the Creative Commons Attribution 4.0 License.
the Creative Commons Attribution 4.0 License.
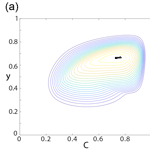
Linking resilience and robustness and uncovering their trade-offs in coupled infrastructure systems
Mehran Homayounfar
John M. Anderies
Chitsomanus P. Muneepeerakul
Robustness and resilience are concepts in systems thinking that have grown in importance and popularity. For many complex social-ecological systems, however, robustness and resilience are difficult to quantify and the connections and trade-offs between them difficult to study. Most studies have either focused on qualitative approaches to discuss their connections or considered only one of them under particular classes of disturbances. In this study, we present an analytical framework to address the linkage between robustness and resilience more systematically. Our analysis is based on a stylized dynamical model that operationalizes a widely used conceptual framework for social-ecological systems. The model enables us to rigorously delineate the boundaries of conditions under which the coupled system can be sustained in a long run, define robustness and resilience related to these boundaries, and consequently investigate their connections. The results reveal the trade-offs between robustness and resilience. They also show how the nature of such trade-offs varies with the choice of certain policies (e.g., taxation and investment in public infrastructure), internal stresses, and uncertainty in social-ecological settings.
- Article
(1442 KB) - Full-text XML
- BibTeX
- EndNote
The concepts of “resilience” and “robustness” have grown considerably in popularity as desirable properties for a wide range of systems. Terms like “resilient communities” and “robust cities” have been used more frequently in public discourse (e.g., Chang and Shinozuka, 2004; Longstaff et al., 2010; Chang et al., 2014). The UK's Water Act 2014 even included “primary duty to secure resilience” as one of the general duties of its Water Services Regulation Authority (Water Act, 2014). Growing with that popularity is some confusion and potential misuse of the terms “robustness” and “resilience” due to imprecision, vagueness, and multiplicity of their definitions. Such a lack of consistency and rigor hinders advances in our understanding of the interplay between these two important system properties.
Relatively speaking, robustness has been defined more consistently and rigorously – as it can be linked to a more familiar concept of sensitivity. For example, according to Carlson and Doyle (2002), robustness in engineering systems refers to the maintenance of system performance either when subjected to external disturbances or internal uncertain parameters. In other words, in robust systems, performance is less sensitive to disturbances or uncertainty.
Robustness may very well be a desirable property of a system but it seems to come with a price. Recent research shows that tuning a system to be robust against certain disturbance regimes almost always reduces system performance and likely increases its vulnerability to other disturbance regimes (Ostrom et al., 2007; Anderies et al., 2007; Bode, 1945; Csete and Doyle, 2002; Wolpert and Macready, 1997). Now, if resilience is also a desirable property of the same system, does it also come at the expense of performance and robustness? Put it another way, is there a trade-off among performance, robustness, and resilience? Such a trade-off, if it exists, is a crucial consideration for governing and/or managing social-ecological systems (SESs).
But resilience, as alluded to above, is trickier to define. According to Holling (1973), resilience refers to the amount of change or disruption required to shift the maintenance of a system along different sets of mutually reinforcing processes and structures. In other words, resilience can be thought of as how far the system is from certain thresholds or boundaries beyond which the system will undergo a regime shift or a quantitative change in system structure or identity. Holling (1996) categorized resilience into two types: engineering resilience, which refers to the ability of a system to return to a steady state following a perturbation and ecological resilience, which refers to the capacity of system to remain in a particular stability domain in the face of perturbations. The latter category is used by many researchers to discuss resilience of SESs, or more generally, coupled infrastructure systems (CISs; Carpenter et al., 2001; Anderies et al., 2006; Folke, 2006; Biggs et al., 2012; Barrett and Constas, 2014; Redman, 2014; Walker et al., 2002, 2004; Gunderson and Holling, 1995; Berkes and Folke, 1998; Carpenter et al., 1999a, b; Scheffer et al., 2000; Berkes et al., 2003; Carpenter and Brock, 2004; Janssen et al., 2004; Folke et al., 2002, 2010, 2016; Cote and Nightingale, 2012; Mitra et al., 2015; Cumming and Peterson, 2017). The CISs term has been introduced to generalize the notions of coupled natural human systems (CNHSs) and SESs; in this context, infrastructure is broadly defined to include human-made, social, and natural infrastructure (e.g., see Anderies et al., 2016). The problem is that these CISs are complex and thus identifying thresholds and potential regime shifts associated with those thresholds is often difficult, if not impossible. In many cases, major aspects of resilience in CISs may not be directly observable and must be actualized indirectly via surrogate attributes (Carpenter et al., 2005; Kerner and Thomas, 2014). Recent significant advances have been made toward identifying early-warning signals that indicate whether a critical threshold is being approached for a wide class of systems (Scheffer et al., 2009, 2012). Still, there are gaps in our understanding of how indicators of resilience and robustness will behave in more complex situations. This lack of a rigorous metric for resilience makes the investigation into their connections, interplay, and trade-offs with robustness and performance impossible.
