the Creative Commons Attribution 4.0 License.
the Creative Commons Attribution 4.0 License.
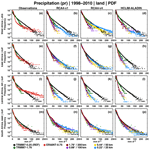
The impact of regional climate model formulation and resolution on simulated precipitation in Africa
Grigory Nikulin
Erik Kjellström
Danijel Belušić
Colin Jones
David Lindstedt
We investigate the impact of model formulation and horizontal resolution on the ability of Regional Climate Models (RCMs) to simulate precipitation in Africa. Two RCMs (SMHI-RCA4 and HCLIM38-ALADIN) are utilized for downscaling the ERA-Interim reanalysis over Africa at four different resolutions: 25, 50, 100, and 200 km. In addition to the two RCMs, two different parameter settings (configurations) of the same RCA4 are used. By contrasting different downscaling experiments, it is found that model formulation has the primary control over many aspects of the precipitation climatology in Africa. Patterns of spatial biases in seasonal mean precipitation are mostly defined by model formulation, while the magnitude of the biases is controlled by resolution. In a similar way, the phase of the diurnal cycle in precipitation is completely controlled by model formulation (convection scheme), while its amplitude is a function of resolution. However, the impact of higher resolution on the time-mean climate is mixed. An improvement in one region/season (e.g. reduction in dry biases) often corresponds to a deterioration in another region/season (e.g. amplification of wet biases). At the same time, higher resolution leads to a more realistic distribution of daily precipitation. Consequently, even if the time-mean climate is not always greatly sensitive to resolution, the realism of the simulated precipitation increases as resolution increases. Our results show that improvements in the ability of RCMs to simulate precipitation in Africa compared to their driving reanalysis in many cases are simply related to model formulation and not necessarily to higher resolution. Such model formulation related improvements are strongly model dependent and can, in general, not be considered as an added value of downscaling.
- Article
(7291 KB) - Full-text XML
- BibTeX
- EndNote
Regional climate modelling is a dynamical downscaling method widely used for downscaling coarse-scale global climate models (GCMs) to provide richer regional spatial information for climate assessments and for impact and adaptation studies (Giorgi and Gao, 2018; Giorgi and Mearns, 1991; Laprise, 2008; Rummukainen, 2010). It is well established that regional climate models (RCMs) are able to provide added value (understood as improved climatology) compared to their driving GCMs. This includes better representation of regional and local weather and climate features as a result of better capturing small-scale processes, including those influenced by topography, coast lines, and mesoscale atmospheric phenomena (Flato et al., 2013; Prein et al., 2016). However, perceived added value from RCMs may have different causes, and it may not always be for the right reason where “right reason” would result from an improved representation of regional processes at smaller scales. Such improvement leads to more accurate simulations on local scales, and can, to some extent, also reduce large-scale GCM biases (Caron et al., 2011; Diaconescu and Laprise, 2013; Sørland et al., 2018). Contrastingly, added value may be attributed to different reasons, not directly related to higher resolution in RCMs but to different model formulation in the RCMs and their driving GCMs. It is possible that the physics of a RCM has been targeted for processes specific to the region it is being run for, giving it a local advantage over GCMs that may have had their physics developed for global application. However, it is questionable if improvements of such “downscaling” via physics can be considered as an added value. In general, RCMs can either reduce or amplify GCM biases, sometimes even changing their signs (Chan et al., 2013).
Issues such as those mentioned above, have raised substantial concerns among regional climate modellers (e.g., Castro, 2005; Xue et al., 2014). It has been pointed out that understanding of the added value remains challenging. It would become even more complicated taking into account the effects of different realizations, such as the size of domain, lateral boundary conditions, geographical location, model resolution, and its internal variability (Di Luca et al., 2015; Hong and Kanamitsu, 2014; Rummukainen, 2016). All the above factors potentially influence RCM simulations, leading to different interpretations of the downscaling effects, therefore the robustness of added value. For example, it was shown that over the Alps, downscaling with multiple RCMs at increasing resolutions in general is able to provide a more realistic precipitation pattern than the forcing GCMs, and it is regarded as added values from RCMs (Giorgi et al., 2016; Torma et al., 2015). Similarly, Lucas-Picher et al. (2017) found added value over the Rocky Mountains, another region with strong topographic influence on hydrological processes. However, the results are not unambiguous, and sometimes limited added value is found when comparing to the forcing data (e.g. Wang and Kotamarthi, 2014) over North America. This implies that the understanding of downscaling effects is context-dependent, and one should carefully interpret GCM and RCM simulations in order to detect robust added value.
Africa is foreseen to be vulnerable to future climate change, which early on inspired efforts to employ RCMs for impact and adaptation studies (e.g. Challinor et al., 2007). Further to previous coordinated downscaling activities over Africa as for example the African Monsoon Multidisciplinary Analyses (AMMA) (Van der Linden and Mitchell, 2009), the Coordinated Regional climate Downscaling Experiment (CORDEX) provides a large ensemble of RCM projections for Africa (Giorgi et al., 2009; Jones et al., 2011). All CORDEX RCMs follow a common experiment protocol including a predefined domain at 50 km resolution and common output variables and format that facilitates assessment of projected climate changes in Africa. Under this framework, RCMs at 50 km horizontal resolution are found to have the capability of providing added value in representing African climatological features compared to their forcing GCMs, which generally have the resolution coarser than 100 km (Dosio et al., 2015; Moufouma-Okia and Jones, 2015; Nikulin et al., 2012).
However, a number of common challenges to accurately simulate precipitation climatology in Africa have also been identified for the RCMs. Individual RCMs may exhibit substantial biases in different aspects of the precipitation climatology such as seasonal mean (Endris et al., 2013; Kalognomou et al., 2013; Kim et al., 2014; Shongwe et al., 2015; Tamoffo et al., 2019), annual cycle (Favre et al., 2016; Kisembe et al., 2019), onset and cessation of the rainy season (Akinsanola and Ogunjobi, 2017; Gbobaniyi et al., 2014), number of wet days, and their intensity (Klutse et al., 2016). At the same time, most of these studies found that such biases often strongly depend on region and season. A RCM with a substantial bias in one region and/or season may accurately simulate precipitation in other regions and seasons. It was also found that the multimodel ensemble usually outperforms individual RCMs, but it is a result of the cancellation of opposite-signed biases in different RCMs.
A number of possible explanations for such RCM precipitation-related biases in Africa were suggested as for example, different convection schemes (see discussion in Kalognomou et al., 2013), land-atmosphere coupling (e.g. Sylla et al., 2013b), and biases in moisture transport (Tamoffo et al., 2019). However, most of the CORDEX-Africa studies are still descriptive and process-based evaluation studies like Tamoffo et al. (2019) are mostly lacking. An additional barrier for more process-based evaluation studies is that the CORDEX requires atmospheric variables at three pressure levels (850, 500, and 200 mbar) to be provided, which seriously limits evaluation of large-scale and regional circulation (e.g. jet streams) and moisture transport in the troposphere.
Another common problem for almost all RCMs in Africa is the phase of the diurnal cycle of precipitation. The majority of RCMs simulate a maximum precipitation intensity around local noon that is too early compared to late afternoon or even late evening maximum evident in observations (Nikulin et al., 2012). This deficiency of the RCMs is related to the convective parameterization used, and some convection schemes, as for example the Kain–Fritsch (KF), may outperform others, producing a more realistic diurnal cycle (Nikulin et al., 2012).
All the above deficiencies in the RCMs show that higher resolution does not necessarily lead to a better performance of the RCMs in terms of precipitation climatology in Africa. It is also not always clear if differences between the CORDEX-Africa RCMs and their driving GCMs are related to higher RCM resolution, RCM internal formulation, or the combination of both. A thorough understanding of such differences and the added value of the CORDEX-Africa RCMs is necessary for robust regional assessments of future climate change and its impacts in Africa.
In this study, we aim to separate the impact of model formulation and resolution on the ability of RCMs to simulate precipitation in Africa. We conduct a series of sensitivity, reanalysis-driven, experiments by applying two different RCMs, one of them in two different configurations, at four horizontal resolutions. Contrasting the different experiments allows us to separate the impact of model formulation and resolution. We present an overview and the first results of the experiments conducted and leave in-depth detailed process studies for different regions to forthcoming papers.
