the Creative Commons Attribution 4.0 License.
the Creative Commons Attribution 4.0 License.
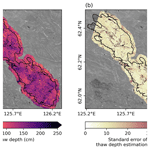
Environmental drivers and remote sensing proxies of post-fire thaw depth in eastern Siberian larch forests
Clement J. F. Delcourt
Moritz Langer
Michael M. Loranty
Brendan M. Rogers
Rebecca C. Scholten
Tatiana A. Shestakova
Anna C. Talucci
Jorien E. Vonk
Sonam Wangchuk
Sander Veraverbeke
Boreal fire regimes are intensifying because of climate change, and the northern parts of boreal forests are underlain by permafrost. Boreal fires combust vegetation and organic soils, which insulate permafrost, and as such deepen the seasonally thawed active layer and can lead to further carbon emissions to the atmosphere. Current understanding of the environmental drivers of post-fire thaw depth is limited but of critical importance. In addition, mapping thaw depth over fire scars may enable a better understanding of the spatial variability in post-fire responses of permafrost soils. We assessed the environmental drivers of post-fire thaw depth using field data from a fire scar in a larch-dominated forest in the continuous permafrost zone in eastern Siberia. Particularly, summer thaw depth was deeper in burned (mean=127.3 cm, standard deviation (SD) = 27.7 cm) than in unburned (98.1 cm, SD=26.9 cm) landscapes 1 year after the fire, yet the effect of fire was modulated by landscape and vegetation characteristics. We found deeper thaw in well-drained upland, in open and mature larch forest often intermixed with Scots pine, and in high-severity burns. The environmental drivers basal area, vegetation density, and burn depth explained 73.3 % of the measured thaw depth variability at the study sites. In addition, we evaluated the relationships between field-measured thaw depth and several remote sensing proxies. Albedo, the differenced normalized burn ratio (dNBR), and the pre-fire normalized difference vegetation index (NDVI) derived from Landsat 8 imagery together explained 66.3 % of the variability in field-measured thaw depth. Moreover, land surface temperature (LST) displayed particularly strong correlations with post-fire thaw depth (r=0.65, p<0.01). Based on these remote sensing proxies and multiple linear regression analysis, we estimated thaw depth over the entire fire scar. Our study reveals some of the governing processes of post-fire thaw depth development and shows the capability of Landsat imagery to estimate post-fire thaw depth at a landscape scale.
- Article
(8872 KB) - Full-text XML
- BibTeX
- EndNote
Permafrost regions, covering Arctic and many boreal ecosystems, store approximately twice as much carbon as is currently in the atmosphere (Hugelius et al., 2014; Miner et al., 2022; Schuur et al., 2022). Permafrost ecosystems are rapidly changing because of climate warming (Natali et al., 2021; Schuur et al., 2022), and changes in fire regimes may accelerate permafrost thaw (Chen et al., 2021b; Descals et al., 2022; Gibson et al., 2018; Scholten et al., 2022). Fires are a major disturbance in permafrost landscapes (Descals et al., 2022; Holloway et al., 2020; Walker et al., 2019), influencing the carbon, energy, and water cycles (Jin et al., 2021; Larjavaara et al., 2017; Li et al., 2021; Liu et al., 2005; Minsley et al., 2016; Randerson et al., 2006).
Boreal fires combust parts of the aboveground biomass and organic soils (Dieleman et al., 2020; Veraverbeke et al., 2021; Walker et al., 2018a, 2020b). When this insulating layer is consumed, charcoal is left on the surface, reducing the albedo immediately after fire in the snow-free season (Rocha et al., 2012; Yoshikawa et al., 2003). This increases the absorption of shortwave radiation and the accumulated heat results in ground warming. Moreover, the combustion of vegetation canopies increases the exposure of the ground to solar radiation and reduces the evaporative cooling effect (French et al., 2016; Johnstone et al., 2010; Li et al., 2021; Liu et al., 2018; Shur and Jorgenson, 2007; Yoshikawa et al., 2003; Zhao et al., 2021). The increased ground heat flux deepens the seasonally thawed active layer above the frozen ground (Holloway et al., 2020; Zhang et al., 2023, 2015). Active layer thickening can enhance soil respiration and consequent emissions of the greenhouse gases carbon dioxide and methane for several decades (Genet et al., 2013; Köster et al., 2018; O'Donnell et al., 2011; Taş et al., 2014; Veraverbeke et al., 2021). Fires thus accelerate permafrost thaw, and although they are a natural component of boreal ecosystems (Kharuk et al., 2021), ongoing changes to the severity and extent of boreal fires (Descals et al., 2022; Turetsky et al., 2011b; Zheng et al., 2023) in combination with climate warming are projected to irreversibly change ice-rich permafrost environments (Chen et al., 2021a; Gibson et al., 2018; Holloway et al., 2020; Zhang et al., 2015).
Previous work has highlighted fires as an important driver of the thickening of the active layer (Brown et al., 2016; Gibson et al., 2018; Jafarov et al., 2013; Zhang et al., 2015). In addition, the active layer thaw depth is strongly controlled by vegetation type and density, organic soil content, soil texture, soil moisture content, topography, and drainage (Bai et al., 2018; Duguay et al., 2013; Fisher et al., 2016; Loranty et al., 2018b; Park et al., 2013). Interactions between these diverse environmental variables lead to a large heterogeneity of the depth of the active layer in the landscape (Holloway et al., 2020; Liu et al., 2012; Shiklomanov and Nelson, 2002). A higher canopy density reduces the amount of solar radiation reaching the ground, which in turn reduces the energy available for soil thawing (Fisher et al., 2016; Stuenzi et al., 2021a, b). In addition, vegetation type and density may alter soil moisture through evapotranspiration, thereby affecting the thermal conditions of the permafrost active layer (Fedorov et al., 2017; Iwahana et al., 2005; Juszak et al., 2016; Marsh et al., 2010). Topography also influences the landscape's vulnerability to burning (Brown et al., 2016). For example, lowlands with limited drainage and wet soils tend to burn with lower severity (Benscoter et al., 2011; Dillon et al., 2011; Holloway et al., 2020; Turetsky et al., 2011a). Thus, the active layer depth is governed by complex interactions between topography, vegetation, and fires (Brown et al., 2016). However, quantitative understanding of these effects over space and time, as well as the strength of underlying drivers, is needed given the intensification of Arctic and boreal fire regimes (Gibson et al., 2018; Michaelides et al., 2019; Natali et al., 2021).
Remote sensing provides information on the ground surface and shallow soils yet cannot directly measure the state of the subsurface. Nevertheless, remote sensing acquires information on various environmental drivers with a known influence on active layer depth or that are indicative of changes in it, such as vegetation status (Brown et al., 2016; Li et al., 2019), ground subsidence (Chen et al., 2020; Michaelides et al., 2019; Molan et al., 2018), land surface temperature (LST) (Bai et al., 2018; Park et al., 2016; Ran et al., 2022; Wen et al., 2022; Yi et al., 2018; Zorigt et al., 2020), surface albedo (Liu et al., 2018; Rogers et al., 2014; Zhao et al., 2021), and fire severity (Delcourt et al., 2021; Lentile et al., 2006; De Santis and Chuvieco, 2007). LST has shown potential to map and model active layer depth over large areas given its relationship to the ground thermal regime (Batbaatar et al., 2020; Hachem et al., 2012; Langer et al., 2010; Obu et al., 2019; Westermann et al., 2017). Obu et al. (2021) produced an active layer thickness (ALT) dataset derived from a remote-sensing-driven model based on Moderate Resolution Imaging Spectroradiometer (MODIS) LST merged with reanalysis near-surface air temperature data. Liu et al. (2024) integrated several MODIS-derived environmental variables, including LST, in a machine learning approach to predict gridded ALT at a spatial resolution of 1 km. However, no studies have used Landsat thermal remote sensing data so far to assess and map thaw depth in a post-fire environment at a spatial resolution of 100 m or less, primarily because of the lower temporal resolution of Landsat data in comparison with, for example, MODIS. The higher spatial resolution from Landsat, however, may offer benefits to map and understand post-fire effects on permafrost soils with more spatial detail, thereby allowing comparisons with field measurements.
In recent years, eastern Siberian larch forests underlain by permafrost have experienced extreme fire activity (Scholten et al., 2022; Talucci et al., 2022a, b; Xu et al., 2022; Zheng et al., 2023). In this study, we investigated post-fire thaw depth in a fire scar in a larch-dominated forest underlain by continuous permafrost in eastern Siberia using field and remote sensing data. Our objectives are twofold. First, we assessed which environmental drivers influence post-fire thaw depth. Second, we investigated which remote sensing proxies relate to field-measured thaw depth. Using these relationships, we mapped post-fire thaw depth over the entire fire scar.
2.1 Study area
Our study area is a fire scar near the Yert rural locality, Republic of Sakha (also known as Yakutia), Russia (Fig. 1). The fire occurred between 30 June and 21 July 2018 and burned approximately 97 000 ha. The area is approximately 200 km west of Yakutsk, the capital of Yakutia. The mean annual air temperature in Yakutsk is −10.2 °C, with means of −42.6 °C in January and 18.7 °C in July, and the mean annual precipitation is 234 mm (Fedorov et al., 2017). The fire scar is in continuous permafrost terrain dominated by Cajander larch (Larix cajanderi), sometimes intermixed with the presence of Scots pine (Pinus sylvestris) and silver birch (Betula pendula), as well as the tall shrubs alder (Alnus spp.) and willow species (Salix spp.). The surface vegetation includes cowberry (Vaccinium vitis-idaea), bog blueberry (Vaccinium uliginosum), crowberry (Empetrum nigrum), rhododendron (Rhododendron dauricum), dog rose (Rosa acicularis), spirea shrub (Spiraea spp.), juniper (Juniperus spp.), fireweed (Epilobium angustifolium), moss (as Ceratodon purpureus and Aulacomnium palustre), and lichens (Delcourt et al., 2021). Cajander larch trees are deciduous needleleaf trees that are adapted to grow on permafrost terrain. They are physiologically and morphologically adapted to withstand the low temperatures, short growing seasons, and cryogenic processes (Abaimov, 2010; Berner et al., 2012). Cajander larch and Scots pine have evolved and adapted to frequent low-severity fires. The fire investigated in this study included a wide range of severities, from low-severity surface fires to high-severity stand-replacing fires (Delcourt et al., 2021).
2.2 Field data
We collected field data in 13 burned and 7 unburned plots (Fig. 1) between 30 July and 8 August 2019, approximately 1 year after the fire (Delcourt et al., 2024). Plot selection aimed to cover a gradient in vegetation composition, fire severity, and landscape position within accessibility constraints. The plots were represented by quadrats of 30 m by 30 m to match the spatial resolution of the Landsat Operational Land Imager (OLI) optical imagery. We aimed to sample plots in areas where fire severity, vegetation, and landscape characteristics were relatively homogeneous (Delcourt et al., 2021).
For each plot, center coordinates and elevation were collected using a high-precision Global Positioning System handheld device (Trimble Geo 7X, GeoExplorer) with 1 m horizontal and vertical accuracy. The geolocation was post-processed using data from the closest reference station (Scripps Orbit and Permanent Array Center (SOPAC), Seismic Station Yakutsk), resulting in decimeter accuracy. A clinometer was used to determine the slope. We also assigned the topographic position of the plot in relation to its surroundings (upland, midslope, lowland) and plot-level site moisture classes following Johnstone et al. (2008). This approach assesses site moisture based on local topographic drainage, thereby accounting for soil texture and permafrost presence. The resulting ordinal scale consists of six site moisture classes ranging between dry and wet (xeric, subxeric, subxeric to mesic, mesic, mesic to subhygric, and subhygric). The six classes represent the potential moisture available for plant growth and should not be confused with actual measurements of soil moisture. This site moisture classification has been used extensively for fire studies in boreal North America (Dieleman et al., 2020; Walker et al., 2018a, b, 2020a).
We measured vegetation characteristics (stand age, basal area, vegetation density, and larch proportion) in a 30 m by 2 m transect in the north–south direction of the plot and with the plot center as the centroid. To characterize vegetation type and density, we inventoried every live and standing dead tree along the transect. Particularly, we recorded tree species, measured diameter at breast height (DBH; 1.3 m), and estimated basal area and stem density.
