the Creative Commons Attribution 4.0 License.
the Creative Commons Attribution 4.0 License.
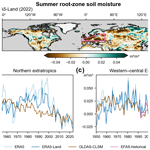
Detecting the human fingerprint in the summer 2022 western–central European soil drought
Dominik L. Schumacher
Mariam Zachariah
Friederike Otto
Clair Barnes
Sjoukje Philip
Sarah Kew
Maja Vahlberg
Roop Singh
Dorothy Heinrich
Julie Arrighi
Maarten van Aalst
Mathias Hauser
Martin Hirschi
Verena Bessenbacher
Lukas Gudmundsson
Hiroko K. Beaudoing
Matthew Rodell
Wenchang Yang
Gabriel A. Vecchi
Luke J. Harrington
Flavio Lehner
Gianpaolo Balsamo
Sonia I. Seneviratne
In the 2022 summer, western–central Europe and several other regions in the northern extratropics experienced substantial soil moisture deficits in the wake of precipitation shortages and elevated temperatures. Much of Europe has not witnessed a more severe soil drought since at least the mid-20th century, raising the question whether this is a manifestation of our warming climate. Here, we employ a well-established statistical approach to attribute the low 2022 summer soil moisture to human-induced climate change using observation-driven soil moisture estimates and climate models. We find that in western–central Europe, a June–August root zone soil moisture drought such as in 2022 is expected to occur once in 20 years in the present climate but would have occurred only about once per century during preindustrial times. The entire northern extratropics show an even stronger global warming imprint with a 20-fold soil drought probability increase or higher, but we note that the underlying uncertainty is large. Reasons are manifold but include the lack of direct soil moisture observations at the required spatiotemporal scales, the limitations of remotely sensed estimates, and the resulting need to simulate soil moisture with land surface models driven by meteorological data. Nevertheless, observation-based products indicate long-term declining summer soil moisture for both regions, and this tendency is likely fueled by regional warming, while no clear trends emerge for precipitation. Finally, our climate model analysis suggests that under 2 ∘C global warming, 2022-like soil drought conditions would become twice as likely for western–central Europe compared to today and would take place nearly every year across the northern extratropics.
- Article
(2222 KB) - Full-text XML
-
Supplement
(3407 KB) - BibTeX
- EndNote
Following a dry spring with above-average air temperatures across much of Europe (Toreti et al., 2022), the 2022 summer was assessed as the “hottest on record” by the European Union's Copernicus Environmental Programme. The unusually hot and dry conditions were accompanied by widespread soil desiccation, particularly in western regions of the continent (Copernicus, 2022a) that experienced a sequence of heat waves (Zachariah et al., 2022) and precipitation shortages. Based on runoff anomalies, it was highlighted in the press that the 2022 European drought could be the “worst in 500 years” (Henley, 2022). This event was preceded by the 2018–2020 drought in Europe (e.g., Boergens et al., 2020; Rakovec et al., 2022), and while 2021 brought relief to dry soils through above-normal precipitation in western parts of the continent (Copernicus, 2021), soil moisture drought indicators point to an incomplete recovery in many areas (NASA GRACE-FO, 2022; EDO, 2022). As such, at least part of Europe was already primed for a severe soil drought in 2022 well before summertime. Unusual heat and drought also characterized the 2022 boreal summer elsewhere, however; for example, China was affected by exceptionally high aridity and temperatures (Ahmedzade et al., 2022), and North America experienced a warm summer with below-average soil moisture (Copernicus, 2022a). In the midlatitudes, extreme summer heat and precipitation shortages are typically fostered by persistent, often near-stationary anticyclones (e.g., Li et al., 2020) or in some cases subtropical ridges (e.g., Sousa et al., 2020), and many areas in Europe were indeed subject to the strongest 500 hPa geopotential height anomalies between May and July 2022 since 1950 (Toreti et al., 2022). Such anticyclonic circulation patterns are intrinsically related to the extratropical jet stream, which is known to simultaneously promote drought and heavy precipitation in different regions for certain (wavy) flow configurations (Lau and Kim, 2012; Coumou et al., 2014), but the underlying dynamics are complex and still not fully understood. Nevertheless, a recent study has suggested that many heat waves in the ongoing century in western Europe have been caused by increasingly frequent and persistent double jets, whose occurrence is closely linked to anticyclonic flow (Rousi et al., 2022). From a global perspective, El Niño–Southern Oscillation (ENSO) has remained in the “La Niña” phase since late 2020 (CPC, 2022), which may have contributed to the hot and dry conditions in parts of both China and North America (Wang et al., 2007; Karori et al., 2013).
While the roles of these and other local and remote dynamic and thermodynamic drivers for the dry and hot 2022 summer are yet to be investigated in detail, it is already clear that the soils in large parts of the northern extratropics were unusually dry. As such, enhanced land–atmosphere coupling (e.g., Seneviratne et al., 2006; Mueller and Seneviratne, 2012; Miralles et al., 2019; Stegehuis et al., 2021) likely contributed to heat waves in Europe, China, and the southwestern United States (Pratt, 2022), and triggered hot and dry summer conditions in large parts of the northern extratropics. On the other hand, the high temperatures likely exacerbated dry soil conditions due to increased land evapotranspiration, as identified in recent drought events in Europe (e.g., Seneviratne et al., 2012; Teuling et al., 2013). This is in line with a detected trend towards decreased water availability in the dry season across land regions in the recent past, 1985–2014, compared to the first half of the 20th century (Padrón et al., 2020). Furthermore, the mechanism of northward “drought propagation” – a causal link between (spring) drought in the Mediterranean, and hot and dry summers in western–central Europe (Vautard et al., 2005; Zampieri et al., 2009) – may also have played a role in the evolution of the 2022 European drought. The extreme conditions manifested in some of the most severe soil moisture droughts on record, e.g., in July 2022, when nearly half of Europe was assigned a drought warning (EC JRC, 2022a). In some areas, shortages of drinkable water due to low water tables were reported, whereas China issued its first nationwide drought alert (Reuters, 2022). In addition, the combination of excessive heat and moisture deficits strongly increased the fire risk in Europe, leading to the highest burned area ever recorded since the start of measurements (EFFIS, 2022).
Low soil moisture typically implies increased water stress for natural vegetation and crops (e.g., Berg and Sheffield, 2018; Liu et al., 2020), which can be further exacerbated by elevated air temperatures and hence heat stress (Seneviratne et al., 2021). According to the 6th Assessment Report from the Intergovernmental Panel on Climate Change (IPCC), there is “medium confidence” that human-induced climate change has contributed to increases in agricultural and ecological droughts in some regions due to evapotranspiration increases (Seneviratne et al., 2021). Nevertheless, while strong evidence for human-induced aggravations of recent heat waves has been reported repeatedly (Seneviratne et al., 2021), such as for the heat wave in western Europe in July 2022 (Zachariah et al., 2022), there are more uncertainties in the contribution of anthropogenic climate change to trends in agricultural drought conditions in single regions. Building on a rapid attribution analysis of the World Weather Attribution (Schumacher et al., 2022), we investigate the role of climate change in the frequency and magnitude of 2022 surface and root zone soil moisture deficits – the latter a measure of agro-ecological drought – for two regions: the western–central Europe (WCE) region in IPCC AR6 (Iturbide et al., 2020), and the northern extratropics, i.e. the land area between 23.5 and 90∘ N (NHET). We restrict our analysis to boreal summer (June–August), the season with the largest spatial extent of droughts in the northern extratropics (Lu et al., 2019), which is also when the widespread 2022 drought conditions peaked. As temperature and precipitation anomalies are known to strongly influence agricultural drought, we also analyze summertime mean temperature and precipitation over the same regions as for soil moisture.
Several regions across the northern extratropics suffered from persistent drought and heat waves in the 2022 summer. Parts of southwestern North America, for example, were reported to experience their driest period in more than 1200 years, causing three water reservoirs in northern Mexico to drain and leading to water insecurity for 5 million residents (Linthicum, 2022). China, and particularly Hunan Province, experienced its longest drought and most severe heat wave on record (CMA, 2022; Ahmedzade et al., 2022; Le Page, 2022). As of 10 August 2022, nearly two-thirds of Europe was affected by drought (Seabrook, 2022). We focus on western–central Europe in the following and explore associated impacts in the context of vulnerability and exposure, since such extreme dry and hot conditions are known to act as a risk multiplier for energy, environmental, and socio-economic vulnerability (Rakovec et al., 2022; Gazol and Camarero, 2022; Naumann et al., 2021).
Since the beginning of May to mid-September, five back-to-back heat waves blanketed large swathes of Europe. Throughout these months, several daily and monthly maximum temperature records were broken across Italy, France, Switzerland, Germany, Poland, Hungary, and Slovenia (see, e.g., Phys.org, 2022; Breteau, 2022; Le News, 2022; Wang, 2022; Twoja Pogoda, 2022; OMSZ, 2022; BBC Weather, 2022). It is estimated that the persistent heat has led to over 24 000 fatalities across Europe, more than 18 000 of which were within western–central Europe – 11 000 in France and over 8000 in Germany alone (Roucaute, 2022; Destatis, 2022a, b). Infrastructure was also impacted as the heat melted roads, buckled railway lines, halted public transportation services, and increased the electricity demand while power stations operated at reduced capacity (Dhanesha and Jones, 2022; Binnie and Twidale, 2022; Rocha, 2022). The hot and dry conditions were also associated with a spike in wildfires; by 24 September, more than 770 000 ha of land had burned throughout the European Union (EU; EFFIS, 2022), which equals nearly 3 times the EU average over 2006–2021 (Copernicus, 2022b). Italy, Slovenia, France, and Romania were particularly affected by these fires (Roscoe, 2022; Lukov, 2022; Korosec, 2022; Dumitrescu, 2022), and by late June, Italy had surpassed its historical wildfire average 3-fold (The Local, 2022).
Europe's prolonged hot and dry weather conditions during the first half of 2022 and ensuing low water reservoir levels led to significant reductions in summer crop yields, most significantly in France, northern Italy, Germany, Slovenia, Hungary, and Romania (EC JRC, 2022b). These significant agricultural impacts are unsurprising, given that this sector is the most water-intensive industry in the region (Heggie, 2020; EEA, 2020a). Compared to their 5-year averages, maize, soybean, and sunflower crops suffered 16, 15, and 12 percent decreases, respectively (Toreti et al., 2022). For example, in northern Italy, the Po River basin experienced its worst water crisis in approximately 70 years, leading to an estimated 30 % reduction in rice crop yields and at least 50 cattle deaths (Clifford, 2022; Coldiretti, 2022). Paired with the Ukraine crisis hiking up the price for fertilizers 4-fold, these decreases in agricultural production led to a “heatflation” of food prices as well as higher feed prices for livestock (DW, 2022; Mendes, 2022). Food prices in China, especially for fruits and vegetables, were also driven up by the heat and drought (Bradsher and Dong, 2022). We note that crop loss poses increasing threats to food security not only in the affected regions but also globally, and is hence it is one of the major impacts of the 2022 drought.
The drought in Europe also had indirect impacts on electricity generation in several European countries (Horowitz, 2022). Lower river flows and thus lower reservoir levels have significantly decreased hydroelectric power generation; for example, in Italy, hydroelectric plants generated 40 %–50 % less power over the summer months, and one plant in Piacenza was temporarily shut down (Good et al., 2022). The low water levels in rivers in Germany also reduced the ability to transport coal by boat, further impacting energy supply (Horowitz, 2022). In France, where nuclear energy provides a clear majority of the electricity, decreased water availability and the associated lack of cooling mandated output reductions and complete shutdowns of nuclear reactors on the Belgium–France border (Kollewe, 2022). These supply constraints coincided with high demand, particularly due to air conditioning during hot periods. We also emphasize here that the drought occurred at a time when Europe was facing a number of other, compounding stressors on its energy supply; the COVID-19 pandemic led to a slow-down in demand for energy in 2020, but demand had rebounded by 2022 while supply had not kept up, leading to an increase in global energy prices. In addition, the war in Ukraine strained ties between Europe and Russia, until recently the main supplier of Europe's natural gas. The restricted supply sent prices soaring, with different impacts across European countries based on their energy mix and import capacity from alternative routes. In Germany, for instance, the energy crisis had far-reaching economic ramifications affecting small and medium-sized enterprises, the backbone of Germany's economy (Kagerl et al., 2022).
The drought also highlighted the vulnerability of Europe's water infrastructure; roughly 66 % of the European population relies on groundwater for its water-related needs, about 60 % are residing in cities where groundwater is over-exploited (EEA, 2020b), and water wastage in public supply systems is estimated at 20 %–40 % of the available water for the entire EU and up to 80 % in individual cities (EEA 2020b; Hirschnitz-Garbers et al., 2016). In response to the 2022 drought, and to effectively conserve water, multiple countries enforced water protection practices; e.g., cities and districts across Germany prohibited extraction from various bodies of water, as well as filling pools, watering lawns, and cleaning cars (Stresing and Wolf, 2022). By early August 2022, over 100 French municipalities relied on water deliveries by truck to overcome potable tap water shortages (Chadwick, 2022), and 62 of 96 of France's departments were at the highest level of drought alert, many of which implemented water restrictions (Al Jazeera, 2022). While the country experienced its driest July in more than half a decade (BBC, 2022) and its reportedly most extreme drought in history (Breeden, 2022), the effects of the below-average rainfall were likely aggravated by unsustainable water use and losses in the water distribution system.
We also point out that until recently drought risk management at the pan-European scale has predominantly focused on coping with financial losses, mainly through calamity funds, mutual funds, and insurance (Bielza Diaz-Caneja et al., 2009). This opposes the current scientific consensus, which entails a shift from reactive to proactive risk management strategies (Wilhite et al., 2007; Blauhut et al., 2016). Blauhut et al. (2022) note that drought risk management planning does not exist on a unified continental scale in Europe, despite the potential benefits for large-scale directive planning in reducing emergency response costs. Following a comprehensive review of drought management practices in 28 European countries and surveying 712 experts across Europe, the paper recommends some key areas to reduce vulnerability and exposure from the planning perspective. Its recommendations include developing a pan-European approach to drought management, allowing for country contextualization while also supporting cross-border drought preparedness efforts.