2.1 The regional climate models
2.1.1 RCA4
The Rossby Centre atmospheric regional climate model – RCA (Jones et al., 2004; Kjellström et al., 2005; Räisänen et al., 2004; Rummukainen et al., 2001; Samuelsson et al., 2011) – is based on the numerical weather prediction model HIRLAM (Undén et al., 2002). To improve model transferability, the latest fourth generation of RCA, RCA4, has a number of modifications for specific physical parameterizations. This includes the modification of convective scheme based on the Bechtold–Kain–Fritsch scheme (Bechtold et al., 2001) with a revised calculation of convective available potential energy (CAPE) profile according to Jiao and Jones (2008) and the introduction of turbulent kinetic energy (TKE) scheme (Lenderink and Holtslag, 2004). The RCA4 model has been applied in many regions worldwide, among them Europe (Kjellström et al., 2016, 2018; Kotlarski et al., 2015), the Arctic (Berg et al., 2013; Koenigk et al., 2015; Zhang et al., 2014), Africa (Nikulin et al., 2018; Wu et al., 2016), South America (Collazo et al., 2018; Wu et al., 2017), South East (Tangang et al., 2018) and South Asia (Iqbal et al., 2017; Rana et al., 2020).
RCA4 has three configurations used for CORDEX simulations that are available through the Earth System Grid Federation (ESGF). They are named (so called RCM version) as v1 (Europe, Arctic, Africa, South East Asia, and Central and North America), v2 (South Asia), and v3 (South America) and differ in some domain-specific retuning. In this study we also include a new configuration – v4. The RCA4-v4 is based on RCA4-v1 but with a change in one parameter leading to reduced turbulent mixing in stable situations (especially momentum mixing). Such a change in the parameter was applied to reduce a prominent dry bias found in the RCA4-v1 CORDEX-Africa simulations over Central Africa (Wu et al., 2016; Tamoffo et al., 2019). Using two parameter settings of RCA4 allows us to examine how sensitive our results are to such small tuning of the same RCM.
2.1.2 HCLIM
HARMONIE-Climate (HCLIM) is a regional climate modelling system designed for a range of horizontal resolutions from tens of kilometres to convection permitting scales of 1–3 km (Belušić et al., 2020; Lindstedt et al., 2015). It is based on the ALADIN-HIRLAM numerical weather prediction system (Belušić et al., 2020; Bengtsson et al., 2017; Termonia et al., 2018). The HCLIM system includes three atmospheric physics packages AROME, ALARO, and ALADIN, which are designed for different horizontal resolutions. The ALADIN model configuration used in this study employs the hydrostatic ARPEGE-ALADIN dynamical core (Temperton et al., 2001), a mass-flux scheme based on moisture convergence closure for parameterizing deep convection (Bougeault, 1985), and SURFEX as the surface scheme (Masson et al., 2013). All details about the version of HCLIM used in this study (HCLIM38), and its applications over different regions can be found in (Belušić et al., 2020). We note that HCLIM38-ALADIN used in the study is not the same model as ALADIN-Climate used in CORDEX (Daniel et al., 2019). We refer to HCLIM38-ALADIN as HCLIM-ALADIN hereafter.
2.2 Experimental design
To investigate the response of both RCA4 and HCLIM-ALADIN to horizontal resolution, we conduct a set of sensitivity experiments driven by the ERA-Interim reanalysis (denoted as ERAINT hereafter; Dee et al., 2011) at four different resolutions. These resolutions are 1.76, 0.88, 0.44, and 0.22∘ for RCA4 with the rotated coordinate system and 200, 100, 50, and 25 km for HCLIM-ALADIN with the Lambert Conformal projection. The 0.44∘ or 50 km resolution is recommended by the CORDEX experiment design and used in the CORDEX-Africa ensemble. Hereafter, the resolution in kilometres is used unless otherwise specified.
Table 1The full domain configuration and time step for the RCA4 and HCLIM-ALADIN simulations. The full domain includes the eight grid point relaxation zone.
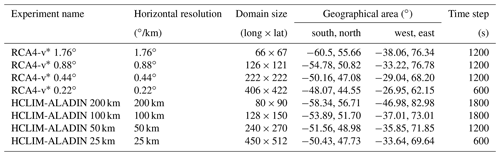
There are two approaches to set up a RCM experiment with simulations at different resolutions. The first approach is to use the same full domain (including the relaxation zone) for all simulations at different resolutions. Size of the full domain is defined by the coarsest resolution in the experiment (200 km in our case). A benefit of such an experiment setup is a consistent lateral boundary forcing for all simulations, given the same full domain. However, an unnecessary large full domain for resolutions finer than 200 km (i.e. 100, 50, and 25 km) leads to larger RCM internal variability (IV) compared to simulations at the same resolutions but with a minimum size full domain. Computational demands at the finer resolutions are also higher in the case of the large full domain. The second approach is to use different (minimum) full domains for different resolutions defined only by size of the active domain (the same for all resolutions) and a necessary relaxation zone (smaller in kilometre for higher resolution). An advantage of this approach is less IV and less computational demand for high-resolution simulations, while a shortcoming is inconsistent lateral boundary forcing (different size of the full domain). We decided to use the second approach with the minimum size of the full domain (less IV and computational demand), although we note that a perfect experiment has to include both approaches, if resources allow. The setup of the simulations at the four resolutions is identical apart from the time step (adjusted to ensure numerical simulation stability) and the size of the full computational domain with the relaxation zone (see Table 1). The relaxation zone has eight grid points in all directions and increases (in kilometres) at coarser resolution, while the interior CORDEX-Africa domain is the same.
As mentioned above, larger size of the computational domain at coarser resolution in our experiment setup may have a potential impact on the simulated climatology, leading to larger IV developed by the RCMs and weaker constraints on the ERAINT forcing. As a simple test for domain-dependent RCM IV we perform an additional experiment with RCA4 at 0.88∘ resolution taking the full computational domain from the 1.76∘ RCA4 simulation. Indeed, for the 1981–2010 climatology, seasonal mean precipitation differences between the two experiments can reach up to 1.25 mm d−1 (up to 25 %) at a few individual grid boxes, often at the edges of the tropical rain belt, although in general they stay below 0.5 mm d−1 (not shown). Seasonal mean temperature also differs with up to 1.25 ∘C regionally (not shown). We do not focus on this single additional sensitivity experiment in the study. A full set of simulations with the same full domain for all RCMs and resolutions is necessary for robust conclusions.
Another source of IV in RCMs is related to different initialization or starting time (e.g. Lucas-Picher et al., 2008; Sanchez-Gomez and Somot, 2018). We perform two additional experiments in order to see how different initialization time impacts the IV in the RCMs. Both RCA4-v1 and ALADIN at 50 km were initialized on 1 January 1980 instead of 1 January 1979 as for all other simulations in the study. It was found that the impact of the different starting time is much smaller than the impact of the larger domain. For both seasonal mean precipitation and temperature, differences between the experiments are small over the African continent, in general, less than 0.5 mm d−1 for precipitation and 0.25 ∘C for temperature (not shown). Similar to the domain-dependent sensitivity experiment above, we do not focus on these two additional initialization sensitivity experiments in the study. A full investigation of the initialization-related RCM IV needs generation of a larger (up to 10 members) ensemble for all RCMs and resolutions.
We note that in general, both regional models – RCA and HCLIM-ALADIN – were developed to operate at a range of tens of kilometres resolution, and their performance at 100 and especially at 200 km may not be optimal. A potential caveat here is that very few RCM physical parameterizations are automatically scaled to run at very coarse resolution. Thus, RCM deficiencies at the coarser resolutions may be partly related to the lack of model retuning. We think that such coarse-resolution simulations are a useful supplement to simulations at a RCM comfortable resolution zone and help us to understand RCM behaviour without additional resolution-dependent tuning. All simulations are conducted without spectral nudging similar to the CORDEX-Africa RCMs (Nikulin et al., 2012) allowing each RCM to develop its own climatology as much as possible. Analysis is done for the CORDEX-Africa domain shown in Fig. 1.
