the Creative Commons Attribution 4.0 License.
the Creative Commons Attribution 4.0 License.
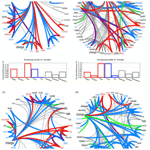
Disentangling the climate divide with emotional patterns: a network-based mindset reconstruction approach
Roger Cremades
Massimo Stella
Extreme political populism has been fiercely spreading climate disinformation for years, contributing to a social divide about climate change. In order to profile how both sides of the climate divide communicate climate change, we collected dissemination materials and analysed the mindset of key actors reaching global audiences. We apply network science to textual content in order to reconstruct and analyse the mindsets of key actors across the climate divide. Here, we show that the emerging mindsets support the identification of emotional patterns – such as hypercritical scepticism masking falsehoods under a trustful promotion of change – linked to a quick and pervasive spread of falsehoods, i.e. an infodemic. We find that, in climate change disinformation, the word “climate” represents a fearsome threat linked to inconsistent science. We show that the word “change” represents a reassuring pattern in climate disinformation, characterised by trust and by low anticipation without risk awareness, except for some fear about policy changes. For climate activism, the word “change” is linked to high levels of negative emotions like anger, disgust, and fear related to a perception of existential threats. Furthermore, the word “children” represents an angering concern in climate disinformation, while climate change activism perceives “children” with trust and joy but also sadness for their anticipated future. Mindset reconstruction has the potential to become a relevant tool for identifying and flagging communication materials linked to disinformation, which amplifies the climate divide and facilitates infodemics.
- Article
(11014 KB) - Full-text XML
- BibTeX
- EndNote
The Intergovernmental Panel on Climate Change (IPCC) affirms that continued climate change is directly impacting human lives and that the risks of injury, disease, and death increase with heat waves, floods, droughts, and fires (Smith et al., 2014). However, contrasting messages from extreme political populism have been fiercely spreading climate disinformation through social and news media for years (Demelle, 2016; Horton, 2020; Watts et al., 2019).
Climate-denying political leaders across world regions – USA, Brazil, Australia, the Netherlands – are just visible elements of an evolving list of hundreds of influential players and think tanks (Desmog, 2021). These think tanks repeatedly appear to be linked to events where influencers take climate-denying positions (YouTube, 2019); often, these events run in parallel to the Conference of the Parties (COP) of the United Nations Climate Change Framework Convention (UNFCCC). The annual COP is the most important climate policy event worldwide. When searching for information about these UNFCCC COP events, content intended to trigger a quick and pervasive spread of falsehoods – i.e. an infodemic – through events organised in parallel by climate disinformation think tanks shows up in multiple media channels, including in prominent video-sharing platforms (see Sect. 2).
These actors and think tanks have been polarising the worldwide public opinion for decades, amplifying the climate divide (Hoffman, 2011; Horton, 2020). On one side of the climate divide, climate change disinformation actively impedes “social consensus” about climate change. Climate change disinformation actors (hereafter referred to as climate disinformation) disseminate misleading information and downplay scientific evidence with the support of politically entrenched think tanks (Demelle, 2016; Desmog, 2021; Horton, 2020).
On the other side of the climate divide, science-based climate change activism (hereafter referred to as climate activism) demands action from policy makers while stressing the importance of climate science in society (Hoffman, 2011; Marris, 2019). While environmental and climate activists are not a novelty, and while cohorts of teenagers and students have been involved in the decarbonisation of UK and US universities since at least 2010 (Healy and Debski, 2017), recently the #FridaysForFuture movement gained unprecedented prominence, demanding climate action from political leaders. The #FridaysForFuture movement adheres to scientific consensus on climate change and has been gathering remarkable media attention since 2019.
Social movements like #FridaysForFuture have been pointed out as instrumental for crossing a tipping point toward major changes of social norms and values that could contribute to stabilising Earth's climate (Otto et al., 2020). Information flows and the feedbacks they might generate are amongst the most important interventions for stabilising Earth's climate (Otto et al., 2020). The fear of information flows and their related feedbacks activating social tipping dynamics towards decarbonisation by certain think tanks provides a possible explanation for their interest in a climate emergency infodemic and polarisation agenda.
The variety of actors involved in the climate divide is immense, and it is fully unclear what underlying patterns could characterise the messages in both sides of this divide. In this context, we structure our investigation as a comparison between key representatives in their ranks, i.e. individuals with outstanding character that managed to exhibit leadership in a history of world-spanning events reaching millions of individuals.
To elaborate on overarching strategies and to understand the validity of proposals for tools dealing with the climate divide, it is fundamental to explore the emotions inflaming this battle of ideas and to uncover weaknesses in the mindset embedded in the communication strategy of those involved (Hoffman, 2011). The communication materials of individuals involved in the climate divide can be expected to exhibit patterns leading to the identification of inflammatory media content. Semantic patterns can be used to unveil emotionally distorted content linked to polarisation (Stella et al., 2018; Stella, 2020).
In this article, we aim to explore the emotional dimension of climate communication as it is linked to the climate divide. Departing from this aim, we have specified the following objectives: first, to explore how the mindsets of key representatives of #FridaysForFuture and of climate-denying think tanks differ when communicating about climate; second, to unveil emotionally distorted content linked to polarisation in key climate disinformation communication events; and third, to provide a scientific basis for unveiling the disinformation content that drives the climate emergency infodemic.
The conceptual and methodological innovations in this article have an exploratory character. Mindset reconstruction exposes the emotional backbone of language, i.e. how words eliciting different emotions are syntactically and semantically linked in language (Stella, 2020; Stella et al., 2018). In order to profile how both sides of the divide communicate “climate change”, we collected communication materials related to climate change and analysed the mindsets of selected actors who have been able to reach global audiences. The methodology is divided into three consecutive steps: (i) identification of key figures globally influencing the climate divide, (ii) data collection, and (iii) application of network science methods for mindset reconstruction and visual representation of the results. The proposed methodology contributes to formalising data-driven approaches in the human dimension of global change, particularly regarding the social and opinion dynamics of the climate divide.
2.1 Identification of key figures
The identification of key figures is based on criteria of leadership and of a history of contribution to global events in the respective networks of #FridaysForFuture and of climate-denying think tanks. This has been a difficult task, because while there are prominent figures on both sides of the climate divide, very few have a truly remarkable history of contribution to international events. Demelle (2016) and Desmog (2021) have been instrumental sources for evaluating climate deniers.