But these knowledge gaps need to be filled if one wishes to make advances in understanding the interplay between social dynamics and planetary boundaries. Given the magnitude of impacts that human activities have on pushing Earth systems toward their planetary boundaries, we need clearer understanding of how social and biophysical factors come together to define the nature of these boundaries. This paper is a step in that direction. In particular, we will build on recent work that mathematically operationalizes the robustness of a SES framework (Anderies et al., 2004) into a formal stylized dynamical model (Muneepeerakul and Anderies, 2017). We will exploit the relative simplicity of the model to rigorously define robustness and resilience of the coupled system. The modeled system will be subject to uncertainty in social and ecological factors, which will affect the well-defined robustness and resilience, thereby enabling us to investigate the interplay and trade-offs between these important properties, as well as investigate how the nature of the interplay and trade-offs are affected by policies implemented by social agents.
Here we analyze a mathematical model developed by Muneepeerakul and Anderies (2017) by subjecting the coupled system to uncertainty in ecological and social factors. The model captures the essential features of a system in which a group of agents shares infrastructure to produce valued flows. Such a system is the archetype of most, if not all, of human sociality: groups produce infrastructure that they cannot produce individually (security, defense, irrigation canals, roads, markets, financial systems, coordination mechanisms, etc.) that significantly increases productivity. The challenge is maintaining this shared infrastructure (e.g., decaying infrastructure is a major problem in the US at the time of writing; ASCE RCIA Advisory Council, 2013). The model allows for mathematical definitions of the boundaries of policy domain that result in a sustainable system in which both human-made and natural infrastructure can be maintained over the long run. Based on these boundaries and uncertainty in the exogenous factors, we define metrics of resilience and robustness associated with each policy choice and investigate the trade-off between them. The basic model presented by Muneepeerakul and Anderies (2017) is described in the Appendix.
Here a policy is defined as a combination of taxation level C and the proportion of tax revenue invested in infrastructure maintenance y that the public infrastructure providers (PIPs) decide to implement in the system. The infrastructure (e.g., canals) enable resource users (RUs) to produce valued goods from a natural resource. The two uncertain factors are the replenishment rate of the natural resource g and the wage w that RUs would earn from working outside the system – a combination of g and w defines a “social-ecological setting” or simply “setting”. There are two boundaries that, once crossed, will cause the system to collapse. The first boundary is called PIP participation constraint (PPC): when the PIPs must invest too much in maintaining the public infrastructure (exceeding the opportunity cost of wP) and/or cannot retain enough revenue for themselves, they will abandon the system for another. The second boundary is the stability condition of the nontrivial equilibrium point (i.e., the “society” in which both PIPs, for example the state, and RUs, for example citizens, participate in and public infrastructure is sufficiently maintained in a long run). Together, these two boundaries delineate a set of policies (C–y combinations) that correspond to sustainable outcomes. The resilience metric, to be developed below, can be thought of as a metric of how far the system is from these boundaries. As the two exogenous factors defining settings, namely g and w, change, the two boundaries and thus the resilience metric also change with them. How sensitive the resilience metric is to these settings is used to define robustness. Here it is worth noting that, while it is possible to examine recovery-based resilience (or the so-called “engineering resilience”), this paper focuses on regime shift-based resilience (traditionally called “ecological resilience”) and its robustness. Quantification of and trade-offs between resilience and robustness is a novel concept that requires expositional clarity. Presenting several metrics of resilience, let alone studying their trade-offs with potentially different metrics of robustness, may confuse the matter and dilute the key messages we attempt to convey. As such, in what follows, we will focus on developing a metric for regime shift-based resilience. With the scope of analysis clarified and suitably bounded, we will now define resilience and robustness more formally.
2.1 Resilience metric
Direct measurement of the above-mentioned resilience, as a specified form of resilience (Walker et al., 2004), in SESs is difficult because boundaries and thresholds that separate domains of dynamics for SESs are difficult to identify (Carpenter et al., 2005; Scheffer et al., 2009, 2012). In this stylized model, however, such boundaries can be clearly identified by the stability condition (SC) and the PPC. Here, we are interested in the resilience of a system's ability to provide sufficient livelihoods for the PIPs and RUs. The basin of attraction for system resilience is defined by those system states (i.e., infrastructure state) in which this is possible, and these system states are directly mapped to the SC and PPC. We will thus define resilience metrics based on the SC and PPC boundaries. Here our goal is to develop resilience metrics that can be meaningfully compared to one another. As such, we identify some desired properties that guide the definitions of these resilience metrics. First, they should be zero at their respective boundaries. Second, positive values indicate greater resilience of the system in a desirable state. These first two properties align with how resilience has been measured, i.e., the distance from the boundary of a basin of attraction (e.g., Anderies et al., 2002; Carpenter et al., 1999a). Third, to facilitate the consideration of relative risks associated with different types of regime shifts that the system may be facing, the metrics should be comparable in magnitude. These properties guide us toward the following definitions of the resilience metrics:
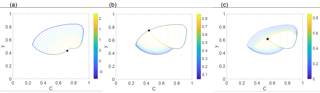
Figure 1Resilience metrics for a specific setting (a g–w combination) inside the sustainable region in the policy space (i.e., C–y plane): (a) RPPC contours; (b) Rstability contours; and (c) Rsystem contours. The black star in panels (a), (b), and (c) indicates the policy with the highest RPPC, Rstability, and Rsystem, respectively.