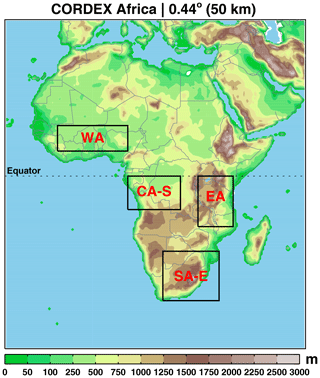
Figure 1Topography (m) for the CORDEX-Africa domain in RCA4 at 50 km resolution. Boxes indicate the four subregions used for spatially averaged analysis: West Africa (WA), East Africa (EA), the southern Central Africa (CA-S), and eastern South Africa (SA-E).
The difference between a RCM and its driving GCM can, in general, be attributed to three sources, namely (i) different resolution, (ii) different physical formulation, and (iii) artefacts of the one-way nesting approach including size of the RCM domain and application of spectral nudging (e.g. Scinocca et al., 2016). The RCA4 0.88∘ simulations and the HCLIM-ALADIN 100 km one represent a slight upscaling of ERAINT (about 0.7∘ or about 77 km at the Equator), and we refer to them as “no added value experiment” (NAVE). No resolution-dependent added value of the RCMs is expected for these NAVE simulations, and all differences between the RCMs and their driving ERAINT are attributed to different physical formulations and to the artefacts of the one-way nesting. Spectral nudging is not used in our experiment, and the one-way nesting term is basically reduced to domain configuration. In contrast, if spectral nudging is used, technical aspects of the nudging (e.g. which wavelengths should be nudged and at what altitudes) also contribute to the one-way nesting term. In practice, it is not straightforward (if possible at all) to separate the impact of different physical formulation and artefacts of the one-way nesting approach. Hereafter, we use “RCM formulation” as a term that includes both RCM physical formulation and domain-dependent RCM configuration (e.g. size of the full domain).
2.3 Observations and reanalysis
Observational datasets in Africa, in general, agree well for large-scale climate features but can deviate substantially at regional and local scales (Fekete et al., 2004; Gruber et al., 2000; Nikulin et al., 2012). To take into account the observational uncertainties, we utilize a number of gridded precipitation datasets. They include three gauged-based datasets: the Global Precipitation Climatology Centre, GPCC, version 7 (Schneider et al., 2014); the Climate Research Unit Time Series, CRU TS, version 3.23 (Harris et al., 2014); and University of Delaware, UDEL, version 4.01 (Legates and Willmott, 1990). All these three datasets are at 0.5∘ horizontal resolution. For the evaluation of precipitation extremes and diurnal cycle simulated by RCMs, we utilize a satellite-based precipitation dataset from the Tropical Rainfall Measuring Mission, TRMM 3B42 version 7 (Huffman et al., 2007), which is at 0.25∘ horizontal resolution and 3-hourly temporal resolution. The TRMM product starts in 1998, and for evaluation of precipitation extremes and diurnal cycle we use a shorter period (1998–2010) in contrast to 1981–2010 used for evaluation of seasonal means and annual cycle. We also note that the TRMM 3B42-v7 precipitation product provides satellite-based precipitation estimates adjusted by the GPCC gauge-based precipitation. This means that monthly mean TRMM 3B42 and GPCC precipitation are almost the same if remapped to the same resolution or averaged over a region.
ERAINT as the driving reanalysis is also used for analysis. In contrast to climate models, ERAINT precipitation is a short term forecast product, and there are several ways to derive ERAINT precipitation (e.g. different spin-up, base time, and forecast steps), which can lead to different precipitation estimates (Dee et al., 2011). ERAINT precipitation for this study is derived by the simplest method, without spin-up as in some of the previous studies (Dosio et al., 2015; Moufouma-Okia and Jones, 2015; Nikulin et al., 2012): 3-hourly precipitation uses the base times of 00:00/12:00 and forecast steps 3/6/9/12 h, while daily precipitation uses base times of 00:00/12:00 and forecast steps of 12 h. The RCMs and ERAINT represent 3-hourly mean precipitation for the 00:00–03:00, 03:00–06:00, ..., 21:00–00:00 intervals, while TRMM precipitation averages represent approximately the 22:30–01:30, 01:30–04:30, ..., 19:30–22:30 UTC intervals.
2.4 Methods
The coarsest resolution of 200 km is used as a reference resolution for spatial maps. The higher-resolution simulations are aggregated to the 200 km grid by the first-order conservative remapping method (Jones, 1999). In this way we expect that the difference among the aggregated simulations at common resolution should mainly be caused by the different treatment for fine-scale processes (Di Luca et al., 2012). For the regional analyses, such as the analysis of annual cycle, diurnal cycle, and daily precipitation intensity, we focus on four subregions, presenting different climate zones in Africa: West Africa (10∘ W–10∘ E, 7.5–15∘ N), East Africa (30–40∘ E, 15–0∘ S), southern Central Africa (10–25∘ E, 10–0∘ S), and eastern South Africa (20–36∘ E, 35–22∘ S) as defined in Fig. 1. The period 1981–2010 is used for the analysis in this study, unless otherwise specified.
3.1 Seasonal mean
In the boreal summer defined here as July–September (JAS), the tropical rain belt (TRB) associated with the intertropical convergence zone (ITCZ) is positioned to its northernmost location with the maximum precipitation north of the Equator (Fig. 2a). CRU, UDEL, and GPCC aggregated to the 200 km resolution, generally agree well with each other, with only slight local differences (Fig. 2a–c). ERAINT overestimates precipitation over Central Africa and along the Guinea coast, while underestimating it over West Africa, north of the Guinea coast (Fig. 2d). All RCA4-v1 simulations have a pronounced dry bias (Fig. 2e–h) that spatially almost coincides with the wet bias in ERAINT and increases at coarser resolution (Fig. 2e–f). RCA4-v4 shows a similar pattern compared to RCA4-v1 but substantially reduces the dry bias over Central Africa at all four resolutions (Fig. 2i–l). For both configurations of RCA4, the smallest dry bias is found at the highest 25 km resolution. At the same time, an overestimation of precipitation north of the central dry-bias region becomes more pronounced, especially for RCA4-v4. HCLIM-ALADIN, in general, shows some similarities to RCA4 with a pronounced dry bias in West and Central Africa at 200 km that is strongly reduced with increasing resolution. However, a wet bias emerges on the northern flank of the rain belt at 50 and 25 km. For JAS there is a common tendency for both RCMs to generate more precipitation at higher resolution, leading to a reduction in the dry biases over Central Africa. Such a bias reduction may be considered as a resolution-related improvement. However, the RCM simulations clearly show that the added value of higher resolution can be region-dependent. An improvement in the simulated precipitation climatology over one region corresponds to deterioration of the climatology over another region. Moufouma-Okia and Jones (2015) found a mixed response to resolution in simulated seasonal mean precipitation over West Africa. Their RCM simulations at 50 and 12 km bear a great deal of similarity with each other, while a simulation at 25 km shows wetter conditions in the Sahel and drier ones near the coastal area in the south (see their Fig. 8). In contrast, Panitz et al. (2014) found almost no difference in seasonal rainfall over West Africa between two RCM simulations at 50 and 25 km. We conclude that for both RCA4 and HCLIM-ALADIN, spatial bias patterns are similar and more related to model formulation, while magnitude of biases are more sensitive to resolution. For example, the sign of the bias pattern in our no added value RCM simulations at 100 km in JAS (Fig. 2f, j, n) is almost opposite to the sign of the bias pattern in the driving ERAINT (Fig. 2d).
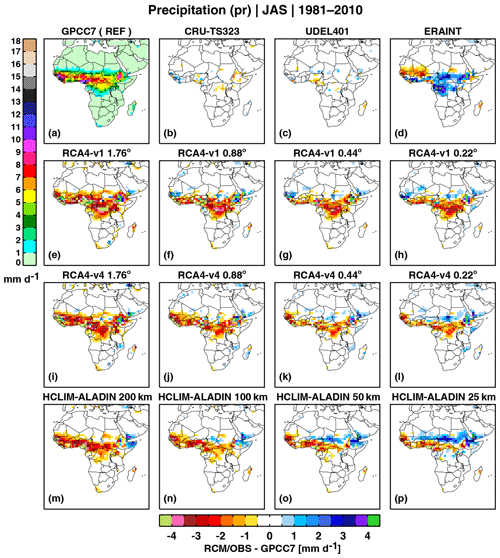
Figure 2GPCC7 mean JAS precipitation for 1981–2010 and differences compared to GPCC7 in (b–d) the other gridded observations, (e–h) the RCA4-v1, (i–l) RCA4-v4, and (m–p) HCLIM-ALADIN simulations. All datasets are aggregated to the coarsest 200 km grid.