To characterize fire severity, we used the Geometrically structured Composite Burn Index (GeoCBI) protocol (De Santis and Chuvieco, 2009). GeoCBI is a visual field-based fire severity index in a continuous numeric scale ranging from 0 to 3. The GeoCBI estimates the cumulative impacts of fire over the vertical structure of forest stands and soil surface. Its field protocol is based on a hierarchical and multi-layered sampling design, splitting the plot into five different strata: substrates (ground surface, litter, duff); herbs, low shrubs, and trees less than 1 m; tall shrubs and trees of 1 to 5 m; intermediate trees of 5 to 20 m; and trees higher than 20 m. The strata are divided into subcategories that are evaluated independently according to various visible fire effects. These subcategories, for example, include changes in the color and condition of the soil, consumption of fuels, and char height on tree boles. The subcategories are rated with decimal values between 0, meaning no fire effect, and 3, reflecting high fire severity. Then, the scores of each stratum are obtained by averaging the scores for all criteria and strata are weighted by their fraction of coverage to derive the plot-level GeoCBI value. Previous studies have used this index to assess fire severity in boreal forests (Delcourt et al., 2021; Dieleman et al., 2020; Rogers et al., 2014).
We estimated burn depth in the burned plots by measuring the vertical distance between the top of the residual soil layer and the uppermost adventitious root on larch trees, hereafter referred to as adventitious root height (ARH), following the methodology described in Delcourt et al. (2021). Adventitious roots are fine lateral roots that develop at upper soil horizons as trees grow, responding to unfavorable temperature and moisture conditions deeper in the soil (Rogers et al., 2014). These roots remain visible on the tree trunks several years after a fire (Kajimoto, 2010; Kajimoto et al., 2003). The position of adventitious roots in the soil column can be used to estimate the height of the pre-fire soil surface (Boby et al., 2010; Kasischke and Johnstone, 2005; Rogers et al., 2014). In unburned plots, we determined the location of the highest adventitious root beneath the moss layer as well as the soil organic layer depth (SOL) and derived a linear relationship between SOL and adventitious root height above the mineral soil. We used this relationship in burned plots to reconstruct pre-fire SOL from measurements of ARH and residual SOL depth. Burn depth was then estimated as the difference between reconstructed pre-fire SOL depth and residual SOL depth.
Thaw depth is defined as a measure of the seasonally unfrozen portion of the soil column (the active layer) that lies atop perennially frozen ground (permafrost) (Rocha et al., 2012). The thaw depth was measured using a standard frost probe (stainless-steel rod), which was inserted into the ground to the depth of resistance by the frozen ground. We performed measurements twice (with 1 m increments) every 7.5 m along the belt transect. In some measurements, we were uncertain whether the depth resistance stemmed from frozen ground or was caused by rocks. In the case of uncertainty, we excluded the measurement from the analysis. Our approach resulted in a maximum of 10 thaw depth measurements per plot, which were then averaged to derive the plot-level thaw depth. All our measurements were made between 30 July and 8 August 2019, and we therefore assume negligible seasonal influences on thaw depth between plots.
2.3 Remote sensing data
Landsat 8 Operational Land Imager (OLI) and Thermal Infrared Sensor (TIRS) Collection 2 Level-2 data were used in this study (Table 1). The OLI reflective and the TIRS thermal bands have spatial resolutions of 30 and 100 m, but the latter are resampled and provided at 30 m for consistency purposes. We acquired post-fire images for 23 July 2019, the closest date to the field campaign. For the pre-fire imagery, we used a cloud-free image from 7 July 2016, near the anniversary date of the post-fire imagery. No cloud-free summer images were available from 2017. The acquisition timing of the pre-fire image aligns with recommendations of Key and Benson (2006), since no other disturbance in the landscape interfered with the subject fire between the summers of 2016 and 2017. Two scenes were mosaicked to cover the entire fire perimeter for both pre- and post-fire imagery (Fig. 1). We used the Landsat data to calculate the pre-fire normalized difference vegetation index (NDVI), post-fire albedo, land surface temperature (LST), and differenced normalized burn ratio (dNBR).
We calculated the pre-fire NDVI as follows:
where ρ represents the reflectance of the Landsat 8 OLI bands 4 (0.64–0.67 µm) and 5 (0.85–0.88 µm). We included the pre-fire NDVI as a proxy for fuel availability.
To retrieve albedo (α), we used the narrow-to-broadband conversion formula from Liang (2001) adapted for Landsat 8 as follows (Naegeli et al., 2017):
where ρ is the reflectance of the Landsat 8 OLI bands 2 (0.45–0.51 µm), 4, 5, 6 (1.57–1.65 µm), and 7 (2.11–2.29 µm).
The dNBR was obtained from the bitemporal difference of the normalized burn ratio (NBR) (Eq. 3) between pre- and post-fire scenes (Eq. 4) (Epting et al., 2005; García and Caselles, 1991; Key and Benson, 2006).
The dNBR is an often-used fire severity index and theoretically can range between −2 and 2. In practice, however, most pixel values range between 0 and 1. A dNBR value of zero denotes an unburned pixel, and increasing dNBR values represent higher fire severity (Allen and Sorbel, 2008; Key and Benson, 2006).
For the LST retrieval, we opted for an approach based on the direct inverse solution of the radiative transfer equation (RTE) (Price, 1983). This is a physically based method that is often used with a single thermal band (Jiménez-Muñoz et al., 2009). Inverting and simplifying the RTE to a thermal band center at wavelength λ, the LST can be calculated as
where refers to the at-sensor spectral radiance of the corresponding thermal band. In this study, we used the Landsat 8 TIRS band 10 (10.60–11.19 µm). ελ represents the land surface emissivity (LSE). K1 and K2 are calibration constants for band 10 of the Landsat 8 TIRS (Ihlen and Zanter, 2019). and are the downwelling and upwelling atmospheric radiances and τλ is the atmospheric transmittance. These atmospheric parameters were derived from the Goddard Earth Observing System Version 5 (GEOS-5) Forward Processing for Instrument Teams (FP-IT) data, which are available as atmospheric auxiliary data for the Landsat 8 products (EROS, 2021).
LSE information is essential for the LST retrieval. LSE determines the efficiency of the surface to convert heat energy into radiant energy. We adopted the NDVI threshold method (NDVITHM) of Sobrino et al. (2004) to estimate LSE, but we adapted the method to the specific characteristics of a fire scar. For that, we first computed the NDVI and the fractional vegetation cover (PV) (Carlson and Ripley, 1997):
where NDVImin=0.2 and NDVImax=0.5 (Sobrino et al., 2004, 2008; Sobrino and Raissouni, 2000). Then, we estimated LSE as follows. The spectral notation (λ) is omitted, since a single TIRS band was used here.
In this approach, pixels with NDVI values between 0 and 0.2 were considered non-vegetated (PV=0). Since our study focuses on a fire scar, an LSE value of 0.962 was assigned to these pixels. This value was derived from charcoal spectra from Veraverbeke et al. (2012, 2014) integrated over the Landsat 8 TIRS band 10 spectral response function. If the pixel had an NDVI value between 0.2 and 0.5, it was a mixture of charcoal and vegetation and LSE depended on the PV value calculated according to Eq. (7). dε accounts for the cavity effect due to surface roughness (i.e., dε=0 for flat surfaces) and was approximated by Eq. (8), with an assumed shape factor F′ of 0.55 following Sobrino et al. (1990). Pixels with NDVI values higher than 0.5 were fully vegetated (PV=1), and we assumed an emissivity of 0.990. Finally, when the pixel had a negative NDVI value corresponding to water surface, an LSE value of 0.993 was assigned (Vanhellemont, 2020).
We calculated the average pixel values for all remote sensing metrics within a three-by-three pixel window centered on a given plot's centroid coordinates, which minimized the effects of potential satellite misregistration when comparing these remote sensing metrics with field data (Ahern et al., 1991). We also compared NDVI, albedo, dNBR, and LST values of burned pixels from within the fire perimeter with values of these variables of unburned pixels within a 2 km buffer from the fire perimeter.
2.4 Statistical approach
Our statistical analysis consisted of two major components. First, we assessed the environmental drivers of post-fire thaw depth using our field observations. Second, we evaluated how remote sensing variables can be used to predict field-measured thaw depth. For both analyses, we used an ordinary least-squares (OLS) multiple linear regression (MLR) model with thaw depth as the response variable using the “statsmodels” Python module (Seabold and Perktold, 2010). We hypothesized that post-fire thaw depth relates to variables associated with topography (topographic position, site moisture, slope, and elevation), vegetation (stand age, basal area, vegetation density, and larch proportion), and fire severity (GeoCBI and burn depth). We used the original data (i.e., non-standardized) and ranked the categorical variables topographic position and site moisture on an ordinal scale to include them in the linear analysis. The selection of the environmental variables for the MLR model was based on a stepwise regression approach testing both forward selection and backward elimination. We calculated the relative importance of the retained variables to the MLR model explanatory power using the “pingouin” Python module (Vallat, 2018), which is based on the R package “relaimpo” (Grömping, 2006). The R2 from the MLR model is partitioned by averaging over orderings among regressors using simple unweighted averages as proposed by Lindeman et al. (1980), providing a decomposition of the explained model variance into non-negative contributions. Finally, we developed an MLR model using the remote sensing metrics (post-fire albedo, dNBR, LST, and pre-fire NDVI) as potential predictor variables to assess their capacity as proxies for post-fire thaw depth. The resulting MLR was then spatially extrapolated to derive a continuous map of post-fire thaw depth over the study area.
In addition to these statistical analyses, which included all field plots, we also focused separately on two burned–unburned plot pairs. While our study area captured large variability in landscape position, vegetation characteristics, and fire severity, these plots had the advantage of being spatially adjacent, with a road acting as the fire barrier. Because of this, the landscape and vegetation characteristics of these plots were very similar. As a result, these plot pairs represent interesting study cases in which the fire effect on thaw depth can be unequivocally separated from the landscape and vegetation influences.
3.1 Environmental drivers of post-fire thaw depth
On average, summer thaw depth was deeper in burned (mean=127.3 cm, standard deviation (SD) = 27.7 cm) than in unburned (98.1 cm, SD=26.9 cm) plots (Fig. 2). This difference was statistically significant at p<0.05. Thaw depth was deeper in uplands (Figs. 3, B1a and d) and well-drained (Figs. 3 and B1b) landscape positions; in open (Figs. 3 and 4b), mature (Figs. 3 and B1e), and mixed forests, which included tree species other than Cajander larch (Figs. 3 and B1f); and in high-severity burns (Figs. 3, 4c, and B1g).
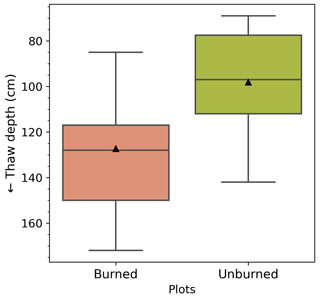
Figure 2The 1-year post-fire summer thaw depth was deeper in burned plots compared to unburned plots. Each box ranges from the first to the third quartile. Whiskers extend to points that lie within 1.5 times the interquartile range. The median is indicated by the horizontal line and the mean by the black triangle.
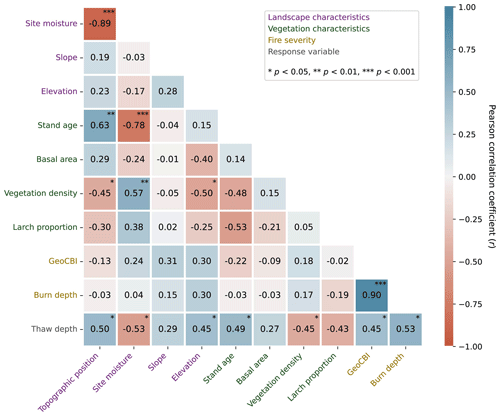
Figure 3Correlation matrix between field-measured environmental variables representing landscape, vegetation and fire severity characteristics, and thaw depth. GeoCBI is the Geometrically structured Composite Burn Index.
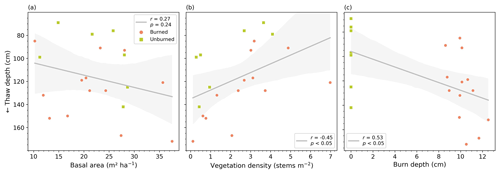
Figure 4Relationships between field-measured thaw depth and (a) basal area, (b) vegetation density, and (c) burn depth. The shading around the regression lines indicates the 95 % confidence interval, while r is the Pearson correlation coefficient.
Based on the results of the stepwise regression for both forward selection and backward elimination, we retained basal area, vegetation density, and burn depth as predictor variables for the MLR (Fig. 4). The multiple linear regression results indicated that the selected environmental drivers together explained 73.3 % of the thaw depth variability. Burn depth was the main contributor to the explanatory power of the MLR model (Table 2).