We define the resilience of the system against abandonment by PIPs as follows:
where πP is the net revenue that PIPs collect and wP is the opportunity cost that they will earn if they choose to work with another system. Positive values of RPPC indicate that the system is resilient against being abandoned by PIPs, while negative values indicate that the system will eventually collapse due to the PIPs' abandonment. It is important to note that πP results from the coupled dynamics of the CIS; this means that RPPC has already integrated the dynamics of infrastructure, resource, and RUs (Eqs. A1, A4, and A5), making it a metric of the system, not of an individual component.
Numerical analysis of the model indicates that the equilibrium becomes unstable when the following Routh–Hurwitz condition (e.g., May, 2001; Kot, 2001) is violated:
where D, T, and J are determinant, trace, and entries, respectively, of the Jacobian matrix of the dynamical system (Eqs. A1, A4, and A5) evaluated at the nontrivial equilibrium point (Eq. A6) – when such an equilibrium point exists. Here, it is worth noting that we focus on the equilibrium point related to the nontrivial sustainable long-term outcome. Analyzing other bifurcations related to other equilibria may be mathematically interesting, but it could make the study less accessible and dilute its key message about the resilience–robustness trade-off of different policies aiming at keeping the system in the basin of attraction of the non-trivial sustainable long-term outcome.
Following the guideline provided by the three desirable properties above, we rearrange terms in Eq. (2) and define the resilience of the system against instability (increased probability of collapse of infrastructure) as follows:
This formulation is parallel to that of the first resilience metric (Eq. 1); it possesses the following three properties: Rstability of zero indicates the boundary between stability and instability, positive Rstability means the system at the equilibrium point is stable, and the magnitudes of Rstability are comparable to those of RPPC (see Fig. 1). Note that Rstability, too, is determined from the coupled dynamics of the CIS; this means that it has integrated the dynamics of infrastructure, resource, and RUs (Eqs. A1, A4, and A5).
This allows us to meaningfully define the overall system resilience as the minimum between the two resilience metrics, namely
Equation (4) implies that Rsystem is positive only when the nontrivial equilibrium point (Eq. A6) exists and is stable; otherwise, the system is considered not resilient and denoted by Rsystem=0; Rsystem thus represents the tension between the PIPs being too greedy (high C and low y) whereby they get close to the stability boundary and “not greedy enough” (low C and high y) whereby they get close to the PPC, given a particular choice for wP. Note that the values of w and wP represent the socioeconomic embedding of the CIS. Therefore, the biophysical structure of the CIS along with the socioeconomic context in which it is embedded co-determine the maximum resilience that can be achieved. Given that the nontrivial equilibrium point exists and is stable, the following applies. If the system is at a greater risk of being abandoned by the PIPs (and eventually collapsing), Rsystem=RPPC; if the system is at a greater risk of becoming unstable (and eventually collapsing), Rsystem=Rstability. Figure 1 illustrates the relationships between RPPC, Rstability, and Rsystem.
2.2 Linking robustness and resilience
As discussed earlier, robustness can be thought of as the opposite of sensitivity. A commonly used measure of sensitivity is variance. Thus, variance of a given function under specific disturbance or uncertainty regimes may be used to indicate robustness of that function against those disturbances or uncertainty regimes (robustness of what to what). In this case, the system function of interest is the system resilience Rsystem. By choosing Rsystem as our function, we can usefully link these concepts. If we use a ball and cup metaphor for resilience, the robustness of resilience refers to the degree at which the geometry of the cup changes as a result of external disturbances and/or parameter changes. However, as we will argue below, relating high variance in Rsystem to low robustness may be misleading and should not be used in evaluating a given policy. By definition, the variance treats “good deviations” and “bad deviations” from the mean equally. For functions with preferred values, such as resilience or profit, values greater than the mean and those lower should not be treated in the same way. Specifically, contribution to a high variance from a heavy tail in the good direction should not be translated to less robustness. This problem also arises in assessing financial risk: what makes an asset risky is the values on the “bad tail” of the distribution (i.e., low or negative profits). This has motivated more and more analyses to switch to considering other measures of risk, such as the conditional value at risk, in evaluating their portfolios of investment (Rockafellar and Uryasev, 2000; Krokhmal et al., 2002; Sarykalin et al., 2014; AitSahlia et al., 2011; Zymler et al., 2013). Intuitively, this means that the shape of the “cup” can be asymmetric and we need to take this into account.