In boreal winter (December–February, DJF), the TRB migrates to its most southerly position covering the latitudes from southern to Central Africa, with the maximum over southern tropical Africa and Madagascar (Fig. 3a). Similar to JAS, observational uncertainties are generally small in DJF, and there is a pronounced wet bias in ERAINT over Central Africa (Fig. 3d). At 25 and 50 km RCA4-v1 has a dipole bias pattern with an underestimation of rainfall over Central Africa and an overestimation over South Africa. At 200 km there is a pronounced deterioration in the simulated rainfall: a strong dry bias appears along the eastern coast and Madagascar, while the wet bias is amplified over large parts of southwestern Africa. At 25 and 50 km RCA4-v4 shows a large-scale dipole bias pattern similar in some degree to RCA4-v1. The RCA4-v4 biases are smaller than the RCA4-v1 ones showing an impact of the retuning (reducing mixing in the boundary layer). The behaviour of RCA4-v4 at coarser resolution is also similar to RCA4-v1. A similar strong dry bias is emerging along the eastern coast at 200 km. However, in contrast to RCA4-v1, the dry bias over the Democratic Republic of the Congo almost completely disappears at both 100 and 200 km. HCLIM-ALADIN simulates almost the same bias pattern at all resolutions, strongly underestimating rainfall over southeastern Africa and overestimating it over the Guinea coast, parts of Central Africa, and southern Africa. There is a tendency to an increase in precipitation with higher resolution in HCLIM-ALADIN: the wet biases are amplified, and the dry biases are reduced. Both RCA4 and HCLIM-ALADIN show a common feature – intensification of the dry bias along the eastern coast of Africa at 200 km. Even if both RCMs have this dry bias in common, there are also differences showing the importance of model formulation. HCLIM-ALADIN has about the same bias pattern at all four resolutions, while the RCA4 bias pattern substantially changes across the resolutions. Such resolution dependency in RCA4 may be related to the fact that RCA4 is based on a limited area model and not developed to operate at 100–200 km resolution. Contrastingly, HCLIM-ALADIN, which is based on a global model, shows more consistent results even at 100–200 km resolution. This indicates that HCLIM-ALADIN parameterizations may be better suited to work also at coarser resolution. Although, we also note that the resolution dependency of the RCA4 bias pattern over southern Africa is similar to that found for the CMIP5 GCMs (Munday and Washington, 2018). They show that the GCMs with the coarsest resolution and respectively the lowest topography have the wettest bias over the Kalahari basin and the driest bias over the southeast African coast, the Mozambique Channel, and Madagascar. Such a bias pattern is related to a smoother barrier to northeasterly moisture transport from the Indian Ocean that penetrates across the high topography of Tanzania and Malawi into subtropical southern Africa. However, in our analysis, HCLIM-ALADIN does not show such resolution-related dependency. In general, similar to JAS, the added value of higher resolution in DJF is region dependent, with higher-resolution biases reduced over one region but amplified over another.
3.2 Annual cycle
The annual cycle of precipitation over the four subregions is shown in Fig. 4. The observed annual cycle of precipitation over West Africa depicts the West African monsoon (WAM) rainfall, with maximum precipitation in August (Fig. 4a). All observational datasets (CRU and UDEL are not shown) and ERAINT agree well with each other, with only a small underestimation of rainfall by ERAINT in June–August. In contrast to the observations, RCA4-v1 has a bimodal annual cycle with a too early onset of the rainy season (Fig. 4b). The simulated rainfall is overestimated in March–May, underestimated in July–August during the active WAM period and is well in line with the observations during the cessation of the WAM rainfall in September–November. RCA4-v4 shows a similar behaviour, but the first rainfall peak in May is reduced, and the annual cycle has a more unimodal shape (Fig. 4c). HCLIM-ALADIN, in general, shows similar features as both configurations of RCA4, although it has more similarities with RCA4-v4 (Fig. 4d). The too early onset of the rainy season is a common problem for many RCMs reported by Nikulin et al. (2012). Our results show that this is not dependent on resolution but instead related to model formulation. Higher resolution reduces the wet bias during the onset of the rainy season for RCA-v1, has no impact for RCA-v4, and amplifies the wet bias in HCLIM-ALADIN. Nevertheless, the impact of higher resolution is more consistent during the rainy season. Increasing resolution tends to increase monsoon rainfall for both RCMs, resulting in smaller dry biases and a pattern closer to the unimodal one in the observations. East and Central Africa have a bimodal annual cycle of rainfall with two peaks around November and May (Fig. 4e, i). GPCC, CRU, and UDEL (both not shown) agree well on the phase and magnitude of the annual cycle for both subregions. ERAINT has a weaker bimodality overestimating precipitation in December–February over East Africa and all year round over Central Africa with the largest wet bias during October–April. Both configurations of RCA4 fail to reproduce the bimodal annual cycle in East Africa at 200 km, underestimating precipitation all year round and showing a single rainfall peak in December (Fig. 4j, k). Increasing resolution reduces the dry bias and leads to an improvement in the shape of the annual cycle. The bimodal shape begins to appear at 100 km and becomes much closer to the observation at 50 and 25 km. Despite some mixed dry and wet biases in different seasons, the 25 and 50 km RCA4 simulations show the best agreement with the observations. In contrast to RCA4, HCLIM-ALADIN simulates the unimodal annual cycle at all four resolutions, and some signs of bimodality only appear at 25 km (Fig. 4h). Similar to RCA4, increasing resolution leads to an increase in precipitation in HCLIM-ALADIN, although a dry bias is a prominent feature from November to May in all HCLIM-ALADIN simulations. For Central Africa, the bimodality of the annual cycle is reproduced well by both RCMs at all resolutions (Fig. 4j–l). HCLIM-ALADIN maintains similar behaviour to that in East Africa, although the difference in precipitation across the resolutions is small (Fig. 4l). On the other hand, for both configurations of RCA4 in Central Africa, increasing resolution leads to decreasing precipitation during the rainy seasons, especially in January. Both RCMs strongly reduce the ERAINT wet bias even in the NAVE at 100 km. Such improvement indicates that model formulation plays a more important role than resolution over Central Africa. For eastern South Africa, the annual cycle of precipitation is unimodal with its maximum during austral summer (Fig. 4m). Similar to West Africa, uncertainties between observational datasets and reanalysis are small. RCA4 in general overestimates rainfall during the rainy season with the largest wet bias at 200 km. Surprisingly, the simulated rainfall is almost the same at 25 and 100 km, while the smallest bias is found at 50 km for both RCA4 configurations. HCLIM-ALADIN also overestimates precipitation during the rainy season at all four resolutions (Fig. 4p). However, the smallest wet bias in the HCLIM-ALADIN simulations is found at 50 and 100 km.
3.3 Diurnal cycle
The diurnal cycle is a prominent feature of forced atmospheric variability with a strong impact on regional- and local-scale thermal and hydrological regimes. The diurnal cycle of precipitation in the tropics is well documented and includes a late afternoon/evening maximum over land (Dai et al., 2007). However, it is still a common challenge for GCMs (Dai, 2006; e.g. Dai and Trenberth, 2004; Dirmeyer et al., 2012), RCMs (e.g. Da Rocha et al., 2009; Jeong et al., 2011; Nikulin et al., 2012), and reanalyses (Nikulin et al., 2012) to accurately represent the diurnal cycle of precipitation.
The TRMM diurnal cycle of precipitation generally shows an increase in rainfall starting around noon with maximum reached at around 18:00 local solar time (LST) (Fig. 5). The ERAINT diurnal cycle is completely out of phase over all subregions with the occurrence of maximum precipitation intensity around local noon. A common feature of ERAINT is an overestimation of precipitation around local noon and an underestimation during the rest of the day. HCLIM-ALADIN shows exactly the same behaviour as ERAINT. Both configurations of RCA4 simulate the diurnal cycle of precipitation more accurately compared to ERAINT and HCLIM-ALADIN. The phase of the diurnal cycle, in general, is pretty well captured over all four subregions. In terms of precipitation intensity RCA4 underestimates rainfall from afternoon to morning over West (Fig. 5b, c) and Central Africa (Fig. 5j, k). Reducing mixing in the boundary layer results in flattening of the diurnal cycle over West Africa (Fig. 5b, c), while there are almost no changes over Central Africa (Fig. 5j, k). RCA4-v1 simulates very well the diurnal cycle over East Africa with only some underestimation in early morning and afternoon (Fig. 5f). RCA4-v4 improves rainfall intensity in early morning but at the same time shows a slightly larger underestimation in afternoon than RCA4-v1 (Fig. 5g). Over southern Africa the RCA4 simulations at 200 km are the closest to the observation (Fig. 5n, o), while the simulations at higher resolutions underestimate the amplitude of the diurnal cycle in the afternoon.