To summarize, the 2022 heat and drought in western–central Europe had far-reaching impacts on a variety of sectors including health, energy, agriculture, and municipal water supply, reflecting the need to reassess drought preparedness and deal with trade-offs in water management. It came at a time when its impacts were interacting with non-climate risks to create compounding and cascading impacts. For example, impacts on power generation due to heat and drought (on hydropower, nuclear, and coal power plants) coincided with increasing energy prices linked to the conflict in Ukraine. Similarly, impacts on agricultural yields in Europe coincided with strained global food supply due to reduced exports from Russia and Ukraine, as well as high fertilizer prices with knock-on effects on inflation in Europe but also on global food prices and therefore food insecurity, resulting in risks cascading across sectors and regions (as flagged as a rising risk in IPCC AR6 WGII; IPCC, 2022). Overall, this event serves as a strong motivation to strive for proactive drought risk management strategies, and developing and coordinating such strategies across the continent is considered an effective approach to improve drought preparedness and resilience.
We examine trends in root zone soil moisture over the two selected regions – WCE and NHET – for quantifying the role of climate change in the widespread 2022 drought conditions that impacted large parts of the northern extratropics, and in particular the European continent. We also compare the results for these variables and regions with the respective estimates based on precipitation and temperature to gain insights into how the soil moisture drought has been influenced by the accompanying precipitation deficits and anomalously high temperatures.
3.1 Observational data
3.1.1 Main datasets
To analyze the drought event, we rely on a mixture of reanalysis and observation-based data. The employed statistical approach (Sect. 3.3), mandates (i) continuous data, ideally since preindustrial times but at least from 1950 onwards (van Oldenborgh et al., 2021), and (ii) data coverage at least until August 2022 to infer the probability – or return period – of the 2022 summer soil drought. Many available soil moisture observations do not meet these criteria; hence, we perform our main analysis for 1950–2022 and with soil moisture estimates derived from land surface models ingesting reanalyzed or observed meteorological data, a common approach for assessing soil moisture trends (e.g., Albergel et al., 2013; Bi et al., 2016; Cheng and Huang, 2016; Deng et al., 2020; Qiao et al., 2021; Almendra-Martín et al., 2022). These datasets are introduced below along with additional meteorological variables used for analysis.
ERA5
The ERA5 reanalysis product by the European Centre for Medium-Range Weather Forecasts (ECMWF) contains simulated estimates of climate variables for the period 1950–present, at 0.25 km × 0.25 km resolution and at hourly intervals (Hersbach et al., 2020). ERA5 uses the ECMWF assimilation system IFS (IFS Cycle 41R2), and simulates land surface processes with the Hydrology Tiled ECMWF Scheme for Surface Exchanges over Land (H-TESSEL) model. For the production of ERA5, more than 200 satellite instruments and conventional meteorological data types are assimilated, including scatterometer soil moisture, rain gauge–radar composites (but only for the contiguous United States of America), and in situ measurements of 2 m temperature and humidity. We use the data of four variables: precipitation, temperature and volumetric soil moisture at surface (0–7 cm), and root zone levels (0–100 cm). ERA5 soil moisture is computed for four vertical soil layers extending down to 289 cm, of which the first three are aggregated here to the root zone soil moisture. Due to unrealistic values in Greenland, especially noticeable prior to ∼ 1970, we mask the affected area prior to calculating the regional mean of the northern extratropics. For other datasets without problematic soil moisture values in Greenland, including or masking the latter results in nearly identical time series as Greenland (about 2×106 km2) only accounts for a small fraction of the entire northern extratropical land area (on the order of 80×106 km2).
Together with the other main soil moisture datasets (and additionally EFAS-historical for western–central Europe), we use ERA5 data for our comparison of summertime root zone soil moisture. However, we refrain from employing ERA5 root zone soil moisture for the statistical analysis because ERA5 has been produced using several production streams (see Table 3 in Hersbach et al., 2020), employing a spin-up period of 1 year for merging the different simulations. This is known to cause discontinuities in the deep soil and manifests in visible jumps in NHET root zone soil moisture (not shown). Even though the effects on WCE root zone soil moisture are not as obvious, we exclude ERA5 root zone soil moisture for both of our domains and rely on ERA5-Land instead. Surface soil moisture is not affected, as its memory timescale is on the order of days to weeks (McColl et al., 2019) and is thus included in the additional surface soil moisture attribution analysis.
ERA5-Land
ERA5-Land is an offline 0.1∘ × 0.1∘ land surface model simulation that ingests ERA5 precipitation and altitude-corrected air temperature, humidity, and pressure to match the higher-resolution land grid (Muñoz-Sabater et al., 2021). The simulations are performed with the land surface model H-TESSEL that is also used to produce ERA5, yet the nonlinear downscaling enables a more realistic simulation of the hydrological cycle in ERA5-Land. We also note that ERA5-Land has been produced with only three production streams and that these streams are merged with a spin-up period of 3 years (rather than 1 year as for ERA5). While minor discontinuities are still evident in the deepest layer of ERA5-Land at 100–289 cm (not shown), the root zone as defined in this study (0–100 cm) is not affected. As such, ERA5-Land does not feature the same collation issues as ERA5 (see above) and is thus more suitable for our attribution study. In addition, restricting the analysis to ERA5-Land and GLDAS-CLSM prevents an overrepresentation of ECMWF products, since ERA5 and ERA5-Land are (by design) closely related.
GLDAS-CLSM
We also employ the NASA Global Land Data Assimilation System Catchment Land Surface Model (GLDAS-CLSM; Rodell et al., 2004; Li et al., 2019). Initialized using the soil moisture and spatial fields from the land surface model climatology for 1 January 1948, the simulations are forced by the global meteorological forcing data from Princeton University (Sheffield et al., 2006) and (after 2003) by ECMWF IFS analysis fields. Run within the Land Information System (LIS; Kumar et al., 2016) framework, this model simulates water storage in the full soil profile at 0.25∘ × 0.25∘ resolution, from which surface (0–2 cm) and root zone (0–100 cm) soil moisture and groundwater can be derived. Outputs are available from 1948 to the present. Beginning in 2003, the model assimilates GRACE/GRACE-FO terrestrial water storage anomaly data from the University of Texas (Save et al., 2016; Save, 2020). The 2003–present data are scaled to the open loop, using scaling factors determined for each grid cell and with a 7 d moving window such that the mean and standard deviation of soil moisture obtained with GRACE data assimilation across 2003–2012 matches the Princeton-forced (1948–2012) climatology in the same period (Houborg et al., 2012). For some grid cells in high latitudes, this results in negative and hence not physically meaningful values, which we remove for our analysis of the northern extratropics.
E-OBS
For the WCE region, we additionally analyze E-OBS (version 25.0e). The E-OBS dataset is a 0.25∘ × 0.25∘ gridded temperature and precipitation dataset for Europe, formed from the interpolation of station-derived meteorological observations (Cornes et al., 2018). E-OBS was used to produce seasonal cycles and climatology and for trend analysis of precipitation and temperature over Europe.
GISTEMP
Finally, as a measure of anthropogenic climate change, we use the global mean surface temperature (GMST) from the National Aeronautics and Space Administration (NASA) Goddard Institute for Space Science (GISS) surface temperature analysis (GISTEMP, Hansen et al., 2010; Lenssen et al., 2019). GMST is represented by anomalies with respect to 1951–1980 and is low-pass filtered with a 4-year running mean prior to analysis.
3.1.2 Supplementary datasets
We emphasize that the soil moisture in reanalyses and land surface model simulations is a derived variable affected both by model formulation and the quality of meteorological forcing (and, in the case of ERA5, the assimilated surface soil moisture data). Compared to meteorological variables such as temperature or precipitation with far better in situ coverage, soil moisture estimates are hence associated with considerable uncertainty. In this context, it is worth noting that in recent decades and particularly in the ongoing millennium, the progressive deployment of satellites and development of more capable sensors has ushered in an era of remote-sensed surface soil moisture estimates. However, microwave remote-sensed soil moisture products typically feature data gaps due to incomplete satellite coverage and radio frequency interference and environmental conditions that prevent the measurement of soil water content such as dense canopies, frozen soil, or snow cover (Llamas et al., 2020; Bessenbacher et al., 2022; Liu et al., 2023). Nevertheless, satellite-based soil moisture estimates can – if adequately gap-filled – provide a valuable alternative perspective for assessing recent large-scale surface soil moisture changes (Bessenbacher et al., 2023). The advances in remote sensing are complemented by the development of increasingly capable approaches in the field of artificial intelligence to extract the most out of the available data, enabling additional lines of evidence. We thus expand our analysis by using data obtained with various observation-driven approaches listed below, including soil moisture estimated by comparatively simple process-based models or neural networks instead of land surface models, ingesting both in situ and remote-sensed measurements.
EFAS-historical
The European Drought Observatory provides information on the current status of drought in Europe, including a soil moisture index (SMI) and SMI anomalies based on the European Flood Awareness System (EFAS). The latter is a hydrological forecasting and monitoring system from the European Commission and the ECMWF, ingesting a range of meteorological forecasts at medium to seasonal timescales as well as observations. The underlying hydrological model is LISFLOOD (EC JRC, 2020), a hydrological rainfall–runoff model, and we employ EFAS-historical simulations (Mazetti et al., 2020) forced with meteorological observations and available every 6 h at 5 km × 5 km for Europe since 1991. Soil moisture is provided at three soil levels (superficial, upper and lower soil), yet these depths vary for each grid cell and are not provided. While soil evaporation is restricted to the superficial layer, plant roots can extract moisture from both the superficial and upper soil layer for transpiration. As the lack of layer depth information prevents vertical aggregation, we rely on the upper soil as a proxy for root zone soil moisture in our study, whereas the superficial layer represents the surface soil moisture.
SoMo.ml
Generated with a long short-term memory neural network ingesting in situ measurements and ERA5 meteorological forcing, SoMo.ml provides global daily soil moisture data from 2000 to 2019 at 0.25∘ × 0.25∘ horizontal resolution (O. and Orth, 2021). Since the in situ soil moisture data collected across more than 1000 sites is based on several sensor types and different calibrations, the creators of SoMo.ml employ ERA5 soil moisture to scale the point-scale measurements. In essence, the mean and standard deviation of soil moisture is inferred from ERA5, whereas point-level in situ data represent the temporal dynamics. With this approach, the machine learning model can be trained around the globe to estimate soil moisture at the grid scale rather than only for individual sites. The resulting data are provided at three depths (0–10 cm, 10–30 cm and 30–50 cm), and we use the uppermost layer as an indicator of surface soil moisture. We note that the performance of this dataset depends on in situ data availability and is hence limited in sparsely monitored areas such as the tropics.
RSSSM
The remote-sensing-based surface soil moisture (RSSSM, Chen et al., 2020) combines 11 high-quality microwave products with a neural network approach, resulting in gap-free, global data. This dataset has been validated against in situ measurements and is provided at 0.1∘ × 0.1∘ every 10 d from 2003–2018.
ESA-CCI gap-filled
The European Space Agency (ESA) Climate Change Initiative (CCI) soil moisture products are currently (v07.1) based on either 5 active, 12 passive, or a blend of all (microwave) sensors and provide remote-sensed surface soil moisture estimates since 1978 (Gruber et al., 2019; Preimesberger et al., 2021). In these products and due to the difficulties outlined above, however, less than half of all global land data points are observed in the years 2003–2020 (Bessenbacher et al., 2023). In addition, trend analyses are complicated by the fact that the sensor coverage is not constant in time and used to be fairly limited; e.g., a majority of the northern extratropics is only covered since 2007 (Dorigo et al., 2017). To address these problems, a gap-filled soil moisture product is currently being developed by ESA-CCI, building on the combined product (blended active + passive) and the application of the DCT-PLS smoothing algorithm (Garcia, 2010) for the gap filling. In addition, GLDAS-Noah v2.1 surface soil temperature data are used for the detection of frozen soil conditions, in which case soil moisture values are gap-filled by a temporal linear interpolation. The gap-filled daily product at 0.25∘ × 0.25∘ is currently available for 2000–2021.
ESA-CCI gap-filled with the multivariate CLIMFILL approach
The recently developed CLIMFILL is a multivariate gap-filling framework (Bessenbacher et al., 2022) that exploits the spatial, temporal, and cross-variable dependence structure of Earth system observations. It has been used to gap-fill a wide range of observations including surface temperature, precipitation, and ESA CCI surface soil moisture on monthly grids from 1995–2020 and at 0.5∘ × 0.5∘ spatial resolution (Bessenbacher et al., 2023). Within this dataset, gaps in surface soil moisture are filled by taking into account information acquired through spatial interpolation of the monthly maps, temporal lagged effects like soil moisture memory, and observed values of related variables at the land surface, for example temperature and precipitation. Bessenbacher et al. (2023) have demonstrated that this approach fills gaps in the data more accurately than univariate interpolation that cannot take into account information from other observed variables.
3.2 Model and experiment descriptions
We use several climate modeling experiments in this study, building on three multi-model ensembles of different model types (Philip et al., 2020a): coupled global circulation models (GCMs), high-resolution models, and GCMs driven by sea surface temperature (SST). All models are evaluated, and the simulations of models that pass the required checks (Sect. 3.4) are combined into a single multi-model ensemble that is subsequently treated under the same framing.
The first set of models used in this analysis comes from the CMIP6 experiment (Eyring et al., 2016). For all simulations, the period 1850–2014 is based on historical coupled simulations, while the SSP5-8.5 scenario is used for the remainder of the 21st century. Models are excluded if they do not provide all relevant variables, do not cover 1850–2100, or include duplicate or missing time steps. The first available ensemble member is used for each model.
The second set of models used in the analysis include the AM2.5C360 (Yang et al., 2021; Chan et al., 2021) and the FLOR (Vecchi et al., 2014) high-resolution climate models developed at Geophysical Fluid Dynamics Laboratory (GFDL). The AM2.5C360 is an GCM based on the same atmosphere as used for the FLOR mode (Delworth et al., 2012; Vecchi et al., 2014) with a horizontal resolution of 25 km. A total of 10 ensemble simulations of the Atmospheric Model Intercomparison Project (AMIP) experiment (1871–2021) are analyzed. These simulations are initialized from 10 different preindustrial conditions but forced by the same SSTs from HadISST1 (Rayner et al., 2003) after group-wise adjustments (Chan et al., 2021), as well as the same historical radiative forcings. The FLOR model, on the other hand, is an atmosphere–ocean coupled GCM with a resolution of 50 km for land and atmosphere and 1∘ for ocean and ice. A total of 10 ensemble simulations from FLOR are analyzed, which cover the period from 1860–2100 and include both the historical and RCP4.5 experiments driven by transient radiative forcings from CMIP5 (Taylor et al., 2012).
