lightly vary the circulation types (Huth et al., 2008) we performed a sensibility analysis (not shown) by moving the domain 10∘ eastward and westward and by extending the domain 10∘ eastward with similar results, which showed that the patterns are very coherent.
3.1 Climatological moisture transport for precipitation to the Arctic region and Arctic Ocean subregions
Figure 4 displays the seasonal cycle of MTP to the AR from four major sources (Atlantic, Pacific, Siberia, and North America) and the relative contributions of each. MTP to the AR exhibits a marked seasonal cycle with a maximum in summer and a minimum in winter; MTP doubles in the highest month (August) relative to the lowest month (February). The percentage of the contribution from each source is relatively constant throughout the year, with the Pacific, North America, Siberia, and the Atlantic contributing about 35, 30, 20, and 15 %, respectively.
The seasonal cycle is quite similar for eight of the 13 AO subregions (Fig. 5), with two other regions (Barents and the central Arctic) exhibiting similar maxima, but with minima in May, and another (Chukchi Sea) with two minima in March and November, and finally Greenland with a minimum in May but no clear maximum in summer, extending the typical summer high values to autumn and winter. The relative contributions of each moisture source are very diverse, with three general patterns: one with the closest source dominating (a Pacific source for the Bering, Chukchi, and Okhotsk seas, a North American source for the Canadian Arctic Archipelago and Hudson Bay, and a Siberian source for the Kara and Laptev seas), another pattern in which two sources share importance throughout the year (Atlantic and Siberian sources for Baffin Bay and Greenland, and Pacific and North American sources for the Beaufort Sea), and a third in which the Siberian source shares importance with two or more sources (Barents Sea, central Arctic, and eastern Siberia).
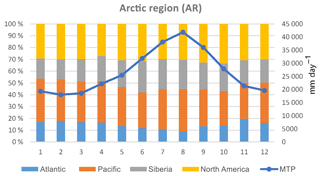
Figure 4Seasonal cycle of moisture transport for precipitation (MTP) to the Arctic region (AR) from the four major sources (Atlantic, Pacific, Siberia, and North America). Values at the right represent absolute values of transport, and those at the left indicate the relative contribution of each source by percentage.
The relative importance of each ocean subregion for MTP to the AO (estimated as the sum of the 13 subregions) is shown in Fig. 6, where the percentage of moisture transport to the whole AO is displayed by region and month. There are four regions where the aggregated MTP received represents more than 60 % of the MTP received for the whole Arctic. Those regions are Greenland, Baffin Bay, the Bering Sea, and the central Arctic, in order of importance. The contributions of Baffin Bay, the Bering Sea, and the central Arctic vary little throughout the year, representing about 20, 12, and 10 % of the MTP received for the whole Arctic, respectively, whereas the contribution of Greenland has a marked seasonal cycle with values around 25 % for autumn and winter, and 10–15 % in spring and summer, seasons when two other sources gain importance, the Hudson Bay and Okhotsk Sea, with percentages close to 10 %. The almost constant contribution of the Barents Sea throughout the year is non-negligible, around 5 %. The remaining sources have contributions lesser than 5 %.
3.2 Moisture transport after and before Arctic sea ice change points
Figure 7 summarises the identified CPs in means identified using the AMOC method in the four series of SIE anomalies (DS, MS, ADS, and AMS) from 1980 to 2016 for the whole Arctic.
Blue points refer to the CPs in DS; for instance, the 21 July daily anomaly series (size of the series: 37 data points, representing 37 annual anomalies of the values of the SIE for the 37 values on 21 July) occurred in 2004. DS only has CPs in the period from July to October. These CPs occurred mostly in 2004, the first part of September in 2006, and the last week of October in 2003. The results of both the BinSeg and PELT methods (results not shown) coincide for every day except for 17 September (2004 based on AMOC and 2006 for the other methods). The red bands in Fig. 7 portray the CPs in the MS data set (July–October). For instance, July monthly anomaly series (size of each series: 37 data points, representing 37 annual anomalies of the values of the SIE for the 37 values in the average monthly July) occurred in 2004. These CPs occurred in 2004 for July and September, a little earlier (2001) for August, and a little later (2005) for October. Both the BinSeg and PELT methods coincide in every month except for October (2005 based on AMOC and BinSeg, and 2006 for PELT). The single green square corresponds to the CP in the 13 505-value series consisting of all days from 1 January 1980 to 31 December 2016 (ADS). The CP occurred on 22 September 2003 and was identified by the BinSeg, although not by PELT (the closest ones are 4 August 2002 and 26 January 2005). The single purple line represents the CP in the 444-value series consisting of all months from January 1980 to December 2016 (AMS). This CP occurred in October 2003. The results of both the BinSeg and PELT methods coincide for this CP, and it is the only one for both. Overall, the results in Fig. 7 suggest that 2003 is the most appropriate year for analysis of differences in moisture transport after and before a single CP date. The average values for ADS before and after the CP were 0.27 and −0.91 million km2 and for AMS 0.41 and −0.74 million km2. The analysis of changes in MTP for the multiple subperiods identified by BinSeg and PELT would merit analysis, but it is out of the objective of this paper.