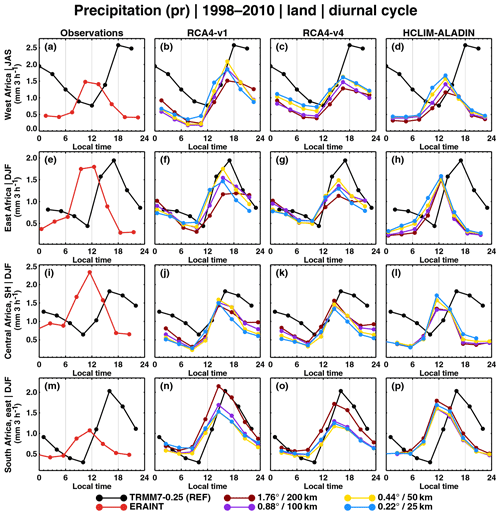
Figure 5Diurnal cycle of 3-hourly mean precipitation over the four subregions for 1998–2010 in observations/ERAINT and as simulated by RCA4 and HCLIM-ALADIN at the four different resolutions. Only land grid boxes are used for averaging over the subregions and only wet days with more than 1 mm d−1 are taken for estimations of the diurnal cycle.
Figure 5 clearly shows that the phase of the diurnal cycle of precipitation in Africa does not depend on resolution but instead depends on model formulation. Both ERAINT, with the Tiedtke convection scheme (Tiedtke, 1989), and HCLIM-ALADIN, with the Bougeault scheme (Bougeault, 1985), trigger precipitation too early during the diurnal cycle, while both configurations of RCA4 with the same Kain–Fritsch (KF) scheme (Bechtold et al., 2001) simulate a much more realistic diurnal cycle. It has previously been shown that the KF scheme is able to reproduce late afternoon rainfall peaks for the regions where moist convection is governed by the local forcing, for example in the southeast US (Liang, 2004) and in tropical South America and Africa (e.g. Bechtold et al., 2004; Da Rocha et al., 2009). Nikulin et al. (2012) also found that a subset of RCMs that employ the KF scheme show an improved representation of the phase of the diurnal cycle in Africa. Our results indicate that the impact of resolution is only seen in the amplitude of the diurnal cycle. However, such impact is not homogeneous across the subregions and the RCMs. For HCLIM-ALADIN, increasing resolution leads to increasing rainfall intensity in all regions but eastern South Africa. RCA4 shows a similar behaviour over West Africa, while there is a mixed response over East and Central Africa. These findings are in line with previous studies investigating resolution effects for GCMs (Covey et al., 2016; Dirmeyer et al., 2012) and for RCMs (Walther et al., 2013). In coarser-scale models (e.g >10 km), increasing resolution only leads to changes in the magnitude but not in the phase of the diurnal cycle of precipitation over land.
Nevertheless, studies conducting sensitivity experiments using resolutions finer than 10 km do find improvements in the representation of the phase (Dirmeyer et al., 2012; Sato et al., 2009; Walther et al., 2013).
3.4 Frequency and intensity of daily precipitation
Figure 6 shows the empirical probability density function (PDF) of daily precipitation intensities over the four subregions. The TRMM7-0.25 dataset, aggregated to the common 1.76∘ resolution (TRMM7-1.76), as expected has a shorter right tail with no precipitation intensities larger than 100 mm d−1 and higher frequency for lower intensities less than 25 mm d−1 (Fig. 6a, e, i, m). The two TRMM7 PDFs provide reference bounds for datasets with resolution between 0.25 and 1.76∘. However, uncertainties in gridded daily precipitation products in Africa are large (Sylla et al., 2013a), and we take the TRMM bounds as an observational approximation focusing more on differences in the simulated PDFs across the four resolutions. Over West, East, and Central Africa ERAINT overestimates the frequency of low (<10 mm d−1) and extremely high (>150 mm d−1) intensities, while it underestimates the frequency of precipitation intensities in between (Fig. 6a, e, i), especially over West Africa (Fig. 6a). In South Africa (Fig. 6m) ERAINT represents the frequency of daily mean precipitation more accurately compared to the other three regions but shows almost no events with more than 150 mm d−1 in contrast to the observations. Both RCMs, in general, have the same tendency to generate more higher-intensity precipitation events with increasing resolution over all four subregions. In West Africa RCA4-v1 strongly underestimates the frequency of intensities with more than 20 mm d−1 at 200, 100, and 50 km (Fig. 6b). A substantial improvement appears only at 25 km where the right tail of the PDF extends up to 250 mm d−1, although the frequency of precipitation events from about 50 to 150 mm d−1 is still underestimated.
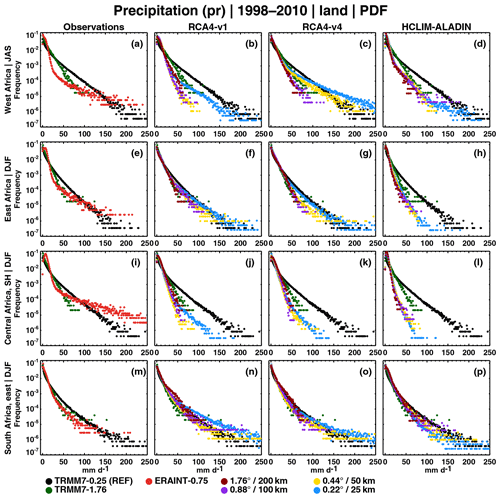
Figure 6Probability distribution function of daily precipitation intensities pooled over the four subregions for 1998–2010 in observations/ERAINT and as simulated by RCA4 and HCLIM-ALADIN at the four different resolutions. TRMM7-1.76 represents TRMM7-0.25 aggregated from its native 0.25∘ resolution to 1.76∘. A base-10 log scale is used for the frequency axis, and the first bin (0–1 mm d−1) is divided by 10. Only land grid boxes are used for pooling over the subregions, and the season is different for the different regions.
The RCA4-v4 configuration markedly reduces the RCA4-v1 biases and shows more realistic PDFs at all four resolutions (Fig. 6c). The RCA4-v4 50 km simulation generates precipitation events up to 250 mm d−1, strongly contrasting the RCA4-v1 simulation at the same resolution (no events for more than 100 mm d−1). However, RCA4-v4 overestimates frequencies of high intensities at 25 km. Such sharp difference between two configurations of RCA4 at the same resolution shows that model formulation also plays an important role for accurately reproducing daily precipitation. Over West Africa all HCLIM-ALADIN simulations overestimate the frequency of low precipitation intensities (less than 10 mm d−1) and underestimate the frequency of intensities in the range of 10–150 mm d−1 (Fig. 6d). Similar to RCA4, higher resolution leads to more high-intensity precipitation events in the HCLIM-ALADIN simulations.
However, RCA4 and HCLIM-ALADIN behave in a different way with increasing resolution. Both RCMs change the PDFs by adding more higher-intensity precipitation events, extending the righthand tail towards higher intensities. In addition, RCA4 also increases the frequency of medium- and high-intensity events, especially going from 50 to 25 km. In East Africa both RCA4 configurations reproduce the observed PDFs almost perfectly (Fig. 6f, g). All four resolutions are located within the TRMM-1.76 and TRMM-0.25 boundaries, and the coarsest and finest resolutions coincide with the respective TRMM PDFs. Contrastingly, HCLIM-ALADIN strongly underestimates the frequency of precipitation events with more than 20 mm d−1 (Fig. 6h) over East Africa, and even the highest 25 km resolution is located below the coarse TRMM-1.76 dataset. In Central Africa both RCMs overestimate the occurrence of intensities less than 20 mm d−1 (Fig. 6j, k, l), especially HCLIM-ALADIN (Fig. 6l), and strongly underestimate the frequency of higher-intensity events. The PDFs at all four resolutions for both RCMs are located below the coarsest TRMM-1.76 PDF. We note that observational uncertainties in precipitation are very large over Central Africa, and we should be careful in the interpretation of Fig. 6j–l. Seasonal mean precipitation, for example, can differ by more than 50 % across different observational datasets (Washington et al., 2013). Additionally, the TRMM dataset is scaled by the gauge-based GPCC precipitation product, while almost no long-term gauges are available in the region (Nikulin et al., 2012). In eastern South Africa RCA4 and HCLIM-ALADIN simulate the precipitation PDFs quite accurately (Fig. 6n–p). An interesting detail is that the 50 km HCLIM-ALADIN simulation shows higher frequency for intensities in the range of 50 to about 200 mm d−1 than the 25 km simulation.