Table 2Summary of the multiple linear regression for the environmental drivers of thaw depth. SE is the standard error of the corresponding variable's coefficient. The R2 column displays the coefficient of determination of each predictor variable separately as well as for the multiple linear regression model. The relative importance indicates the contribution of each independent variable to the multiple linear regression model R2.
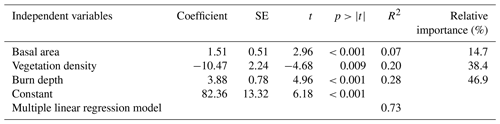
3.2 Remote sensing proxies of thaw depth
Within the fire perimeter, there was considerable spatial variability in albedo, dNBR, LST, and pre-fire NDVI (Figs. 5 and 6). Albedo values inside the fire perimeter had a mean of 0.10 (SD=0.02) compared to mean of 0.12 (SD=0.02) outside the perimeter (Figs. 5a and 6a). The dNBR values of our 13 burned field plots ranged between 0.37 and 0.80, with a mean of 0.59 (SD=0.13), while for the entire fire perimeter the mean was 0.41 (SD=0.27, Figs. 5b and 6b). In addition, LST values inside the fire scar were on average 5.24 K higher than the LST values outside the fire scar (Figs. 5c and 6c). However, for some parts of the fire scar, LST values exceeded the surrounding unburned pixels by approximately 20 K. For the pre-fire NDVI, the distributions of values inside and outside the fire perimeter were similar (Figs. 5d and 6d), with a mean NDVI value of 0.78 (SD=0.08) inside the perimeter and a mean NDVI value of 0.79 (SD=0.07) outside the perimeter.
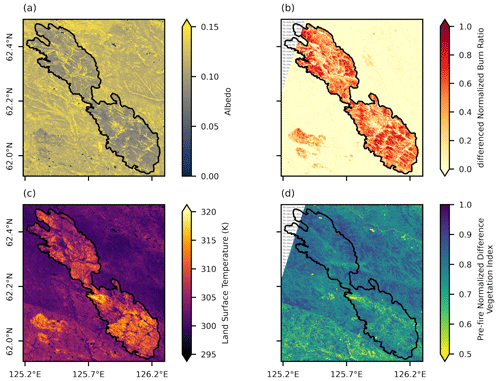
Figure 5Maps showing (a) albedo, (b) differenced normalized burn ratio, (c) land surface temperature, and (d) pre-fire normalized difference vegetation index derived from Landsat 8 imagery. In (b) and (d), the northwestern tip with “no data” indicates a part of the study area that was not covered by the Landsat 8 scenes.
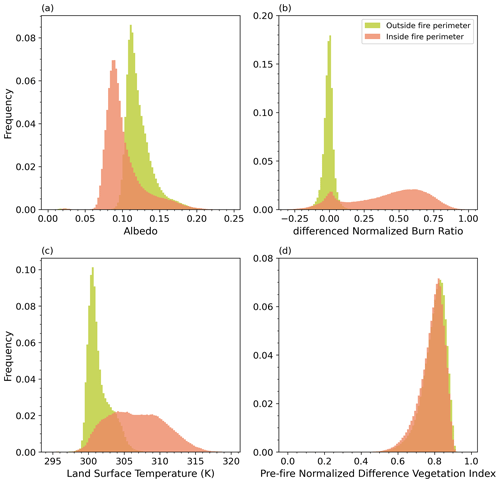
Figure 6Relative frequency distributions of remote sensing proxies derived from Landsat 8 imagery inside and outside the fire perimeters: (a) albedo, (b) differenced normalized burn ratio, (c) land surface temperature, and (d) pre-fire normalized difference vegetation index. The pixels outside the fire perimeter were within 2 km of the fire perimeter.
Thaw tended to be deeper in the plots with lower summer albedo (Fig. 7a), higher dNBR, (Fig. 7b), higher LST (Fig. 7c), and lower pre-fire NDVI (Fig. 7d). The correlation with LST was the strongest, while the correlations between thaw depth and dNBR, albedo, and pre-fire NDVI were not statistically significant at p<0.05 (Fig. C1).
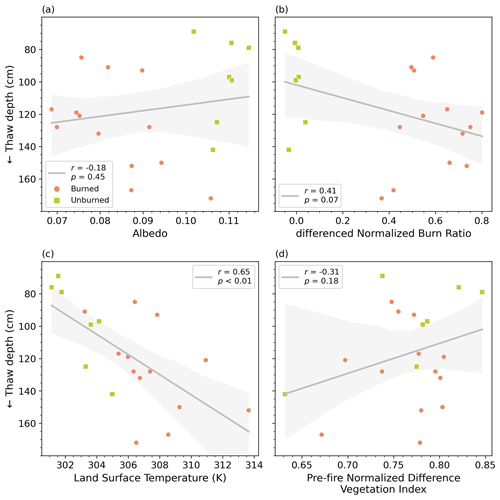
Figure 7Scatter plots and linear regression lines between field-measured thaw depth and remotely sensed (a) albedo, (b) differenced normalized burn ratio, (c) land surface temperature, and (d) pre-fire normalized difference vegetation index. The shading indicates the 95 % confidence interval for the regressions and r is the Pearson correlation coefficient.
Stepwise forward selection indicated that LST was the only variable to be selected for the linear regression, capturing 42.9 % of the variability in field-measured thaw depth. By contrast, stepwise backward elimination pointed dNBR, albedo, and pre-fire NDVI as the variables to be retained in the MLR model. Taken together, these three remote sensing proxies explained 66.3 % of field-measured thaw depth variability. dNBR was the most important predictor in this model configuration (Table 3).
Table 3Summary of the multiple linear regression for the remote sensing proxies of thaw depth. SE is the standard error of the corresponding variable's coefficient. The R2 column displays the coefficient of determination of each predictor variable separately as well as for the multiple linear regression model. The relative importance indicates the contribution of each independent variable to the multiple linear regression model R2.
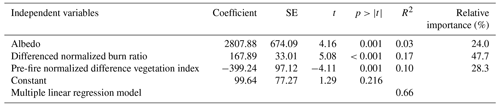
The MLR model for thaw depth based on dNBR, albedo, and pre-fire NDVI enabled spatially continuous thaw depth estimates over the study area, including uncertainty estimates (Fig. 8). We decided on this MLR configuration due to the higher R2 when compared to LST alone. Post-fire thaw depth varied largely within the fire scar, for example between 83.49 cm (5th percentile) and 228.39 cm (95th percentile), with a mean of 139.72 cm (SD=43.76 cm). In contrast, for the pixels outside the fire perimeter, the estimated thaw depth varied between 66.59 cm (5th percentile) and 237.99 cm (95th percentile), with a mean of 125.08 cm (SD=52.15 cm) (Fig. 8c). On average, burned areas thus experienced 14.64 cm deeper summer thaw depth 1 year after the fire than unburned areas.
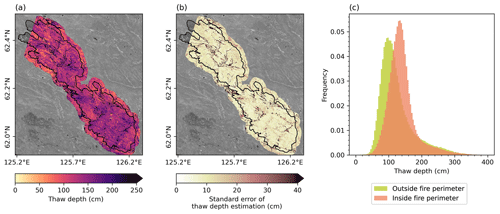
Figure 8Maps of (a) estimated thaw depth, (b) its standard error, and the (c) relative frequency distribution for the pixels inside and outside the fire perimeter. The area outside the fire scar considers a 2 km buffer from the fire perimeter. The northwestern portion of the fire scar was not covered by the pre-fire Landsat imagery, so there is no estimate of thaw depth in this area. In (a) and (b), post-fire Landsat 8 Operational Land Imager (OLI) band 5 is used as the background image.
3.3 Differences between neighboring burned and unburned plots
Our field dataset contained two pairs of burned–unburned plots, which were separated by a road (Fig. 9). The landscape and vegetation characteristics were very similar between adjacent burned and unburned plots and represented mature larch-dominated forest in a subxeric to mesic environment (Table 4). The burned plots experienced medium to high fire severity with GeoCBI values of approximately 2.5 and burn depth from 9.89 to 10.52 cm. The thaw depth in the two burned plots was 53 and 73 cm deeper compared to the paired unburned control plots. In addition, LST values in the burned plots were between 3 and 5 K higher than in the unburned plots, and the burned plots experienced a clear drop in albedo.
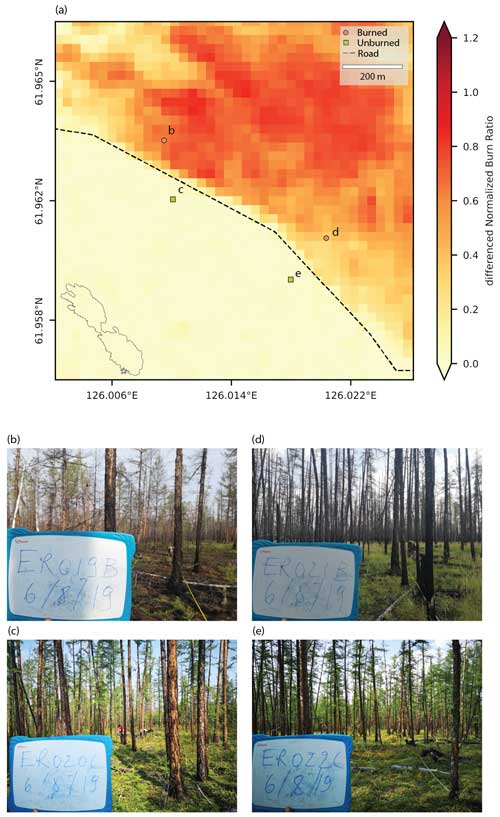
Figure 9Location of the two pairs of burned–unburned plots in the study area (a), including field photographs, which contain the plot identification labels (b–e).
4.1 Environmental drivers of post-fire thaw depth
Fire accelerates permafrost active layer thickening (Gibson et al., 2018). However, this process is also modulated by topographic and vegetation characteristics (Brown et al., 2015, 2016; Fisher et al., 2016). We found that well-drained terrain positions generally experience deeper thaw. Previous studies have reported contrasting findings concerning moisture effects. For example, Fisher et al. (2016) found deeper thaw with increased soil surface moisture. Other studies, in contrast, found thinner active layers for wet soils (Clayton et al., 2021; Zhang et al., 2005) because these wet soils require more energy for all ice to melt (i.e., latent heat of fusion), resulting in shallower active layers (Clayton et al., 2021). We found that forest density was a thaw-limiting factor, in agreement with Fisher et al. (2016), who identified canopy shading and evapotranspiration as mechanisms to explain this relationship between forest density and active layer depth. With denser canopies, less solar radiation can reach the ground, be absorbed, and be converted into heat, resulting in a shallower thaw (Fedorov et al., 2017; Iwahana et al., 2005; Juszak et al., 2014, 2016). Denser vegetation may also transpire more, thereby reducing the thermal conductivity in the soil (Fisher et al., 2016; Iwahana et al., 2005). Moreover, the presence of Cajander larch trees is closely coupled with permafrost (Herzschuh, 2020). In turn, permafrost helps to provide sufficient water to the trees by preventing it from draining away quickly (Zhang et al., 2011). Our results reflect this vegetation–permafrost interaction, where plots with fewer larch trees showed more pronounced thaw. Our field observations also showed that larch trees were prevalent and tend to dominate in mesic and hydric parts of the landscape, including in lowlands with shallow permafrost thaw. Conversely, Scots pine was more prevalent in the drier upland areas, which demonstrated deeper thaw (Fig. 3). Scots pine trees in our study region tend to occur on moderately warm and dry landscape positions such as ridges and hilltops. These locations were characterized by sandy soils and deeper thaw. In contrast, larch trees are dominant in lowland depressions of watersheds, characterized by cool and often waterlogged soils and shallower permafrost thaw (Isaev et al., 2010). Eastern Siberian larch trees are one of the only tree species that can successfully grow on permafrost soils with very shallow thaw depth. This is due to their ability to develop an adventitious rooting system (Herzschuh, 2020; Kajimoto, 2010). Larch and also Scots pine have evolved under periodic fire conditions, with the capacity to regenerate and grow after fire (Kharuk et al., 2021). These competitive advantages have led to the establishment of larch dominance in Siberian permafrost environments, and fire occurrence has been a prerequisite for this evolutionary process. However, increased permafrost degradation could lead to a shift in dominant tree species, with larch trees being replaced by pine trees and other tree species (Zhang et al., 2011).