The third ensemble considered in this study is the HighResMIP SST-forced model ensemble (Haarsma et al., 2016), the simulations for which span from 1950–2050. The SST and sea ice forcings for the period 1950–2014 are obtained from the 0.25∘ × 0.25∘ Hadley Centre Global Sea Ice and Sea Surface Temperature dataset that have undergone area-weighted regridding to match the climate model resolution. For the “future” time period (2015–2050), SST and sea ice data are derived from RCP8.5 (CMIP5) data and combined with greenhouse gas forcings from SSP5-8.5 (CMIP6) simulations (see Sect. 3.3 of Haarsma et al., 2016, for further details). It is worth noting that this ensemble only has outputs for moisture in the upper portion of the soil column (i.e., the upper 10 cm of the soil layer) but not moisture in the total soil column; therefore, it is not considered in the analysis of root zone soil moisture.
3.3 Statistical methods
In this study we analyze summer (June–August) mean time series of soil moisture, precipitation, and temperature, averaged over both western–central Europe and the northern extratropics, as defined in Sect. 1. Methods for observational and model analysis and for model evaluation and synthesis are used according to the World Weather Attribution Protocol, described in Philip et al. (2020a), with supporting details found in van Oldenborgh et al. (2021) and Ciavarella et al. (2021). The essence of the approach we employ here is that event indices – regional summertime averages of soil moisture, precipitation, and temperature – are represented with continuous probability distributions conditional on GMST, which enables us to estimate how the intensity (event magnitude) and probability of occurrence have changed under human-induced climate change. We characterize the 2022 summer drought by first determining the return time of the event with the observation-based products and then querying the model distributions at the corresponding return level. The analysis steps include (i) trend calculation from observations, (ii) model validation, (iii) multi-method multi-model attribution, and (iv) synthesis of the attribution statement. We note that, regardless of the underlying emission scenario, model data from 1850–2022 and from 1850–2050 are used to conduct the present-vs.-past and future-vs.-present climate analyses, but these time periods only indicate the amount of data used to fit the statistical model and hence infer the relationship between event indices and GMSTs. We then rely on global warming levels to calculate the return periods, the probability ratio (PR – the factor-change in the event's probability) and change in intensity of the drought event. For our comparison of the present (2022) to the past (1850–1900) climate, the GMST changes with respect to the present amount to −1.2 ∘C according to the Global Warming Index (https://www.globalwarmingindex.org, last access: 12 February 2024), and for comparing additional changes in the future to the present, we use +0.8 ∘C relative to the 2022 GMSTs (+2.0 ∘C with respect to preindustrial conditions). In other words, we are conditioning the analysis on observed and simulated global warming levels and not on specific time frames. As such, it does not matter when the future warming is reached in any given model simulation, which allows us to combine models with different emission scenarios and still perform a consistent analysis.
To statistically model the event, we approximate the variable of interest – e.g., soil moisture – by a Gaussian distribution that incorporates a dependency on global warming. For soil moisture and precipitation, we model the mean and scale parameters as exponential functions of GMST (for details see Kew et al., 2021), whereas for temperature, the mean parameter depends linearly on GMST (details in Philip et al., 2020b), which is in line with other research (Wartenburger et al., 2017; Seneviratne and Hauser, 2020). As such, we use a Gaussian distribution that scales (soil moisture, precipitation) or shifts (temperature) with GMST, and note that all climate variables of interest are reasonably Gaussian distributed, as one would expect when examining large regions and seasonal averages (e.g., Schär et al., 2004; Wang et al., 2019). Where applicable (see Sect. 3.3), multiple initial condition ensemble members are pooled together for the statistical evaluation and analysis (e.g., the 10-member AMIP AM2.5C360 ensemble). Finally, results from observations and models that pass the validation tests are synthesized into a single attribution statement.
To facilitate comparisons between different models and the observation-driven products, all soil moisture data were scaled prior to the statistical analysis by dividing through the respective 1950–2022 June–August standard deviation. All statistical analyses were performed with the Climate Explorer or using xarray and scipy in python.
3.4 Model evaluation
Because observation-driven soil moisture products feature large uncertainties owing to the different land surface models employed and their inherent deficiencies (Gevaert et al., 2018), as well as the limitations of remote sensing particularly for the root zone soil moisture (Babaeian et al., 2019), we rely on precipitation and temperature as proxies for moisture supply and demand in our model evaluation. Rather than directly evaluating the statistical parameters for soil moisture, we require all models to pass validation for the respective domain (WCE, NHET) for both precipitation and temperature and the 1950–2022 period. For these variables, we assess the models' fitness for purpose in three ways. First, we qualitatively compare the seasonal cycles in models to observations, checking for the timing and relative amplitudes of peaks and troughs. Second, we compare the spatial pattern of mean summer temperatures for both regions. Third, we check if the parameters of the fitted statistical distribution (Gaussian shifting with GMST for temperature, Gaussian scaling with GMST for precipitation) in models are compatible with those from observation-based estimates. For the observational parameter range, wherever applicable, all of the respective listed observation-based datasets are considered. Models whose statistical parameter range lies within the observational range (95 % confidence interval) are considered “good”, whereas overlapping ranges are “reasonable”. Additionally, wherever available, the seasonal cycle and spatial pattern of soil moisture were also evaluated against ERA5-Land estimates – these were typically found to be “reasonable” in the models that passed the combined precipitation and temperature validation. Supplement Tables S1 and S2 show the model evaluation results for the root zone soil moisture in the WCE and NHET region, respectively, whereas Tables S3 and S4 present the results for the same regions and surface soil moisture. Only models with an overall performance of “reasonable” or better were used for the attribution analysis. Based on the capability of the model to capture the seasonal cycle, spatial pattern, and statistical properties for temperature and precipitation, a model must pass at least six checks, or eight for models with soil moisture available for evaluation, such as the CMIP6 models, each of which without a single “bad” performance.
3.5 Synthesis
All synthesis figures presented in this study show the changes in probability (a) and intensity (b) of the variable of interest (soil moisture, temperature, precipitation) for the observation-based products (blue) and models (red) and follow the standard analysis method employed by the World Weather Attribution (Philip et al., 2020a). To combine the two lines of evidence into a synthesized assessment, a representation error is first added (in quadrature) to the observations. The rationale behind this is that we consider observations as equally valid representations of a singular climate realization with the same underlying true natural (internally generated) variability. Therefore, the mean deviation of individual datasets to the overall mean best estimate indicates a representation error (of observations with respect to reality), shown in the synthesis figures as white boxes around the natural – that is, internally generated – variability (light blue bars). The dark blue bar shows the average over the observation-based products (black marker) and the total uncertainty (width of the bar) based on natural variability and representation errors. Instead of representation errors, next, a term to account for intermodel spread is added (in quadrature) to the natural variability of the models. Note that while this term is based on the scatter of model means (analogous to the representation error for observations), we interpret model simulations as independent climate realizations. Consequently, we only add this term granted that the differences between models cannot solely be explained by natural variability, which is the case here. The intermodel spread is shown in the synthesis figures as white boxes around the light red bars. The dark red bar surrounding the model average (black marker) is based on a weighted mean using the respective uncorrelated uncertainties due to natural variability plus intermodel spread. Specifically, the weights are given by the inverse sum of the squared model variability (i.e., the square of the light red bars) and the squared intermodel spread (i.e., the square of the white bars).
Observation-based products and models are combined into a single result in two ways. Firstly, we neglect common model uncertainties beyond the intermodel spread that is depicted by the model average, and compute the average of models and observations using the total respective uncertainties as weights (widths of dark red and blue bars). The resulting weighted average is indicated by the magenta bar. Due to common model uncertainties, the true model uncertainty can be larger than indicated by the intermodel spread. Therefore, we also show the more conservative estimate of an unweighted, direct average of observations (dark red bar) and models (dark blue bar) contributing 50 % each, indicated by the white box around the magenta bar in the synthesis figures. Note that so as to not distort the synthesis, we limit very high probability ratios to 10 000.
4.1 Comparing soil moisture across several datasets
The summer of 2022 featured root zone soil moisture deficits across much of the northern extratropics (Fig. 1a). We begin our analysis by examining regionally averaged July–August soil moisture for NHET (Fig. 1b), which is remarkably similar in the last 2 decades, with a consistent downward trend for all main datasets (described in Sect. 3.1.1). In the 20th century, the correspondence between different soil moisture estimates is clearly worse, and both ERA5 and ERA5-Land indicate an upward trend, whereas GLDAS-CLSM already features a downward trend. This disagreement is most likely a consequence of observation density generally increasing in time (e.g., Dorigo et al., 2015), and the limited availability of satellite data, especially prior to 1979 (e.g., Dorigo et al., 2012). Nevertheless, all datasets used here indicate that the summer of 2022 featured pronounced – yet not unprecedented – soil moisture deficits averaged across the northern extratropics. Zooming into western–central Europe (delineated in Fig. 1a), we find a good correspondence across all datasets except for the first few decades, providing strong evidence for declining root zone soil moisture since about 1980 (Fig. 1c). Such downward trends have also been noted in other studies (e.g., Trnka et al., 2015; Scherrer et al., 2022). Overall, the 2022 summer drought signal is stronger in western–central Europe than in the larger domain, with ERA5, ERA5-Land, and GLDAS-CLSM pointing to the driest regionally averaged root zone soil moisture since 1950. EFAS-historical, the hydrological forecasting and monitoring system used by the EDO and restricted to Europe, indicates that only the summer of 2015 was slightly drier than 2022, but is otherwise consistent with the main datasets.
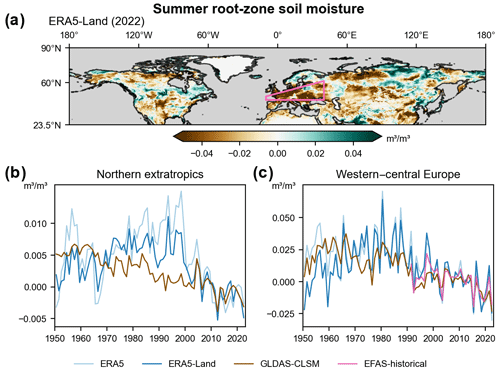
Figure 1(a) Mean summer (June–August) root zone soil moisture in 2022 over the northern extratropics (NHET), shown for the ERA5-Land dataset and expressed as anomalies with respect to 1950–2022. Western–central Europe (WCE) is highlighted by the pink contour. (b) Summer root zone soil moisture averaged over the northern extratropics for the main datasets used for analysis, with the 2003–2018 baseline subtracted to facilitate the comparison. (c) Like (b) but for western–central Europe. Note that the supplementary dataset EFAS-historical is also shown, but this product is only available for Europe and hence not used for (b), and that the second (“upper“) soil layer – which does not represent a fixed depth, unlike for the other datasets displayed here (1 m) – is selected to represent the root zone.
Since the root zone soil moisture can only be observed through elaborate, sparse, and highly heterogeneously distributed in situ measurements, we cannot rely on direct observations for our analysis. Surface soil moisture, on the other hand, can be sensed from space, although there are several caveats, such as dense vegetation resulting in canopy rather than soil water measurements, as well as limited spatiotemporal coverage, although the latter has been improving. Nonetheless, the main datasets feature largely similar root zone and surface soil moisture interannual variability and long-term changes. This is easiest observed when comparing the datasets without subtracting the baseline as done in Fig. 2, although the correspondence of soil moisture between the surface layer and root zone is lower in ERA5 than for GLDAS-CLSM and ERA5-Land. Nevertheless, the overall temporal evolution of summer soil moisture in the surface layer and root zone is consistent in both regions for all main datasets, which is plausible given that soil moisture near the surface and in deeper layers is inherently connected through infiltration and diffusion processes (e.g., Albergel et al., 2008). Considering that Berg et al. (2017) reported stronger surface drying than in deeper soil layer for CMIP5 projections, we also compare the historic long-term changes in surface and root zone soil moisture by representing the respective time series as percentage changes for both domains and GLDAS-CLSM and ERA5-Land (Fig. S1). While there is a stronger decrease in surface than root zone soil moisture for NHET based on GLDAS-CLSM, comparatively minor drying gradients between the surface and root zone emerge for NHET using ERA5-Land, and similarly for WCE with both datasets. Our findings do suggest that soil moisture decreased more near the surface than in deeper layers during the 1950–2022 period, yet the extent of this surface drying gradient remains unclear and might be negligibly small.
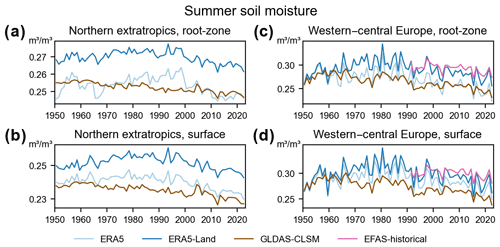
Figure 2Summer soil moisture averaged over the northern extratropics and western–central Europe for the same datasets as in Fig. 1 but for both the root zone (a, c) and surface layer (b, d) for the two domains. No baseline is subtracted here for a convenient comparison of individual products across the different soil depths.
Next, we extend our analysis by comparing surface soil moisture across a total of seven and eight products for the northern extratropics and western–central Europe, respectively, by adding several supplementary datasets that incorporate either microwave-sensed or in situ soil moisture measurements (see also Sect. 3.1.2). In ERA5-Land, the spatial pattern of soil moisture anomalies is fairly similar for the surface and the root zone (cf. Figs. 1a, 3a), and this also applies to the other main soil moisture datasets. For the mean surface soil moisture across the northern extratropics (Fig. 3b), almost all datasets agree on an overall decline in the last 2 decades. Only the satellite-based, gap-free RSSSM shows an upward trend for 2003–2018, as already noted by its creators (Chen et al., 2020). The downward trend is also observed for the gap-filled products from ESA-CCI and CLIMFILL, which show a remarkable correspondence even though the original, non-gap-filled soil moisture from ESA-CCI features upward trends for the northern extratropics, especially prior to 2008 (not shown). None of the supplementary datasets available for NHET cover the year 2022, but ESA-CCI and CLIMFILL do not seem to exhibit the clear decline evident for the ECMWF products and GLDAS-CLSM after 2017. Otherwise, both of these remote-sensed estimates show a particularly good agreement with GLDAS-CLSM, whereas SoMo.ml, a machine-learning based product relying on in situ soil moisture and ERA5 meteorological forcing, is largely consistent with ERA5 but portrays a slightly weaker downward tendency.