In general, we see the improvement of simulated daily rainfall intensities with increasing resolution across the African continent. There are many studies showing a similar resolution-dependent improvement over both complex terrains and flat regions (e.g. Chan et al., 2013; Huang et al., 2016; Lindstedt et al., 2015; Olsson et al., 2015; Prein et al., 2016; Torma et al., 2015; Walther et al., 2013). Our results are in agreement with the above studies and confirm increasing fidelity of simulated daily rainfall intensities with increasing resolution.
In this study we have investigated the impact of model formulation and spatial resolution on simulated precipitation in Africa. A series of sensitivity, ERA-Interim reanalysis-driven experiments, were conducted by applying two different RCMs (RCA4 and HCLIM-ALADIN) at four resolutions (about 25, 50, 100, and 200 km). The 100 km experiment, at a resolution a bit coarser than the driving ERA-Interim reanalysis, by default does not provide any resolution-dependent added value, while such added value is expected for the 50 and 25 km experiments. The 200 km experiment is an about 3 times upscaling of ERAINT to a resolution of many CMIP5 GCMs and should only be considered as a supplementary experiment since RCMs do not aim to operate at such a coarse resolution. In addition to the two different RCMs, the standard CORDEX configuration of RCA4 is supplemented by another configuration with reduced mixing in the boundary layer. Such a configuration was developed to deal with a strong dry bias of RCA4 in Central Africa. Contrasting the two different RCMs and the two different configurations of the same RCM at the four different resolutions allows us to separate the impact of model formulation and resolution on simulated rainfall in Africa.
Even if the results often depend on region and season and a clear separation of the impact of model formulation and resolution is not always straightforward, we found that model formulation has the primary control over many aspects of the precipitation climatology in Africa. The 100 km NAVE shows that patterns of spatial biases in seasonal mean precipitation are mostly defined by model formulation. These patterns are very different between the driving ERAINT and RCMs, sometimes even with opposite signs, exemplified by the two configurations of RCA4 in JAS (Fig. 1e–l). Resolution in general controls the magnitude of biases, and for both RCA4 and HCLIM-ALADIN higher resolution usually leads to an increase in precipitation amount while preserving large-scale bias patterns. A side effect of such an increase in precipitation amount is that an improvement in one region (e.g. reduction in dry biases) often corresponds to a deterioration in another region (amplification of wet biases) as for HCLIM-ALADIN in JAS (Fig. 1m–p). Nevertheless, on average the smallest biases in seasonal means are found for the simulations at 50 and 25 km resolution.
The impact of model formulation and resolution on the annual cycle of precipitation is mixed and strongly depends on region and season. For example, in both West and Central Africa the shape of the annual cycle for the 100 km NAVE is different from ERAINT. However, the impact of model formulation is opposite between these two regions. In West Africa both RCMs deteriorate the ERAINT annual cycle by simulating a too early onset of the rainy season. In contrast, over Central Africa, both models improve the ERAINT annual cycle by reducing a strong wet bias and changing the unimodal annual cycle to a bimodal one similar to the observations. The impact of resolution can also be different. In West and East Africa, higher resolution (50 and 25 km) leads to an improvement in the annual cycle (more realistic shape and smaller biases). In contrast, over Central Africa, the 25 km RCA4 simulations show the largest biases, while the HCLIM-ALADIN simulations at all four resolutions are almost similar. In general, it is difficult to draw a conclusion about a common impact of model formulation and resolution on the annual cycle.
The phase of the diurnal cycle in Africa is completely controlled by model formulation (convection scheme), while its amplitude is a function of resolution. Both ERAINT and HCLIM-ALADIN show a too early precipitation maximum around noon, while RCA4 simulates a much more realistic diurnal cycle with an evening maximum. Higher resolution does not change the phase of the diurnal cycle but rather its amplitude, although the impact of resolution on the amplitude is mixed across the four subregions and time of the day.
A pronounced and well-known impact of higher resolution on daily precipitation intensities is a more realistic distribution of daily precipitation. Our results also show that higher resolution, in general, improves the distribution of daily precipitation. This includes reduced overestimation of the number of days with low precipitation intensities and reduced underestimation of the number of days with high intensities. The latter results in extending the righthand tail of the distribution towards higher intensities similar to observations. This also means that at higher resolutions the time-mean climate (e.g. seasonal mean and annual cycle) is made up of more realistic underpinning daily precipitation than at lower resolutions. It is also worth emphasizing that if low resolution models are not able to simulate high-rainfall days, then it will be difficult for them to say anything robust about projected climate changes in high-rainfall events. However, regionally, model formulation can also play an important role in the distribution of daily precipitation. For example, in West Africa the 50 km RCA4-v4 configuration with reduced mixing in the boundary layer shows a remarkable improvement in the shape of the PDF (Fig. 6c) compared to the standard RCA4-v1 configuration at the same resolution (Fig 6b). Moreover, the RCA4-v4 configuration at 50 km shows almost the same PDF as RCA4-v1 at 25 km. Such contrast indicates that for daily precipitation intensities model formulation can have the same impact as doubled resolution.
Improvements in simulated precipitation in high-resolution RCMs relative to coarse-scale GCMs are often attributed as being a resolution-dependent added value of downscaling. Our results show that for Africa improvements are not only related to higher resolution but also to different model formulation between the RCMs and their driving reanalysis. A common framework for quantifying added value of downscaling is to evaluate some aspects of the climate in high-resolution RCM simulations and in their coarse-resolution driving reanalysis or GCMs over a historical period (Di Luca et al., 2015; e.g. Hong and Kanamitsu, 2014; Rummukainen, 2016). If the RCM simulations show smaller biases compared to reference observations than the driving GCMs, one can conclude that RCMs provide an added value and vice versa. However, such a framework does not separate the impact of different model formulation between RCMs and their driving GCMs and higher resolution in the RCM simulations. Our results indicate that improvements in RCM simulations may simply be related to different model formulation and not necessarily to higher resolution. In general, model formulation related improvements cannot be considered as an added value of downscaling as such improvements are strongly model dependent and cannot be generalized. However, such formulation-related and region-specific improvements from RCMs could in principle be also used in GCMs.
Within the commonly used RCM evaluation framework, e.g. the CORDEX evaluation experiment, it is not straightforward, if possible at all, to isolate the impact of model formulation and resolution in RCM simulations. We show that running RCMs at about the same resolution as a driving reanalysis (e.g. ERAINT at about 80 km or ERA5 at about 30 km) helps to separate the impacts of model formulation and higher resolution in dynamical downscaling. We propose that such a simple additional experiment can be an integral part of the RCM evaluation framework in order to elucidate the added value of downscaling. In our study, as the first step, we focus only on precipitation that has large relevance for climate change impact studies. As the next step, we foresee similar studies looking also at other variables and especially at processes and drivers relevant for regional climate.
Moreover, the same NAVE framework can be used for quantifying the added value in RCM-based future climate projections. For this, one needs to downscale GCMs at their native resolution in addition to the standard CORDEX resolutions (25 or 50 km). The RCM projections at the native GCM resolution serve as the NAVE in the climate change context. A potential caveat, already mentioned in our study, is that RCMs are generally developed and tuned to operate at resolution of tens of kilometres. “Downscaling” a GCM at its native resolution, for example 150 or 200 km, may lead to artefacts related to a lack of RCM retuning for coarser resolution. Nerveless, more and more GCMs, for example in CMIP6, have resolution finer than 100 km that allows application of the NAVE.
The analysis is done in MATLAB, and IDL and codes can be provided by request as they are but without support on implementing them in another computing environment.