Greta Thunberg can be traced as the originator of the #FridaysForFuture. After her innovative way of demonstrating gained prominence, her initially single-student protest gained scale and lead to a global school strike movement. Afterwards, she gave speeches in many global centres of power and met with multiple global leaders. At the time of writing this article, she is perhaps the only globally mediatic figure of this movement.
Christopher Monckton was ranked by Demelle (2016) as one of the top 10 climate deniers, and Desmog (2021) mentions him in the context of multiple climate-related events and actions spanning across world regions for more than a decade.
2.2 Data collection
Data originates from key public speeches directly or incidentally linked to international bodies, national institutions, and diplomacy hubs – for example, a COP of the UNFCCC, the UN, the World Economic Forum at Davos, the UK parliament, or climate disinformation conferences.
The selected key public speeches reached broad audiences beyond the auditorium and have been disseminated by multiple media channels, including television, newspapers, and video-sharing platforms like YouTube (YouTube, 2019). In particular, we selected 11 public speeches made by Greta Thunberg from 2018 to 2020 as well as three much larger speeches made by Christopher Monckton in 2019 at events organised in Madrid in parallel to UNFCCC's COP 25 and at a climate disinformation conference in Washington. Thunberg's speeches included a total of 600 sentences and 9168 words, whereas Monckton's speeches included a total of 568 sentence and 15 178 words. The word counts here take into consideration repetitions and do not include lemmatisation, which is rather performed within the construction of forma mentis networks.
By using text from public speeches, we overcome the difficulties of preserving the privacy of under-age citizens that are a known part of the #FridaysForFuture movement (Marris, 2019).
2.3 From words to mindset reconstruction with forma mentis networks
The mental lexicon is an idealised system that acquires, stores, processes, and produces language (Vitevitch, 2019). The mental lexicon represents the structure of conceptual associations in language, as used by each individual. As a purely cognitive system, the mental structure of conceptual associations in the lexicon can be extracted and analysed from communication materials under the assumption of the individual's authorship. Communication materials like texts are an open view of the mindset of the authors, which is a proxy for the structure of language and its associations in the human mind. For instance, Teixeira et al. (2021) reconstructed associations in suicide letters to assess how suicide ideation altered perceptions of concepts like “life” and “love” in comparison to healthy individuals.
Forma mentis networks are a representation of the emotional content of the mental lexicon and the relations between the meanings involved (Stella, 2020). We use forma mentis networks to show how an individual person conceptually and emotionally structures their mindset about climate change. Mindset reconstruction with forma mentis networks exposes the emotional backbone of language, and such exposure highlights the attitudes towards “climate change” fuelling the climate divide (Fig. 1, Lexicon 1).
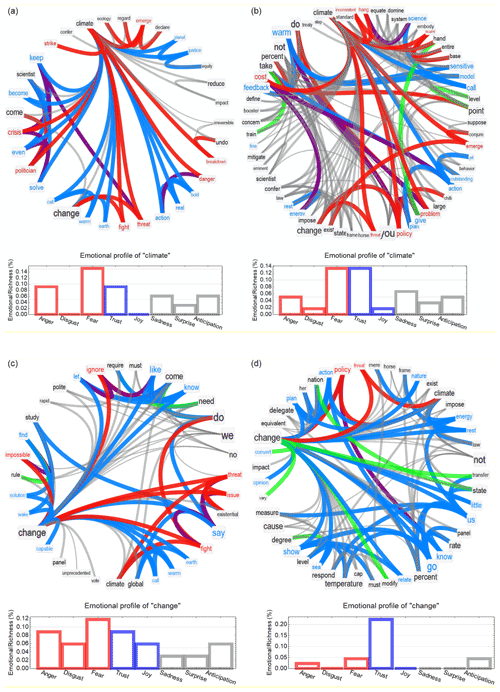
Figure 1Speakers' mindset reconstruction around “climate” (a, b) and “change” (c, d) in the speeches of Greta Thunberg (a, c) and Christopher Monckton (b, d). Links indicate syntactic and semantic relationships between words in speeches. Links are coloured if linking at least a positive, negative, or neutral word or synonyms (blue, red, grey, green). Blue, red, and black (positive, negative, and neutral) words indicate how they are perceived in language according to the NRC Emotion Lexicon (see Sect. 2). Font size expresses the relative importance of the words, reflecting their centrality in the speeches. Emotions are self-explanatory, except for anticipation, which is a projection into future expectations (see Stella, 2020). We refer the reader to Lexicon 1 for an interpretation of the figure.
To build the forma mentis networks, syntactic networks are used as a proxy of the mental lexicon. Relations between words come from syntactic and semantic dependencies in speeches and written text. Syntactic dependencies specify features or meanings of words. For instance, in “the pen is on the table”, the syntactic relationship “pen”–“table” specifies the location of the word “pen”. In textual forma mentis networks (TFMNs), as implemented here and in Stella (2020), syntactic links between words are detected through artificial intelligence (AI) rather than by human intervention. In this work, the AI performing syntactic parsing is a multilayer perceptron, i.e. a neural network architecture where different layers of nodes perform computations iteratively and can learn to predict specific output based on extensive input. Chen and Manning (2014) trained a multilayer perceptron with three layers to identify syntactic relationships in English from a dataset with 39 000 sentences. The AI achieved an accuracy of 92 % in correctly assessing whether two words were syntactically linked or not. In a single sentence, once retrieved, syntactic links create a tree graph T, where words are nodes and where links indicate syntactic dependencies – e.g. in “the pen is on the table”, “on” depends on “the”, and they are thus linked. Directly considering these trees would be problematic, since grammatical rules for stop-words (i.e. prepositions and articles) would automatically make the latter largely connected nodes, e.g. “the” will appear more frequently in sentences and thus get more connections. To address this issue, we build new syntactic links between all pairs of non-stop-words on T if they are separated by, at most, K=4 syntactic dependencies. This approach leads to networks of non-stop-words clustered by local syntactic dependencies. To reduce language variability, we also lemmatise words with WordNet (Miller, 1995); e.g. “pens” and “pen” in the text are represented by a single “pen” node. We enrich TFMNs semantically by considering semantic relationships indicating overlaps in meaning, i.e. synonyms, as extracted from WordNet 3.0 (Miller, 1995). Nodes or words in TFMNs can thus be connected syntactically and semantically. Words are also attributed psycholinguistic labels expressing valence or pleasantness. A single word can be identified as “positive”, “negative”, or “neutral”, as indicated by human raters involved in a psychological mega-study (see Stella, 2020). Links are treated as undirected and unweighted. Only for visualisation purposes, links between any two neutral words appearing more than once are highlighted in thicker grey lines. Links involving one positive (negative) word are highlighted in cyan (red). Links between one positive word and one negative word are highlighted in purple. Green links indicate synonyms.