Figure 8 portrays the differences between mean values of MTP until 2003 and mean values after 2003 for every source region (Fig. 1c) for the AR, which includes continental and oceanic areas (Fig. 1a). The quantities in the plot result from averaging daily values of MTP. The statistical significance of the differences has been estimated by comparing daily values of MTP before and after the CP, and the sample size is large enough (30 × 23 years vs. 30 × 13 years) to permit application of Student's t test. The pattern of changes in MTP before and after the CP for the AR shows no significant changes in late winter and spring, a significant decrease in MTP in summer, and increased MTP in autumn and early winter, with the exception of October. The summer decrease is statistically significant for the contributions of Pacific and Siberian sources throughout the entire summer, for the Atlantic source in early summer, and for the North American source in late summer. The autumn–early winter increase is statistically significant (red asterisks) for the contributions of the North American source in three months (September, November, and December), for the Siberian and the Atlantic sources in two months (September and November and October and December, respectively), and for the Pacific source only in one month (November). As, according to Fig. 7 mainly for DS and MS, 2004 could also be interpreted as the main CP, we tested results of changes in MTP by changing 2003 by 2004 with almost identical results (not shown).
These results are coherent with any of the mechanisms referred in the introduction. Thus, (i) a lower MTP in early summer (as occurred since 2003) implies lower precipitation (in this season mainly as snowfall), which would result in a decrease in the surface albedo and thus increasing melt (Cheng et al., 2008); (ii) a lower MTP in late summer (as has occurred since 2003) could be due to a less probability of occurrence of rainfall storms with possible flooding over the ice, which would favour the formation of superimposed ice and consequently is consistent with increasing melt; (iii) a higher MTP in early autumn (September) (as has occurred since 2003) implies higher precipitation (in this season mainly as rainfall), something generally related to sea ice melt; (iv) a higher MTP in late autumn and early winter (as has occurred since 2003) implies higher precipitation (in this season mainly as snowfall), enhancing thermal insulation and thus reducing sea ice growth in (Leppäranta, 1993). The rigorous checking of these implications merits further analysis but it is out of the scope of this paper since it would imply knowing details over the precipitation form (snow or rain) for the different Arctic regions with good temporal and geographical resolution, and even to analyse specific precipitation episodes to know if these are responsible for flooding or not.
However, because Arctic ice cover is extensive in geographical domain covering the subregion affected by very different atmospheric circulation patterns, this pattern of change in MTP could be non-homogeneous for the entire AO and its subregions. Finer-scale analysis can be performed by restricting the analysis to oceanic areas only (the 13 AO subregions and the whole AO defined as the sum of these regions, Fig. 1b). The differences between mean values of MTP until 2003 and mean values after 2003 for every source region for the AO (Fig. 9 top left) is quite similar to the AR without significant changes in late winter and spring, with decreased MTP in summer and increased MTP in autumn and early winter, now also including October. Small differences are observed in the contributions of each source to the change of MTP toward the AO, with the Pacific source becoming much less important for the summer decrease and more important in the autumn increase. The consistent increment is especially remarkable after the CP of MTP for all the moisture sources in September, the month when Arctic SIE is lowest.
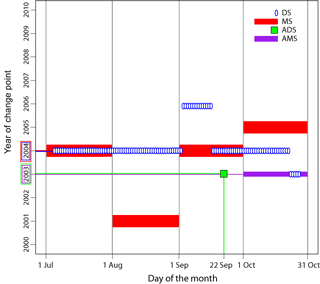
Figure 7Summary of the identified change points (CPs) in means identified using the AMOC method with the four series of ice extent anomalies for the whole Arctic (DS, MS, ADS, and AMS) from 1980 to 2016. The blue points refer to the change points in DS; the red lines portray change points in the MS (July–October). The green square corresponds to the change point in the ADS, and the purple line represents the change point in the AMS.
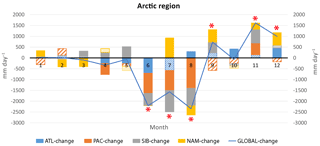
Figure 8Differences between mean values of moisture transport for precipitation (MTP) until 2003 and mean values after 2003 for every source region. Filled bars show those differences that are statistically significant at the 95 % confidence level for decreases after the CP. Statistical significance of the differences in total MTP (sum of the four sources) is displayed with a red asterisk.