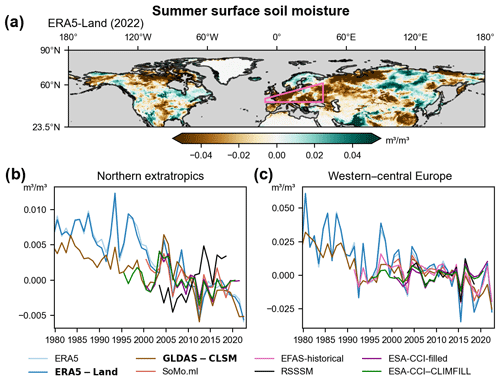
Figure 3(a) Summer average surface soil moisture in 2022 over the northern extratropics (NHET) shown for the ERA5-Land dataset and expressed as anomalies with respect to 1950–2022. Western–central Europe (WCE) is highlighted by the pink box. (b) Summer surface soil moisture averaged over the northern extratropics for several datasets, with the 2003–2018 baseline subtracted to facilitate the comparison. Panel (c) is like (b) but for western–central Europe. Note that for EFAS-historical, the first (“superficial“) soil layer – which does not represent a fixed depth, contrary to the other datasets shown here – is selected to represent the surface soil moisture. The two main datasets employed for both the root zone and surface soil moisture event attribution are highlighted in the legend (bold font).
While there seems to be some inconsistency in regard to long-term changes in NHET soil water content, we are not aware of any recent studies that have discussed positive northern hemispheric or global soil moisture trends. A tendency toward drying – especially for the surface and during summer – has been reported in several analyses (e.g., Sheffield and Wood, 2008 Cheng and Huang, 2016; Deng et al., 2020; Qiao et al., 2021). This increases our confidence in the two selected soil moisture datasets for further analyses, GLDAS-CLSM and ERA5-Land, as both feature downward trends for the surface and for the root zone soil moisture. We cannot reliably assess, however, whether these products are truly more accurate than, e.g., RSSSM, which features a recent surface soil moisture increase at the hemispheric or global scale.
For western–central Europe, on the other hand, none of the eight available surface soil moisture products indicates clear upward trends in the ongoing millennium, and the overall agreement between the different estimates is better than for the northern extratropics (Fig. 3c). We remark that, based on the last 2 decades, the remote-sensed products show smaller drying tendencies than the other datasets used here but also point out that the short available time period complicates such assessments. Moreover, this domain is much more observationally constrained than the entire northern extratropics, and in particular the high latitudes, and we hence deem the choice of soil moisture dataset for the attribution analysis less critical than for the larger domain.
Finally, we also briefly inspect the total water storage as measured by the Gravity Recovery and Climate Experiment (GRACE). These data are not only measured in a fundamentally different manner than remote-sensed surface soil moisture, but also represent the sum of all above- and below-surface water storages (e.g., canopy water, rivers and lakes, groundwater, and of course soil moisture) and hence typically serve as a proxy for groundwater drought. As such, the downward trends evident for both domains (Fig. S2), which is especially pronounced for western–central Europe, cannot directly validate long-term changes in soil moisture. Nevertheless, the observed drying tendency is fully consistent with the declining root zone soil moisture in the last 2 decades evident in Fig. 1. Overall, we conclude that the comparison to supplementary datasets strengthens our analysis but also emphasize that the observation-based attribution of the 2022 soil drought to human-induced climate change may be associated with more uncertainty than represented by GLDAS-CLSM and ERA5-Land alone, particularly for the northern extratropics.
4.2 Event return period and long-term trend analysis
In the next step, we investigate the probability of the 2022 soil drought as well as the anthropogenic fingerprint for both analysis regions. Whereas the previous section presented soil moisture as a function of time, here we explore the relationship between the warming climate and soil moisture.
4.2.1 Western–central Europe
We fit June–August root zone soil moisture averaged over the WCE region as a function of GMST, as described in Philip et al. (2020a), for ERA5-Land (Fig. 4a) and GLDAS-CLSM (Fig. 4b). The left-hand panels depict soil moisture as a function of the GMST anomaly, while the right-hand panels show the corresponding Gaussian-distribution-based return period curves in the present 2022 climate (red lines) and the past 1.2 ∘C cooler climate (blue lines). The return periods are 12 and 23 years according to ERA5-Land and GLDAS-CLSM, respectively. We average and round this to a return period of 20 years for the remainder of the analysis. We obtain probability ratios well above 1 (95 % confidence interval of 4 to 450) for ERA5-Land, and estimates based on GLDAS-CLSM are several orders of magnitude higher with a lower bound of 53 000, suggesting an even stronger warming signal (Table S5). We also estimate the mean change in WCE summer root zone soil moisture from the past to the present climate, which yields intensity changes with best estimates (confidence intervals) of −9 % (−13 % to −4 %) for ERA5-Land and −14 % (−16 % to −11 %) for GLDAS-CLSM. Despite the apparent mismatch of the probability ratios, there is an overlap in confidence intervals of mean intensity changes. The latter are less sensitive than the probability ratios to the inferred relationships between global warming and long-term soil moisture changes, since they are derived from the linear trend between the covariate (here GMST) and the index (here regional summer mean root zone soil moisture) rather than the ratio of occurrence probabilities, for which the denominator – the probability of the event for the past climate – can become very small, as is the case for GLDAS-CLSM.
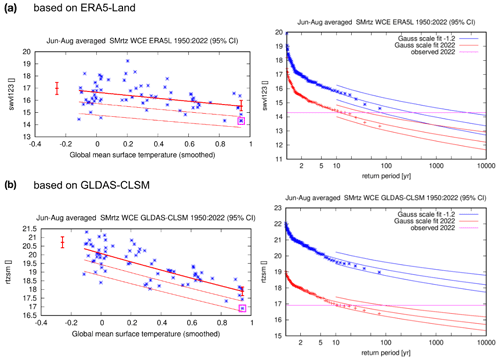
Figure 4Summer WCE root zone soil moisture under global warming. Gaussian fit with location parameter scaling proportional to GMST and constant dispersion parameter for the WCE region and based on (a) ERA5-Land and (b) GLDAS-CLSM. The 2022 event is included in the fit. On the left-hand side, observed summer mean surface soil moisture is shown as a function of the smoothed GMST. The thick red line denotes the time-varying location parameter. The vertical red lines show the 95 % confidence interval for the location parameter, for the current (2022) climate and a 1.2 ∘C cooler climate. The 2022 observation is highlighted with the magenta box. On the right-hand side, return time plots for the climate of 2022 (red) and a climate with GMST 1.2 ∘C cooler (blue) are shown. The observations are shown twice: once shifted up to the current climate and once shifted down to the climate of the late 19th century. The markers show the data and the lines show the fits and uncertainty from the bootstrap. The magenta line shows the magnitude of the 2022 event analyzed here.
Overall, these results indicate that an event such as the 2022 summer drought in WCE has become far more likely due to our warming climate. We also perform an analogous analysis for the June–August average temperature and precipitation (Figs. S3 and S4). Temperature shows very strong trends with probability ratios of at least 170 for E-OBS data and even much larger for ERA5 data. This corresponds to a change in intensity of about 1.7 to 2 ∘C (for details see Table S6). The return period used for the model analysis of temperature in the WCE region is 20 years. Trends in precipitation are much smaller and encompass no change (Table S7), and we employ a return period for the model analysis of a low-precipitation event in the WCE region of 10 years.
4.2.2 Northern extratropics
Repeating our analysis for the northern extratropics, we find 2022 summer root zone soil drought return periods of about 6 and 20 years for GLDAS-CLSM and ERA5-Land, respectively (Fig. 5). The resulting average of 13 years is slightly lower than, but still comparable to, the mean return period determined for WCE (17.5 years), and we proceed with the same value of 20 years for the subsequent model analyses such that our results for WCE and NHET can be easily compared. Our conclusions are not affected by this choice. ERA5-Land-based data gives a probability ratio of around 700 (50 to 70 000), and GLDAS-CLSM is even larger, with a lower bound already on the order of 10 million. The corresponding changes in intensity of root zone soil moisture are −2.4 % (−3.2 % to −1.5 %) for ERA5-Land, and −3.1 % (−3.6 % to −2.7 %) for GLDAS-CLSM. Compared to the much smaller European region, there is thus a weaker tendency toward soil drying in summer, yet the warming-induced change in probability of occurrence of a 2022-like soil moisture deficit is even higher in NHET. We attribute this to the fact that the interannual variability of climate variables tends to decrease at larger spatial scales, especially for precipitation but also for temperature (Giorgi, 2002; Lehner et al., 2020), so that the anthropogenic signal emerges more clearly for the northern extratropics despite weaker downward soil moisture trends. We complement our investigation by analyzing the June–August average temperature and precipitation for the NHET domain (Figs. S5 and S6), with temperature showing strong trends and very large probability ratios for ERA5 data. This indicates that such a hot summer would have been virtually impossible without climate change, and the corresponding change in intensity is about 1.9 ∘C with a 95 % confidence interval of 1.7 to 2.1 ∘C (for details see Table S9). The return period used for the model analysis of temperature in the NHET region is 10 years. As for the WCE region, the trend in precipitation is much smaller and encompasses no change; see Table S10 for details. The return period used for the model analysis of a low-precipitation event in the NHET region is 10 years.
In a final step, we combine results from observations-based products – the offline reanalysis or observation-driven land surface model simulations ERA5-Land and GLDAS-CLSM – and models that passed the evaluation. This synthesis, explained in Sect. 3.5, enables us to give overarching attribution statements building on all the employed simulations and observation-driven estimates.
5.1 Western–central Europe root zone soil moisture
For probability ratios of WCE, Fig. 6 reveals large representation errors (white bars surrounding observational estimates), owing to the fact that the confidence intervals of observation-based estimates (light blue shading) do not overlap. The model uncertainty is comparatively low, and the probability ratio averaged across models of 2.2 (0.4 to 13) is notably lower than for the observation-based estimates with 546 (0.1 to 2.3×106). When combining models and “observations” according to their visualized uncertainties, the high representation error results in a synthesis dominated by the models, with a probability ratio best estimate of 2.8 (0.5 to 16). This partly holds for the change in intensity as well, for which the models also show a weaker signal than the observation-driven soil moisture products, synthesized to a best estimate of −3.7 % (−7.4 % to 0.1 %). We point out that here, consistent with the World Weather Attribution Protocol (Philip et al., 2020a; van Oldenborgh et al., 2021), we rely on historical climate simulations extended with one of the climate scenarios up to the event year, 2022. This makes the statistical analysis more robust due to the larger sample size, from 1850 onwards, compared to the observation-driven estimates.
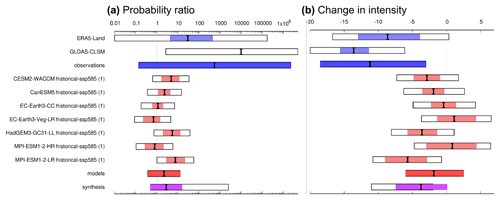
Figure 6Synthesis for WCE root zone soil moisture. Synthesized (a) probability ratios and (b) intensity changes (%) when comparing the return period and magnitudes of the 2022 summer root zone soil moisture for the WCE region in the current climate and a 1.2 ∘C cooler climate. Note that while the employed observation-based products are restricted to 1950–2022, for models we make use of the additional available data for the statistical analysis (1850–2022).
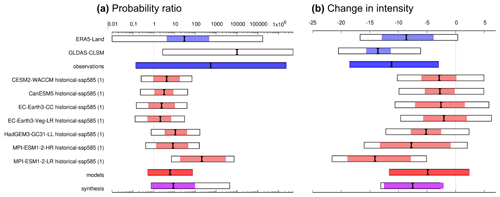
Figure 7As in Fig. 6 but restricting the model data to 1950–2022 and hence using consistent time periods among observation-driven estimates and models.
Nonetheless, we repeat this analysis in the next step, enforcing a uniform analysis period of 1950–2022 for all datasets and models to use a consistent time period for models and observations. The resulting synthesis plot (Fig. 7) is the product of the same methodological steps used to create Fig. 6. Given that the long-term evolution of soil moisture is dominated by global warming in the models, these figures should depict similar best estimates since our analysis evaluates soil moisture changes as a function of warming rather than time. Compared to recent decades since about the mid-1980s, when the period of global dimming had ended (Wild et al., 2005), there was little warming between 1850 and 1950, and soil moisture is expected to portray at most weak trends. For some models, such as CESM2-WACCM, WCE root zone soil moisture is fully consistent with this expectation, depicting a moderate decline up until 1980, followed by sharp decrease (Fig. S7). Other models, such as MPI-ESM1-2-HR, feature increasing soil moisture from preindustrial times into the second half of the 20th century for the same region, followed by clear downward trends. In other words, for some models, the sign of the apparent relationship between GMST and soil moisture changes, which is consistent with a study pointing to potentially nonlinear scaling of soil moisture with global warming (Lehner and Coats, 2021) and can mask the emerging response to strong global warming in recent decades within our (linear) statistical framework. This is why when we restrict the analysis period to 1950 onwards, Fig. 7 depicts a probability ratio on the order of 10 for MPI-ESM1-2-HR, whereas it is < 1 in Fig. 6. For CESM2-WACCM, on the other hand, whose long-term root zone soil moisture in WCE evolves in line with the nonlinear GMST increase, the probability ratio remains between 4 and 5 for both analysis periods.
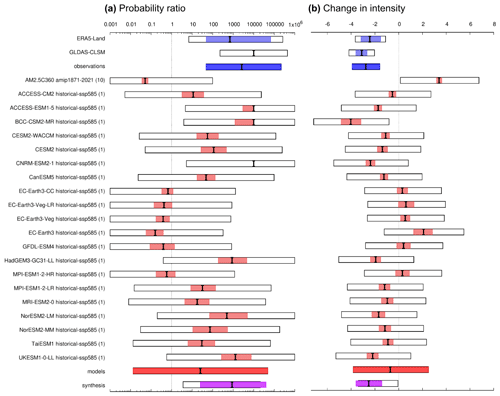
Figure 8Synthesis for NHET root zone soil moisture. Synthesized (a) probability ratios and (b) intensity changes (%) when comparing the return period and magnitudes of the 2022 June–August root zone soil moisture for the northern extratropics in the current climate and a 1.2 ∘C cooler climate. Note that while the employed observation-based products are restricted to 1950–2022, for models we make use of the additional available data for the statistical analysis (1850–2022).