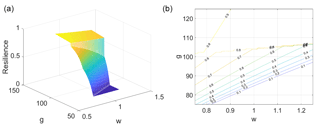
Figure 2Variation of Rsystem of a CIS with a fixed policy (C,y) over 10 000 settings associated with uncertainty characterized by : (a) Rsystem surface and (b) Rsystem contours. The values of Rsystem are used to calculate the mean, , and the below-mean mean, μR<μ. In this particular case, the resilience does not change much when g is greater than about 100, but becomes more sensitive to both g and w when g is lower than 100.
Following this logic, we propose to use a “below-mean mean” as a new robustness metric: the mean of all resilience values lower than the mean. This new definition of the robustness metric has several desirable features. First, it can now be appropriately thought of as a robustness metric in the sense that the higher the value, the more robust the system (unlike the variance for which low variance means high robustness). Second, by using the mean as the threshold value for bad deviations, we remove some arbitrariness associated with prescribing a certain quantile (e.g., 5th or 10th quantile) in calculating the conditional value at risk. Third, it still carries some information about the sensitivity of the resilience metric to outside factors – the information that variance conveys; that is, the higher the below-mean mean (i.e., the bad deviations from the mean are small and the below-mean mean is close to the mean), the less sensitive – and thus more robust – the resilience metric.
In this study, we subject the modeled system to uncertainty in one natural factor and one social factor, namely the natural replenishment rate of the resource g, and the payoff that a RU earns from working outside the system w. Thus, we are computing how the resilience of the system to shocks and/or variation in state variables changes as the parameters g and w change (i.e., we are uncertain about the underlying social-ecological setting of the system). In particular, we assume that g is uniformly distributed over the range [75, 125] and w is uniformly distributed over the range [0.75, 1.25]. A social-ecological setting, or setting, is defined as a combination of g and w. For a given policy (a C–y combination), we calculate Rsystem for 10 000 settings (i.e., 10 000 g–w combinations, see Fig. 2). Then, from these 10 000 values of the resilience metric Rsystem, we calculate the mean, , and use it as the resilience metric of the coupled system with a given policy, and the below-mean mean, , as the metric for robustness of resilience. This metric measures the robustness of the capacity of the system to cope with variation in state variables IHM,R, and U to fundamental uncertainty about the underlying setting of the system.
The surfaces and contours of the system resilience metric, , and the associated different policies (C–y) over the policy space are shown in Fig. 3a and b, respectively. The policies with sustainable outcomes are located in the middle of the policy space, with peaking in the center and declining as policies become more extreme in either direction. Our analysis also shows that is more or less proportional to the fraction of settings (g–w combinations) under which the system with that particular policy (a C–y combination) results in a sustainable outcome (Rsystem>0). A similar concept has been used in the robust decision making literature (e.g., Groves and Lempert, 2007; Bryant and Lempert, 2010).
The surfaces and contours of the robustness of μR<μ associated with different policies over the policy space are shown in Fig. 3c and d, respectively. The μR<μ “landscape” is more irregular, having two local maxima with one being more dominant than the other. The region with high robustness appears to be in the same general areas as the region with high resilience. These features reflect the nonlinear interplay between the model parameters and model structure and may affect the nature of the trade-off between robustness and resilience reported in Figs. 4 and 5.
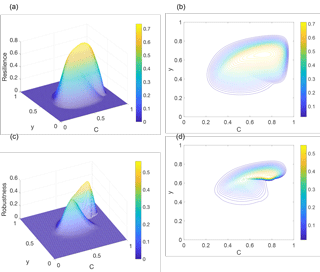
Figure 3The mean, , and the below-mean mean of Rsystem, μR<μ, over the entire decision space. (a) Surface of the resilience metric, ; (b) Contours of ; (c) Surface of the robustness, the below-mean mean (μR<μ); (d) Contours of μR<μ.
We explore the interplay between and μR<μ in Fig. 4. Figure 4 shows that there are no perfect policies in the sense that no policies yield both maximum resilience and maximum robustness. Recall that the robustness indicates how sensitive Rsystem itself is to uncertainty in the underlying setting of the system (e.g., g and w). The best policies are those along the Pareto frontier in the resilience–robustness space: among this set of Pareto-optimal policies, an increase in resilience is necessarily accompanied by a decrease in robustness, clearly illustrating the trade-off between robustness and resilience. Fig. 5 illustrates where the Pareto-optimal policies are located in the policy space.
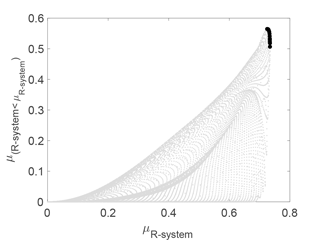
Figure 4Resilience–robustness trade-off. Each point represents, and μR<μ of the coupled system with a given policy. The black dots represent a set of Pareto-optimal policies.