Fire results in a rise in surface and soil temperature, which consequently increases thaw depth (Gibson et al., 2018; Jiang et al., 2015; Li et al., 2019; Nossov et al., 2013; Smith et al., 2015). Our results are in agreement with the overall pattern of rapid active layer thickening in the years immediately after the fire (Holloway et al., 2020). We found a mean difference of about 30 cm in field-measured summer thaw depth between burned and unburned plots 1 year after the fire, and our study thereby also fills a data gap for the effects of fires on active layer thickening in Siberia (Petrov et al., 2022; Ponomarev et al., 2020). Our case studies of two burned–unburned plot pairs divided by a fire barrier, though limited in sample size, helped us to separate the impact of fire on the active layer thaw from topographic and vegetation influences.
Increasing fire severity tends to increase thaw depth (Alexander et al., 2018; Holloway et al., 2020; Jafarov et al., 2013; Jiang et al., 2015; Li et al., 2019). The positive correlations between burn depth and thaw depth and between GeoCBI and thaw depth found in this study are consistent with these previous results. In addition, fire severity is often influenced by vegetation and topographic conditions. In our study, for example, the well-drained plots are on upland landscape positions with deeper thaw depths, while lowland areas are usually wetter with limited drainage and shallow thaw (Figs. 3 and B1). These wet lowland ecosystems also tend to burn with lower severity (Benscoter et al., 2011; Dillon et al., 2011; Holloway et al., 2020; Turetsky et al., 2011a). The thaw measurements in burned plots, as measured in the field, are conservative estimates as we did not account for the combustion of the organic layer in these measurements. We found a mean burn depth of 10.2 cm (SD=1.0 cm; Fig. 4c) in our burned plots. As a consequence, the thaw depth in reference to the pre-fire ground level is even deeper than compared to the post-fire ground level. This further elucidates the importance of fire severity, or burn depth, as a driver of post-fire permafrost thaw.
4.2 Remote sensing proxies of thaw depth
Charcoal residues after a fire are dark in color, decreasing the summer albedo in the fire scar. More solar radiation is absorbed by the darker surface, leading to a thickening of the thawed layer. Liu et al. (2018) and Zhao et al. (2021) assessed post-fire summer albedo change in the boreal forests of eastern Siberia and North America. They reported small immediate (1 year after the fire) albedo declines: a maximum decline of 0.02 in Siberia and a mean decline of 0.01 in North America. We found a mean drop of 0.02, equaling a relative decrease of 17 %, in summer albedo due to fire. The studies by Liu et al. (2018) and Zhao et al. (2021) used MODIS products with coarser spatial resolution, which may partly explain the differences from our study, which used 30 m Landsat data. While the individual relationship between albedo and thaw depth was not strong, albedo may still be a useful metric to assess fire influences on permafrost soils, in part because albedo is found to be a good proxy for aboveground fire severity (Fig. C1; Rogers et al., 2014). In line with previous studies (Barrett et al., 2010; Delcourt et al., 2021; Rogers et al., 2014; Veraverbeke et al., 2015), the dNBR was also strongly correlated with burn depth and as a result reasonably strongly related to thaw depth (r=0.41, p=0.07). Somewhat surprisingly, pre-fire NDVI did not perform well as a proxy for fuel density as it showed a low correlation with the field-measured pre-fire vegetation density (Fig. C1). This may be because the NDVI in Siberian larch forest is strongly influenced by understory vegetation, thereby confounding its value as a proxy for forest density (Bendavid et al., 2023; Loranty et al., 2018a).
We found that LST was the strongest stand-alone remotely sensed predictor of post-fire thaw depth. This can be explained by the fact that it captures variations in topography, vegetation, and fire severity, which all showed important influences on post-fire thaw depth. LST has been used before to model ALT (Obu et al., 2019; Ran et al., 2022; Wen et al., 2022) and to study the impacts of fire on biophysical processes (Liu et al., 2018; Zhao et al., 2021). Although these studies used LST with a different approach, using time series at a lower spatial resolution, we have built upon these studies by relating LST to post-fire thaw depth in a predictive model at landscape scale.
We demonstrated the potential of Landsat imagery to predict post-fire thaw depth, resulting in a spatially continuous map of post-fire thaw depth at landscape scale. The MLR model using the Landsat derived proxies was able to explain 66.3 % of the field-measured thaw depth variability. This performance is only slightly lower than the 73.3 % explained variability based on only field data. Despite this achievement, some limitations are worth mentioning. The Landsat 8 TIRS images have a native spatial resolution of 100 m but are distributed at 30 m after a non-reversible cubic convolution resampling. Even with data collected at a lower native spatial resolution than the optical bands, LST was still our best stand-alone remote sensing proxy for post-fire thaw depth. The availability of LST data at a higher spatial resolution would therefore likely be beneficial for the model performance. In addition, our field dataset was somewhat limited in size. Because of this, the MLR models should be seen as first-order statistical models of the relationships between field-measured and spaceborne environmental variables with thaw depth. As more measurements of post-fire permafrost thaw may become available over time (Loranty et al., 2021), more advanced statistical approaches, including machine learning techniques, could be explored to better understand and map post-fire permafrost thaw over larger spatiotemporal scales.
We used a snapshot of LST that temporally overlapped with the field data collection, using only one image. Alternatively, one could use all available images within the growing season and gap-fill with, e.g., coarse-spatial-resolution but high-temporal-resolution MODIS LST imagery. It might thereby be possible to apply the concept of growing or thawing degree days, which is often used to approximate thaw depth evolution and ALT (Hinkel and Nelson, 2003; Romanovsky and Osterkamp, 1997; Streletskiy et al., 2015, 2008). In addition, we measured and estimated thaw depth in the middle of the growing season. However, the most common variable of interest for intercomparison is ALT, i.e., the maximum depth at the end of the growing season (Zhang et al., 2021; Michaelides et al., 2019; WMO, 2016). The rate at which the active layer deepens decreases towards the end of the growing season. The thaw depth in August has been found to be approximately 6 % less than the ALT (Boike et al., 1998; Clayton et al., 2021; Hinkel et al., 2001; Michaelides et al., 2019; Zhang and Stamnes, 1998). Streletskiy et al. (2008) found that 95 % to 99 % of maximum thaw propagation is achieved by mid-August and that landscape-specific thaw depth patterns are related to landform elements that show spatial regularity at the landscape scale. We thus assumed that the spatial variability of the mid-season thaw depth is a reliable indicator of the spatial variability of ALT at the end of the growing season in the study region.
For future research, in addition to optical and thermal data, synthetic aperture radar (SAR) datasets can be used to study spatiotemporal variations of fire effects on permafrost landscapes. SAR data enable the measurement of various physical properties of fire scars, including surface roughness and near-surface soil moisture. Moreover, the interferometric SAR (InSAR) technique allows precise measurements of ground movement, with millimeter vertical accuracy (Strozzi et al., 2018). The effectiveness of this approach has been demonstrated in previous studies conducted in permafrost environments in Alaska and Siberia (Michaelides et al., 2019; Yanagiya et al., 2023; Yanagiya and Furuya, 2020). Future work could integrate fire-induced permafrost subsidence from InSAR with optical and thermal data to predict post-fire thaw depth based on multi-sensor satellite products.
Boreal fires combust parts or all of the aboveground vegetation and soil organic layer. The resulting loss of insulation of the permafrost soil leads to an increased ground heat flux, promoting permafrost thaw. With the escalating extent and severity of fires in Siberia, there is a growing need to better understand the processes that govern fire-induced permafrost thaw, including spatial estimations of thaw depth. Here, we evaluated the impact of fire, landscape, and vegetation characteristics on post-fire thaw depth. In addition, we assessed the capacity of remote sensing proxies to estimate post-fire thaw depth at a landscape scale. Using field data from a recent fire scar in eastern Siberian larch-dominated forests, we confirmed that increasing fire severity indeed induces deeper thaw. This process was, however, mediated by landscape and vegetation characteristics. Thaw depth was deeper in well-drained uplands, characterized by open and mature forests, which included mixed stands of Cajander larch and Scots pine. The environmental drivers basal area, vegetation density, and burn depth together explained 73.3 % of the thaw depth variability. We explored the use of Landsat-8-derived metrics as proxies for post-fire thaw depth. A multiple linear regression model ingesting albedo, dNBR, and pre-fire NDVI explained 66.3 % of the field-measured variability in thaw depth. LST individually explained 42.9 % of this variability. The correlation between LST and thaw depth was particularly strong, highlighting the potential of LST as a proxy for post-fire thaw depth. We estimated post-fire thaw depth at a landscape scale by spatially extrapolating the regression model of the Landsat data. Future work could further investigate the consistency of our findings across space and time to better understand the impact of fire on permafrost ecosystems across the Arctic–boreal region.
Table A1Summary of the remote sensing metrics used in the study. ρ represents the reflectance of the Landsat 8 Operational Land Imager (OLI) bands. refers to the Landsat 8 Thermal Infrared Sensor (TIRS) band 10 radiance. K1 and K2 are calibration constants. and are the downwelling and upwelling atmospheric radiances and τλ is the atmospheric transmittance.
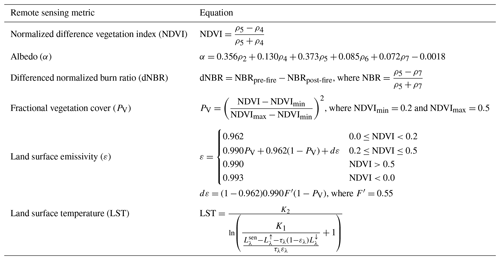
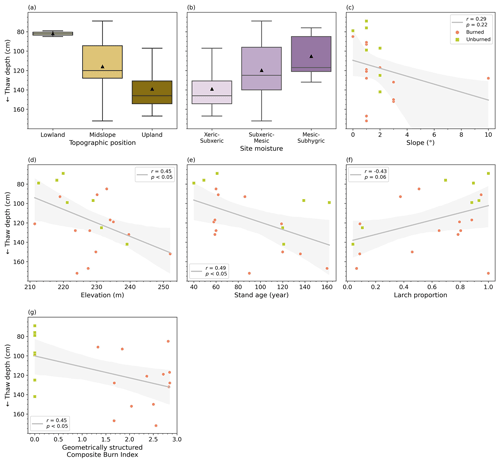
Figure B1Relationships between thaw depth and (a) topographic position, (b) site moisture, (c) slope, (d) elevation, (e) stand age, (f) larch proportion, and (g) Geometrically structured Composite Burn Index. In (a) and (b), each box ranges from the first to the third quartile. Whiskers extend to points that lie within 1.5 times the interquartile range. The median is indicated by the horizontal line and the mean by the black triangle. For site moisture, classes were grouped together for better visualization. In the regression lines, shading indicates the 95 % confidence interval and r is the Pearson correlation coefficient.
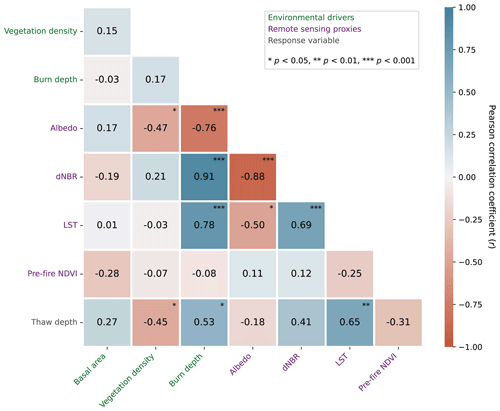
Figure C1Correlation matrix of selected environmental drivers, remote sensing proxies, and thaw depth. GeoCBI is the Geometrically structured Composite Burn Index. LST is the land surface temperature. dNBR refers to the differenced normalized burn ratio and NDVI to the normalized difference vegetation index.
Field data can be accessed at https://doi.org/10.5281/zenodo.10840088 (Delcourt et al., 2024). Landsat 8 Operational Land Imager (OLI) and Thermal Infrared Sensor (TIRS) data are publicly available and courtesy of the US Geological Survey. The code with the statistical methods used to analyze the data can be obtained from the corresponding author upon request.
LRD and SV conceptualized the research and developed the methodology. CJFD and SV organized the field campaign. CJFD, BMR, RCS, TAS, and SV collected the field data. LRD performed the formal analysis, investigation, and visualization. LRD wrote and prepared the original draft with inputs from SV and SW. CJFD, ML, MML, BMR, RCS, TAS, ACT, JEV, SW, and SV reviewed and edited the manuscript. SV acquired the funding, administrated the project, and supervised the research.
The contact author has declared that none of the authors has any competing interests.
Publisher's note: Copernicus Publications remains neutral with regard to jurisdictional claims made in the text, published maps, institutional affiliations, or any other geographical representation in this paper. While Copernicus Publications makes every effort to include appropriate place names, the final responsibility lies with the authors.