For most models, Fig. 7 shows both higher probability ratios and stronger mean soil moisture declines than Fig. 6, with a synthesized probability ratio of 8.8 (0.8 to 93.6) and changes in intensity of −7.5 % (−12.5 % to −2.1 %.). Consequently, the model probability ratios are more consistent with ERA5-Land, although GLDAS-CLSM still features a much stronger warming signal. In terms of the more robust changes in intensity, GLDAS-CLSM is similar to MPI-ESM1-2-LR, the model with the strongest signal, whereas ERA5-Land is closer to the remaining models. As outlined in Sect. 4.1, the different observation-driven datasets agree on the decline in WCE root zone soil moisture after 1980, yet the ECMWF products suggest an upward tendency prior to 1980 that is largely absent in GLDAS-CLSM. Such disagreements are also found among the models; e.g., MPI-ESM1-2-LR suggests that WCE root zone soil moisture decreases notably sooner than MPI-ESM1-2-HR and is similar to GLDAS-CLSM with downward trends since about 1960. GLDAS-CLSM and MPI-ESM1-2-LR likely indicate the strongest anthropogenic fingerprint in root zone soil moisture precisely because of this, as our statistical approach can infer a stronger link between global warming and soil moisture changes. Finally, we remark that among the 25 available CMIP6 models used here (of which 7 passed the validation), all agree that based on 1950–2022, the best estimate of the probability ratio is at least 1 and oftentimes on the order of 10 or higher. Nonetheless, the lower bounds of the probability ratio for all validated models and observation-based estimates – with MPI-ESM1-2-LR and GLDAS-CLSM being the sole exceptions – are below 1, while the corresponding upper bound of the change in intensity is positive, suggesting that a weaker or even opposing response to global warming than suggested by the best estimates is possible (albeit unlikely). Overall, our analysis indicates a human-induced summer root zone soil moisture decline in western–central Europe, rendering the 2022 soil drought more likely than in a preindustrial climate, although the associated uncertainties are high.
5.2 Northern extratropics root zone soil moisture
Moving on to the northern extratropics, for which far more models have passed validation (always performed for the respective domain), the synthesized probability ratio using the weighted average is much larger than for the WCE region, with a probability ratio of 877 (25 to 39 900). The unweighted synthesis, that is, averaged giving equal weight to observation-based estimates (blue bar) and models (red bar), has a similarly large upper bound, whereas the lower bound amounts to 4 (Fig. 8a). For such high probability ratios, the exact quantification of the best estimate is highly uncertain; hence, we use the (weighted) lower bound as the synthesized result, which suggests that anthropogenic climate change has increased the likelihood of the NHET root zone soil moisture event by a factor of at least 20. The synthesized change in intensity is −2.5 % (−3.6 % to −1.4 %) when combining individual models and observation-based estimates according to their total uncertainties (Fig. 8b) and is similar for an unweighted average. Overall, Fig. 8 shows that most validated models agree with the employed observational datasets in that an event such as the 2022 summer soil drought has become more likely under global warming. But while the observation-based products agree that the probability ratio is larger than 1 (and the change in intensity below 0) based on their confidence intervals, a clear majority of the models feature a weaker and less certain warming response. Compared to WCE (cf. Figs. 6 or 7), the intermodel spread is higher, whereas the representation error of the observation-based products is lower. We note again, however, that in light of our findings in Sect. 4.1, GLDAS-CLSM and ERA5-Land may not sufficiently capture the true “observational” uncertainty, and hence we emphasize here that these root zone soil moisture attribution results, particularly for the NHET region, should be interpreted with caution.
5.3 Temperature and precipitation
The 2022 summer was characterized by unusually hot and dry conditions in western–central Europe and across much of the northern extratropics, as evidenced by positive temperature anomalies and precipitation deficits. For WCE and compared to the entire analysis period (1950–2022), we obtain standardized precipitation anomalies of −1.4σ and −2σ according to ERA5 and E-OBS, whereas temperature anomalies amount to 2.3σ in both products. ERA5 features similar anomalies in the northern extratropics, with −1.3σ and 2.2σ for precipitation and temperature, respectively. Considering these pronounced anomalies, excessive heat and precipitation shortages likely played an important role in the occurrence of soil drought in the 2022 summer. As noted in Sect. 4.2.1 and 4.2.2, however, we have found clear upward temperature trends for temperature yet no clear precipitation changes for the two regions in ERA5 (and E-OBS for WCE). Here, we also include model results to further examine changes in precipitation and temperature (Figs. S8–11). Using the same synthesis procedure, the weighted average for temperature in the WCE region is PR = 2430 (214–26400), with a change in intensity of 1.8 (1.1 to 2.5) ∘C, (Fig. S8). Similarly, for the northern extratropics, the change in intensity is 1.9 (1.7 to 2.1) ∘C. The synthesized probability ratio, on the other hand, is even higher than for WCE; it is so high that we refrain from a quantification and instead limit the values to 10 000 (see also Sect. 3.5), thereby confirming the finding from the observational analysis that the extreme temperatures over the NHET region would have been virtually impossible without climate change (Fig. S10). This is consistent with our soil moisture analysis, for which a stronger warming signal emerged in the larger region. In contrast, the change in precipitation is centered around 1 for both regions (Figs. S9 and S11), with no clear changes in intensity.
These results suggest that for both domains, trends in root zone soil moisture are likely fueled by increasing temperatures, since no clear signal emerges for precipitation. Previous research by Cheng and Huang (2016), who argue that the interannual to decadal variability of soil moisture tends to be controlled by precipitation, whereas long-term changes are dominated by upward temperature trends, is consistent with our findings. This does not imply that precipitation shortages were irrelevant for the occurrence of soil drought in the 2022 summer but rather that these rainfall deficits are primarily manifestations of natural variability. The regional summer temperatures are of course also subject to natural variability but additionally reveal a clear warming signal that considerably boosts the probability of occurrence of marked positive anomalies such as in 2022.
5.4 Surface soil moisture
To complement our statistical analysis of the relationship between the warming climate and root zone soil moisture in western–central Europe and the northern extratropics, we also attribute the 2022 surface soil moisture drought. Since long-term changes in observation-based root zone and surface soil moisture estimates seem largely consistent for both domains (Fig. 2), we expect similar results to those for the analysis of agro-ecological drought. ERA5 – which, unlike ERA5-Land, assimilates soil moisture data from scatterometers – is also considered for the analysis of surface soil moisture because the discontinuities due to the use of multiple production streams for the reanalysis only affect deeper soil layers.
We provide the event return period and long-term trend analysis for surface soil moisture in the Supplement (Figs. S18–19) and proceed with the same return period of 20 years as for root zone soil moisture. For the surface soil moisture in western–central Europe, the synthesized probability ratio using the weighted average is 8.0 (1.1 to 59.2), whereas the unweighted upper bound is much larger at 1350, and the lower bound in this case is similar to the weighted average, as shown in Fig. S12a. As for WCE root zone soil moisture, we use the rounded best estimate as the synthesized result, suggesting anthropogenic climate change has increased the likelihood of the WCE surface soil moisture event by a factor of about 8. The change in intensity for the same event is shown in Fig. S12b and averages −9.0 % (−14.5 % to −3.3 %).
For the surface soil moisture in the northern extratropics, the synthesized probability ratio using the weighted average is again much larger than for the WCE region, with a probability ratio of 320 (5.4 to 21800), as shown in Fig. S13a. Consistent with our analysis of root zone soil moisture in the northern extratropics, we rely on the lower bound as the synthesized result; anthropogenic climate change has increased the likelihood of the NHET surface soil moisture event by a factor of at least 5. The change in intensity for the same event is shown in Fig. S13b, suggesting an average of −3.1 % (−5.2 % to −1.0 %).
5.5 Synthesis for an additional warming of 0.8 ∘C
We also assessed how the frequency and intensity of the two types of soil moisture drought in both regions would change in a 0.8 ∘C warmer world compared to today. For all event definitions, a further increase in intensity and a ∼ 2–30-fold further increase in the frequency of such an event are found (Figs. S14–17). For WCE (NHET) and in terms of best estimates, the PR of root zone and surface soil moisture droughts amount to 1.6 and 2.3 (14.6 and 32.4), respectively. In combination with the strong trends in temperature extremes, these results strengthen our confidence in the soil moisture results, even though an exact quantification is difficult due to the difficulties in measuring soil moisture and resulting large discrepancies in observation-based datasets.
Extending our rapid attribution analysis (Schumacher et al., 2022), we find evidence for a global warming-induced summer root zone soil moisture decline in western–central Europe, and several observation-driven soil moisture estimates agree on a downward trend since at least 1980. Our analysis suggests that the large uncertainties, also due to a lack of in situ root zone soil moisture observations except for a few hundred stations, make it difficult to communicate precise numbers. Nevertheless, the synthesized probability ratio for a 2022-like summer drought in western–central Europe is likely larger than 1 and amounts to about 5 (2.8 when using 1850–2022 model data, and 8.8 for 1950–2022). In other words, combining observation-driven and model evidence, we find that anthropogenic climate change has made such an event more probable. We emphasize here, however, that the lower bound of the synthesized probability ratio is below 1, and hence we cannot exclude the possibility that the likelihood of an event such as the 2022 soil drought has not been modulated (or even decreased) by human-induced global warming. For the northern extratropics, our analysis suggests a stronger overall warming signal, with a probability ratio of at least 20, and associated decline in root zone soil moisture. The “observational” uncertainty is higher than for western–central Europe, however, and hence this result should be treated with caution. Moreover, observation-based soil moisture products do not agree on when the warming signal becomes evident, with GLDAS-CLSM displaying drying tendencies about 2 decades earlier than the ECMWF products for both regions. Similarly, nearly all CMIP6 models display declining summertime root zone soil moisture throughout the 21st century in western–central Europe and averaged over the northern extratropics, but there is no agreement whether this decline started in recent decades or already in preindustrial times. This could indicate that long-term soil moisture changes are not solely driven by global warming, and hence only emerge clearly in the presence of strong warming. In snow-dominated regions it is also possible that changes in snowpack and precipitation partitioning in winter and spring influence soil moisture droughts in the subsequent summer (Wieder et al., 2022).
Nonetheless, and in line with previous research, our results point to increasing temperatures as a key driver behind declining soil moisture in western–central Europe and across much of the northern extratropics. Our analysis of surface soil moisture provides additional evidence for an enhanced tendency toward soil drought in both regions, with similar results to those obtained for the root zone. According to the reanalysis and observation-driven land surface models ERA5-Land and GLDAS-CLSM, low summer soil moisture such as observed in 2022 happens about once in 20 years in today's climate in both regions. For a preindustrial climate (1.2 ∘C cooler than the present), a similarly intense soil drought would take place in western–central Europe roughly once per century, and even less often in the northern extratropics. In this context, we point out that our analysis has been largely restricted to the lower bounds and best estimates of the synthesized probability ratios and intensity changes. This appears adequate considering that the uncertainty in the attribution of extremes in soil moisture is higher than for variables such as temperature, and hence we intentionally stay on the conservative side. Even so, in light of the high upper bounds, we also mention the possibility that our best estimates underestimate the decline in soil moisture in response to a warming climate, in which case widespread drought conditions as in the 2022 summer would have been virtually impossible without human-induced climate change. Moreover, the models analyzed also show that soil moisture drought will continue to increase with additional global warming – in western–central Europe, a 2022-like event or worse is expected to occur about every 10 years once a warming level of 2 ∘C is reached, and nearly every single year in the northern extratropics. In other words, for 0.8 ∘C additional warming compared to the present, the mean probability ratios of surface and root zone soil moisture drought in western–central Europe and the northern extratropics amount to about 2 and 20, respectively. This is consistent with projected long-term trends in climate models as reported, e.g., in the IPCC AR6 (IPCC, 2021), and should serve as a strong motivation to increase our efforts to limit future global warming.
The code used to process the data and perform analysis can be obtained from the corresponding author upon request.
The data used for the statistical analyses are available via the Climate Explorer (https://climexp.knmi.nl/WCE_NHETDrought2022.cgi, KNMI, 2022).
The supplement related to this article is available online at: https://doi.org/10.5194/esd-15-131-2024-supplement.
HKB, SL, WY, MaH, MHir, VB, and DLS prepared data for analysis and/or contributed post-processing code. MZ, FO, CB, SP, SK, SL, WY, and DLS analyzed the data. MZ, FO, CB, SP, SK, MV, RS, DH, JA, MvA, LJH and DLS wrote the first manuscript draft. All authors reviewed and edited the manuscript.
At least one of the (co-)authors is a member of the editorial board of Earth System Dynamics. The peer-review process was guided by an independent editor, and the authors also have no other competing interests to declare.
Publisher’s note: Copernicus Publications remains neutral with regard to jurisdictional claims made in the text, published maps, institutional affiliations, or any other geographical representation in this paper. While Copernicus Publications makes every effort to include appropriate place names, the final responsibility lies with the authors.
This analysis was funded through the XAIDA project by the European Union's Horizon 2020 Research and Innovation Programme under grant agreement no. 101003469.
This research has been supported by Horizon 2020 (grant no. 101003469).
This paper was edited by Richard Betts and reviewed by Xing Yuan and one anonymous referee.
Ahmedzade, T., Horton, J., Mwai, P., and Song, W.: China, Europe, US drought: Is 2022 the driest year recorded?, https://www.bbc.com/news/62751110 (last access: 27 February 2023), 2022.
Al Jazeera: “Historic” drought prompts French government into action, https://www.aljazeera.com/news/2022/8/5/france-orders-crisis-task-force-over-historic-drought (last access: 13 March 2023), 2022.
Albergel, C., Rüdiger, C., Pellarin, T., Calvet, J.-C., Fritz, N., Froissard, F., Suquia, D., Petitpa, A., Piguet, B., and Martin, E.: From near-surface to root-zone soil moisture using an exponential filter: an assessment of the method based on in-situ observations and model simulations, Hydrol. Earth Syst. Sci., 12, 1323–1337, https://doi.org/10.5194/hess-12-1323-2008, 2008.
Albergel, C., Dorigo, W., Reichle, R. H., Balsamo, G., de Rosnay, P., Muñoz-Sabater, J., Isaksen, L., de Jeu, R., and Wagner, W.: Skill and Global Trend Analysis of Soil Moisture from Reanalyses and Microwave Remote Sensing, J. Hydrometeorol., 13, 1259–1277, https://doi.org/10.1175/JHM-D-12-0161.1, 2013.
Almendra-Martín, L., Martínez-Fernández, J., Piles, M., González-Zamora, Á., Benito-Verdugo, P., and Gaona, J.: Analysis of soil moisture trends in Europe using rank-based and empirical decomposition approaches, Glob. Planet. Change, 215, 103868, https://doi.org/10.1016/j.gloplacha.2022.103868, 2022.
Babaeian, E., Sadeghi, M., Jones, S. B., Montzka, C., Vereecken, H., and Tuller, M.: Ground, Proximal, and Satellite Remote Sensing of Soil Moisture, Rev. Geophys., 57, 530–616, https://doi.org/10.1029/2018RG000618, 2019.
BBC: France experiencing worst drought on record, https://www.bbc.co.uk/newsround/62456540 (last access: 13 March 2023), 2022.