In this paper, we exploit the simplicity of a stylized model to quantitatively link resilience and robustness by computing how the CIS resilience to shocks in state variables changes with parameters. In this way, we compute the robustness of CIS resilience to uncertainty in the underlying CIS setting. The resilience metric developed here is a measure of how far the CIS is from the boundaries beyond which it will collapse. The model affords us with expressions of these boundaries, which clearly show how social and biophysical factors interplay to define these boundaries. With a concrete definition of resilience, resilience itself can be considered as the “of what” in the “robustness of what to what” notion. In particular, we use the below-mean mean of the quantitatively defined resilience metric as the metric of robustness. Consequently, this enables us to rigorously investigate the interplay between the two important, but not always well-defined, system properties. A key finding is the fundamental trade-off between resilience and robustness: there are no perfect policies in governing a CIS, only Pareto-optimal ones. Specifically, policies designed to maximize the resilience of a CIS to shocks on timescales at which the state variables play out may be very sensitive to being wrong about our understanding of the underlying dynamics of the CIS in question.
Importantly, we hope this work will stimulate further advances in rigorous studies of CISs that address such subtle, policy-relevant questions, a few of which we briefly discussed here. More dimensions can be considered in defining Pareto-optimality. Figure 5 may give an impression that the set of Pareto-optimal policies is confined to a small region in the policy space, which would imply that PIPs do not have that many choices – even in a simple CIS like the one studied. But that would be a wrong impression. In addition to resilience and robustness (as defined here), a policy maker or a social planner may be interested in other types of robustness with different “of what” and “to what” components. They may also be concerned about other system properties, e.g., productivity, user participation, etc. As more dimensions are considered, the set of Pareto-optimal policies grow. In the same spirit as that of the work done here, these other dimensions should be defined rigorously.
This work also lends itself to more rigorous studies of “adaptive governance.” In the present study, the governance structure, represented by a policy (a combination of C and y), is fixed. A natural next step is to explore if a policy is allowed to change, how one may improve the resilience and robustness of a CIS, and/or alter the nature of their inherent trade-offs. For example, if C and y are to be functions of other factors, e.g., resource availability and outside incentives, what functional forms should they take to improve the system's resilience and robustness? Indeed, in the absence of transparent metrics, attempts to explore such adaptive policies are severely limited.
Additionally, agents in a CIS may have the capacity to change their behavior in response to changes in policy, environmental conditions, technological changes, and so on. In this study, strategic behavior and the decision-making process are assumed unchanged in the analysis. Adaptation in strategic behavior of agents will subsequently alter the nature of resilience, its robustness, and their trade-offs. Capturing such effects of adaptation requires structural changes to the model, e.g., in terms of specification of payoffs or even the formulation of the dynamical equations. With such adaptive social agents, how should one devise adaptive governance to enhance resilience and robustness of a CIS? Addressing such a question is a theoretically intriguing future research direction with great practical implications.
In keeping with the theme of “social dynamics and planetary boundaries in Earth system modelling,” our results shed light on how social and biophysical factors may interplay to define boundaries of a sustainable coupled infrastructure system. While the modeled system here is admittedly simple, our methodology and results constitute a step toward quantitatively and meaningfully combining social and biophysical factors into indicators of boundaries of more complex systems. Just as in this work, once those boundaries are clearly defined, calculation and discussion of resilience and robustness can become concrete.
No data sets were used in this article.
Basic model. Here we briefly describe the basic model presented by Muneepeerakul and Anderies (2017). The model shows dynamic behavior of three principal variables, namely the state of the public infrastructure, IHM, resource level, R, and the fraction of time a user makes use of infrastructure, U, through Eqs. (A1, A4, and A5). The schematic diagram of this system of equations is shown in Fig. A1.
In this context, IHM depends on PIPs in term of maintenance cost and has a positive relationship with the capacity of users to create resource flows. Eq. (A1) illustrates the dynamics of IHM as follows:
where δ is the infrastructure's depreciation rate and H(IHM) states functional relationship of public infrastructure and productivity of each RU. According to Muneepeerakul and Anderies (2017), many shared infrastructures can be modeled by threshold functions. Given that H(IHM) shows threshold behavior, they used a piecewise linear function to capture such behavior through Eq. (A2).
where h represents the maximum amount of harvest by each user under no restriction and I0 and Im are the lower bound and upper bound thresholds of IHM, respectively. Also, M(…) is the maintenance function (Eq. A3) and depends on the social structure of the system.
In Eq. (A3), given the number of users N, RUNH(IHM) is the total harvest from the natural infrastructure. The RUs sell total harvest at price p to generate revenue. Subsequently, they assign a proportion C of revenue to PIP's for their contribution. Meanwhile, the PIP's spend proportion y of C on maintaining public infrastructure through the maintenance function M(…). Also, μ2 is the maintenance effectiveness of PIP's investment.
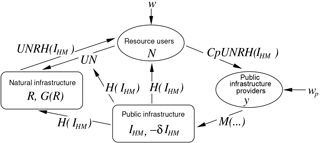
Figure A1Schematic diagram of the dynamical system model. Taken from Muneepeerakul and Anderies (2017).