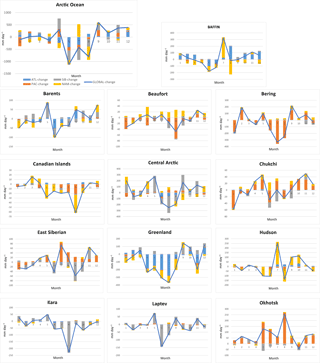
Figure 9Differences between mean values of moisture transport for precipitation (MTP) until 2003 and mean values after 2003 for every source region for the Arctic Ocean (AO) and its 13 subregions. Statistical significance of the differences is displayed in Table 1.
Table 1Summary of the statistical significance of the MTP differences estimated by comparing daily values of moisture transport before and after the CP, based on the Student's t test. Circles indicate statistical significance at the 95 % confidence level for increases after the CP; crosses indicate statistical significance at the 95 % confidence level for decreases after the CP.
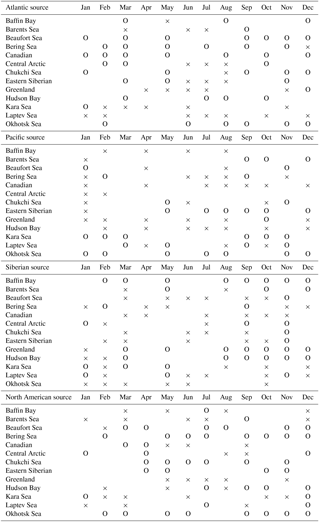
The analysis by subregion allowed us to identify which subregions contributed most to change in MTP in the AO. As in the case of the AR, the statistical significance of the differences (Table 1) has been estimated by comparing daily values of moisture transport before and after the CP. The diminished contribution of the Atlantic source to the AO in June and July is mainly attributed to the reduction of transport to Greenland; this decrease is not observed in August because of the compensation for the decrease to Greenland by the increase to Baffin Bay. A moderate increase in October and a small decrease in December were also attributed to changes occurring in Greenland. The slightly decreased contribution of the Pacific to the AO in summer was caused by the compensation for the decreased contribution of the Bering Sea (strong decrease) by a strong increase in the contribution of the Okhotsk Sea, with some influence of other regions such as the Beaufort Sea (decrease) and eastern Siberia (increase). There is greater concordance in autumn and winter, with generally slight increments, which resulted in a small increase in the contribution of the Pacific to the AO. The greatly decreased contribution of the Siberian source to the AO in summer is attributed mainly to the strong decrease in MTP to the central Arctic and Laptev Sea, and the generally slight increment of the contribution of the Siberian source for these regions in autumn and early winter (with the exception of October); these changes result in similar behaviour for the AO. The different changes of the contribution of the North American source to the AO in July (increase) and August (decrease) reproduce the common changes observed in the contributions to the Baffin and Hudson bays. The slight increase in contribution to the AO in September and October again is attributed to compensation for the increase to Hudson Bay by a strong decrease in September to the Canadian Arctic Archipelago and light decrease in October to Baffin Bay and the Barents Sea; the increased contribution of this source to the AO in December is the result of increased contribution to the central Arctic and the Barents Sea, partially compensated for by a slight decrease to the Hudson and Baffin bays.
3.3 Checking the Lagrangian results by analysing changes in vertical integrated moisture fluxes and atmospheric circulation patterns after and before Arctic sea ice change points
It is useful to check the results derived from the Lagrangian approach to estimate MTP with other Eulerian approaches such as the computation of vertical integrated moisture flux (VIMF). Supplement Fig. S1 shows the climatological VIMF by month in the period from June to December (left panel) and the difference between the periods after the CP and before the CP for the zonal component (central panel) and the meridional component of VIMF (right panel). This methodology cannot estimate specific changes in the MTP from each source to each sink as the more sophisticated Lagrangian approach does, but it is able to show whether patterns are compatible with the identified changes. For instance, in June (Fig. 10), the Atlantic source provides moisture for precipitation in the subregion of the Arctic Ocean named Greenland in our study, as revealed by the flux vectors in the top panel. However, the track of moisture from the source to the sink is clearly hindered for the period after the CP relative to the period before the CP, as revealed by negative values of the zonal component (blue colours in middle Fig. 10) in the band 45–60∘ N latitude (less moisture transported from west to east, the direction of the source–receptor path) and negative values of meridional winds (blue colours in bottom Fig. 10) in the band 20–60∘ W longitude (less moisture transported from south to north, the direction of the source–receptor path). We have carried out this analysis for each month, and for source and sink areas, and the patterns can explain all the significant results found in the Lagrangian analysis with almost absolute agreement (Fig. S1).