We thank Trofim C. Maximov and Roman E. Petrov for logistical and field support. We are grateful to Frédéric van Vessem, who performed a first exploratory analysis of the thaw depth dataset as part of his MSc thesis. We thank the reviewers for their thoughtful comments and efforts to improve our manuscript.
This work was supported by the Dutch Research Council (NWO) through a Vidi grant (grant no. 016.Vidi.189.070) and by the European Research Council (ERC) through a Consolidator grant under the European Union's Horizon 2020 research and innovation program (grant no. 101000987), both awarded to Sander Veraverbeke. Tatiana A. Shestakova acknowledges the support received from the Beatriu de Pinòs Programme and the Ministry of Research and Universities of the Government of Catalonia (2020 BP 00126).
This paper was edited by Anping Chen and reviewed by Rongyun Tang and one anonymous referee.
Abaimov, A. P.: Geographical Distribution and Genetics of Siberian Larch Species, in: Permafrost Ecosystems, edited by: Osawa, A., Zyryanova, O., Matsuura, Y., Kajimoto, T., and Wein, R., Springer, Dordrecht, 41–58, https://doi.org/10.1007/978-1-4020-9693-8_3, 2010.
Ahern, F. J., Erdle, T., Maclean, D. A., and Kneppeck, I. D.: A quantitative relationship between forest growth rates and Thematic Mapper reflectance measurements, Int. J. Remote Sens., 12, 387–400, https://doi.org/10.1080/01431169108929660, 1991.
Alexander, H. D., Natali, S. M., Loranty, M. M., Ludwig, S. M., Spektor, V. V., Davydov, S., Zimov, N., Trujillo, I., and Mack, M. C.: Impacts of increased soil burn severity on larch forest regeneration on permafrost soils of far northeastern Siberia, Forest Ecol. Manag., 417, 144–153, https://doi.org/10.1016/j.foreco.2018.03.008, 2018.
Allen, J. L. and Sorbel, B.: Assessing the differenced Normalized Burn Ratio's ability to map burn severity in the boreal forest and tundra ecosystems of Alaska's national parks, Int. J. Wildland Fire, 17, 463–475, https://doi.org/10.1071/WF08034, 2008.
Bai, X., Yang, J., Tao, B., and Ren, W.: Spatio-temporal variations of soil active layer thickness in Chinese boreal forests from 2000 to 2015, Remote Sens.-Basel, 10, 1225, https://doi.org/10.3390/rs10081225, 2018.
Barrett, K., Kasischke, E. S., McGuire, A. D., Turetsky, M. R., and Kane, E. S.: Modeling fire severity in black spruce stands in the Alaskan boreal forest using spectral and non-spectral geospatial data, Remote Sens. Environ., 114, 1494–1503, https://doi.org/10.1016/j.rse.2010.02.001, 2010.
Batbaatar, J., Gillespie, A. R., Sletten, R. S., Mushkin, A., Amit, R., Liaudat, D. T., Liu, L., and Petrie, G.: Toward the detection of permafrost using land-surface temperature mapping, Remote Sens.-Basel, 12, 695, https://doi.org/10.3390/rs12040695, 2020.
Bendavid, N. S., Alexander, H. D., Davydov, S. P., Kropp, H., Mack, M. C., Natali, S. M., Spawn-Lee, S. A., Zimov, N. S., and Loranty, M. M.: Shrubs Compensate for Tree Leaf Area Variation and Influence Vegetation Indices in Post-Fire Siberian Larch Forests, J. Geophys. Res.-Biogeo., 128, e2022JG007107, https://doi.org/10.1029/2022JG007107, 2023.
Benscoter, B. W., Thompson, D. K., Waddington, J. M., Flannigan, M. D., Wotton, B. M., De Groot, W. J., and Turetsky, M. R.: Interactive effects of vegetation, soil moisture and bulk density on depth of burning of thick organic soils, Int. J. Wildland Fire, 20, 418–429, https://doi.org/10.1071/WF08183, 2011.
Berner, L. T., Beck, P. S. A., Loranty, M. M., Alexander, H. D., Mack, M. C., and Goetz, S. J.: Cajander larch (Larix cajanderi) biomass distribution, fire regime and post-fire recovery in northeastern Siberia, Biogeosciences, 9, 3943–3959, https://doi.org/10.5194/bg-9-3943-2012, 2012.
Boby, L. A., Schuur, E. A. G., Mack, M. C., Verbyla, D., and Johnstone, J. F.: Quantifying fire severity, carbon, and nitrogen emissions in Alaska's boreal forest, Ecol. Appl., 20, 1633–1647, https://doi.org/10.1890/08-2295.1, 2010.
Boike, J., Roth, K., and Overduin, P. P.: Thermal and hydrologic dynamics of the active layer at a continuous permafrost site (Taymyr Peninsula, Siberia), Water Resour. Res., 34, 355–363, https://doi.org/10.1029/97WR03498, 1998.
Brown, D. R. N., Jorgenson, M. T., Douglas, T. A., Romanovsky, V. E., Kielland, K., Hiemstra, C., Euskirchen, E. S., and Ruess, R. W.: Interactive effects of wildfire and climate on permafrost degradation in Alaskan lowland forests, J. Geophys. Res.-Biogeo., 120, 1619–1637, https://doi.org/10.1002/2015JG003033, 2015.
Brown, D. R. N., Jorgenson, M. T., Kielland, K., Verbyla, D. L., Prakash, A., and Koch, J. C.: Landscape effects of wildfire on permafrost distribution in interior Alaska derived from remote sensing, Remote Sens.-Basel, 8, 654, https://doi.org/10.3390/rs8080654, 2016.
Carlson, T. N. and Ripley, D. A.: On the relation between NDVI, fractional vegetation cover, and leaf area index, Remote Sens. Environ., 62, 241–252, https://doi.org/10.1016/S0034-4257(97)00104-1, 1997.
Chen, J., Wu, Y., O'Connor, M., Cardenas, M. B., Schaefer, K., Michaelides, R., and Kling, G.: Active layer freeze-thaw and water storage dynamics in permafrost environments inferred from InSAR, Remote Sens. Environ., 248, 112007, https://doi.org/10.1016/j.rse.2020.112007, 2020.
Chen, Y., Lara, M. J., Jones, B. M., Frost, G. V., and Hu, F. S.: Thermokarst acceleration in Arctic tundra driven by climate change and fire disturbance, One Earth, 4, 1718–1729, https://doi.org/10.1016/j.oneear.2021.11.011, 2021a.
Chen, Y., Romps, D. M., Seeley, J. T., Veraverbeke, S., Riley, W. J., Mekonnen, Z. A., and Randerson, J. T.: Future increases in Arctic lightning and fire risk for permafrost carbon, Nat. Clim. Change, 11, 404–410, https://doi.org/10.1038/s41558-021-01011-y, 2021b.
Clayton, L. K., Schaefer, K., Battaglia, M. J., Bourgeau-Chavez, L., Chen, J., Chen, R. H., Chen, A., Bakian-Dogaheh, K., Grelik, S., Jafarov, E., Liu, L., Michaelides, R. J., Moghaddam, M., Parsekian, A. D., Rocha, A. V., Schaefer, S. R., Sullivan, T., Tabatabaeenejad, A., Wang, K., Wilson, C. J., Zebker, H. A., Zhang, T., and Zhao, Y.: Active layer thickness as a function of soil water content, Environ. Res. Lett., 16, 055028, https://doi.org/10.1088/1748-9326/abfa4c, 2021.
De Santis, A. and Chuvieco, E.: Burn severity estimation from remotely sensed data: Performance of simulation versus empirical models, Remote Sens. Environ., 108, 422–435, https://doi.org/10.1016/j.rse.2006.11.022, 2007.
De Santis, A. and Chuvieco, E.: GeoCBI: A modified version of the Composite Burn Index for the initial assessment of the short-term burn severity from remotely sensed data, Remote Sens. Environ., 113, 554–562, https://doi.org/10.1016/j.rse.2008.10.011, 2009.
Delcourt, C. J. F., Combee, A., Izbicki, B., Mack, M. C., Maximov, T., Petrov, R., Rogers, B. M., Scholten, R. C., Shestakova, T. A., van Wees, D., and Veraverbeke, S.: Evaluating the differenced normalized burn ratio for assessing fire severity using sentinel-2 imagery in northeast siberian larch forests, Remote Sens.-Basel, 13, 2311, https://doi.org/10.3390/rs13122311, 2021.
Delcourt, C. J. F., Rogers, B. M., Akhmetzyanov, L., Izbicki, B., Scholten, R. C., Shestakova, T., van Wees, D., Mack, M. M., Sass-Klaassen, U., and Veraverbeke, S.: Burned and Unburned Boreal Larch Forest Site Data, Northeast Siberia, Zenodo [data set], https://doi.org/10.5281/zenodo.10840088, 2024.
Descals, A., Gaveau, D. L. A., Verger, A., Sheil, D., Naito, D., and Peñuelas, J.: Unprecedented fire activity above the Arctic Circle linked to rising temperatures, Science, 378, 532–537, https://doi.org/10.1126/science.abn9768, 2022.
Dieleman, C. M., Rogers, B. M., Potter, S., Veraverbeke, S., Johnstone, J. F., Laflamme, J., Solvik, K., Walker, X. J., Mack, M. C., and Turetsky, M. R.: Wildfire combustion and carbon stocks in the southern Canadian boreal forest: Implications for a warming world, Glob. Change Biol., 26, 6062–6079, https://doi.org/10.1111/gcb.15158, 2020.
Dillon, G. K., Holden, Z. A., Morgan, P., Crimmins, M. A., Heyerdahl, E. K., and Luce, C. H.: Both topography and climate affected forest and woodland burn severity in two regions of the western US, 1984 to 2006, Ecosphere, 2, 1–33, https://doi.org/10.1890/ES11-00271.1, 2011.
Duguay, C. R., Zhang, T., Leverington, D. W., and Romanovsky, V. E.: Satellite Remote Sensing of Permafrost and Seasonally Frozen Ground, in: Geophysical Monograph Series, vol. 163, Blackwell Publishing Ltd, 91–118, https://doi.org/10.1029/163GM06, 2013.
EROS (Earth Resources Observation and Science Center): GEOS-5 FP-IT, Collection 2, U.S. Geological Survey [data set], https://doi.org/10.5066/P9NP30Z1, 2021.
Epting, J., Verbyla, D., and Sorbel, B.: Evaluation of remotely sensed indices for assessing burn severity in interior Alaska using Landsat TM and ETM+, Remote Sens. Environ., 96, 328–339, https://doi.org/10.1016/j.rse.2005.03.002, 2005.
Fedorov, A. N., Iwahana, G., Konstantinov, P. Y., Machimura, T., Argunov, R. N., Efremov, P. V., Lopez, L. M. C., and Takakai, F.: Variability of Permafrost and Landscape Conditions Following Clear Cutting of Larch Forest in Central Yakutia, Permafrost Periglac., 28, 331–338, https://doi.org/10.1002/ppp.1897, 2017.
Fisher, J. P., Estop-Aragonés, C., Thierry, A., Charman, D. J., Wolfe, S. A., Hartley, I. P., Murton, J. B., Williams, M., and Phoenix, G. K.: The influence of vegetation and soil characteristics on active-layer thickness of permafrost soils in boreal forest, Glob. Change Biol., 22, 3127–3140, https://doi.org/10.1111/gcb.13248, 2016.
French, N. H. F., Whitley, M. A., and Jenkins, L. K.: Fire disturbance effects on land surface albedo in Alaskan tundra, J. Geophys. Res.-Biogeo., 121, 841–854, https://doi.org/10.1002/2015JG003177, 2016.
García, M. J. L. and Caselles, V.: Mapping burns and natural reforestation using thematic Mapper data, Geocarto Int., 6, 31–37, https://doi.org/10.1080/10106049109354290, 1991.
Genet, H., McGuire, A. D., Barrett, K., Breen, A., Euskirchen, E. S., Johnstone, J. F., Kasischke, E. S., Melvin, A. M., Bennett, A., Mack, M. C., Rupp, T. S., Schuur, A. E. G., Turetsky, M. R., and Yuan, F.: Modeling the effects of fire severity and climate warming on active layer thickness and soil carbon storage of black spruce forests across the landscape in interior Alaska, Environ. Res. Lett., 8, 045016, https://doi.org/10.1088/1748-9326/8/4/045016, 2013.