The second variable is the resource level, R. They assumed the dynamics of resource to be
Natural infrastructure is assumed to invoke the conservation law comprising of regenerating capacity ( and total unit of harvest, RUNH(IHM). The definition presented for G is the simplest model for natural infrastructure where g and d are the natural replenishment and the loss rates, respectively.
The strategic behavior of the resource users (RUs) is captured by employing a replicator equation. Indeed, replicator dynamics provide modelers with simple, realistic social mechanism where agents follow and replicate better-off strategies. The two possible strategies considered for RUs are staying inside the system with the associated payoff of or leaving the system with the payoff of πw=w. According to the replicator equation:
The replicator equation represents the fraction of time that RUs assign to working inside system given C and y. Like RUs, there is also two alternatives for PIPs, working inside the system or working for another CIS which leads to system failure. Meanwhile, C and y characterize the strategy or policy of PIPs. The PIPs will participate in this coupled system only when . In other words, the PIPs maintain the system when they are better-off than working outside. This condition is termed the PIP Participation Constraint (PPC).
Based on the system of three differential equations (Eqs. A1, A4, and A5), the sustainable equilibria, i.e., long-term system outcomes that satisfy the stability condition and PPC, can be expressed as follows:
where (indicates dimensionless) and , a dimensionless group representing the relative lucrativeness of the system, namely the ratio of potential income – with the entire resource flow turned into income – relative to outside wage. The results reported in this study are based on the following parameter values: h=0.0005; δ=0.1; I0=0.3; Im=3; g=100; d=0.02; N=1000; r=0.15; p=10; w=1; wp=100; and μ2=0.001.
MH, RM, JMA, and CPM designed the study. MH carried out the analysis. MH and RM prepared the manuscript with contributions from all co-authors.
The authors declare that they have no conflict of interest.
This article is part of the special issue “Social dynamics and planetary boundaries in Earth system modelling”. It is not associated with a conference.
John M. Anderies and Rachata Muneepeerakul acknowledge the support from the
grant NSF GEO-1115054. Rachata Muneepeerakul and Mehran Homayounfar
acknowledge support from the Army Research Office/Army Research Laboratory.
The research reported in this grant was supported in whole or in part by the
Army Research Office/Army Research Laboratory under award no. W911NF1810267
(Multi-University Research Initiative). The views and conclusions contained
in this document are those of the authors and should not be interpreted as
representing the official policies either expressed or implied of the Army
Research Office or the US Government. The authors also thank the editor and
three anonymous referees for their constructive and useful comments.
Edited by: Jonathan Donges
Reviewed by: three anonymous referees
AitSahlia, F., Wang, C. J., Cabrera, V. E., Uryasev, S., and Fraisse, C. W.: Optimal crop planting schedules and financial hedging strategies under ENSO-based climate forecasts, Ann. Oper. Res., 190, 201–220, 2011.
Anderies, J. M., Janssen, M. A., and Walker, B. H.: Grazing Management, Resilience, and the Dynamics of a Fire-Driven Rangeland System, Ecosystems, 5, 23–44, https://doi.org/10.1007/s10021-001-0053-9, 2002.
Anderies, J. M., Janssen, M., and Ostrom, E.: A Framework to Analyze the Robustness of Social-Ecological Systems from an Institutional Perspective, Ecol. Soc., 9, 18, http://www.ecologyandsociety.org/vol9/iss1/art18/inline.html, 2004.
Anderies, J. M., Ryan, P., and Walker, B. H.: Loss of Resilience, Crisis, and Institutional Change: Lessons from an Intensive Agricultural System in Southeastern Australia, Ecosystems, 9, 865–878, https://doi.org/10.1007/s10021-006-0017-1, 2006.
Anderies, J. M., Rodriguez, A. A., Janssen, M. A., and Cifdaloz, O.: Panaceas, Uncertainty, and the Robust Control Framework in Sustainability Science, P. Natl. Acad. Sci. USA, 104, 15194–15199, https://doi.org/10.1073/pnas.0702655104, 2007.
Anderies, J. M., Janssen, M., and Schlager, E.: Institutions and the performance of coupled infrastructure systems, Int. J. Commons, 10, 495–516, https://doi.org/10.18352/ijc.651, 2016.
ASCE RCIA Advisory Council: Report card for America's Infrastrcutre, Tech. Rep., American Society of Civil Engineers, 2013.
Barrett, C. B. and Constas, M. A.: Toward a Theory of Resilience for International Development Applications, P. Natl. Acad. Sci. USA, 111, 14625–14630, https://doi.org/10.1073/pnas.1320880111, 2014.
Berkes, F. and Folke, C.: Linking Social and Ecological Systems for Resilience and Sustainability, Linking Social and Ecological Systems: Management Practices and Social Mechanisms for Building Resilience, 1, 13–20, 1998.
Berkes, F., Colding, J., and Folke, C.: Navigating Social-Ecological Systems: Building Resilience for Complexity and change Building, p. 393, https://doi.org/10.1016/j.biocon.2004.01.010, 2003.