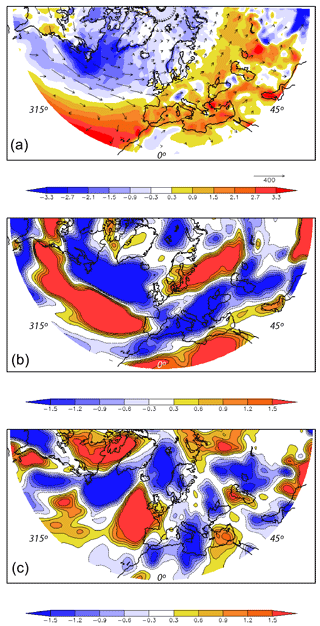
Figure 10(a) The climatological vertical integrated moisture flux (VIMF) (vector, kg m−1 s−1) and its divergence (shaded, mm yr−1) in June for the European sector, (b) the difference between the periods after vs. before the CP for the zonal component of VIMF, and (c) as for panel (b) but for the meridional component of VIMF.
An additional demonstration of the robustness of these results can be derived by analysing the changes in atmospheric circulation responsible for changes in moisture transport. Changes in MTP may be related to alterations in moisture sources caused by changes in circulation patterns, to changes in the intensity of the moisture sources because of enhanced evaporation, or to a combination of these two mechanisms. At a daily scale, changes in intensity are negligible, but changes in circulation may be significant. Thus, to analyse whether there are important differences in MTP from sources to sinks associated with different circulation patterns, and how such differences could affect MTP in the AR, we grouped individual days into four circulation types using the methodology developed by Fettweis et al. (2011) and explained in Sect. 2. We have separated the analysis into four sectors centred on the four sources of moisture to the AR to filter out annular effects, as we are interested in regional influences.
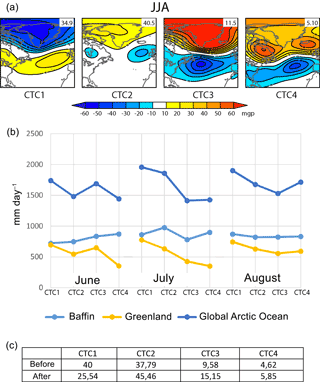
Figure 11(a) Anomalies of geopotential height at 850 hPa (Z850) for the four types of circulation centred in the Atlantic sector in summer (classes CTC1 to CTC4), and the percentages of days grouped in each class. (b) Average moisture transport for precipitation (MTP) for each class and summer months from the Atlantic source to the Arctic Ocean (AO), and the two dominant subregions, the Barents Sea and Greenland. (c) Change of frequency of these circulation types (classes) before and after the change point.
Supplement Fig. S2 shows the summer and autumn types of circulation centred on the four source areas (Atlantic, Pacific, Siberia, and North America), with each individual map representing the anomalies of geopotential height at 850 hPa (Z850) for the four different classes found (from CTC1 to CTC4, with the percentage of days grouped). The average MTPs for each class before and after the CP are summarised in Table S2, whereas changes in the frequency of each class before and after the CP are shown in Table S3 (statistical significance changes were calculated using a z test to compare two sample proportions; Sprinthall, 2011). One of these sectors and one season can serve us as an example of these results: the Atlantic during summer (Fig. 11). The anomalies of geopotential height at 850 hPa (Z850) for the four different classes found (Fig. 11 top) show patterns that resemble the known teleconnection patterns in the region (Barnston and Livezey, 1987 and http://www.cpc.ncep.noaa.gov/data/teledoc/telecontents.shtml, last access: 18 December 2017). Thus, CTC1 has a zonal dipolar structure that resembles the positive phase of the eastern Atlantic pattern (a north–south dipole of anomaly centres that are positive in the southern one and span the North Atlantic); this pattern is closely related to enhanced precipitation on Greenland. CTC2 is slightly similar to the negative phase of the eastern Atlantic and western Russia (negative height anomalies located over Europe and northern China, and positive height anomalies located over the central North Atlantic and north of the Caspian Sea), associated with enhanced precipitation on the Barents Sea. CTC3 resembles the negative phase of the North Atlantic Oscillation (at above-normal heights across the high latitudes of the North Atlantic and below-normal heights over the central North Atlantic and western Europe), related to diminished precipitation on Greenland and enhanced precipitation on the Barents Sea. CTC4 is similar to the positive phase of the Scandinavian pattern (positive height anomalies over Scandinavia and western Russia) associated with diminished precipitation on the Barents Sea. Figure 11b shows the average MTP for each class in the summer months from the Atlantic source to the AO (dark blue line) and the two dominant subregions, the Barents Sea and Greenland (light blue and orange lines, respectively). CTC1 is the circulation type responsible for the highest MTP in the three summer months, and CTC3 and CTC4 are responsible for the lowest MTP, depending on the month. The change of frequency of these classes representing circulation types before and after the CP (Fig. 11 bottom) shows a decrease in the days belonging to CTC1, with the highest MTP from 40 to 25.4 days, and an increase in days belonging to CTC3 (from 9.58 to 15.15) and CTC4 (from 4.62 to 5.85), the two classes with the lowest MTP. These results are absolutely consistent with our Lagrangian results of changes in MTP. A similar analysis to the one for this sector, moisture source region, and season can be performed for all the significant results found in the Lagrangian analysis with very good agreement.