The ERA-Interim reanalysis is available at https://apps.ecmwf.int/datasets/ (ECMWF, 2019); the GPCC dataset is available at https://www.dwd.de/EN/ourservices/gpcc/gpcc.html (GPCC, 2019); the CRU dataset is available at https://catalogue.ceda.ac.uk/uuid/5dca9487dc614711a3a933e44a933ad3 (UEA, 2019); the UDEL dataset is available at http://climate.geog.udel.edu/~climate/html_pages/download.html (University of Delaware, 2019); the TRMM dataset is available at https://pmm.nasa.gov/data-access/downloads/trmm (GSFC, 2019). The RCA4 and HCLIM-ALADIN data can be provided by request.
MW performed RCA4 simulations and all the analysis and wrote the initial draft. GN developed the experiment design and provided guidance for the analysis. EK and GN revised the initial draft. CJ is responsible for setting up the new RCA4 configuration (v4). DB and DL are responsible for performing the HCLIM-ALADIN simulations over Africa. All the authors contributed with discussions and revisions.
The authors declare that they have no conflict of interest.
We thank one anonymous reviewer and John Scinocca for their very helpful comments. The authors also thank the European Centre for Medium-Range Weather Forecasts (ECMWF), the Global Precipitation Climatology Centre (GPCC), the British Atmospheric Data Centre (BADC), the University of East Anglia (UEA), the University of Delaware, and the Goddard Space Flight Center (GSFC) for providing data. All simulations were conducted on the supercomputer in the National Supercomputer Centre, Linköping, Sweden.
This research has been supported by the FRACTAL (http://www.fractal.org.za, last access: 25 April 2020) and the AfriCultuReS (http://africultures.eu/, last access: 25 April 2020) projects. FRACTAL (grant agreement no. NE/M020088/1) is part of the multi-consortia Future Climate for Africa (FCFA) programme – jointly funded by the UK Department for International Development (DFID) and the Natural Environment Research Council (NERC). AfriCultuReS has received funding from the European Union Horizon 2020 research and innovation programme under grant agreement no. 774652.
This paper was edited by Vivek Arora and reviewed by John Scinocca and one anonymous referee.
Akinsanola, A. A. and Ogunjobi, K. O.: Evaluation of present-day rainfall simulations over West Africa in CORDEX regional climate models, Environ. Earth Sci., 76, 366, https://doi.org/10.1007/s12665-017-6691-9, 2017.
Bechtold, P., Bazile, E., Guichard, F., Mascart, P., and Richard, E.: A mass-flux convection scheme for regional and global models, Q. J. Roy. Meteor. Soc., 127, 869–886, 2001.
Bechtold, P., Chaboureau, J. P., Beljaars, A., Betts, K., Köhler, M., Miller, M., and Redelsperger, J. L.: The simulation of the diurnal cycle of convective precipitation over land in a global model, Q. J. Roy. Meteor. Soc., 130, 3119–3137, 2004.
Belušić, D., de Vries, H., Dobler, A., Landgren, O., Lind, P., Lindstedt, D., Pedersen, R. A., Sánchez-Perrino, J. C., Toivonen, E., van Ulft, B., Wang, F., Andrae, U., Batrak, Y., Kjellström, E., Lenderink, G., Nikulin, G., Pietikäinen, J.-P., Rodríguez-Camino, E., Samuelsson, P., van Meijgaard, E., and Wu, M.: HCLIM38: a flexible regional climate model applicable for different climate zones from coarse to convection-permitting scales, Geosci. Model Dev., 13, 1311–1333, https://doi.org/10.5194/gmd-13-1311-2020, 2020.
Bengtsson, L., Andrae, U., Aspelien, T., Batrak, Y., Calvo, J., de Rooy, W., Gleeson, E., Hansen-Sass, B., Homleid, M., Hortal, M., Ivarsson, K.-I., Lenderink, G., Niemelä, S., Nielsen, K. P., Onvlee, J., Rontu, L., Samuelsson, P., Muñoz, D. S., Subias, A., Tijm, S., Toll, V., Yang, X., and Køltzow, M. Ø.: The HARMONIE–AROME Model Configuration in the ALADIN–HIRLAM NWP System, Mon. Weather Rev., 145, 1919–1935, 2017.
Berg, P., Döscher, R., and Koenigk, T.: Impacts of using spectral nudging on regional climate model RCA4 simulations of the Arctic, Geosci. Model Dev., 6, 849–859, https://doi.org/10.5194/gmd-6-849-2013, 2013.
Bougeault, P.: A Simple Parameterization of the Large-Scale Effects of Cumulus Convection, Mon. Weather Rev., 113, 2108–2121, 1985.
Caron, L.-P., Jones, C. G., and Winger, K.: Impact of resolution and downscaling technique in simulating recent Atlantic tropical cylone activity, Clim. Dynam., 37, 869–892, 2011.
Castro, C. L., Pielke Sr., R. A., and Leoncini, G.: Dynamical downscaling: Assessment of value retained and added using the Regional Atmospheric Modeling System (RAMS), J. Geophys. Res., 110, D05108, https://doi.org/10.1029/2004JD004721, 2005.
Challinor, A., Wheeler, T., Garforth, C., Craufurd, P., and Kassam, A.: Assessing the vulnerability of food crop systems in Africa to climate change, Climatic Change, 83, 381–399, 2007.
Chan, S. C., Kendon, E. J., Fowler, H. J., Blenkinsop, S., Ferro, C. A. T., and Stephenson, D. B.: Does increasing the spatial resolution of a regional climate model improve the simulated daily precipitation?, Clim. Dynam., 41, 1475–1495, 2013.
Collazo, S., Lhotka, O., Rusticucci, M., and Kyselỳ, J.: Capability of the SMHI-RCA4 RCM driven by the ERA-Interim reanalysis to simulate heat waves in Argentina, Int. J. Climatol., 38, 483–496, 2018.
Covey, C., Gleckler, P. J., Doutriaux, C., Williams, D. N., Dai, A., Fasullo, J., Trenberth, K., and Berg, A.: Metrics for the Diurnal Cycle of Precipitation: Toward Routine Benchmarks for Climate Models, J. Climate, 29, 4461–4471, 2016.
Dai, A.: Precipitation Characteristics in Eighteen Coupled Climate Models, J. Climate, 19, 4605–4630, 2006.
Dai, A. and Trenberth, K. E.: The Diurnal Cycle and Its Depiction in the Community Climate System Model, J. Climate, 17, 930–951, 2004.
Dai, A., Lin, X., and Hsu, K.-L.: The frequency, intensity, and diurnal cycle of precipitation in surface and satellite observations over low- and mid-latitudes, Clim. Dynam., 29, 727–744, 2007.
Daniel, M., Lemonsu, A., Déqué, M., Somot, S., Alias, A., and Masson, V.: Benefits of explicit urban parameterization in regional climate modeling to study climate and city interactions, Clim. Dynam., 52, 2745–2764, 2019.
Da Rocha, R. P., Morales, C. A., Cuadra, S. V., and Ambrizzi, T.: Precipitation diurnal cycle and summer climatology assessment over South America: An evaluation of Regional Climate Model version 3 simulations, J. Geophys. Res., 114, D10108, https://doi.org/10.1029/2008JD010212, 2009.
Dee, D. P., Uppala, S. M., Simmons, A. J., Berrisford, P., Poli, P., Kobayashi, S., Andrae, U., Balmaseda, M. A., Balsamo, G., Bauer, P., Bechtold, P., Beljaars, A. C. M., van de Berg, L., Bidlot, J., Bormann, N., Delsol, C., Dragani, R., Fuentes, M., Geer, A. J., Haimberger, L., Healy, S. B., Hersbach, H., Hólm, E. V., Isaksen, L., Kållberg, P., Köhler, M., Matricardi, M., McNally, A. P., Monge-Sanz, B. M., Morcrette, J.-J., Park, B.-K., Peubey, C., de Rosnay, P., Tavolato, C., Thépaut, J. N., and Vitart, F.: The ERA-Interim reanalysis: Configuration and performance of the data assimilation system, Q. J. Roy. Meteor. Soc., 137, 553–597, 2011.
Diaconescu, E. P. and Laprise, R.: Can added value be expected in RCM-simulated large scales?, Clim. Dynam., 41, 1769–1800, 2013.