Notice that syntactic parsing is different from considering word co-occurrences. In the example “climate change is a terrible, catastrophic, problematic, crucial issue”, the words “change” and “issue” are evidently syntactically related, but they are neither adjacent nor close in the layout of the sentence. Syntactic parsing and our TFMNs would thus link these words, unlike a word co-occurrence network of adjacent words (i.e. where links would be between “climate” and “change”, “change” and “is”, “is” and “a”, etc.).
TFMNs represent syntactic and semantic networks of words labelled on an affective level. These networks encode the structure of associative knowledge, expressed through semantic and syntactic word associations in one or more texts. Stella (2020) showed that, in labelled data, this network construction successfully identifies keywords in tagged texts. Investigating the structure of TFMNs can thus be informative about ways of associating ideas and structuring emotional stances. Here, we investigated TFMNs by focusing on network neighbourhoods, which are interpreted as semantic frames providing contextual information, i.e. the set of words that were syntactically and/or semantically associated with a target word to specify the meaning of the latter. In “the pen is on the table”, the neighbourhood of “pen” would be “table”, specifying the location of the “pen” itself. According to frame semantics in cognitive science (Fillmore and Baker, 2001), the meaning attributed to a target word in a text can be reconstructed by considering its syntactic, semantic, and emotional associations. Focusing on direct associations, i.e. at distance one from a given target, network neighbourhoods encode contextual knowledge that indicates how the same concept (e.g. represented by the word “failure”) can be framed in different ways within various narratives (e.g. “failure is a disappointing experience” vs. “failure is a learning opportunity”). TFMNs automatise the identification of semantic frames in texts as network neighbourhoods or, in other words, as ego-centred networks of radius 1 (Newman, 2003), surrounding a target word or idea. Reconstructing these neighbourhoods enables a quantitative understanding of how concepts were framed in texts. This approach has been used for texts of varying sizes, including for suicide notes of about 120 words (Teixeira et al., 2021), where “love” was found to be framed with considerably sadder jargon compared to reference associations to “love” provided by mentally healthy individuals.
Emotions populating a given semantic frame are computed through the NRC Emotion Lexicon (Mohammad and Turney, 2013), which is a large-scale lexicon mapping 14,000 English words to 8 emotional states, like fear, anger, joy, anticipation, sadness, trust, surprise, and disgust, which go far beyond simple positive and negative sentiment polarities. Emotional profiling is performed through counting operations. In a given semantic frame or neighbourhood, let L be the list of words eliciting at least one emotion according to the NRC Emotion Lexicon. The emotional richness r(e) is then defined as the number of words in L that elicit emotion e, normalised by the neighbourhood size. Emotional richness r(e) thus defines the probability of finding one word that elicits a given emotion by sampling uniformly and at random a single word in a specific semantic frame surrounding a target idea or concept.
Notice that network construction and visualisation were both performed within Mathematica 11.3. Network construction adopted the commands TextStructure[] (syntactic parsing) and WordData[] (lemmatisation, deletion of articles and prepositions) – see, for reference, https://www.wolfram.com/language/11/text-and-language-processing/explore-the-structure-of-texts.html?product=language (last access: 19 July 2022). Network visualisation adopted a hierarchical edge bundling clustering, placing nodes on a circular embedding while grouping clusters of links together (see Holten, 2006).
The words in the forma mentis networks also identify their key concepts in the analysed speeches with the size of the words (see Fig. 1); larger words were represented as possessing a higher closeness centrality in the speeches (see Eq. 1). Closeness centrality is defined as the inverse average distance between a word and all its neighbours in the full network (Metcalf and Casey, 2016). A previous study (Stella, 2020) showed that closeness centrality is able to identify the prominent concepts of short texts, i.e. the main words that provide grounding to a short narrative. This motivates our choice to use closeness centrality as an estimator for concept prominence in texts. Equation (1) is used for calculating the closeness centrality (Metcalf and Casey, 2016) of each concept:
where C is the closeness centrality for each node in the graph G – in this case, a network made of words from speeches and written text, where links indicate syntactic (e.g. “pen”–“table” in the sentence “the pen is on the table”) and synonym relationships (e.g. “nice” and “good” overlap in meaning in the sentence “you are nice and good”). G is the whole network, which includes words (nodes) and semantic and syntactic links as extracted from all sentences in a speech or text. v is the node in network G, which in our case is a word in a speech or written text; the closeness centrality is computed for this v node. w represents any other node in network G. N is the number of nodes in network G. d is the shortest path network distance, i.e. the smallest number of links between nodes (words) v and w in the graph G.
As detailed in the Methodology above, mindset reconstruction exposes the emotional backbone of language (Stella et al., 2018; Stella 2020). Such exposure importantly allows one to highlight the attitudes towards “climate change” that fuel the climate divide. In order to profile how both sides of the divide perceive “climate change”, we illustrate their emotional and semantic patterns in Figs. 1-4 and Lexicon 1, accompanied by Figs. A1–A12. Overall, here, we show that speeches in climate activism rely mostly on trust and hope with links to anger, while climate disinformation shows clear patterns of hypercritical misinformation masked under trust-inspiring content.