Gibson, C. M., Chasmer, L. E., Thompson, D. K., Quinton, W. L., Flannigan, M. D., and Olefeldt, D.: Wildfire as a major driver of recent permafrost thaw in boreal peatlands, Nat. Commun., 9, 3041, https://doi.org/10.1038/s41467-018-05457-1, 2018.
Grömping, U.: Relative Importance for Linear Regression in R: The Package relaimpo, J. Stat. Softw., 17, , 1–27, https://doi.org/10.18637/jss.v017.i01, 2006.
Hachem, S., Duguay, C. R., and Allard, M.: Comparison of MODIS-derived land surface temperatures with ground surface and air temperature measurements in continuous permafrost terrain, The Cryosphere, 6, 51–69, https://doi.org/10.5194/tc-6-51-2012, 2012.
Herzschuh, U.: Legacy of the Last Glacial on the present-day distribution of deciduous versus evergreen boreal forests, Global Ecol. Biogeogr., 29, 198–206, https://doi.org/10.1111/geb.13018, 2020.
Hinkel, K. M. and Nelson, F. E.: Spatial and temporal patterns of active layer thickness at Circumpolar Active Layer Monitoring (CALM) sites in northern Alaska, 1995–2000, J. Geophys. Res., 108, 8168, https://doi.org/10.1029/2001JD000927, 2003.
Hinkel, K. M., Paetzold, F., Nelson, F. E., and Bockheim, J. G.: Patterns of soil temperature and moisture in the active layer and upper permafrost at Barrow, Alaska: 1993–1999, Global Planet. Change, 29, 293–309, https://doi.org/10.1016/S0921-8181(01)00096-0, 2001.
Holloway, J. E., Lewkowicz, A. G., Douglas, T. A., Li, X., Turetsky, M. R., Baltzer, J. L., and Jin, H.: Impact of wildfire on permafrost landscapes: A review of recent advances and future prospects, Permafrost Periglac., 31, 371–382, https://doi.org/10.1002/ppp.2048, 2020.
Hugelius, G., Strauss, J., Zubrzycki, S., Harden, J. W., Schuur, E. A. G., Ping, C.-L., Schirrmeister, L., Grosse, G., Michaelson, G. J., Koven, C. D., O'Donnell, J. A., Elberling, B., Mishra, U., Camill, P., Yu, Z., Palmtag, J., and Kuhry, P.: Estimated stocks of circumpolar permafrost carbon with quantified uncertainty ranges and identified data gaps, Biogeosciences, 11, 6573–6593, https://doi.org/10.5194/bg-11-6573-2014, 2014.
Ihlen, V. and Zanter, K.: Landsat 8 (L8) Data Users Handbook, Version 5.0, LSDS-1574, Sioux Falls, South Dakota, 116 pp., 2019.
Isaev, A. P., Protopopov, A. V., Protopopova, V. V., Egorova, A. A., Timofeyev, P. A., Nikolaev, A. N., Shurduk, I. F., Lytkina, L. P., Ermakov, N. B., Nikitina, N. V., Efimova, A. P., Zakharova, V. I., Cherosov, M. M., Nikolin, E. G., Sosina, N. K., Troeva, E. I., Gogoleva, P. A., Kuznetsova, L. V., Pestryakov, B. N., Mironova, S. I., and Sleptsova, N. P.: Vegetation of Yakutia: Elements of Ecology and Plant Sociology, Springer, Dordrecht, 143–260, https://doi.org/10.1007/978-90-481-3774-9_3, 2010.
Iwahana, G., Machimura, T., Kobayashi, Y., Fedorov, A. N., Konstantinov, P. Y., and Fukuda, M.: Influence of forest clear-cutting on the thermal and hydrological regime of the active layer near Yakutsk, eastern Siberia, J. Geophys. Res.-Biogeo., 110, G02004, https://doi.org/10.1029/2005jg000039, 2005.
Jafarov, E. E., Romanovsky, V. E., Genet, H., McGuire, A. D., and Marchenko, S. S.: The effects of fire on the thermal stability of permafrost in lowland and upland black spruce forests of interior Alaska in a changing climate, Environ. Res. Lett., 8, 035030, https://doi.org/10.1088/1748-9326/8/3/035030, 2013.
Jiang, Y., Rocha, A. V., O'Donnell, J. A., Drysdale, J. A., Rastetter, E. B., Shaver, G. R., and Zhuang, Q.: Contrasting soil thermal responses to fire in Alaskan tundra and boreal forest, J. Geophys. Res.-Earth, 120, 363–378, https://doi.org/10.1002/2014JF003180, 2015.
Jiménez-Muñoz, J. C., Cristobal, J., Sobrino, J. A. J. A., Sòria, G., Ninyerola, M., Pons, X., Jimenez-Munoz, J. C., Cristobal, J., Sobrino, J. A. J. A., Soria, G., Ninyerola, M., Pons, X., and Pons, X.: Revision of the Single-Channel Algorithm for Land Surface Temperature Retrieval From Landsat Thermal-Infrared Data, IEEE T. Geosci. Remote, 47, 339–349, https://doi.org/10.1109/TGRS.2008.2007125, 2009.
Jin, X. Y., Jin, H. J., Iwahana, G., Marchenko, S. S., Luo, D. L., Li, X. Y., and Liang, S. H.: Impacts of climate-induced permafrost degradation on vegetation: A review, Adv. Clim. Change Res., 12, 29–47, https://doi.org/10.1016/j.accre.2020.07.002, 2021.
Johnstone, J. F., Hollingsworth, T. N., and Chapin, F. S.: A key for predicting postfire successional trajectories in black spruce stands of interior Alaska, https://doi.org/10.2737/PNW-GTR-767, 2008.
Johnstone, J. F., Hollingsworth, T. N., Chapin, F. S., and Mack, M. C.: Changes in fire regime break the legacy lock on successional trajectories in Alaskan boreal forest, Glob. Change Biol., 16, 1281–1295, https://doi.org/10.1111/j.1365-2486.2009.02051.x, 2010.
Juszak, I., Erb, A. M., Maximov, T. C., and Schaepman-Strub, G.: Arctic shrub effects on NDVI, summer albedo and soil shading, Remote Sens. Environ., 153, 79–89, https://doi.org/10.1016/j.rse.2014.07.021, 2014.
Juszak, I., Eugster, W., Heijmans, M. M. P. D., and Schaepman-Strub, G.: Contrasting radiation and soil heat fluxes in Arctic shrub and wet sedge tundra, Biogeosciences, 13, 4049–4064, https://doi.org/10.5194/bg-13-4049-2016, 2016.
Kajimoto, T.: Root System Development of Larch Trees Growing on Siberian Permafrost, in: Permafrost Ecosystems, edited by: Osawa, A., Zyryanova, O., Matsuura, Y., Kajimoto, T., and Wein, R., Springer, Dordrecht, 303–330, https://doi.org/10.1007/978-1-4020-9693-8_16, 2010.
Kajimoto, T., Matsuura, Y., Osawa, A., Prokushkin, A. S., Sofronov, M. A., and Abaimov, A. P.: Root system development of Larix gmelinii trees affected by micro-scale conditions of permafrost soils in central Siberia, Plant Soil, 255, 281–292, https://doi.org/10.1023/A:1026175718177, 2003.
Kasischke, E. S. and Johnstone, J. F.: Variation in postfire organic layer thickness in a black spruce forest complex in interior Alaska and its effects on soil temperature and moisture, Can. J. Forest Res., 35, 2164–2177, https://doi.org/10.1139/x05-159, 2005.
Key, C. H. and Benson, N. C.: Landscape Assessment (LA), in: FIREMON: Fire effects monitoring and inventory system, edited by: Lutes, D. C., Keane, R. E., Caratti, J. F., Key, C. H., Benson, N. C., Sutherland, S., and Gangi, L. J., U. S. Department of Agriculture, Forest Service, Rocky Mountain Research Station, Fort Collins, CO, 1–55, https://doi.org/10.2737/RMRS-GTR-164, 2006.
Kharuk, V. I., Ponomarev, E. I., Ivanova, G. A., Dvinskaya, M. L., Coogan, S. C. P., and Flannigan, M. D.: Wildfires in the Siberian taiga, Ambio, 50, 1953–1974, https://doi.org/10.1007/s13280-020-01490-x, 2021.
Köster, E., Köster, K., Berninger, F., Prokushkin, A., Aaltonen, H., Zhou, X., and Pumpanen, J.: Changes in fluxes of carbon dioxide and methane caused by fire in Siberian boreal forest with continuous permafrost, J. Environ. Manage., 228, 405–415, https://doi.org/10.1016/j.jenvman.2018.09.051, 2018.
Langer, M., Westermann, S., and Boike, J.: Spatial and temporal variations of summer surface temperatures of wet polygonal tundra in Siberia – implications for MODIS LST based permafrost monitoring, Remote Sens. Environ., 114, 2059–2069, https://doi.org/10.1016/j.rse.2010.04.012, 2010.
Larjavaara, M., Berninger, F., Palviainen, M., Prokushkin, A., and Wallenius, T.: Post-fire carbon and nitrogen accumulation and succession in Central Siberia, Sci. Rep.-UK, 7, 12776, https://doi.org/10.1038/s41598-017-13039-2, 2017.
Lentile, L. B., Holden, Z. A., Smith, A. M. S., Falkowski, M. J., Hudak, A. T., Morgan, P., Lewis, S. A., Gessler, P. E., and Benson, N. C.: Remote sensing techniques to assess active fire characteristics and post-fire effects, Int. J. Wildland Fire, 15, 319–345, https://doi.org/10.1071/WF05097, 2006.
Li, X., Jin, H., He, R., Huang, Y., Wang, H., Luo, D., Jin, X., Lanzhi, L., Wang, L., Li, W., Wei, C., Chang, X., Yang, S., and Yu, S.: Effects of forest fires on the permafrost environment in the northern Da Xing'anling (Hinggan) mountains, Northeast China, Permafrost Periglac., 30, 163–177, https://doi.org/10.1002/ppp.2001, 2019.
Li, X. Y., Jin, H. J., Wang, H. W., Marchenko, S. S., Shan, W., Luo, D. L., He, R. X., Spektor, V., Huang, Y. D., Li, X. Y., and Jia, N.: Influences of forest fires on the permafrost environment: A review, Adv. Clim. Change Res., 12, 48–65, https://doi.org/10.1016/j.accre.2021.01.001, 2021.
Liang, S.: Narrowband to broadband conversions of land surface albedo I, Remote Sens. Environ., 76, 213–238, https://doi.org/10.1016/S0034-4257(00)00205-4, 2001.
Lindeman, R. H., Merenda, P. F., and Gold, R. Z.: Introduction to Bivariate and Multivariate Analysis, Scott, Foresman, Glenview, IL, ISBN-10: 0673150992, ISBN-13: 978-0673150998, 1980.
Liu, H., Randerson, J. T., Lindfors, J., and Chapin, F. S.: Changes in the surface energy budget after fire in boreal ecosystems of interior Alaska: An annual perspective, J. Geophys. Res.-Atmos., 110, D13101, https://doi.org/10.1029/2004JD005158, 2005.
Liu, L., Schaefer, K., Zhang, T., and Wahr, J.: Estimating 1992–2000 average active layer thickness on the Alaskan North Slope from remotely sensed surface subsidence, J. Geophys. Res.-Earth, 117, F01005, https://doi.org/10.1029/2011JF002041, 2012.
Liu, Z., Ballantyne, A. P., and Cooper, L. A.: Increases in Land Surface Temperature in Response to Fire in Siberian Boreal Forests and Their Attribution to Biophysical Processes, Geophys. Res. Lett., 45, 6485–6494, https://doi.org/10.1029/2018GL078283, 2018.
Liu, Z., Kimball, J. S., Ballantyne, A., Watts, J. D., Natali, S. M., Rogers, B. M., Yi, Y., Klene, A. E., Moghaddam, M., Du, J., and Zona, D.: Widespread deepening of the active layer in northern permafrost regions from 2003 to 2020, Environ. Res. Lett., 19, 014020, https://doi.org/10.1088/1748-9326/ad0f73, 2024.
Loranty, M. M., Davydov, S., Kropp, H., Alexander, H., Mack, M., Natali, S., and Zimov, N.: Vegetation Indices Do Not Capture Forest Cover Variation in Upland Siberian Larch Forests, Remote Sens.-Basel, 10, 1686, https://doi.org/10.3390/rs10111686, 2018a.