This study shows that a drastic Arctic sea ice decline occurred in 2003 and that this decline was accompanied by a change in the moisture transport from the main Arctic moisture sources, which then results in changes in the precipitation over the Arctic (MTP). The pattern of change consists of a general decrease after vs. before 2003 in the moisture transport in summer and an increase in autumn and early winter, with different contributions depending on the moisture source and ocean subregion. This change in the pattern of moisture transport is not only statistically significant but also consistent with Eulerian flux diagnosis, changes in circulation type frequency, and any of the known mechanisms affecting snowfall or rainfall on ice in the Arctic. The consistent increment after the CP of MTP for all moisture sources in September, the month when the extent of Arctic sea ice is lowest, is particularly remarkable. These results suggest that ice melt at a multi-year scale is favoured by a decrease in moisture transport in summer and an increase in autumn and early winter.
The results of this paper also reveal another important conclusion: the assumed and partially documented enhanced poleward moisture transport from lower latitudes as a consequence of increased moisture from climate change (Zhang et al., 2012; Gimeno et al., 2015) has not been simple or constant in its links with enhanced Arctic precipitation throughout the year in the present climate. Major moisture sources for the Arctic did not provide more moisture for precipitation to the Arctic in summer after 2003, the change point (CP) for Arctic sea ice, than before, although they did provide more moisture in autumn and early winter. Because the enhanced Arctic precipitation projected by most models for the end of the century (Bintaja and Selten, 2014) is partly attributed to enhanced poleward moisture transport from latitudes lower than 70∘ N (Bintanja and Andry, 2017), where the major sources studied herein are located, our results raise questions of whether this change has occurred so simply in the current climate, and these questions merit further study.
The data used are listed in the references, tables, and Supplement.
The supplement related to this article is available online at: https://doi.org/10.5194/esd-9-611-2018-supplement.
The authors declare that they have no conflict of interest.
This article is part of the special issue “The 8th EGU Leonardo Conference: From evaporation to precipitation: the atmospheric moisture transport”. It is a result of the 8th EGU Leonardo Conference, Ourense, Spain, 25–27 October 2016.
The authors acknowledge funding by the Spanish government within the EVOCAR
(CGL2015-65141-R) project, which is also funded by FEDER (European Regional
Development Fund).
Edited by: Ricardo Trigo
Reviewed by: two anonymous referees
Årthun, M., Eldevik, T., Smedsrud, L. H., Skagseth, Ø, and Ingvaldsen, R. B.: Quantifying the influence of Atlantic heat on Barents Sea ice variability and retreat, J. Climate, 25), 4736–4743, https://doi.org/10.1175/JCLI-D-11-00466.1, 2012.
Barnston, A. G. and Livezey, R. E.: Classification, seasonality and persistence of low-frequency atmospheric circulation patterns, Mon. Weather Rev., 115, 1083–1126, https://doi.org/10.1175/1520-0493(1987)115<1083:CSAPOL>2.0.CO;2, 1987.
Belleflamme A., Fettweis, X., Lang, C., and Erpicum, M.: Current and future atmospheric circulation at 500 hPa over Greenland simulated by the CMIP3 and CMIP5 global models, Clim. Dynam., 41, 2061–2080, https://doi.org/10.1007/s00382-012-1538-2, 2012.
Bintaja, R. and Andry, O.: Towards a rain-dominated Arctic, Nat. Clim. Change, 7, 263–267, https://doi.org/10.1038/nclimate3240, 2017.
Bintaja, R. and Selten, F. M.: Future increases in Arctic precipitation linked to local evaporation and sea-ice retreat, Nature, 509, 479–482, https://doi.org/10.1038/nature13259, 2014.
Boisvert, L. N., Petty, A. A., and Stroeve, J. C.: The Impact of the Extreme Winter 2015/16 Arctic Cyclone on the Barents–Kara Seas, Mon. Weather Rev., 144, 4279–4287, 2016.
Cheng, B., Zhang, Z., Vihma, T., Johansson, M., Bian, L., Li, Z., and Wu, H.: Model experiments on snow and ice thermodynamics in the Arctic Ocean with CHINARE2003 data, J. Geophys. Res., 113, C09020, https://doi.org/10.1029/2007JC004654, 2008.