BBC Weather: Europe heatwave breaks multiple June records, https://www.bbc.com/weather/features/62001812 (last access: 27 February 2023), 2022.
Berg, A. and Sheffield, J.: Climate Change and Drought: the Soil Moisture Perspective, Curr. Clim. Chang. Rep., 4, 180–191, https://doi.org/10.1007/s40641-018-0095-0, 2018.
Bessenbacher, V., Seneviratne, S. I., and Gudmundsson, L.: CLIMFILL v0.9: a framework for intelligently gap filling Earth observations, Geosci. Model Dev., 15, 4569–4596, https://doi.org/10.5194/gmd-15-4569-2022, 2022.
Bessenbacher, V., Schumacher, D. L., Hirschi, M., Seneviratne, S. I., and Gudmundsson, L.: Gap-filled Multivariate Observations of Global Land–climate Interactions, JGR Atmos., 128, e2023JD039099, https://doi.org/10.1029/2023JD039099, 2023.
Bi, H., Ma, J.,Zheng, W. and Zeng, J.: Comparison of Soil Moisture in GLDAS Model Simulations and in situ Observations over the Tibetan Plateau, J. Geophys. Res.-Atmos., 121, 2658–2678, https://doi.org/10.1002/2015JD024131, 2016.
Bielza Diaz-Caneja, M., Conte, C., and Gallego Pinilla, F.: Risk management and agricultural insurance schemes in Europe, Joint Research Centre, Institute for the Protection and Security of the Citizen, Publications Office, https://data.europa.eu/doi/10.2788/24307 (last access: 13 March 2023), 2009.
Binnie, I. and Twidale, S.: Europe's power system feels the heat as cooling demand soars, https://www.reuters.com/world/europe/europes-power-system-feels-heat-cooling-demand-soars-2022-07-21/ (last access: 13 March 2023), 2022.
Blauhut, V., Stahl, K., Stagge, J. H., Tallaksen, L. M., De Stefano, L., and Vogt, J.: Estimating drought risk across Europe from reported drought impacts, drought indices, and vulnerability factors, Hydrol. Earth Syst. Sci., 20, 2779–2800, https://doi.org/10.5194/hess-20-2779-2016, 2016.
Blauhut, V., Stoelzle, M., Ahopelto, L., Brunner, M. I., Teutschbein, C., Wendt, D. E., Akstinas, V., Bakke, S. J., Barker, L. J., Bartošová, L., Briede, A., Cammalleri, C., Kalin, K. C., De Stefano, L., Fendeková, M., Finger, D. C., Huysmans, M., Ivanov, M., Jaagus, J., Jakubínský, J., Krakovska, S., Laaha, G., Lakatos, M., Manevski, K., Neumann Andersen, M., Nikolova, N., Osuch, M., van Oel, P., Radeva, K., Romanowicz, R. J., Toth, E., Trnka, M., Urošev, M., Urquijo Reguera, J., Sauquet, E., Stevkov, A., Tallaksen, L. M., Trofimova, I., Van Loon, A. F., van Vliet, M. T. H., Vidal, J.-P., Wanders, N., Werner, M., Willems, P., and Živković, N.: Lessons from the 2018–2019 European droughts: a collective need for unifying drought risk management, Nat. Hazards Earth Syst. Sci., 22, 2201–2217, https://doi.org/10.5194/nhess-22-2201-2022, 2022.
Boergens, E., Güntner, A., Dobslaw, H., and Dahle, C.: Quantifying the Central European droughts in 2018 and 2019 with GRACE Follow-On, Geophys. Res. Lett., 47, e2020GL087285, https://doi.org/10.1029/2020GL087285, 2020.
Bradsher, K. and Dong, J.: China's Record Drought Is Drying Rivers and Feeding Its Coal Habit, last modified: 29 August 2022, https://www.nytimes.com/2022/08/26/business/economy/china-drought-economy-climate.html (last access: 13 March 2023), 2022.
Breeden, A.: “Most Severe” Drought Grips France as Extreme Heat Persists in Europe, https://www.nytimes.com/2022/08/05/world/europe/france-drought-europe-heat.html (last access: 13 March 2023), 2022.
Breteau, P.: Heat wave: View the high-temperature records broken in France in June and July, https://www.lemonde.fr/en/les-decodeurs/ (last access: 27 February 2023), 2022.
Chadwick, L.: More than 100 French towns without drinking water amid “historic drought”, https://www.euronews.com/my-europe/ (last access: 13 March 2023), 2022.
Chan, D., Vecchi, G. A., Yang, W., and Huybers, P.: Improved simulation of 19th- and 20th-century North Atlantic hurricane frequency after correcting historical sea surface temperatures, Sci. Adv., 7, eabg6931, https://doi.org/10.1126/sciadv.abg6931, 2021.
Chen, Y., Feng, X., and Fu, B.: An improved global remote-sensing-based surface soil moisture (RSSSM) dataset covering 2003–2018, Earth Syst. Sci. Data, 13, 1–31, https://doi.org/10.5194/essd-13-1-2021, 2021.
Cheng, S. and Huang, J.: Enhanced soil moisture drying in transitional regions under a warming climate, J. Geophys. Res.-Atmos., 121, 2542–2555, https://doi.org/10.1002/2015JD024559, 2016.
Ciavarella, A., Cotterill, D., Stott, P., Kew, S., Philip, S., van Oldenborgh, G. J., Skålevåg, A., Lorenz, P., Robin, Y., Otto, F., Hauser, M., Seneviratne, S. I., Lehner, F., and Zolina, O.: Prolonged Siberian heat of 2020 almost impossible without human influence, Climatic Change, 166, 9, https://doi.org/10.1007/s10584-021-03052-w, 2021.
Clifford, C.: Italy has declared a state of emergency because of drought: “There is no doubt that climate change is having an effect”, the prime minister said, https://www.cnbc.com/2022/07/05/ (last access: 13 March 2023), 2022.
CMA: China Meteorological Administration: Combined intensity of heat wave events has reached the strongest since 1961 according to BCC, https://www.cma.gov.cn/en2014/news/News/202208/t20220821_5045788.html (last access: 27 February 2023), 2022.
Coldiretti: Siccità: devasta il raccolto di riso italiano (−30 %), https://www.coldiretti.it/economia/siccita-devasta-il-raccolto-di-riso-italiano-30 (last access: 13 March 2023), 2022.
Copernicus: Precipitation, relative humidity and soil moisture for August 2021, Hydrological climate bulletin, https://climate.copernicus.eu/precipitation-relative-humidity-and-soil-moisture-august-2021 (last access: 27 February 2023), 2021.
Copernicus: Summer 2022 Europe's hottest on record, https://climate.copernicus.eu/copernicus-summer-2022-europes-hottest-record (last access: 27 February 2023), 2022a.
Copernicus: Europe's summer wildfire emissions highest in 15 years, https://atmosphere.copernicus.eu/europes-summer-wildfire-emissions-highest-15-years (last access: 13 March 2023), 2022b.
Coumou, D., Petoukhov, V., Rahmstorf, S., Petri, S., and Schellnhuber, H. J.: Quasi-resonant circulation regimes and hemispheric synchronization of extreme weather in boreal summer, P. Natl. Acad. Sci. USA, 111, 12331–12336, https://doi.org/10.1073/pnas.1412797111, 2014.
Cornes, R. C., van der Schrier, G., van den Besselaar, E. J., and Jones, P. D.: An Ensemble Version of the E-OBS Temperature and Precipitation Data Sets, J. Geophys. Res.-Atmos., 123, 9391–9409, https://doi.org/10.1029/2017JD028200, 2018.
CPC: Climate Prediction Center: Historical El Nino/La Nina episodes (1950-present), https://origin.cpc.ncep.noaa.gov/products/ (last access: 27 February 2023), 2022.
Delworth, T. L., Rosati, A., Anderson, W., Adcroft, A. J., Balaji, V., Benson, R., Dixon, K., Griffies, S. M., Lee, H.-C., Pacanowski, R. C., Vecchi, G. A., Wittenberg, A. T., Zeng, F., and Zhang, R.: Simulated Climate and Climate Change in the GFDL CM2.5 High-Resolution Coupled Climate Model, J. Clim., 25, 2755–2781, https://doi.org/10.1175/JCLI-D-11-00316.1, 2012.
Deng, Y., Wang, S., Bai, X., Luo, G., Wu, L., Cao, Y., Li, H., Li, C., Yang, Y., Hu, Z., and Tian, S.: Variation trend of global soil moisture and its cause analysis, Ecol. Indicat., 110, 105939, https://doi.org/10.1016/j.ecolind.2019.105939, 2020.
DESTATIS: Federal Statistical Office of Germany: Sonderauswertung zu Sterbefallzahlen: Entwicklung im Jahr 2022, https://www.destatis.de/DE/Themen/Gesellschaft-Umwelt/Bevoelkerung/Sterbefaelle-Lebenserwartung/sterbefallzahlen.html{#} (last access: 13 March 2023), 2022a.
DESTATIS: Federal Statistical Office of Germany: Sterbefallzahlen im Juni 2022 um 8 % über dem mittleren Wert der Vorjahre, press release nr. 295, https://www.destatis.de/DE/Presse/ (last access: 13 March 2023), 2022b.
Dhanesha, N. and Jones, B.: It's so hot in Europe that roads are literally buckling: The world wasn't built for this heat, https://www.vox.com/2022/7/20/23270092/europe-uk-heat-wave-roads-trains-climate-change (last access: 13 March 2023), 2022.
Dorigo, W. A., Jeu, R. D., Chung, D., Parinussa, R., Liu, Y., Wagner, W., and Fernández-Prieto, D.: Evaluating global trends (1988–2010) in harmonized multi-satellite surface soil moisture, Geophys. Res. Lett., 39, 18405, https://doi.org/10.1029/2012GL052988, 2012.
Dorigo, W. A., Gruber, A., De Jeu, R. A. M., Wagner, W. T., Stacke, T., Loew, A., Albergel, C., Brocca, L., Chung, D., Parinussa, R. M., and Kidd, R.: Evaluation of the ESA CCI soil moisture product using ground-based observations, Remote Sens. Environ., 162, 380–395, https://doi.org/10.1016/j.rse.2014.07.023, 2015.
Dorigo, W., Wagner, W., Albergel, C., Albrecht, F., Balsamo, G., Brocca, L., Chung, D., Ertl, M., Forkel, M., Gruber, A., Haas, E., Hamer, P. D., Hirschi, M., Ikonen, J., de Jeu, R., Kidd, R., Lahoz, W., Liu, Y. Y., Miralles, D., Mistelbauer, T., Nicolai-Shaw, N., Parinussa, R., Pratola, C., Reimer, C., van der Schalie, R., Seneviratne, S. I., Smolander, T., and Lecomte, P.: ESA CCI Soil Moisture for improved Earth system understanding: State-of-the art and future directions, Remote Sens. Environ., 203, 185–215, https://doi.org/10.1016/j.rse.2017.07.001, 2017.
Dumitrescu, R.: Romania sees sevenfold increase in vegetation fires in 2022, https://www.romania-insider.com/romania-sevenfold-increase-vegetation-fires-2022 (last access: 13 March 2023), 2022.
DW: Deutsche Welle: Serious drought hits Europe and beyond, https://www.dw.com/en/serious-drought-hitting-europe-wider-world/a-62786406 (last access: 13 March 2023), 2022.
EC JRC: European Commission Joint Research Centre: LISFLOOD – a distributed hydrological rainfall-runoff model, Model documentation, https://ec-jrc.github.io/lisflood-model/Lisflood_Model.pdf (last access: 13 March 2023), 2020.
EC JRC: European Commission Joint Research Centre: Droughts in Europe in July 2022: almost half of the EU + UK territory at risk, https://joint-research-centre.ec.europa.eu/ (last access: 27 February 2023), 2022a.
EC JRC: European Commission's Joint Research Centre: Summer drought keeps its grip on Europe, https://joint-research-centre.ec.europa.eu/ (last access: 13 March 2023), 2022b.
EDO: European Drought Observatory: Drought Map Generator, https://edo.jrc.ec.europa.eu/edov2/php/index.php?id=1181 (last access: 13 March 2023), 2022.
EEA: European Environment Agency: Water use by sectors, https://www.eea.europa.eu/archived/archived-content-water-topic/water-resources/water-use-by-sectors (last access: 13 March 2023), 2020a.
EEA: European Environment Agency: The Problems of water stress, https://www.eea.europa.eu/publications/92-9167-025-1/page003.html (last access: 13 March 2023), 2020b.
EFFIS: European Forest Fire Information System: Seasonal Trend for European Union (charts updated every 7 days, archive available), https://effis.jrc.ec.europa.eu/apps/effis.statistics/seasonaltrend (last access: 27 February 2023), 2022.
Eyring, V., Bony, S., Meehl, G. A., Senior, C. A., Stevens, B., Stouffer, R. J., and Taylor, K. E.: Overview of the Coupled Model Intercomparison Project Phase 6 (CMIP6) experimental design and organization, Geosci. Model Dev., 9, 1937–1958, https://doi.org/10.5194/gmd-9-1937-2016, 2016.
Garcia, D.: Robust smoothing of gridded data in one and higher dimensions with missing values, Comput. Stat. Data An., 54, 1167–1178, https://doi.org/10.1016/j.csda.2009.09.020, 2010.
Gazol, A. and Camarero, J. J.: Compound climate events increase tree drought mortality across European forests, Sci. Total Environ., 816, 151604, https://doi.org/10.1016/j.scitotenv.2021.151604, 2022.
Gevaert, A. I., Miralles, D. G., de Jeu, R. A. M., Schellekens, J., and Dolman, A. J.: Soil moisture-temperature coupling in a set of land surface models, J. Geophys. Res.-Atmos., 123, 1481–1498, https://doi.org/10.1002/2017JD027346, 2018.
Giorgi, F.: Dependence of the surface climate interannual variability on spatial scale, Geophys. Res. Lett., 29, 16-1–16-4, https://doi.org/10.1029/2002GL016175, 2002.
Good, R., Sandford, A., and Askew, J.: Drought and heatwaves: How is Europe tackling unprecedented water shortages?, https://www.euronews.com/2022/08/08/ (last access: 13 March 2023), 2022.
Gruber, A., Scanlon, T., van der Schalie, R., Wagner, W., and Dorigo, W.: Evolution of the ESA CCI Soil Moisture climate data records and their underlying merging methodology, Earth Syst. Sci. Data, 11, 717–739, https://doi.org/10.5194/essd-11-717-2019, 2019.