Biggs, R., Schlüter, M., Biggs, D., Bohensky, E. L., BurnSilver, S., Cundill, G., Dakos, V., Daw, T. M., Evans, L. S., Kotschy, K., Leitch, A. M., Meek, C., Quinlan, A., Raudsepp-Hearne, C., Robards, M. D., Schoon, M. L., Schultz, L., and West, P. C.: Toward Principles for Enhancing the Resilience of Ecosystem Services, Annu. Rev. Env. Resour., 37, 421–448, https://doi.org/10.1146/annurev-environ-051211-123836, 2012.
Bode, H. W.: Network Analysis and Feedback Amplifier Design. Bell Telephone Laboratories Series, p. 551, 1945.
Bryant, B. P. and Lempert, R. J.: Thinking inside the Box: A Participatory, Computer-Assisted Approach to Scenario Discovery, Technol. Forecast. Soc., 77, 34–49, https://doi.org/10.1016/j.techfore.2009.08.002, 2010.
Carlson, J. M. and Doyle, J.: Complexity and Robustness, P. Natl. Acad. Sci. USA, 99, 2538–2545, https://doi.org/10.1073/pnas.012582499, 2002.
Carpenter, S., Walker, B., Anderies, J. M., and Abel, N.: From Metaphor to Measurement: Resilience of What to What?, Ecosystems, 4, 765–781, https://doi.org/10.1007/s10021-001-0045-9, 2001.
Carpenter, S. R. and Brock, W. A.: Spatial Complexity, Resilience, and Policy Diversity: Fishing on Lake-Rich Landscapes, Ecol. Soc., 9, 8, 2004.
Carpenter, S. R., Ludwig, D., and Brock, W. A.: Management of Eutrophication for Lakes Subject to Potentially Irreversible Change, Ecol. Appl., 9, 751–771, https://doi.org/10.2307/2641327, 1999a.
Carpenter, S., Brock, W., and Hanson, P.: Ecological and Social Dynamics in Simple Models of Ecosystem Management, Conserv. Ecol., 3, 1–31, https://doi.org/10.5751/ES-00122-030204, 1999b.
Carpenter, S. R., Westley, F., and Turner, M. G.: Surrogates for Resilience of Social-Ecological Systems, Ecosystems, 8, 941–944, https://doi.org/10.1007/s10021-005-0170-y, 2005.
Chang, S. E. and Shinozuka, M.: Measuring Improvements in the Disaster Resilience of Communities, Earthq. Spectra, 20, 739–755, https://doi.org/10.1193/1.1775796, 2004.
Chang, S. E., McDaniels, T., Fox, J., Dhariwal, R., and Longstaff, H.: Toward Disaster-Resilient Cities: Characterizing Resilience of Infrastructure Systems with Expert Judgments, Risk Anal., 34, 416–434, https://doi.org/10.1111/risa.12133, 2014.
Cote, M. and Nightingale, A. J.: Resilience Thinking Meets Social Theory, Prog. Hum. Geog., 36, 475–489, https://doi.org/10.1177/0309132511425708, 2012.
Csete, M. E. and Doyle, J. C.: Reverse Engineering of Biological Complexity, Science, 295, 1664–1669, https://doi.org/10.1126/science.1069981, 2002.
Cumming, G. S. and Peterson, G. D.: Unifying Research on Social–Ecological Resilience and Collapse, Trends Ecol. Evol., 32, 695–713, https://doi.org/10.1016/j.tree.2017.06.014, 2017.
Folke, C.: Resilience: The Emergence of a Perspective for Social–ecological Systems Analyses, Global Environ. Chang., 16, 253–267, https://doi.org/10.1016/j.gloenvcha.2006.04.002, 2006.
Folke, C., Carpenter, S., Elmqvist, T., Gunderson, L., Holling, C. S., and Walker, B.: Resilience and Sustainable Development: Building Adaptive Capacity in a World of Transformations, AMBIO, 31, 437–440, https://doi.org/10.1639/0044-7447(2002)031[0437:RASDBA]2.0.CO;2, 2002.
Folke, C., Carpenter, S. R., Walker, B., Scheffer, M., Chapin, T., and Rockström, J.: Resilience Thinking: Integrating Resilience, Adaptability and Transformability, Ecol. Soc., 15, 20, https://doi.org/10.5751/ES-03610-150420, 2010.
Folke, C., Biggs, R., Norström, A. V., Reyers, B., and Rockström, J.: Social-Ecological Resilience and Biosphere-Based Sustainability Science, Ecol. Soc., 21, 41, https://doi.org/10.5751/ES-08748-210341, 2016.
Groves, D. G. and Lempert, R. J.: A New Analytic Method for Finding Policy-Relevant Scenarios, Global Environ. Chang., 17, 73–85, https://doi.org/10.1016/j.gloenvcha.2006.11.006, 2007.
Gunderson, L. H. and Holling, C. S.: Barriers and Bridges to the Renewal of Ecosystems and Institutions, Columbia University Press, 1995.