Cohen, J., Screen, J. A., Furtado, J. C., Barlow, M., Whittleston, D., Coumou, D., and Jones, J.: Recent Arctic amplification and extreme mid-latitude weather, Nat. Geosci., 7, 627–637, https://doi.org/10.1038/ngeo2234, 2014.
Dee, D. P., Uppala, S. M., Simmons, A. J., Berrisford, P., Poli, P., Kobayashi, S., and Vitart, F.: The ERA-Interim reanalysis: Configuration and performance of the data assimilation system, Q. J. Roy. Meteor. Soc., 137, 553–597, https://doi.org/10.1002/qj.828, 2011.
Ding, Q., Schweiger, A., L'Heureux, M., Battisti, D. S., Po-Chedley, S., Johnson, N. C., Blanchard-Wrigglesworth, E., Harnos, K., Zhang, Q., Eastman, R., and Steig, E. J.: Influence of high-latitude atmospheric circulation changes on summertime Arctic sea ice, Nat. Clim. Change, 7, 289–295, https://doi.org/10.1038/nclimate3241, 2017.
Fetterer, F., Knowles, K., Meier, W., and Savoie, M.: Sea Ice Index, Version 2. [1979–2016]. Boulder, Colorado USA, NSIDC: National Snow and Ice Data Center, https://doi.org/10.7265/N5736NV7 (last access: 22 November 2017), 2016.
Fettweis, X., Mabille, G., Erpicum, M., Nicolay, S., and Van den Broeke, M.: The 1958–2009 Greenland ice sheet surface melt and the mid-tropospheric atmospheric circulation, Clim. Dynam., 36, 139–159, https://doi.org/10.1007/s00382-010-0772-8, 2011.
Gimeno, L., Drumond, A., Nieto, R., Trigo, R. M., and Stohl, A.: On the origin of continental precipitation, Geophys. Res. Lett., 37, L13804, https://doi.org/10.1029/2010GL043712, 2010.
Gimeno, L., Stohl, A., Trigo, R .M., Domínguez, F., Yoshimura, K., Yu, L., Drumond, A., Durán-Quesada, A. M., and Nieto, R.: Oceanic and Terrestrial Sources of Continental Precipitation, Rev. Geophys., 50, RG4003, https://doi.org/10.1029/2012RG000389, 2012.
Gimeno, L., Nieto, R., Drumond, A., Castillo, R., and Trigo, R. M.: Influence of the intensification of the major oceanic moisture sources on continental precipitation, Geophys. Res. Lett., 40, 1443–1450, https://doi.org/10.1002/grl.50338, 2013.
Gimeno, L., Vázquez, M., Nieto, R., and Trigo, R. M.: Atmospheric moisture transport: the bridge between ocean evaporation and Arctic ice melting, Earth Syst. Dynam., 6, 583–589, https://doi.org/10.5194/esd-6-583-2015, 2015.
Gimeno, L., Dominguez, F., Nieto, R., Trigo, R. M., Drumond, A., Reason, C., and Marengo, J.: Major Mechanisms of Atmospheric Moisture Transport and Their Role in Extreme Precipitation Events, Annu. Rev. Env. Resour., 41, 117–141, https://doi.org/10.1146/annurev-environ-110615-085558, 2016.
Graversen, R. G. and Burtu, M.: Arctic amplification enhanced by latent energy transport of atmospheric planetary waves, Q. J. Roy. Meteor. Soc., 142, 2046–2054, https://doi.org/10.1002/qj.2802, 2016.
Graversen, R. G., Mauritsen, T., Drijfhout, S., Tjernström, M., and Mårtensson, S.: Warm winds from the Pacific caused extensive Arctic sea-ice melt in summer 2007, Clim. Dynam., 36, 2103–2112, https://doi.org/10.1007/s00382-010-0809-z, 2011.
Huth, R., Beck, C., Philipp, A., Demuzere, M., Ustrnul, Z., Cahynová, M., Kyselý, J., and Tveito, O. E.: Classifications of Atmospheric Circulation Patterns – Recent Advances and Applications, Annals of the New York Academy of Sciences: Trends and Directions in Climate Research, 1146, 105–152, 2008.
IPCC: Climate Change. The physical science basis, in: Contribution of working group 1 to the fifth assessment report of the intergovernmental panel on climate change, edited by: Stocker, T. F., Qin, D., Planttner, G. K., Tignor, M., Allen, S. K., Boschung, J., Nauels, A., Xia, Y., Bex, V., and Midgley, P. M., Cambridge University Press, Cambridge, UK and New York, NY, USA, 2013.
Jakobson, E., Vihma, T., Palo, T., Jakobson, L., Keernik, H., and Jaagus, J.: Validation of atmospheric reanalyses over the central Arctic Ocean, Geophys. Res. Lett., 39, L10802, https://doi.org/10.1029/2012GL051591, 2012.