Haarsma, R. J., Roberts, M. J., Vidale, P. L., Senior, C. A., Bellucci, A., Bao, Q., Chang, P., Corti, S., Fučkar, N. S., Guemas, V., von Hardenberg, J., Hazeleger, W., Kodama, C., Koenigk, T., Leung, L. R., Lu, J., Luo, J.-J., Mao, J., Mizielinski, M. S., Mizuta, R., Nobre, P., Satoh, M., Scoccimarro, E., Semmler, T., Small, J., and von Storch, J.-S.: High Resolution Model Intercomparison Project (HighResMIP v1.0) for CMIP6, Geosci. Model Dev., 9, 4185–4208, https://doi.org/10.5194/gmd-9-4185-2016, 2016.
Hansen, J., Ruedy, R., Sato, M., and Lo, K.: Global surface temperature change, Rev. Geophys., 48, RG4004, https://doi.org/10.1029/2010RG000345, 2010.
Heggie, J.: Solving Italy's water problem: It's time to fix the dripping tap, https://www.nationalgeographic.com/science/article/partner-content-solving-the-water-problem-in-italy (last access: 13 March 2023), 2020.
Henley, J.: Europe's rivers run dry as scientists warn drought could be worst in 500 years, https://www.theguardian.com/environment/2022/aug/ (last access: 27 February 2023), 2022.
Hersbach, H., Bell, B., Berrisford, P., et al.: The ERA5 global reanalysis, Q. J. Roy. Meteor. Soc., 146, 1999–2049, https://doi.org/10.1002/qj.3803, 2020.
Hirschnitz-Garbers, M., Tan, A. R., Gradmann, A., and Srebotnjak, T.: Key drivers for unsustainable resource use – categories, effects and policy pointers, J. Clean. Prod., 132, 13–31, https://doi.org/10.1016/j.jclepro.2015.02.038, 2016.
Horowitz, J.: Europe's Scorching Summer Puts Unexpected Strain on Energy Supply, https://www.nytimes.com/2022/08/18/world/europe/drought-heat-energy.html (last access: 13 March 2023), 2022.
Houborg, R., Rodell, M., Li, B., Reichle, R., and Zaitchik, B. F.: Drought indicators based on model-assimilated gravity recovery and climate experiment (GRACE) terrestrial water storage observations, Water Resour. Res., 48, W07525, https://doi.org/10.1029/2011WR011291, 2012.
Hoyer, S. and Hamman, J.: xarray: N-D labeled Arrays and Datasets in Python, J. Open Res. Softw.,5, p. 10, https://doi.org/10.5334/jors.148, 2017.
IPCC: Climate Change 2021: The Physical Science Basis, Contribution of Working Group I to the Sixth Assessment Report of the Intergovernmental Panel on Climate Change, edited by: Masson-Delmotte, V., Zhai, P., Pirani, A., Connors, S. L., Péan, C., Berger, S., Caud, N., Chen, Y., Goldfarb, L., Gomis, M. I., Huang, M., Leitzell, K., Lonnoy, E., Matthews, J. B. R., Maycock, T. K., Waterfield, T., Yelekçi, O., Yu, R., and Zhou, B., Cambridge University Press, Cambridge, United Kingdom and New York, NY, USA, 2391 pp., 2021.
IPCC: Climate Change 2022: Impacts, Adaptation, and Vulnerability. Contribution of Working Group II to the Sixth Assessment Report of the Intergovernmental Panel on Climate Change, edited by: Pörtner, H.-O., Roberts, D. C., Tignor, M., Poloczanska, E. S., Mintenbeck, K., Alegría, A., Craig, M., Langsdorf, S., Löschke, S., Möller, V., Okem, A., and Rama, B., Cambridge University Press. Cambridge University Press, Cambridge, UK and New York, NY, USA, 3056 pp., 2022.
Iturbide, M., Gutiérrez, J. M., Alves, L. M., Bedia, J., Cerezo-Mota, R., Cimadevilla, E., Cofiño, A. S., Di Luca, A., Faria, S. H., Gorodetskaya, I. V., Hauser, M., Herrera, S., Hennessy, K., Hewitt, H. T., Jones, R. G., Krakovska, S., Manzanas, R., Martínez-Castro, D., Narisma, G. T., Nurhati, I. S., Pinto, I., Seneviratne, S. I., van den Hurk, B., and Vera, C. S.: An update of IPCC climate reference regions for subcontinental analysis of climate model data: definition and aggregated datasets, Earth Syst. Sci. Data, 12, 2959–2970, https://doi.org/10.5194/essd-12-2959-2020, 2020.
Kagerl, C., Moritz, M., Roth, D., Stegmaier, J., Stepanok, I., and Weber, E.: Energiekrise und Lieferstopp für Gas: Auswirkungen auf die Betriebe in Deutschland, Wirtschaftsdienst, 102, 486–491, https://doi.org/10.1007/s10273-022-3211-7, 2022.
Karori, M. A., Li, J., and Jin, F.: The Asymmetric Influence of the Two Types of El Niño and La Niña on Summer Rainfall over Southeast China, J. Clim., 26, 4567–4582, https://doi.org/10.1175/JCLI-D-12-00324.1, 2013.
Kew, S. F., Philip, S. Y., Hauser, M., Hobbins, M., Wanders, N., van Oldenborgh, G. J., van der Wiel, K., Veldkamp, T. I. E., Kimutai, J., Funk, C., and Otto, F. E. L.: Impact of precipitation and increasing temperatures on drought trends in eastern Africa, Earth Syst. Dynam., 12, 17–35, https://doi.org/10.5194/esd-12-17-2021, 2021.
KNMI: Pacific Northwest heat [data set], https://climexp.knmi.nl/pacificheat_timeseries.cgi (last access: 12 February 2024), 2022.
Kollewe, J.: EDF cuts output at nuclear power plants as French rivers get too warm, https://www.theguardian.com/business/2022/ (last access: 13 March 2023), 2022.
Korosec, M.: Karst Region on Fire – The Largest, Historic Wildfire on Record in Slovenia spreads fast under the new extreme heat dome Heatwave event over Europe, https://www.severe-weather.eu/global-weather/ (last access: 13 March 2023), 2022.
Kumar, S. V., Zaitchik, B. F., Peters-Lidard, C. D., Rodell, M., Reichle, R., Li, B., Jasinski, M., Mocko, D., Getirana, A., De Lannoy, G., Cosh, M. H., Hain, C. R., Anderson, M., Arsenault, K. R., Xia, Y., and Ek, M.: Assimilation of gridded GRACE terrestrial water storage estimates in the North American land data assimilation system, J. Hydrometeorol., 17, 1951–1972, https://doi.org/10.1175/JHM-D-15-0157.1, 2016.
Llamas, R. M., Guevara, M., Rorabaugh, D., Taufer, M., and Vargas, R.: Spatial Gap-Filling of ESA CCI Satellite-Derived Soil Moisture Based on Geostatistical Techniques and Multiple Regression, Remote Sens., 12, 665, https://doi.org/10.3390/rs12040665, 2020.
Lau, W. K. M. and Kim, K. M.: The 2010 Pakistan flood and Russian heat wave: teleconnection of hydrometeorological extremes, J. Hydrometeorol., 13, 392–403, https://doi.org/10.1175/JHM-D-11-016.1, 2012.
Lehner, F. and Coats, S.: Does regional hydroclimate change scale linearly with global warming?, Geophys. Res. Lett., 48, e2021GL095127, https://doi.org/10.1029/2021GL095127, 2021.
Le News: 2022 Switzerland's second hottest summer since 1864, https://lenews.ch/2022/09/02/2022-switzerlands-second-hottest-summer-since-1864/ (last access: 27 February 2023), 2022.
Le Page, M.: Heatwave in China is the most severe ever recorded in the world, https://www.newscientist.com/article/ (last access: 13 March 2023), 2022.
Lehner, F., Deser, C., Maher, N., Marotzke, J., Fischer, E. M., Brunner, L., Knutti, R., and Hawkins, E.: Partitioning climate projection uncertainty with multiple large ensembles and CMIP5/6, Earth Syst. Dynam., 11, 491–508, https://doi.org/10.5194/esd-11-491-2020, 2020.
Lenssen, N. J. L., Schmidt, G. A., Hansen, J. E., Menne, M. J., Persin, A., Ruedy, R., and Zyss, D.: Improvements in the GISTEMP uncertainty model, J. Geophys. Res.-Atmos., 124, 6307–6326, https://doi.org/10.1029/2018JD029522, 2019.
Li, B., Rodell, M., Kumar, S., Beaudoing, H. K., Getirana, A., Zaitchik, B. F., de Goncalves, L. G., Cossetin, C., Bhanja, S., Mukherjee, A., Tian, S., Tangdamrongsub, N., Long, D., Nanteza, J., Lee, J., Policelli, F., Goni, I. B., Daira, D., Bila, M., de Lannoy, G., Mocko, D., Steele-Dunne, S. C., Save, H., and Bettadpur, S.: Global GRACE Data Assimilation for Groundwater and Drought Monitoring: Advances and Challenges, Water Resour. Res., 55, 7564–7586, https://doi.org/10.1029/2018WR024618, 2019.
Li, M., Yao, Y., Simmonds, I., Luo, D., Zhong, L., and Chen, X.: Collaborative impact of the NAO and atmospheric blocking on European heatwaves, with a focus on the hot summer of 2018, Environ. Res. Lett., 15, 114003, https://doi.org/10.1088/1748-9326/aba6ad, 2020.
Linthicum, K.: Taps have run dry in Monterrey, Mexico, where there is water for factories but not for residents, https://www.latimes.com/world-nation/ (last access: 27 February 2023), 2022.
Liu, L., Gudmundsson, L., Hauser, M., Qin, D., Li, S., and Seneviratne, S. I.: Soil moisture dominates dryness stress on ecosystem production globally, Nat. Commun., 11, 4892, https://doi.org/10.1038/s41467-020-18631-1, 2020.
Liu, K., Li, X., Wang, S., and Zhang, H.: A robust gap-filling approach for European Space Agency Climate Change Initiative (ESA CCI) soil moisture integrating satellite observations, model-driven knowledge, and spatiotemporal machine learning, Hydrol. Earth Syst. Sci., 27, 577–598, https://doi.org/10.5194/hess-27-577-2023, 2023.
Lu, J., Carbone, G. J., and Grego, J. M.: Uncertainty and hotspots in 21st century projections of agricultural drought from CMIP5 models, Sci. Rep., 9, 4922, https://doi.org/10.1038/s41598-019-41196-z, 2019.
Lukov, Y.: France firefighters battle “monster” wildfire near Bordeaux, https://www.bbc.com/news/world-europe-62503775 (last access: 13 March 2023), 2022.
Mazzetti, C., Decremer, D., Barnard, C., and Blick, M.: River discharge and related historical data from the European Flood Awareness System, v4.0, Copernicus Climate Change Service (C3S) Climate Data Store (CDS), https://doi.org/10.24381/cds.e3458969, 2020.
McColl, K. A., He, Q., Lu, H., and Entekhabi, D.: Short-term and long-term surface soil moisture memory time scales are spatially anticorrelated at global scales, J. Hydrometeorol., 20, 1165–1182, https://doi.org/10.1175/JHM-D-18-0141.1, 2019.
Mendes, L.: “Heatflation” warning as 2022 EU crop harvests affected by climate change, https://www.tradefinanceglobal.com/posts/ (last access: 13 March 2023), 2022.
Mueller, B. and Seneviratne, S. I.: Hot days induced by precipitation deficits at the global scale, P. Natl Acad. Sci. USA, 109, 12398–12403, https://doi.org/10.1073/pnas.1204330109, 2012.
Muñoz-Sabater, J., Dutra, E., Agustí-Panareda, A., Albergel, C., Arduini, G., Balsamo, G., Boussetta, S., Choulga, M., Harrigan, S., Hersbach, H., Martens, B., Miralles, D. G., Piles, M., Rodríguez-Fernández, N. J., Zsoter, E., Buontempo, C., and Thépaut, J.-N.: ERA5-Land: a state-of-the-art global reanalysis dataset for land applications, Earth Syst. Sci. Data, 13, 4349–4383, https://doi.org/10.5194/essd-13-4349-2021, 2021.
Miralles, D. G., Gentine, P., Seneviratne, S. I., and Teuling, A. J.: Land–atmospheric feedbacks during droughts and heatwaves: state of the science and current challenges, Ann. New York Acad. Sci., 1436, 19–35, https://doi.org/10.1111/nyas.13912, 2019.
Pratt, S.: A Long-lasting Western Heatwave, https://earthobservatory.nasa.gov/images/150318/a-long-lasting-western-heatwave (last access: 27 February 2023), 2022.
Preimesberger, W., Scanlon, T., Su, C.-H., Gruber, A., and Dorigo, W.: Homogenization of Structural Breaks in the Global ESA CCI Soil Moisture Multisatellite Climate Data Record, IEEE Trans. Geosci. Remote Sens., 59, 2845–2862, https://doi.org/10.1109/TGRS.2020.3012896, 2021.
NASA GRACE-FO: Map Archive, https://nasagrace.unl.edu/Archive.aspx (last access: 13 March 2023), 2022.
Naumann, G., Cammalleri, C., Mentaschi, L., and Feyen, L.: Increased economic drought impacts in Europe with anthropogenic warming, Nat. Clim. Change, 11, 485–491, https://doi.org/10.1038/s41558-021-01044-3, 2021.
Padrón, R.S., Gudmundsson, L., Decharme, B., Ducharne, A., Lawrence, D. M., Mao, J., Peano, D., Krinner, G., Kim, H., and Seneviratne, S. I.: Observed changes in dry-season water availability attributed to human-induced climate change, Nat. Geosci., 13, 477–481, https://doi.org/10.1038/s41561-020-0594-1, 2020.
Phys.org: Italy heatwave peaks with 16 cities on red alert as Tuscany burns, https://phys.org/news/2022-07-italy-heatwave-peaks-cities-red.html (last access: 27 February 2023), 2022.
Qiao L., Zuo, Z., Xiao, D., and Bu, L.: Detection, Attribution, and Future Response of Global Soil Moisture in Summer, Front. Earth Sci., 9, 745185, https://doi.org/10.3389/feart.2021.745185, 2021.
O., S. and Orth, R.: Global soil moisture data derived through machine learning trained with in-situ measurements, Sci. Data, 8, 1–14, https://doi.org/10.1038/s41597-021-00964-1, 2021.
OMSZ: Országos Meteorológiai Szolgálat, Hungarian Meteorological Service: Több melegrekord is megdőlt, https://www.met.hu/omsz/OMSZ_hirek/index.php?id=4849andm=2andhir=Tobb_melegrekord_is_megdolt, (last access: 27 February 2023), 2022.