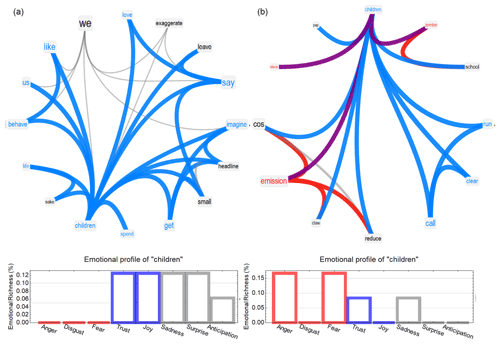
Figure 2Speakers' mindset reconstruction around “Children” in the speeches of Greta Thunberg (a) and Christopher Monckton (b). We refer the reader to Fig. 1 for a detailed explanation of the colour code and to Lexicon 1 for an interpretation of the figure.
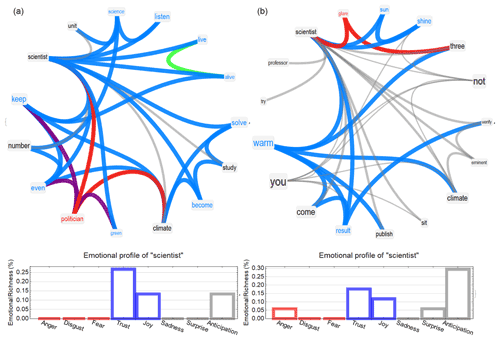
Figure 3Speakers' mindset reconstruction around “Scientist” in the speeches of Greta Thunberg (a) and Christopher Monckton (b). We refer the reader to Fig. 1 for a detailed explanation of the colour code and to Lexicon 1 for an interpretation of the figure.
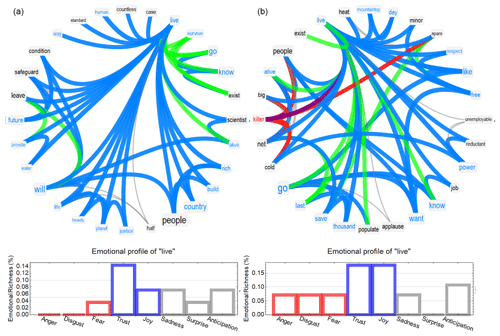
Figure 4Speakers' mindset reconstruction around “live” in the speeches of Greta Thunberg (a) and Christopher Monckton (b). We refer the reader to Fig. 1 for a detailed explanation of the colour code and to Lexicon 1 for an interpretation of the figure.
Lexicon 1: A lexicon of the climate divide, with the associated emotions on both sides.
-
Action. For climate activism, it means hope for a better future, one much wanted and needed; it is propositional, toward the elicitation of a revolution-like call to action. For climate disinformation, it is just a sad, bureaucratic cost – although something positive, it does not lead to any practical safeguarding initiative (Figs. A5 and A10).
-
Believe. Climate disinformation angrily believes that there is scarce contradictory evidence, while climate activism's beliefs are strongly propositional about setting goals to avoid the danger of inaction (Fig. A6).
-
Change. For climate disinformation, there is a pattern characterised by trust and low anticipation without risk awareness; overall, it is a perception of “change” that is reassuring – there is no sense of threat, no problem at all, except for some fear about policy changes. For climate activism, change is linked to high levels of negative emotions like anger, disgust, and fear, which are related to a perception of existential threats (Fig. 1).
-
Children. This is an angering concern for climate disinformation. Climate activism perceives children with trust and joy but also sadness for their anticipated future (Fig. 2).
-
Climate. This is a fearsome threat that is linked to inconsistent science for climate disinformation and to scary tipping points for climate activism (Fig. 1).
-
Future. This is relatively absent in climate disinformation. On the other hand, it inspires trust linked to future awareness in climate activism (Fig. A8).
-
Ignore. This is a large and central concept for climate activism; it is counterfactually associated with trust that people will come to make change happen. “Ignore” is only peripheral for climate disinformation and is linked to trust regarding the potential profits of global warming (Fig. A7).
-
Leader. This refers to someone to trust and follow in climate disinformation. However, in climate activism, it is someone who triggers anger, as linked to “politicians” and “emissions”, while still inspiring trust (Fig. A9).
-
Live. Climate activism uses this term carefully, associating “live” with trusting in the conditions of human survival and planetary justice, while climate disinformation does not display a coherent pattern (Fig. 4).
-
Number. Climate activism stays positive and lacks objections to numbers coming from current science, while climate disinformation displays an opposite pattern of strong anxiety, projecting into the future a sense of exaggerated imbalance in terms of the issues at hand (Fig. A11).
-
Science. This inspires mostly negative emotions of anger, disgust, and fear in climate disinformation. On the other hand, it is a matter of trust associated with listening and numbers for climate activists (Fig. A1).
-
Scientist. They are viewed as isolated prophets that provide facts for narratives of climate disinformation, specifically around changes in solar radiation, and are a source of anticipation. Contrastingly, for climate activism, they are people that politicians need to listen to, experts that solve problems (Fig. 3).
In their promoted mindsets, climate disinformation resorts to a wide variety of trust-related semantic associations, reducing scientists to isolated prophets that provide alternative facts, which they relate to disinformation attempts to convince the public with alternative scientific evidence on global warming. Despite presenting alternative facts, negative emotional associations with “climate” – such as “hysteria” and “catastrophe” – are only present in climate disinformation, while climate activism gives more relevance to “breakdown”, “danger”, and “threat” (Fig. A3).
Anticipation, which is a projection into the future and is characterised by both anxiety and excitement, is a stronger emotion for climate activism, specifically concerning concepts of “leadership”, “listen” (Fig. A2), “children”, and “threat”. Climate disinformation concentrates anticipation toward “studies” and “numbers” due to the anxiety that scientific facts create within the climate disinformation community. The emotion of surprise is linked to “children” and “future” (Fig. A8) for climate activism, while climate disinformation associates it to the “numbers” behind climate science. Sadness is very strong in the climate activism arena for concepts like “children”, “action”, or “believe” and appears also to be linked to “future”, “climate”, “leader”, and “live”.
Climate disinformation displays high levels of sadness only around the term “believe”. Joy is counterfactually high for terms like “children” and “action” in climate activism, which can be explained by the emotions of hope and the sense of belonging to a growing group (Lerner et al., 2015).
Trust, an emotion strongly used by outstanding visionary leaders (Mumford, 2006), is consistently high for climate activists, with very high values associated with its science-based grounds. Instead, climate disinformation projects trust towards future-centred terms like “change”, “live”, and “study” (Fig. A12), linked to reports with alternative facts from their own dissemination activities.