Kapsch, M.-L., Graversen, R. G., and Tjernstrom, M.: Springtime atmospheric energy transport and the control of Arctic summer sea-ice extent, Nat. Clim. Change, 3, 744–748, https://doi.org/10.1038/nclimate1884, 2013.
Killick, R. and Eckley, I.: changepoint: An R Package for changepoint analysis. Journal of Statistical Software 58, 1–15, https://doi.org/10.18637/jss.v058.i03, 2014.
Koenigk, T., Brodeau, L., Graversen, R. G., Karlsson, J., Svensson, G., Tjernström, M., Willén, U., and Wyser, K.: Arctic climate change in the 21st century in an ensemble of AR5 scenario projections with EC-Earth, Clim. Dynam., 40, 2719–2743, https://doi.org/10.1007/s00382-012-1505-y, 2013.
Lee, H. J., Kwon, M. O., Yeh, S.-W., Kwon, Y.-O., Park, W., Park, J.-H., Kim, Y. H., and Alexander, M. A.: Impact of Poleward Moisture Transport from the North Pacific on the Acceleration of Sea Ice Loss in the Arctic since 2002, J. Climate, 30, 6757–6769, https://doi.org/10.1175/JCLI-D-16-0461.1, 2017.
Leppäranta, M.: A review of analytical models of sea-ice growth, Atmos. Ocean, 31, 123–138, https://doi.org/10.1080/07055900.1993.9649465, 1993.
Lorenz, C. and Kunstmann, H.: The hydrological cycle in three state-of-the-art reanalyses: Intercomparison and performance analysis, J. Hydrometeorol., 13, 1397–1420, https://doi.org/10.1175/JHM-D-11-088.1, 2012.
Mortin, J., Svensson, G., Graversen, R. G., Kapsch, M.-L., Stroeve, J. C., and Boisvert, L. N.: Melt onset over Arctic sea ice controlled by atmospheric moisture transport, Geophys. Res. Lett., 43, 6636–6642, https://doi.org/10.1002/2016GL069330, 2016.
Numaguti, A.: Origin and recycling processes of precipitating water over the Eurasian continent: Experiments using an atmospheric general circulation model, J. Geophys. Res., 104, 1957–1972, https://doi.org/10.1029/1998JD200026, 1999.
Ogi, M. and Wallace, J. M.: Summer minimum Arctic sea ice extent and the associated summer atmospheric circulation, Geophys. Res. Lett., 34, L12705, https://doi.org/10.1029/2007GL029897, 2007.
Park, H.-S., Lee, S., Son, S.-W., Feldstein, S. B., and Kosaka, Y.: The impact of poleward moisture and sensible heat flux on Arctic winter sea ice variability, J. Climate, 28, 5030–5040, https://doi.org/10.1175/JCLI-D-15-0074.1, 2015a.
Park, H.-S., Lee, S., Kosaka, Y., Son, S.-W., and Kim, S.-W.: The impact of Arctic winter infrared radiation on early summer sea ice, J. Climate, 28, 6281–6296, https://doi.org/10.1175/JCLI-D-14-00773.1, 2015b.
Philipp, A., Bartholy, J., Beck, C., Erpicum, M., Esteban, P., Fettweis, X., and Tymvios, S.: COST733CAT – a database of weather and circulation type classifications, Phys. Chem. Earth, 35, 360–373, https://doi.org/10.1016/j.pce.2009.12.010, 2010.
Post, E., Bhatt, U. S., Bitz, C. M., Brodie, J. F., Fulton, T. L., Hebblewhite, M., and Walker, D. A.: Ecological consequences of sea-ice decline, Science, 341, 519–524, https://doi.org/10.1126/science.1235225, 2013.
Ramos, A. M., Nieto, R., Tomé, R., Gimeno, L., Trigo, R. M., Liberato, M. L. R., and Lavers, D. A.: Atmospheric rivers moisture sources from a Lagrangian perspective, Earth Syst. Dynam., 7, 371–384, https://doi.org/10.5194/esd-7-371-2016, 2016.
Rigor, I. G., Wallace, J. M., and Colony, R. L.: Response of sea ice to the Arctic Oscillation, J. Climate, 15, 2648–2663, https://doi.org/10.1175/1520-0442(2002)015<2648:ROSITT>2.0.CO;2, 2002.
Rinke, A., Maturilli, M., Graham, R. M., Matthes, H., Handorf, D., Cohen, L., and Moore, J. C.: Extreme cyclone events in the Arctic: Wintertime variability and trend, Environ. Res. Lett., 12, 094006, https://doi.org/10.1088/1748-9326/aa7def, 2017.