Loranty, M. M., Abbott, B. W., Blok, D., Douglas, T. A., Epstein, H. E., Forbes, B. C., Jones, B. M., Kholodov, A. L., Kropp, H., Malhotra, A., Mamet, S. D., Myers-Smith, I. H., Natali, S. M., O'Donnell, J. A., Phoenix, G. K., Rocha, A. V., Sonnentag, O., Tape, K. D., and Walker, D. A.: Reviews and syntheses: Changing ecosystem influences on soil thermal regimes in northern high-latitude permafrost regions, Biogeosciences, 15, 5287–5313, https://doi.org/10.5194/bg-15-5287-2018, 2018b.
Loranty, M., Talucci, A., Berner, L., Breen, A., Buma, B., Delcourt, C., Dieleman, C., Douglas, T., Frost, G., Gaglioti, B., Gibson, C., Hewitt, R., Hollingsworth, T., Lara, M., Mack, M., Manies, K., Natali, S., O'Donnell, J., Olefeldt, D., Paulson, A., Rocha, A., Rogers, B., Sistla, S., Sizov, O., Turetsky, M., Veraverbeke, S., and Walvoord, M.: A Synthesis of Wildfire Impacts on Permafrost Thaw Depth Across Arctic and Boreal Ecosystems, in: AGU Fall Meeting Abstracts, 13–17 December 2021, 14 December 2021, New Orleans, LA, B23C-01, https://agu.confex.com/agu/fm21/meetingapp.cgi/Paper/818424 (last access: 14 November 2024), 2021.
Marsh, P., Bartlett, P., MacKay, M., Pohl, S., and Lantz, T.: Snowmelt energetics at a shrub tundra site in the western Canadian Arctic, Hydrol. Process., 24, 3603–3620, https://doi.org/10.1002/hyp.7786, 2010.
Michaelides, R. J., Schaefer, K., Zebker, H. A., Parsekian, A., Liu, L., Chen, J., Natali, S., Ludwig, S., and Schaefer, S. R.: Inference of the impact of wildfire on permafrost and active layer thickness in a discontinuous permafrost region using the remotely sensed active layer thickness (ReSALT) algorithm, Environ. Res. Lett., 14, 035007, https://doi.org/10.1088/1748-9326/aaf932, 2019.
Miner, K. R., Turetsky, M. R., Malina, E., Bartsch, A., Tamminen, J., McGuire, A. D., Fix, A., Sweeney, C., Elder, C. D., and Miller, C. E.: Permafrost carbon emissions in a changing Arctic, Nat. Rev. Earth Environ., 3, 55–67, https://doi.org/10.1038/s43017-021-00230-3, 2022.
Minsley, B. J., Pastick, N. J., Wylie, B. K., Brown, D. R. N., and Andy Kass, M.: Evidence for nonuniform permafrost degradation after fire in boreal landscapes, J. Geophys. Res.-Earth, 121, 320–335, https://doi.org/10.1002/2015JF003781, 2016.
Molan, Y. E., Kim, J. W., Lu, Z., Wylie, B., and Zhu, Z.: Modeling wildfire-induced permafrost deformation in an Alaskan boreal forest using InSAR observations, Remote Sens.-Basel, 10, 405, https://doi.org/10.3390/rs10030405, 2018.
Naegeli, K., Damm, A., Huss, M., Wulf, H., Schaepman, M., and Hoelzle, M.: Cross-Comparison of Albedo Products for Glacier Surfaces Derived from Airborne and Satellite (Sentinel-2 and Landsat 8) Optical Data, Remote Sens.-Basel, 9, 110, https://doi.org/10.3390/rs9020110, 2017.
Natali, S. M., Holdren, J. P., Rogers, B. M., Treharne, R., Duffy, P. B., Pomerance, R., and MacDonald, E.: Permafrost carbon feedbacks threaten global climate goals, P. Natl. Acad. Sci. USA, 118, e2100163118, https://doi.org/10.1073/pnas.2100163118, 2021.
Nossov, D. R., Torre Jorgenson, M., Kielland, K., and Kanevskiy, M. Z.: Edaphic and microclimatic controls over permafrost response to fire in interior Alaska, Environ. Res. Lett., 8, 035013, https://doi.org/10.1088/1748-9326/8/3/035013, 2013.
Obu, J., Westermann, S., Bartsch, A., Berdnikov, N., Christiansen, H. H., Dashtseren, A., Delaloye, R., Elberling, B., Etzelmüller, B., Kholodov, A., Khomutov, A., Kääb, A., Leibman, M. O., Lewkowicz, A. G., Panda, S. K., Romanovsky, V., Way, R. G., Westergaard-Nielsen, A., Wu, T., Yamkhin, J., and Zou, D.: Northern Hemisphere permafrost map based on TTOP modelling for 2000–2016 at 1 km2 scale, Earth-Sci. Rev., 193, 299–316, https://doi.org/10.1016/j.earscirev.2019.04.023, 2019.
Obu, J., Westermann, S., Barboux, C., Bartsch, A., Delaloye, R., Grosse, G., Heim, B., Hugelius, G., Irrgang, A., Kääb, A. M., Kroisleitner, C., Matthes, H., Nitze, I., Pellet, C., Seifert, F. M., Strozzi, T., Wegmüller, U., Wieczorek, M., and Wiesmann, A.: ESA Permafrost Climate Change Initiative (Permafrost_cci): Permafrost active layer thickness for the Northern Hemisphere, v3.0, https://doi.org/10.5285/67a3f8c8dc914ef99f7f08eb0d997e23, 28 June 2021.
O'Donnell, J. A., Harden, J. W., McGuire, A. D., and Romanovsky, V. E.: Exploring the sensitivity of soil carbon dynamics to climate change, fire disturbance and permafrost thaw in a black spruce ecosystem, Biogeosciences, 8, 1367–1382, https://doi.org/10.5194/bg-8-1367-2011, 2011.
Park, H., Walsh, J., Fedorov, A. N., Sherstiukov, A. B., Iijima, Y., and Ohata, T.: The influence of climate and hydrological variables on opposite anomaly in active-layer thickness between Eurasian and North American watersheds, The Cryosphere, 7, 631–645, https://doi.org/10.5194/tc-7-631-2013, 2013.
Park, H., Kim, Y., and Kimball, J. S.: Widespread permafrost vulnerability and soil active layer increases over the high northern latitudes inferred from satellite remote sensing and process model assessments, Remote Sens. Environ., 175, 349–358, https://doi.org/10.1016/j.rse.2015.12.046, 2016.
Petrov, M. I., Fedorov, A. N., Konstantinov, P. Y., and Argunov, R. N.: Variability of Permafrost and Landscape Conditions Following Forest Fires in the Central Yakutian Taiga Zone, Land-Basel, 11, 496, https://doi.org/10.3390/land11040496, 2022.
Ponomarev, E., Masyagina, O., Litvintsev, K., Ponomareva, T., Shvetsov, E., and Finnikov, K.: The effect of post-fire disturbances on a seasonally thawed layer in the permafrost larch forests of central Siberia, Forests, 11, 790, https://doi.org/10.3390/F11080790, 2020.
Price, J. C.: Estimating surface temperatures from satellite thermal infrared data – A simple formulation for the atmospheric effect, Remote Sens. Environ., 13, 353–361, https://doi.org/10.1016/0034-4257(83)90036-6, 1983.
Ran, Y., Li, X., Cheng, G., Che, J., Aalto, J., Karjalainen, O., Hjort, J., Luoto, M., Jin, H., Obu, J., Hori, M., Yu, Q., and Chang, X.: New high-resolution estimates of the permafrost thermal state and hydrothermal conditions over the Northern Hemisphere, Earth Syst. Sci. Data, 14, 865–884, https://doi.org/10.5194/essd-14-865-2022, 2022.
Randerson, J. T., Liu, H., Flanner, M. G., Chambers, S. D., Jin, Y., Hess, P. G., Pfister, G., Mack, M. C., Treseder, K. K., Welp, L. R., Chapin, F. S., Harden, J. W., Goulden, M. L., Lyons, E., Neff, J. C., Schuur, E. A. G., and Zender, C. S.: The impact of boreal forest fire on climate warming, Science, 314, 1130–1132, https://doi.org/10.1126/science.1132075, 2006.
Rocha, A. V., Loranty, M. M., Higuera, P. E., MacK, M. C., Hu, F. S., Jones, B. M., Breen, A. L., Rastetter, E. B., Goetz, S. J., and Shaver, G. R.: The footprint of Alaskan tundra fires during the past half-century: Implications for surface properties and radiative forcing, Environ. Res. Lett., 7, 044039, https://doi.org/10.1088/1748-9326/7/4/044039, 2012.
Rogers, B. M., Veraverbeke, S., Azzari, G., Czimczik, C. I., Holden, S. R., Mouteva, G. O., Sedano, F., Treseder, K. K., and Randerson, J. T.: Quantifying fire-wide carbon emissions in interior Alaska using field measurements and Landsat imagery, J. Geophys. Res.-Biogeo., 119, 1608–1629, https://doi.org/10.1002/2014JG002657, 2014.
Romanovsky, V. E. and Osterkamp, T. E.: Thawing of the Active Layer on the Coastal Plain of the Alaskan Arctic, Permafrost Periglac., 8, 1–22, https://doi.org/10.1002/(SICI)1099-1530(199701)8:1<1::AID-PPP243>3.0.CO;2-U, 1997.
Scholten, R. C., Coumou, D., Luo, F., and Veraverbeke, S.: Early snowmelt and polar jet dynamics co-influence recent extreme Siberian fire seasons, Science, 378, 1005–1009, https://doi.org/10.1126/science.abn4419, 2022.
Schuur, E. A. G., Abbott, B. W., Commane, R., Ernakovich, J., Euskirchen, E., Hugelius, G., Grosse, G., Jones, M., Koven, C., Leshyk, V., Lawrence, D., Loranty, M. M., Mauritz, M., Olefeldt, D., Natali, S., Rodenhizer, H., Salmon, V., Schädel, C., Strauss, J., Treat, C., and Turetsky, M.: Permafrost and Climate Change: Carbon Cycle Feedbacks From the Warming Arctic, Annu. Rev. Env. Resour., 47, 343–371, https://doi.org/10.1146/annurev-environ-012220-011847, 2022.
Seabold, S. and Perktold, J.: Statsmodels: Econometric and Statistical Modeling with Python, in: Proceedings of the 9th Python in Science Conference, 28 June–3 July 2010, Austin, Texas, 92–96, https://doi.org/10.25080/Majora-92bf1922-011, 2010.
Shiklomanov, N. I. and Nelson, F. E.: Active-layer mapping at regional scales: A 13 year spatial time series for the Kuparuk region, north-central Alaska, Permafrost Periglac., 13, 219–230, https://doi.org/10.1002/ppp.425, 2002.
Shur, Y. L. and Jorgenson, M. T.: Patterns of permafrost formation and degradation in relation to climate and ecosystems, Permafrost Periglac., 18, 7–19, https://doi.org/10.1002/ppp.582, 2007.
Smith, S. L., Riseborough, D. W., and Bonnaventure, P. P.: Eighteen Year Record of Forest Fire Effects on Ground Thermal Regimes and Permafrost in the Central Mackenzie Valley, NWT, Canada, Permafrost Periglac., 26, 289–303, https://doi.org/10.1002/ppp.1849, 2015.
Sobrino, J. A. and Raissouni, N.: Toward remote sensing methods for land cover dynamic monitoring: Application to Morocco, Int. J. Remote Sens., 21, 353–366, https://doi.org/10.1080/014311600210876, 2000.
Sobrino, J. A., Caselles, V., and Becker, F.: Significance of the remotely sensed thermal infrared measurements obtained over a citrus orchard, ISPRS J. Photogramm., 44, 343–354, https://doi.org/10.1016/0924-2716(90)90077-O, 1990.
Sobrino, J. A., Jiménez-Muñoz, J. C., and Paolini, L.: Land surface temperature retrieval from LANDSAT TM 5, Remote Sens. Environ., 90, 434–440, https://doi.org/10.1016/j.rse.2004.02.003, 2004.
Sobrino, J. A., Jimenez-Munoz, J. C., Soria, G., Romaguera, M., Guanter, L., Moreno, J., Plaza, A., and Martinez, P.: Land Surface Emissivity Retrieval From Different VNIR and TIR Sensors, IEEE T. Geosci. Remote, 46, 316–327, https://doi.org/10.1109/TGRS.2007.904834, 2008.
Streletskiy, D., Anisimov, O., and Vasiliev, A.: Permafrost Degradation, in: Snow and Ice-Related Hazards, Risks, and Disasters, Elsevier, 303–344, https://doi.org/10.1016/B978-0-12-394849-6.00010-X, 2015.