Fear is higher for terms like “climate change”, “threat”, “issue” (Fig. A4), and “believe” in climate activism, while for climate disinformation fear appears to be very intense in relation to “children”. Anger is again linked to “children” and also to “believe” in climate disinformation, while for climate activism anger is associated with “climate change” and “leader”. Last but not least, disgust appears to be linked to how much both sides “ignore” each other.
Figure 1 (top left) illustrates that climate activism perceives “climate” as being overwhelmed by the threat of climate breakdown, whereas climate disinformation associates “climate” with neutral concepts expressing “inconsistent science” (top right). Such a dichotomy reverberates in the mental construct of “change”, a neutral concept by itself in common language. In climate activism, “change” is associated with concepts that strongly elicit anger and fear but also trust, an emotion identifiable in relation to outstanding visionary leaders (Mumford, 2006). Climate activism gives relevance to “breakdown”, “danger”, and “threat”, concepts characterising charismatic, value-based mindsets (Mumford, 2006) and revolutionary speeches (Jasper, 2011; Kramer et al., 2014). Stunningly, in climate disinformation, such a threatened perception was completely absent (Fig. 1, bottom left) and left space for a wide variety of trust-evoking associations with regard to attempts to convince the public with alternative facts on global warming.
Climate activism combines anger (towards inaction), fear (of an approaching threat), and trust (in solving this crisis) and perceives “climate change” as an indispensable “call-to-action” fight. This “call-to-action” is urgently motivated by a combination of emotions: anger against political leaders, fear about the dangers of inaction and against existential climate threats, disgust about a stolen future, and an overall ambition against climate change. This “call-to-action” renders climate activism's mindset entwined with revolutionary emotions. In fact, emotions like anger, hope, and despair are well known for accelerating the social tipping dynamics of large-scale social protests and revolutions (Jasper, 2011).
Furthermore, it is known that outstanding future-focused leaders, often promoters of such revolutions, rely on emotional styles revolving around trust, joy, and anticipation (Mumford, 2006); detecting these emotions in a future-oriented topic like climate change can provide insights into how charismatic #FridaysForFuture can be. Cognitive and semantic contagion require conscious information processing – e.g. interpretation and acceptance – whereas emotional contagion can lead to a faster transfer of moods among people, involving both implicit and explicit mechanisms (Kramer et al., 2014). Positive emotions like trust and joy have been reported to cause a “ripple effect”, i.e. a “pandemic” or “tsunami” of massive contagion of positive sentiment that drives the social behaviour of the whole collective in synchrony (Barsade, 2002). In other words, the emotions and perceptions linked to climate activism have been described as rippling better through society and thus reaching larger social audiences (Jasper, 2011; Mumford, 2006) in comparison to the emotional profile adopted by climate disinformation.
In fact, conceptual associations and emotions indicate that climate disinformation promotes hypercritical scepticism that hides under a generally trustful promotion of change and includes (i) discussing numbers in terms of imbalanced exaggerations; (ii) referring to scientists in a stereotypical way, i.e. isolated individuals that attempt to provide abstract, theoretical evidence to climate disinformation; (iii) displaying negative emotions towards children; and (iv) being fearful of public policy interventions. These hypercritical attitudes clash with the communication style of the #FridaysForFuture movement, which Marris (2019) describes as projecting greater moral integrity due to a lack of immediate vested interests.
As reported in the semantic–emotional analysis around other concepts (see Lexicon 1), climate disinformation displays high levels of sadness only around the term “believe”. Joy is counterfactually high for terms like “children” and “action” (Fig. A5) in climate activism, which can be explained by the emotions of hope and the sense of belonging to a growing group (Lerner et al., 2015).
These hypercritical attitudes disrupt public awareness of the climate emergency and compromise public consensus in stabilising Earth's climate (Bloodhart et al., 2019). They prevent policy makers from acting against the risks posed by climate change (Hoffman, 2011; Watts et al., 2019). Thus, they obstruct the Paris Agreement and the formation of foreseen social tipping dynamics towards decarbonisation (Otto et al., 2020).
We have shown that applying network science to textual content and analysing the emerging mindset can support research about infodemics, i.e. the quick and pervasive spread of falsehoods. We have identified the emotional patterns of disinformation, such as hypercritical scepticism masked by a trustful promotion of change. The reconstructed mindsets and the emotional patterns identified provide new pointers on climate disinformation.
Climate disinformation sustains a chain reaction that triggers a major divide at the global scale, which threats sustainability, human health, and ultimately the global economy (Hoffman, 2011). Infodemics strongly depend on their emotional and perceptual content, much like viruses spreading across populations according to their genetic information. Recent studies highlighted how contagions of distorted perceptions and misinformation greatly influence human responses to the climate threat (Bloodhart et al., 2019).
Emotions and their contagion, much like a pathogen spreading over societies (Kramer et al., 2014), have been instrumental in large-scale societal changes like revolutions – from Maoist China to Nicaragua and Czechoslovakia (Jasper, 2011) – and are instrumental in the emergence of charismatic social and political leaders (Mumford, 2006). Nevertheless, the parallelism in the emotional patterns of a revolution could just be anecdotal. As a matter of fact, the call to action by #FridaysForFuture is limited to policy making. And objectively, the movement often finds a “glass ceiling” with regards to how they could trigger change beyond their demonstrations and judicial actions (Luisa-Marie Neubauer, personal communication, 2019).
Tracing this emotional parallelism with massive social movements is important, because recent calls to civil disobedience by leading climate diplomats (Figueres and Rivett-Carnac, 2020) could create game-changing developments if related to large-scale emotional contagions but could also be hindered by disinformation. These interactions between propelling and hindering factors point us towards future work on the opinion dynamics of the climate divide, both within and between sides.
Despite the amount of meaning found in the results and the showcased pointers for identifying misinformation via emotions, a more detailed analysis focussing on a larger set of relevant leaders by world region – including more subjects from a diversity of geographies – would improve the depth of the insights and their potential for representativeness.