Roberts, A., Cassano, J., Döscher, R., Hinzman, L., Holland, M., Mitsudera, H., Sumi, A., Walsh, J. E., Alessa, L., Alexeev, V., Arendt, A., Altaweel, M., Bhatt, U., Cherry, J., Deal, C., Elliot, S., Follows, M., Hock, R., Kliskey, A., Lantuit, H., Lawrence, D., Maslowski, W., McGuire, A. D., Overduin, P. P., Overeem, I., Proshutinsky, A., Romanovsky, V., Sushama, L., and Truffer, M.: A science plan for regional arctic system modeling a report by the arctic research community for the National Science Foundation, Office of Polar Programs , International Arctic Research Center (IARC) Publication 10-0001, University of Alaska, Fairbanks, AK, USA, 55 p., 2010.
Screen, J. A. and Simmonds, I.: The central role of diminishing sea ice in recent Arctic temperature amplification, Nature, 464, 1334–1337, https://doi.org/10.1038/nature09051, 2010.
Sprinthall, R. C.: Basic Statistical Analysis, 9th ed., Pearson Education, London, UK, 2011.
Stohl, A. and James, P.: A Lagrangian analysis of the atmospheric branch of the global water cycle. Part I: Method description, validation, and demonstration for the August 2002 flooding in central Europe, J. Hydrometeorol., 5, 656–678, https://doi.org/10.1175/1525-7541(2004)005<0656:ALAOTA>2.0.CO;2, 2004.
Stohl, A. and James, P.: A Lagrangian analysis of the atmospheric branch of the global water cycle. Part II: Moisture transports between the Earth's ocean basins and river catchments, J. Hydrometeorol., 6, 961–984, https://doi.org/10.1175/JHM470.1, 2005.
Tang, Q., Zhang, X., and Francis, J. A.: Extreme summer weather in northern mid-latitudes linked to a vanishing cryosphere, Nat. Clim. Change, 4, 45–50, https://doi.org/10.1038/nclimate2065, 2014.
Tjernström, M., Shupe, M. D., Brooks, I. M., Persson, P. O. G., Prytherch, J., Salisbury, D. J., Sedlar, J., Achtert, P., Brooks, B. J., Johnston, P. E., Sotiropoulou, G., and Wolfe, D.: Warm-air advection, air mass transformation and fog causes rapid ice melt, Geophys. Res. Lett., 42, 5594–5602, https://doi.org/10.1002/2015GL064373, 2015.
Vázquez, M., Nieto, R., Drumond, A., and Gimeno, L.: Moisture transport into the Arctic: Source-receptor relationships and the roles of atmospheric circulation and evaporation, J. Geophys. Res.-Atmos., 121, 13493–13509, https://doi.org/10.1002/2016JD025400, 2016.
Vázquez, M., Nieto, R. Drumond, A., and Gimeno, L.: Extreme Sea Ice Loss over the Arctic: An Analysis Based on Anomalous Moisture Transport, Atmosphere, 8, 1–18, https://doi.org/10.3390/atmos8020032, 2017.
Vihma, T., Screen, J., Tjernström, M., Newton, B., Zhang, X., Popova, V., Deser, C., Holland, M., and Prowse, T.: The atmospheric role in the Arctic water cycle: A review on processes, past and future changes, and their impacts, J. Geophys. Res.-Biogeosciences, 121 586–620, https://doi.org/10.1002/2015JG003132, 2016.
Wegmann, M., Orsolini, Y., Vázquez, M., Gimeno, L., Nieto, R., Bulygina, O., Jaiser, R., Handorf, D., Rinke, A., Dethloff, K., Sterin, A., and Brönnimann, S.: Arctic moisture source for Eurasian snow cover variations in autumn, Environ. Res. Lett., 10, 054015, https://doi.org/10.1088/1748-9326/10/5/054015, 2015.
Woods, C. and Caballero, R.: The role of moist intrusions in winter Arctic warming and sea ice decline, J. Climate, 29, 4473–4485, https://doi.org/10.1175/JCLI-D-15-0773.1, 2016.
Woods, C., Caballero, R., and Svensson, G.: Large-scale circulation associated with moisture intrusions into the Arctic during winter, Geophys. Res. Lett., 40, 4717–4721, https://doi.org/10.1002/grl.50912, 2013.
Zhang, Y., Maslowski, W., and Semtner, A. J.: Impact of mesoscale ocean currents on sea ice in high-resolution Arctic ice and ocean simulations, J. Geophys. Res., 104, 18409–18429, https://doi.org/10.1029/1999JC900158, 1999.
Zhang, X., He, J., Zhang, J., Polyakov, I., Gerdes, R., Inoue, J., and Wu, P.: Enhanced poleward moisture transport and amplified northern high-latitude wetting trend, Nat. Clim. Change, 3, 47–51, https://doi.org/10.1038/nclimate1631, 2012.