Streletskiy, D. A., Shiklomanov, N. I., Nelson, F. E., and Klene, A. E.: Thirteen Years of Observations at Alaskan CALM Sites: Long-Term Active Layer and Ground Surface Temperature Trends, in: Proceedings of the Ninth International Conference on Permafrost, 29 June–3 July 2008, Fairbanks, Alaska, 1727–1732, ISBN: 978-0-9800179-3-9, 2008.
Strozzi, T., Antonova, S., Günther, F., Mätzler, E., Vieira, G., Wegmüller, U., Westermann, S., and Bartsch, A.: Sentinel-1 SAR Interferometry for Surface Deformation Monitoring in Low-Land Permafrost Areas, Remote Sens.-Basel, 10, 1360, https://doi.org/10.3390/rs10091360, 2018.
Stuenzi, S. M., Boike, J., Cable, W., Herzschuh, U., Kruse, S., Pestryakova, L. A., Schneider von Deimling, T., Westermann, S., Zakharov, E. S., and Langer, M.: Variability of the surface energy balance in permafrost-underlain boreal forest, Biogeosciences, 18, 343–365, https://doi.org/10.5194/bg-18-343-2021, 2021a.
Stuenzi, S. M., Boike, J., Gädeke, A., Herzschuh, U., Kruse, S., Pestryakova, L. A., Westermann, S., and Langer, M.: Sensitivity of ecosystem-protected permafrost under changing boreal forest structures, Environ. Res. Lett., 16, 084045, https://doi.org/10.1088/1748-9326/ac153d, 2021b.
Talucci, A. C., Loranty, M. M., and Alexander, H. D.: Siberian taiga and tundra fire regimes from 2001–2020, Environ. Res. Lett., 17, 025001, https://doi.org/10.1088/1748-9326/ac3f07, 2022a.
Talucci, A. C., Loranty, M. M., and Alexander, H. D.: Spatial patterns of unburned refugia in Siberian larch forests during the exceptional 2020 fire season, Global Ecol. Biogeogr., 31, 2041–2055, https://doi.org/10.1111/geb.13529, 2022b.
Taş, N., Prestat, E., McFarland, J. W., Wickland, K. P., Knight, R., Berhe, A. A., Jorgenson, T., Waldrop, M. P., and Jansson, J. K.: Impact of fire on active layer and permafrost microbial communities and metagenomes in an upland Alaskan boreal forest, ISME J., 8, 1904–1919, https://doi.org/10.1038/ismej.2014.36, 2014.
Turetsky, M. R., Donahue, W. F., and Benscoter, B. W.: Experimental drying intensifies burning and carbon losses in a northern peatland, Nat. Commun., 2, 514, https://doi.org/10.1038/ncomms1523, 2011a.
Turetsky, M. R., Kane, E. S., Harden, J. W., Ottmar, R. D., Manies, K. L., Hoy, E., and Kasischke, E. S.: Recent acceleration of biomass burning and carbon losses in Alaskan forests and peatlands, Nat. Geosci., 4, 27–31, https://doi.org/10.1038/ngeo1027, 2011b.
Vallat, R.: Pingouin: statistics in Python, J. Open Source Softw., 3, 1026, https://doi.org/10.21105/joss.01026, 2018.
Vanhellemont, Q.: Automated water surface temperature retrieval from Landsat 8/TIRS, Remote Sens. Environ., 237, 111518, https://doi.org/10.1016/j.rse.2019.111518, 2020.
Veraverbeke, S., Hook, S. J., and Harris, S.: Synergy of VSWIR (0.4–2.5 µm) and MTIR (3.5–12.5 µm) data for post-fire assessments, Remote Sens. Environ., 124, 771–779, https://doi.org/10.1016/j.rse.2012.06.028, 2012.
Veraverbeke, S., Stavros, E. N., and Hook, S. J.: Assessing fire severity using imaging spectroscopy data from the Airborne Visible/Infrared Imaging Spectrometer (AVIRIS) and comparison with multispectral capabilities, Remote Sens. Environ., 154, 153–163, https://doi.org/10.1016/j.rse.2014.08.019, 2014.
Veraverbeke, S., Rogers, B. M., and Randerson, J. T.: Daily burned area and carbon emissions from boreal fires in Alaska, Biogeosciences, 12, 3579–3601, https://doi.org/10.5194/bg-12-3579-2015, 2015.
Veraverbeke, S., Delcourt, C. J. F., Kukavskaya, E., Mack, M., Walker, X., Hessilt, T., Rogers, B., and Scholten, R. C.: Direct and longer-term carbon emissions from arctic-boreal fires: A short review of recent advances, Curr. Opin. Environ. Sci. Hlth., 23, 100277, https://doi.org/10.1016/j.coesh.2021.100277, 2021.
Walker, X. J., Baltzer, J. L., Cumming, S. G., Day, N. J., Johnstone, J. F., Rogers, B. M., Solvik, K., Turetsky, M. R., and Mack, M. C.: Soil organic layer combustion in boreal black spruce and jack pine stands of the Northwest Territories, Canada, Int. J. Wildland Fire, 27, 125–134, https://doi.org/10.1071/WF17095, 2018a.
Walker, X. J., Rogers, B. M., Baltzer, J. L., Cumming, S. G., Day, N. J., Goetz, S. J., Johnstone, J. F., Schuur, E. A. G., Turetsky, M. R., and Mack, M. C.: Cross-scale controls on carbon emissions from boreal forest megafires, Glob. Change Biol., 24, 4251–4265, https://doi.org/10.1111/gcb.14287, 2018b.
Walker, X. J., Baltzer, J. L., Cumming, S. G., Day, N. J., Ebert, C., Goetz, S., Johnstone, J. F., Potter, S., Rogers, B. M., Schuur, E. A. G., Turetsky, M. R., and Mack, M. C.: Increasing wildfires threaten historic carbon sink of boreal forest soils, Nature, 572, 520–523, https://doi.org/10.1038/s41586-019-1474-y, 2019.
Walker, X. J., Baltzer, J. L., Bourgeau-Chavez, L. L., Day, N. J., De groot, W. J., Dieleman, C., Hoy, E. E., Johnstone, J. F., Kane, E. S., Parisien, M. A., Potter, S., Rogers, B. M., Turetsky, M. R., Veraverbeke, S., Whitman, E., and Mack, M. C.: ABoVE: Synthesis of Burned and Unburned Forest Site Data, AK and Canada, 1983–2016, https://doi.org/10.3334/ORNLDAAC/1744, 2020a.
Walker, X. J., Rogers, B. M., Veraverbeke, S., Johnstone, J. F., Baltzer, J. L., Barrett, K., Bourgeau-Chavez, L., Day, N. J., de Groot, W. J., Dieleman, C. M., Goetz, S., Hoy, E., Jenkins, L. K., Kane, E. S., Parisien, M. A., Potter, S., Schuur, E. A. G., Turetsky, M., Whitman, E., and Mack, M. C.: Fuel availability not fire weather controls boreal wildfire severity and carbon emissions, Nat. Clim. Change, 10, 1130–1136, https://doi.org/10.1038/s41558-020-00920-8, 2020b.
Wen, A., Wu, T., Wu, X., Zhu, X., Li, R., Ni, J., Hu, G., Qiao, Y., Zou, D., Chen, J., Wang, D., and Lou, P.: Evaluation of MERRA-2 land surface temperature dataset and its application in permafrost mapping over China, Atmos. Res., 279, 106373, https://doi.org/10.1016/j.atmosres.2022.106373, 2022.
Westermann, S., Peter, M., Langer, M., Schwamborn, G., Schirrmeister, L., Etzelmüller, B., and Boike, J.: Transient modeling of the ground thermal conditions using satellite data in the Lena River delta, Siberia, The Cryosphere, 11, 1441–1463, https://doi.org/10.5194/tc-11-1441-2017, 2017.
WMO: The Global Observing System for Climate: Implementation Needs, GCOS-200, 316 pp., https://library.wmo.int/idurl/4/55469 (last access: 14 November 2024), 2016.
Xu, W., Scholten, R. C., Hessilt, T. D., Liu, Y., and Veraverbeke, S.: Overwintering fires rising in eastern Siberia, Environ. Res. Lett., 17, 045005, https://doi.org/10.1088/1748-9326/ac59aa, 2022.
Yanagiya, K. and Furuya, M.: Post-Wildfire Surface Deformation Near Batagay, Eastern Siberia, Detected by L-Band and C-Band InSAR, J. Geophys. Res.-Earth, 125, e2019JF005473, https://doi.org/10.1029/2019JF005473, 2020.
Yanagiya, K., Furuya, M., Danilov, P., and Iwahana, G.: Transient Freeze-Thaw Deformation Responses to the 2018 and 2019 Fires Near Batagaika Megaslump, Northeast Siberia, J. Geophys. Res.-Earth, 128, e2022JF006817, https://doi.org/10.1029/2022JF006817, 2023.
Yi, Y., Kimball, J. S., Chen, R. H., Moghaddam, M., Reichle, R. H., Mishra, U., Zona, D., and Oechel, W. C.: Characterizing permafrost active layer dynamics and sensitivity to landscape spatial heterogeneity in Alaska, The Cryosphere, 12, 145–161, https://doi.org/10.5194/tc-12-145-2018, 2018.
Yoshikawa, K., Bolton, W. R., Romanovsky, V. E., Fukuda, M., and Hinzman, L. D.: Impacts of wildfire on the permafrost in the boreal forests of interior Alaska, J. Geophys. Res.-Atmos., 108, 8148, https://doi.org/10.1029/2001jd000438, 2003.
Zhang, C., Douglas, T. A., and Anderson, J. E.: Modeling and mapping permafrost active layer thickness using field measurements and remote sensing techniques, Int. J. Appl. Earth Obs., 102, 102455, https://doi.org/10.1016/j.jag.2021.102455, 2021.
Zhang, C., Douglas, T. A., Brodylo, D., and Jorgenson, M. T.: Linking repeat lidar with Landsat products for large scale quantification of fire-induced permafrost thaw settlement in interior Alaska, Environ. Res. Lett., 18, 015003, https://doi.org/10.1088/1748-9326/acabd6, 2023.
Zhang, N., Yasunari, T., and Ohta, T.: Dynamics of the larch taiga-permafrost coupled system in Siberia under climate change, Environ. Res. Lett., 6, 024003, https://doi.org/10.1088/1748-9326/6/2/024003, 2011.
Zhang, T. and Stamnes, K.: Impact of climatic factors on the active layer and permafrost at Barrow, Alaska, Permafrost Periglac., 9, 229–246, https://doi.org/10.1002/(SICI)1099-1530(199807/09)9:3<229::AID-PPP286>3.0.CO;2-T, 1998.
Zhang, T., Frauenfeld, O. W., Serreze, M. C., Etringer, A., Oelke, C., McCreight, J., Barry, R. G., Gilichinsky, D., Yang, D., Ye, H., Ling, F., and Chudinova, S.: Spatial and temporal variability in active layer thickness over the Russian Arctic drainage basin, J. Geophys. Res.-Atmos., 110, 1–14, https://doi.org/10.1029/2004JD005642, 2005.
Zhang, Y., Wolfe, S. A., Morse, P. D., Olthof, I., and Fraser, R. H.: Spatiotemporal impacts of wildfire and climate warming on permafrost across a subarctic region, Canada, J. Geophys. Res.-Earth, 120, 2338–2356, https://doi.org/10.1002/2015JF003679, 2015.
Zhao, J., Wang, L., Hou, X., Li, G., Tian, Q., Chan, E., Ciais, P., Yu, Q., and Yue, C.: Fire Regime Impacts on Postfire Diurnal Land Surface Temperature Change Over North American Boreal Forest, J. Geophys. Res.-Atmos., 126, e2021JD035589, https://doi.org/10.1029/2021JD035589, 2021.
Zheng, B., Ciais, P., Chevallier, F., Yang, H., Canadell, J. G., Chen, Y., van der Velde, I. R., Aben, I., Chuvieco, E., Davis, S. J., Deeter, M., Hong, C., Kong, Y., Li, H., Li, H., Lin, X., He, K., and Zhang, Q.: Record-high CO2 emissions from boreal fires in 2021, Science, 379, 912–917, https://doi.org/10.1126/science.ade0805, 2023.
Zorigt, M., Myagmar, K., Orkhonselenge, A., van Beek, E., Kwadijk, J., Tsogtbayar, J., Yamkhin, J., and Dechinlkhundev, D.: Modeling permafrost distribution over the river basins of Mongolia using remote sensing and analytical approaches, Environ. Earth Sci., 79, 308, https://doi.org/10.1007/s12665-020-09055-7, 2020.