In addition, given the recent converging evidence of positive emotions fostering engagement with policies tackling climate change (Schneider et al., 2021), the methods outlined in here might have a significant impact in terms of detecting positive affect and emotions in next-generation communication efforts aiming to rally actions in response to the climate emergency. Nevertheless, the availability of emotional dictionaries is often limited to the English language, which sets a barrier when working on other languages.
We conclude that mindset reconstruction could be an important tool to deal with disinformation communication materials facilitating the climate divide. Mindset reconstruction of textual content provides a scientific basis for detecting climate-related hypercritical attitudes and fuelling discourses. Hence, mindset reconstruction could help to design strategies that narrow the climate divide by countering infodemics in climate-related communication. The innovative techniques we have shown – at the fringe of AI and cognitive science – could support climate policy in multiple ways, like (i) flagging online communication materials containing conceptual associations that are distorted by disinformation content (Hills, 2019), (ii) highlighting key sources of emotions commonly adopted by supporters of the climate divide, complementing recent human coding approaches to emotion detection in the climate change debate (Hahnel et al., 2020), and (iii) measuring levels of trust in the specific semantic frames surrounding large institutions and expressed in massive social media debates about climate change (Marris, 2019). Further work includes the automated training of cognitive tools for in vivo flagging of online disinformation content in several languages and the study of their influence on the opinion dynamics of pro-active climate debates.
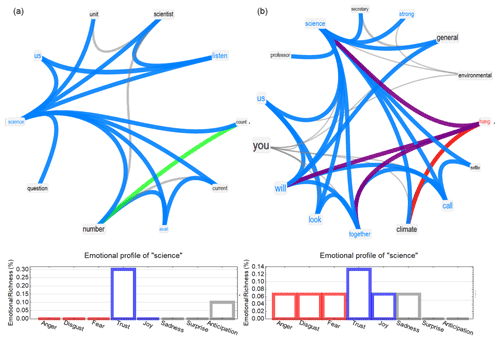
Figure A1Speakers' mindset reconstruction around “Science” in the speeches of Greta Thunberg (a) and Christopher Monckton (b). We refer the reader to Fig. 1 for a detailed explanation of the colour code and to Lexicon 1 for an interpretation of the figure.
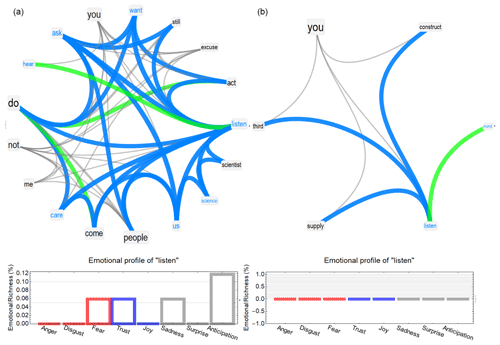
Figure A2Speakers' mindset reconstruction around “listen” in the speeches of Greta Thunberg (a) and Christopher Monckton (b). We refer the reader to Fig. 1 for a detailed explanation of the colour code and to Lexicon 1 for an interpretation of the figure.
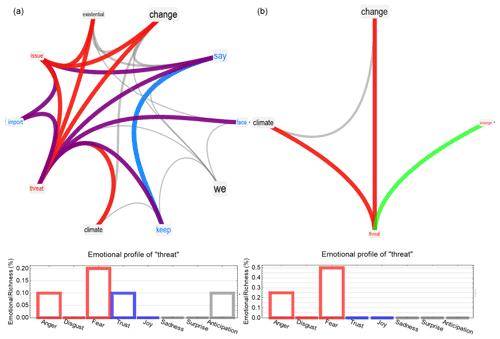
Figure A3Speakers' mindset reconstruction around “threat” in the speeches of Greta Thunberg (a) and Christopher Monckton (b). We refer the reader to Fig. 1 for a detailed explanation of the colour code and to Lexicon 1 for an interpretation of the figure.
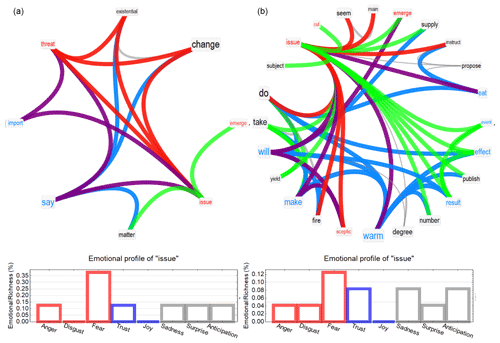
Figure A4Speakers' mindset reconstruction around “issue” in the speeches of Greta Thunberg (a) and Christopher Monckton (b). We refer the reader to Fig. 1 for a detailed explanation of the colour code and to Lexicon 1 for an interpretation of the figure.
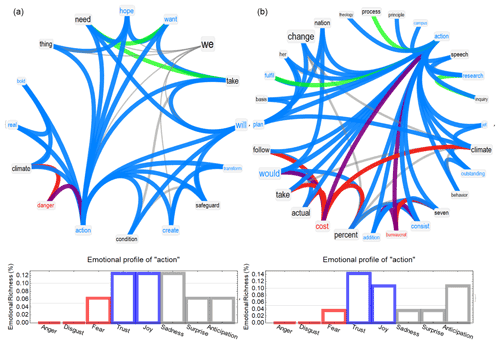
Figure A5Speakers' mindset reconstruction around “action” in the speeches of Greta Thunberg (a) and Christopher Monckton (b). We refer the reader to Fig. 1 for a detailed explanation of the colour code and to Lexicon 1 for an interpretation of the figure.
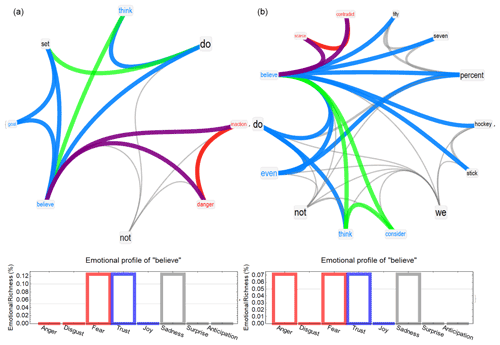
Figure A6Speakers' mindset reconstruction around “believe” in the speeches of Greta Thunberg (a) and Christopher Monckton (b). We refer the reader to Fig. 1 for a detailed explanation of the colour code and to Lexicon 1 for an interpretation of the figure.