Holling, C. S.: Resilience and Stability of Ecological Systems, Annu. Rev. Ecol. Syst., 4, 1–23, https://doi.org/10.1146/annurev.es.04.110173.000245, 1973.
Holling, C. S.: Engineering Resilience versus Ecological Resilience, Engineering Within Ecological Constraints, 1996, 31–44, https://doi.org/10.17226/4919, 1996.
Janssen, M. A., Anderies, J. M., and Walker, B. H.: Robust Strategies for Managing Rangelands with Multiple Stable Attractors, J. Environ. Econ. Manag., 47, 140–162, https://doi.org/10.1016/S0095-0696(03)00069-X, 2004.
Kerner, D. and Thomas, J.: Resilience Attributes of Social-Ecological Systems: Framing Metrics for Management, Resources, 3, Multidisciplinary Digital Publishing Institute, 672–702, https://doi.org/10.3390/resources3040672, 2014.
Kot, M.: Elements of Mathematical Ecology, Cambridge University Press, 2001.
Krokhmal, P., Palmquist, J., and Uryasev, S.: Portfolio optimization with conditional value-at-risk objective and constraints, J. Risk, 4, 43–68, 2002.
Longstaff, P. H., Armstrong, N. J., Perrin, K., Parker, W. M., and Hidek, M.: Building Resilient Communities: A Preliminary Framework for Assessment, Homeland Security Affairs, 6, 1–23, 2010.
May, R. M.: Stability and Complexity in Model Ecosystems, Princeton University Press, 2001.
Mitra, C., Kurths, J., and Donner, R. V.: An Integrative Quantifier of Multistability in Complex Systems Based on Ecological Resilience, Sci. Rep., 5, 1–10, https://doi.org/10.1038/srep16196, 2015.
Muneepeerakul, R. and Anderies, J. M.: Strategic Behaviors and Governance Challenges in Social-Ecological Systems, Earth's Future, 5, 865–76, https://doi.org/10.1002/2017EF000562, 2017.
Ostrom, E., Janssen, M. A., and Anderies, J. M.: Going beyond Panaceas, P. Natl. Acad. Sci. USA, 104, 15176–15178, https://doi.org/10.1073/pnas.0701886104, 2007.
Redman, C. L.: Should Sustainability and Resilience Be Combined or Remain Distinct Pursuits?, Ecol. Soc., 19, 37, https://doi.org/10.5751/ES-06390-190237, 2014.
Rockafellar, R. T. and Uryasev, S.: Optimization of conditional value-at-risk, J. Risk, 2, 21–41, 2000.
Sarykalin, S., Serraino, G., and Uryasev, S.: Value-at-Risk vs. Conditional Value-at-Risk in Risk Management and Optimization, INFORMS TutORials in Operations Research, 27000294, https://doi.org/10.1287/educ.1080.0052, 2014.
Scheffer, M., Brock, W., and Westley, F.: Socioeconomic Mechanisms Preventing Optimum Use of Ecosystem Services: An Interdisciplinary Theoretical Analysis, Ecosystems, 3, 451–471, https://doi.org/10.1007/s100210000040, 2000.
Scheffer, M., Bascompte, J., Brock, W. A., Brovkin, V., Carpenter, S. R., Dakos, V., Held, H., van Nes, E. H., Rietkerk, M., and Sugihara, G.: Early-Warning Signals for Critical Transitions, Nature, 461, 7260, https://doi.org/10.1038/nature08227, 2009.
Scheffer, M., Carpenter, S. R., Lenton, T. M., Bascompte, J., Brock, W., Dakos, V., van de Koppel, J., van de Leemput, I. A., Levin, S. A., van Nes, E. H., Pascual, M., and Vandermeer, J.: Anticipating Critical Transitions, Science, 6105, 344–348, https://doi.org/10.1126/science.1225244, 2012.
Walker, B., Carpenter, S., Anderies, J., Abel, N., Cummings, G., Janssen, M., Lebel, L., Norberg, J., Peterson, G. D., and Pritchard, R.: Resilience Management in Social-Ecological Systems: A Working hypothesis for a Participatory Approach, Conserv. Ecol., 6, p. 14, 2002.
Walker, B., Holling, C. S., Carpenter, S. R., and Kinzig, A.: Resilience, Adaptability and Transformability in Social–Ecological Systems, Ecol. Soc., 9, 5, https://doi.org/10.5751/ES-00650-090205, 2004.
Water Act: Chap. 21, available at: http://www.legislation.gov.uk/ukpga/2014/21/contents/enacted (last access: April 2018), 2014.
Wolpert, D. H. and Macready, W. G.: No Free Lunch Theorems for Optimization, IEEE T. Evolut. Comput., 1, 67–82, https://doi.org/10.1109/4235.585893, 1997.
Zymler, S., Kuhn, D., and Rustem, B.: Worst-Case Value at Risk of Nonlinear Portfolios, Management Science, 59, 172–188, https://doi.org/10.1287/mnsc.1120.1615, 2013.