Philip, S., Kew, S., van Oldenborgh, G. J., Otto, F., Vautard, R., van der Wiel, K., King, A., Lott, F., Arrighi, J., Singh, R., and van Aalst, M.: A protocol for probabilistic extreme event attribution analyses, Adv. Stat. Clim. Meteorol. Oceanogr., 6, 177–203, https://doi.org/10.5194/ascmo-6-177-2020, 2020a.
Philip, S. Y., Kew, S. F., van der Wiel, K.,Wanders, N., and van Oldenborgh, G. J.: Regional differentiation in climate change induced drought trends in the Netherlands, Environ. Res. Lett., 15, 094081, https://doi.org/10.1088/1748-9326/ab97ca, 2020b.
Rakovec, O., Samaniego, L., Hari, V., Markonis, Y., Moravec, V., Thober, S., Hanel, M., and Kumar, R.: The 2018–2020 multi-year drought sets a new benchmark in Europe, Earth's Future, 10, e2021EF002394, https://doi.org/10.1029/2021EF002394, 2022.
Rayner, N. A., Parker, D. E., Horton, E.B., Folland, C. K., Alexander, L. V., Rowell, D. P., Kent, E. C., and Kaplan, A.: Global analyses of sea surface temperature, sea ice and night marine air temperature since the late nineteenth century, J. Geophys. Res.-Atmos., 108, 4407, https://doi.org/10.1029/2002JD002670, 2003.
Reuters: China issues first national drought alert, battles to save crops in extreme heatwave, Shanghai, https://www.reuters.com/world/china/ (last access: 27 February 2023), 2022.
Rocha, P. A.: Heat Wave Is Pushing Europe's Energy System to the Limit, https://www.bloomberg.com/news/articles/2022-07-18/heat-wave-is-pushing-europe-s-energy-system-to-the-limit{#} (last access: 13 March 2023), 2022.
Rodell, M., Houser, P., Jambor, U., Gottschalck, J., Mitchell, K., Meng, C.-J., Arsenault, K., Cosgrove, B., Radakovich, J., Bosilovich, M., Entin, J. K., Walker, J. P., Lohmann, D., and Toll, D.: The global land data assimilation system, Bull. Am. Meteorol. Soc., 85, 381–394, https://doi.org/10.1175/bams-85-3-381, 2004.
Roscoe, M.: Huge fire rages in Massarosa (Lucca) Italy as evacuations continue, https://euroweeklynews.com/2022/07/19/fire-massarosa-lucca-italy/ (last access: 13 March 2023), 2022.
Roucaute, D.: Heat wave 'likely' to have caused over 11,000 additional deaths in France this summer, https://www.lemonde.fr/en/france/article (last access: 13 March 2023), 2022.
Rousi, E., Kornhuber, K., Beobide-Arsuaga, G., Luo, F., and Coumou, D.: Accelerated western European heatwave trends linked to more-persistent double jets over Eurasia, Nat. Commun., 13, 3851, https://doi.org/10.1038/s41467-022-31432-y, 2022.
Save, H., Bettadpur, S., and Tapley, B. D.: High-resolution CSR GRACE RL05 mascons, J. Geophys. Res.-Sol. Ea., 121, 7547–7569, https://doi.org/10.1002/2016jb013007, 2016.
Save, H.: CSR GRACE and GRACE-FO RL06 Mascon Solutions v02, GRACE [data set], https://doi.org/10.15781/cgq9-nh24, 2020.
Schär, C., Vidale, P., Lüthi, D., Frei, C., Häberli, C., Liniger, M. A., and Appenzeller, C.: The role of increasing temperature variability in European summer heatwaves, Nature, 427, 332–336, https://doi.org/10.1038/nature02300, 2004.
Scherrer, S.C., Hirschi, M., Spirig, C., Maurer, F., and Kotlarski. S.: Trends and drivers of recent summer drying in Switzerland, Environ. Res. Commun., 4, 025004, https://doi.org/10.1088/2515-7620/ac4fb9, 2022.
Schumacher, D. L., Zachariah, M., Otto, F., et al.: High temperatures exacerbated by climate change made 2022 Northern Hemisphere soil moisture droughts more likely, World Weather Attribution, https://www.worldweatherattribution.org/ (last access: 27 February 2023), 2022.
Seabrook, V.: Climate crisis: Drought hitting more than half of Europe – these are the consequences, https://news.sky.com/story/ (last access: 27 February 2023), 2022.
Seneviratne, S. I., Lüthi, D., Litschi, M., and Schär, C.: Land–atmosphere coupling and climate change in Europe, Nature, 443, 205–209, https://doi.org/10.1038/nature05095, 2006.
Seneviratne, S. I., Lehner, I., Gurtz, J., Teuling, A. J., Lang, H., Moser, U., Grebner, D., Menzel, L., Schroff, K., Vitvar, T., and Zappa, M.: Swiss prealpine Rietholzbach research catchment and lysimeter: 32 year time series and 2003 drought event, Water Resour. Res., 48, 6, https://doi.org/10.1029/2011WR011749, 2012.
Seneviratne, S. I. and Hauser, M.: Regional climate sensitivity of climate extremes in CMIP6 versus CMIP5 multimodel ensembles, Earth's Future, 8, e2019EF001474, https://doi.org/10.1029/2019EF001474,2020.
Seneviratne, S. I., Zhang, X., Adnan, M., et al.: Weather and Climate Extreme Events in a Changing Climate. In Climate Change 2021: The Physical Science Basis, Contribution of Working Group I to the Sixth Assessment Report of the Intergovernmental Panel on Climate Change, edited by: Masson-Delmotte, V., Zhai, P., Pirani, A., Connors, S. L., Péan, C., Berger, S., Caud, N., Chen, Y., Goldfarb, L., Gomis, M. I., Huang, M., Leitzell, K., Lonnoy, E., Matthews, J. B. R., Maycock, T. K., Waterfield, T., Yelekçi, O., Yu, R., and Zhou, B., Cambridge University Press, Cambridge, United Kingdom and New York, NY, USA, 1513–1766, https://doi.org/10.1017/9781009157896.013, 2021.
Sheffield, J., Goteti, G., and Wood, E. F.: Development of a 50-year high-resolution global dataset of meteorological forcings for land surface modeling, J. Clim., 19, 3088–3111, https://doi.org/10.1175/JCLI3790.1, 2006.
Sheffield, J. and Wood, E. F.: Global Trends and Variability in Soil Moisture and Drought Characteristics, 1950–2000, from Observation-Driven Simulations of the Terrestrial Hydrologic Cycle, J. Clim., 21, 432–458, https://doi.org/10.1175/2007JCLI1822.1, 2008.
Sousa, P.M., Barriopedro, D., García-Herrera, R., Ordóñez, C., Soares, P. M. M., and Trigo, R. M.: Distinct influences of large-scale circulation and regional feedbacks in two exceptional 2019 European heatwaves, Commun. Earth Environ., 1, 48, https://doi.org/10.1038/s43247-020-00048-9, 2020.
Stegehuis, A. I., Vogel, M. M., Vautard, R., Ciais, P., Teuling, A. J., and Seneviratne, S. I.: Early summer soil moisture contribution to western European summer warming, J. Geophys. Res.-Atmos., 126, e2021JD034646, https://doi.org/10.1029/2021JD034646, 2021.
Stresing, L. and Wolf, C.: So trocken ist es aktuell in Deutschland und Europa, https://www.n-tv.de/infografik/ (last access: 13 March 2023), 2022.
Taylor, K. E., Stouffer, R. J., and Meehl, G. A.: An overview of CMIP5 and the experiment design, Bull. Am. Meteorol. Soc., 93, 485–498, https://doi.org/10.1175/BAMS-D-11-00094.1, 2012.
Teuling, A. J., Van Loon, A. F., Seneviratne, S. I., Lehner, I., Aubinet, M., Heinesch, B., Bernhofer, C., Grünwald, T., Prasse, H., and Spank, U.: Evapotranspiration amplifies European summer drought, Geophys. Res. Lett., 40, 2071–2075, https://doi.org/10.1002/grl.50495, 2013.
The Local: Italian wildfires “three times worse” than average as heatwave continues, https://www.thelocal.it/20220627/ (last access: 13 March 2023), 2022.
Toreti, A., Bavera, D., Acosta Navarro, Jager, A. de, Di Ciollo, C., Maetens, W., Magni, D., Masante, D., Mazzeschi, M., Spinoni, J., Niemeyer, S., Cammalleri, C., and Hrast Essenfelder, A.: Drought in Europe August 2022, Publications Office of the European Union, Luxembourg, JRC130493, https://doi.org/10.2760/264241, 2022.
Trnka, M., Brázdil, R., Možný, M., Štěpánek, P., Dobrovolný, P., Zahradníček, P., Balek, J., Semerádová, D., Dubrovský, M., Hlavinka, P., Eitzinger, J., Wardlow, B., Svoboda, M., Hayes, M., and Žalud, Z.: Soil moisture trends in the Czech Republic between 1961 and 2012, Int. J. Climatol., 35, 3733–3747, https://doi.org/10.1002/joc.4242, 2015.
Twoja Pogoda: Najpierw w czerwcu, a teraz w lipcu takiego żaru jeszcze nie było. Nawet 38 stopni. Gdzie było najgoręcej (“First in June and now in July, this heat is unprecedented. Up to 38 ∘C. Where was it hottest?”), https://www.twojapogoda.pl/wiadomosc/2022-07-02/ (last access: 27 February 2023), 2022.
van Oldenborgh, G. J., van der Wiel, K., Kew, S., Philip, S., Otto, F., Vautard, R., King, A., Lott, F., Arrighi, J., Singh, R., and van Aalst, M.: Pathways and pitfalls in extreme event attribution, Climatic Change, 166, 13, https://doi.org/10.1007/s10584-021-03071-7, 2021.
Vautard, R., Yiou, P., D'Andrea, F., de Noblet, N., Viovy, N., Cassou, C., Polcher, J., Ciais, P., Kageyama, M., and Fan, Y.: Summertime European heat and drought waves induced by wintertime Mediterranean rainfall deficit, Geophys. Res. Lett., 34, L07711, https://doi.org/10.1029/2006GL028001, 2007.
Vecchi, G. A., Delworth, T., Gudgel, R., Kapnick, S., Rosati, A., Wittenberg, A. T., Zeng, F., Anderson, W., Balaji, V., Dixon, K., Jia, L., Kim, H.-S., Krishnamurthy, L., Msadek, R., Stern, W. F., Underwood, S. D., Villarini, G., Yang, X., and Zhang, S.: On the seasonal forecasting of regional tropical cyclone activity, J. Clim., 27, 7994–8016, https://doi.org/10.1175/JCLI-D-14-00158.1, 2014.
Virtanen, P., Gommers, R., Oliphant, T. E., Haberland, M., et al.: SciPy 1.0: Fundamental Algorithms for Scientific Computing in Python, Nat. Method., 17, 261–272, https://doi.org/10.1038/s41592-019-0686-2, 2020.
Wang, Z., Chang, C.-P., and Wang, B.: Impacts of El Niño and La Niña on the U.S. Climate during Northern Summer, J. Clim., 20, 2165–2177, https://doi.org/10.1175/JCLI4118.1, 2007.
Wang, Y., Luo, Y., and Shafeeque, M.: Using a Gaussian function to describe the seasonal courses of monthly precipitation and potential evapotranspiration across the Yellow River Basin, China, J. Hydrometeorol., 20, 2185–2201, https://doi.org/10.1175/jhm-d-19-0019.1, 2019.
Wang, A.: for CareOurEarth: Record-Breaking Heatwaves Around the World In 2022, https://www.careourearth.com/record-breaking-heatwaves-around-the-world-in-2022/ (last access: 27 February 2023), 2022.
Wartenburger, R., Hirschi, M., Donat, M. G., Greve, P., Pitman, A. J., and Seneviratne, S. I.: Changes in regional climate extremes as a function of global mean temperature: an interactive plotting framework, Geosci. Model Dev., 10, 3609–3634, https://doi.org/10.5194/gmd-10-3609-2017, 2017.
Wieder, W. R., Kennedy, D., Lehner, F., Musselman, K. N., Rodgers, K. B., Rosenbloom, N., Simpson, I. R., and Yamaguchi, R.: Pervasive alterations to snow-dominated ecosystem functions under climate change, P. Natl. Acad. Sci. USA, 19, e2202393119, https://doi.org/10.1073/pnas.2202393119, 2022.
Wild, M., Gilgen, H., Roesch, A., Ohmura, A., Long, C. N., Dutton, E. G., Forgan, B., Kallis, A., Russak, V., and Tsvetkov, A.: From dimming to brightening: Decadal changes in solar radiation at earth's surface, Science, 308, 847–850, https://doi.org/10.1126/science.1103215, 2005.
Wilhite, D. A., Svoboda, M. D., and Hayes, M. J.: Understanding the Complex Impacts of Drought: A Key to Enhancing Drought Mitigation and Preparedness, Water Resour. Manag., 21, 763–774, https://doi.org/10.1007/s11269-006-9076-5, 2007.
Yang, W., Hsieh, T.-L., and Vecchi, G. A.: Hurricane annual cycle controlled by both seeds and genesis probability, P. Natl. Acad. Sci. USA, 118, e2108397118, https://doi.org/10.1073/pnas.2108397118, 2021.
Zachariah, M., Vautard, R., Schumacher, D. L., et al.: Without human-caused climate change temperatures of 40 ∘C in the UK would have been extremely unlikely, World Weather Attribution, https://www.worldweatherattribution.org/wp-content/uploads/UK-heat-scientific-report.pdf (last access: 27 February 2023), 2022.
Zampieri, M., D'Andrea, F., Vautard, R., Ciais, P., de Noblet-Ducoudré, N., and Yiou, P.: Hot European Summers and the Role of Soil Moisture in the Propagation of Mediterranean Drought, J. Clim., 22, 4747–4758, https://doi.org/10.1175/2009JCLI2568.1, 2009.
- Abstract
- Introduction
- Event description and associated impacts
- Data and methods
- Observation-based analysis
- Hazard synthesis using observation-based datasets and models
- Conclusions
- Code availability
- Data availability
- Author contributions
- Competing interests
- Disclaimer
- Acknowledgements
- Financial support
- Review statement
- References
- Supplement
- Abstract
- Introduction
- Event description and associated impacts
- Data and methods
- Observation-based analysis
- Hazard synthesis using observation-based datasets and models
- Conclusions
- Code availability
- Data availability
- Author contributions
- Competing interests
- Disclaimer
- Acknowledgements
- Financial support
- Review statement
- References
- Supplement