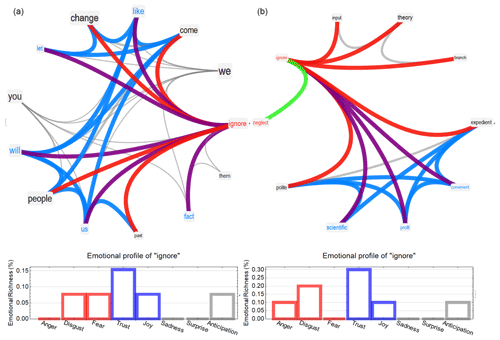
Figure A7Speakers' mindset reconstruction around “ignore” in the speeches of Greta Thunberg (a) and Christopher Monckton (b). We refer the reader to Fig. 1 for a detailed explanation of the colour code and to Lexicon 1 for an interpretation of the figure.
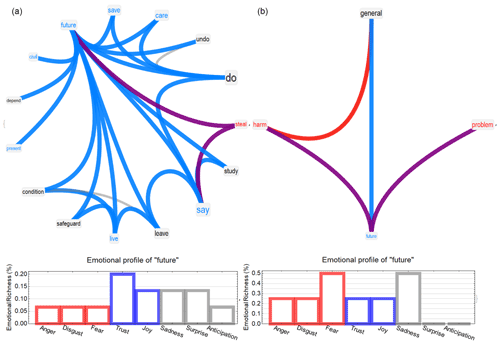
Figure A8Speakers' mindset reconstruction around “future” in the speeches of Greta Thunberg (a) and Christopher Monckton (b). We refer the reader to Fig. 1 for a detailed explanation of the colour code and to Lexicon 1 for an interpretation of the figure.
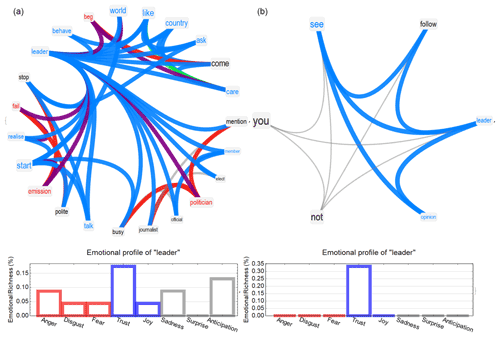
Figure A9Speakers' mindset reconstruction around “leader” in the speeches of Greta Thunberg (a) and Christopher Monckton (b). We refer the reader to Fig. 1 for a detailed explanation of the colour code and to Lexicon 1 for an interpretation of the figure.
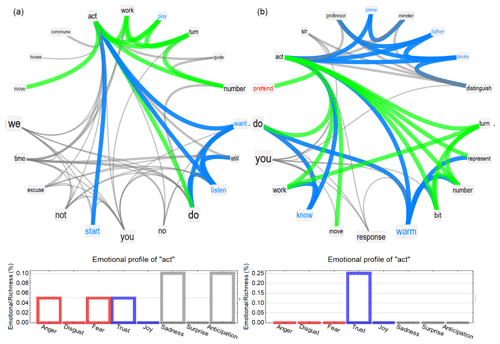
Figure A10Speakers' mindset reconstruction around “act” in the speeches of Greta Thunberg (a) and Christopher Monckton (b). We refer the reader to Fig. 1 for a detailed explanation of the colour code and to Lexicon 1 for an interpretation of the figure.
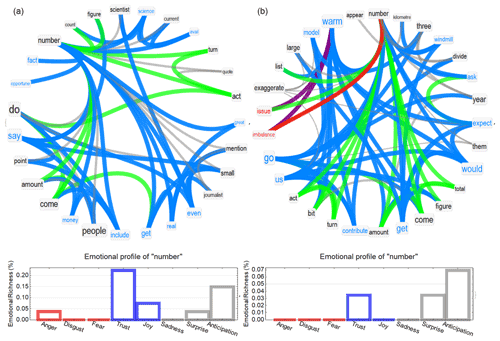
Figure A11Speakers' mindset reconstruction around “number” in the speeches of Greta Thunberg (a) and Christopher Monckton (b). We refer the reader to Fig. 1 for a detailed explanation of the colour code and to Lexicon 1 for an interpretation of the figure.
The textual forma mentis networks investigated in this paper can be built through code available online in Mathematica (https://peerj.com/articles/cs-295/#supp-1; Stella, 2022a).
Our underlying research data are available online, in public domain, in the form of online public speeches and transcripts. For convenience, we have stored them on an Open Science Foundation repository at https://osf.io/ysdxf/ (Stella, 2022b).
RC and MS envisioned the study. MS and RC collected the data and analysed them. RC and MS drafted the manuscript.
The contact author has declared that neither of the authors has any competing interests.
Publisher's note: Copernicus Publications remains neutral with regard to jurisdictional claims in published maps and institutional affiliations.
The authors thank the organisers of the Winter Workshop on Complex Systems, editions 2019 and 2020, and gratefully acknowledge the feedback received at the Conference on Complex Systems 2020. The authors gratefully acknowledge the multiple helpful suggestions given by editor Hermann Held, reviewer Mary Sanford, and an anonymous reviewer.
The article processing charges for this open-access publication were covered by the Helmholtz-Zentrum Hereon.
This paper was edited by Hermann Held and reviewed by Mary Sanford and one anonymous referee.
Barsade, S. G.: The ripple effect: Emotional contagion and its influence on group behavior, Admin. Sci. Quart., 47, 644–675, 2002.
Bloodhart, B., Swim, J. K., and Dicicco, E.: “Be Worried, be VERY Worried”: Preferences for and Impacts of Negative Emotional Climate Change Communication, Front. Commun., 3, 63, https://doi.org/10.3389/fcomm.2018.00063, 2019.
Chen, D. and Manning, C. D.: A fast and accurate dependency parser using neural networks, in: Proceedings of the 2014 conference on empirical methods in natural language processing (EMNLP), October 2014, Doha, Qatar, https://aclanthology.org/D14-1082.pdf (last access: 28 October 202), 740–750, 2014.
Demelle, B.: Before the flood. Top 10 Climate Deniers, https://www.beforetheflood.com/explore/the-deniers/top-10-climate-deniers/ (last access: March 2020), 2016.
Desmog: An extensive database of individual climate deniers involved in the global warming denial industry, https://www.desmogblog.com/global-warming-denier-database (last access: March 2020), 2021.
Figueres, C. and Rivett-Carnac, T.: Tackling two crises at once, New Scientist, 245, 23, https://doi.org/10.1016/S0262-4079(20)30683-7, 2020.
Fillmore, C. J. and Baker, C. F.: Frame semantics for text understanding. in: Vol. 6, Proceedings of WordNet and Other Lexical Resources Workshop, June 2001, Pittsburgh, USA, https://course.ccs.neu.edu/csg224/resources/framenet/framenet.pdf (last access: 28 October 2022), 2001.
Hahnel, U. J., Mumenthaler, C., and Brosch, T.: Emotional foundations of the public climate change divide. Climatic Change, 161, 9–19, 2020.
Healy, N. and Debski, J.: Fossil fuel divestment: Implications for the future of sustainability discourse and action within higher education, Local Environ., 22, 699–724, 2017.
Hills, T. T.: The dark side of information proliferation, Perspect. Psycholog. Sci., 14, 323–330, 2019.
Hoffman, A. J.: The growing climate divide, Nat. Clim. Change, 1, 195–196, 2011.
Holten, D.: Hierarchical edge bundles: Visualization of adjacency relations in hierarchical data, IEEE T. Visualiz. Comput. Graph., 12, 741–748, 2006.
Horton, R.: Offline: Facts are not enough, Lancet, 395, 546, https://doi.org/10.1016/s0140-6736(20)30405-0, 2020.
Jasper, J. M.: Emotions and social movements: Twenty years of theory and research, Annu. Rev. Sociol., 37, 285–303, 2011.
Kramer, A. D., Guillory, J. E., and Hancock, J. T.: Experimental evidence of massive-scale emotional contagion through social networks, P. Natl. Acad. Sci. USA, 111, 8788–8790, 2014.
Lerner, J. S., Li, Y., Valdesolo, P., and Kassam, K. S.: Emotion and decision making, Annu. Rev. Psychol., 66, 799–823, 2015.
Marris, E.: Why young climate activists have captured the world's attention, Nature, 573, 471–473, 2019.
Metcalf, L. and Casey, W. (Eds.): Chapter 5 – Graph theory, in: Cybersecurity and applied mathematics, Syngress, 67–94, ISBN 978-0-12-804452-0, 2016.
Miller, G. A.: WordNet: a lexical database for English, Commun. ACM, 38, 39–41, 1995.
Mohammad, S. M. and Turney, P. D.: Crowdsourcing a word–emotion association lexicon, Comput. Intel., 29, 436–465, 2013.
Mumford, M. D.: Pathways to outstanding leadership: A comparative analysis of charismatic, ideological, and pragmatic leaders, Lawrence Erlbaum Associates Publishers, https://doi.org/10.4324/9780415963633, 2006.
Newman, M. E.: Ego-centered networks and the ripple effect, Social Netw., 25, 83–95, 2003.
Otto, I. M., Donges, J. F., Cremades, R., Bhowmik, A., Hewitt, R. J., Lucht, W., Rockström, J., Allerberger, F., McCaffrey, M., Doe, S. S., and Lenferna, A.: Social tipping dynamics for stabilizing Earth's climate by 2050, P. Natl. Acad. Sci. USA, 117, 2354–2365, 2020.
Schneider, C. R., Zaval, L., and Markowitz, E. M.: Positive emotions and climate change, Curr. Opin. Behav. Sci., 42, 114–120, 2021.
Smith, K. R., Woodward, A., Campbell-Lendrum, D., Chadee, D. D., Honda, Y., Liu, Q., Olwoch, J. M., Revich, B., and Sauerborn, R.: Human health: impacts, adaptation, and co-benefits, in: Climate Change 2014: Impacts, Adaptation, and Vulnerability, Part A: Global and Sectoral Aspects, Contribution of Working Group II to the Fifth Assessment Report of the Intergovernmental Panel on Climate Change, edited by: Field, C. B., Barros, V. R., Dokken, D. J., Mach, K. J., Mastrandrea, M. D., Bilir, T. E., Chatterjee, M., Ebi, K. L., Estrada, Y. O., Genova, R. C., Girma, B., Kissel, E. S., Levy, A. N., MacCracken, S., Mastrandrea, P. R., and White, L. L., Cambridge University Press, Cambridge, UK and New York, NY, USA, 709–754, ISBN 978-1-107-05807-1, 2014.
Stella, M.: Commented Code for building TFMNs in Mathematica 11.3, PeerJ, https://peerj.com/articles/cs-295/23supp-1 (last access: 1 October 2022), 2022a.
Stella, M.: Supplementary Material for: Disentangling the climate divide with emotional patterns: a network-based mindset reconstruction approach, OSF [data set], https://osf.io/ysdxf/ (last access: 1 October 2022), 2022b.
Stella, M.: Text-mining forma mentis networks reconstruct public perception of the STEM gender gap in social media, Peer J., 6, e295, https://doi.org/10.7717/peerj-cs.295, 2020.
Stella, M., Ferrara, E., and De Domenico, M.: Bots increase exposure to negative and inflammatory content in online social systems, P. Natla. Acad. Sci. USA, 115, 12435–12440, 2018.
Teixeira, A. S., Talaga, S., Swanson, T. J., and Stella, M.: Revealing semantic and emotional structure of suicide notes with cognitive network science, Scient. Rep., 11, 1–15, 2021.
Vitevitch, M.: Network Science in Cognitive Psychology, Routledge, ISBN 978-1-138-64017-7, 2019.
Watts, N., Amann, M., Arnell, N., Ayeb-Karlsson, S., Belesova, K., Boykoff, M., Byass, P., Cai, W., Campbell-Lendrum, D., Capstick, S., and Chambers, J.: The 2019 report of The Lancet Countdown on health and climate change: ensuring that the health of a child born today is not defined by a changing climate, Lancet, 394, 1836–1878, 2019.
Youtube: Rebutting the Climate Delusion of the UN in Madrid (sic), https://www.youtube.com/watch?v=a3W8EVEEKQ8 (last access: January 2022), 2019.