Di Luca, A., de Elía, R., and Laprise, R.: Potential for added value in precipitation simulated by high-resolution nested Regional Climate Models and observations, Clim. Dynam., 38, 1229–1247, https://doi.org/10.1007/s00382-011-1068-3, 2012.
Di Luca, A., de Elía, R., and Laprise, R.: Challenges in the Quest for Added Value of Regional Climate Dynamical Downscaling, Current Climate Change Reports, 1, 10–21, 2015.
Dirmeyer, P. A., Cash, B. A., Kinter, J. L., Jung, T., Marx, L., Satoh, M., Stan, C., Tomita, H., Towers, P., Wedi, N., Achuthavarier, D., Adams, J. M., Altshuler, E. L., Huang, B., Jin, E. K., and Manganello, J.: Simulating the diurnal cycle of rainfall in global climate models: resolution versus parameterization, Clim. Dynam., 39, 399–418, 2012.
Dosio, A., Panitz, H.-J., Schubert-Frisius, M., and Lüthi, D.: Dynamical downscaling of CMIP5 global circulation models over CORDEX-Africa with COSMO-CLM: evaluation over the present climate and analysis of the added value, Clim. Dynam., 44, 2637–2661, 2015.
Endris, H. S., Omondi, P., Jain, S., Lennard, C., Hewitson, B., Chang'a, L., Awange, J. L., Dosio, A., Ketiem, P., Nikulin, G., Panitz, H.-J., Büchner, M., Stordal, F., and Tazalika, L.: Assessment of the Performance of CORDEX Regional Climate Models in Simulating East African Rainfall, J. Climate, 26, 8453–8475, 2013.
European Centre for Medium-Range Weather Forecasts (ECMWF): ECMWF Re-Analysis datasets, available at: https://apps.ecmwf.int/datasets/, last access: 20 September 2019.
Favre, A., Philippon, N., Pohl, B., Kalognomou, E.-A., Lennard, C., Hewitson, B., Nikulin, G., Dosio, A., Panitz, H.-J., and Cerezo-Mota, R.: Spatial distribution of precipitation annual cycles over South Africa in 10 CORDEX regional climate model present-day simulations, Clim. Dynam., 46, 1799–1818, 2016.
Fekete, B. M., Vörösmarty, C. J., Roads, J. O., and Willmott, C. J.: Uncertainties in Precipitation and Their Impacts on Runoff Estimates, J. Climate, 17, 294–304, 2004.
Flato, G., Marotzke, J., Abiodun, B., Braconnot, P., Chou, S. C., Collins, W., Cox, P., Driouech, F., Emori, S., Eyring, V., Forest, C., Gleckler, P., Guilyardi, E., Jakob, C., Kattsov, V., Reason, C., and Rummukainen, M.: Evaluation of Climate Models, Climate Change 2013: The Physical Science Basis. Contribution of Working Group I to the Fifth Assessment Report of the Intergovernmental Panel on Climate Change, Cambridge, UK and New York, NY, USA, 741–866, https://doi.org/10.1017/CBO9781107415324, 2013.
Gbobaniyi, E., Sarr, A., Sylla, M. B., Diallo, I., Lennard, C., Dosio, A., Dhiédiou, A., Kamga, A., Klutse, N. A. B., Hewitson, B., Nikulin, G., and Lamptey, B.: Climatology, annual cycle and interannual variability of precipitation and temperature in CORDEX simulations over West Africa, Int. J. Climatol., 34, 2241–2257, 2014.
Giorgi, F. and Gao, X.-J.: Regional earth system modeling: review and future directions, Atmos. Ocean. Sci. Lett., 11, 189–197, 2018.
Giorgi, F. and Mearns, L. O.: Approaches to the simulation of regional climate change: A review, Rev. Geophys., 29, 191–216, https://doi.org/10.1029/90RG02636, 1991.
Giorgi, F., Jones, C., and Asrar, G. R.: Addressing climate information needs at the regional level: the CORDEX framework, WMO Bull., 58, 175–183, 2009.
Giorgi, F., Torma, C., Coppola, E., Ban, N., Schär, C., and Somot, S.: Enhanced summer convective rainfall at Alpine high elevations in response to climate warming, Nat. Geosci., 9, 584–589, 2016.
Global Precipitation Climatology Centre (GPCC): GPCC Landsurface Monitoring Monthly Product, available at: https://www.dwd.de/EN/ourservices/gpcc/gpcc.html, last access: 20 September 2019.
Goddard Space Flight Center (GSFC): The Tropical Rainfall Measuring Mission (TRMM) Multi-satellite Precipitation Analysis, available at: https://pmm.nasa.gov/data-access/downloads/trmm, last access: 20 September 2019.
Gruber, A., Su, X., Kanamitsu, M., and Schemm, J.: The Comparison of Two Merged Rain Gauge–Satellite Precipitation Datasets, B. Am. Meteorol. Soc., 81, 2631–2644, 2000.
Harris, I., Jones, P. D., Osborn, T. J., and Lister, D. H.: Updated high-resolution grids of monthly climatic observations – the CRU TS3. 10 Dataset, Int. J. Climatol., 34, 623–642, 2014.
Hong, S. Y. and Kanamitsu, M.: Dynamical downscaling: Fundamental issues from an NWP point of view and recommendations, Asia-Pac. J. Atmos. Sci., 50, 83–104, 2014.
Huang, X., Rhoades, A. M., Ullrich, P. A., and Zarzycki, C. M.: An evaluation of the variable-resolution CESM for modeling California's climate, J. Adv. Model. Earth Syst., 8, 345–369, 2016.
Huffman, G. J., Bolvin, D. T., Nelkin, E. J., Wolff, D. B., Adler, R. F., Gu, G., Hong, Y., Bowman, K. P., and Stocker, E. F.: The TRMM Multisatellite Precipitation Analysis (TMPA): Quasi-Global, Multiyear, Combined-Sensor Precipitation Estimates at Fine Scales, J. Hydrometeorol., 8, 38–55, 2007.
Iqbal, W., Syed, F. S., Sajjad, H., Nikulin, G., Kjellström, E., and Hannachi, A.: Mean climate and representation of jet streams in the CORDEX South Asia simulations by the regional climate model RCA4, Theor. Appl. Climatol., 129, 1–19, 2017.
Jeong, J.-H., Walther, A., Nikulin, G., Chen, D., and Jones, C.: Diurnal cycle of precipitation amount and frequency in Sweden: observation versus model simulation, Tellus A, 63, 664–674, 2011.
Jiao, Y. and Jones, C.: Comparison Studies of Cloud- and Convection-Related Processes Simulated by the Canadian Regional Climate Model over the Pacific Ocean, Mon. Weather Rev., 136, 4168–4187, 2008.
Jones, C., Willén, U., Ullerstig, A., and Hansson, U.: The Rossby Centre Regional Atmospheric Climate Model part I: model climatology and performance for the present climate over Europe, Ambio, 33, 199–210, 2004.
Jones, C., Giorgi, F., and Asrar, G.: The Coordinated Regional Downscaling Experiment: CORDEX – an international downscaling link to CMIP5, CLIVAR exchanges, 16, 34–40, 2011.
Jones, P. W.: First- and Second-Order Conservative Remapping Schemes for Grids in Spherical Coordinates, Mon. Weather Rev., 127, 2204–2210, 1999.
Kalognomou, E.-A., Lennard, C., Shongwe, M., Pinto, I., Favre, A., Kent, M., Hewitson, B., Dosio, A., Nikulin, G., Panitz, H.-J., and Büchner, M.: A Diagnostic Evaluation of Precipitation in CORDEX Models over Southern Africa, J. Climate, 26, 9477–9506, 2013.
Kim, J., Waliser, D. E., Mattmann, C. A., Goodale, C. E., Hart, A. F., Zimdars, P. A., Crichton, D. J., Jones, C., Nikulin, G., Hewitson, B., Jack, C., Lennard, C., and Favre, A.: Evaluation of the CORDEX-Africa multi-RCM hindcast: systematic model errors, Clim. Dynam., 42, 1189–1202, 2014.
Kisembe, J., Favre, A., Dosio, A., Lennard, C., Sabiiti, G., and Nimusiima, A.: Evaluation of rainfall simulations over Uganda in CORDEX regional climate models, Theor. Appl. Climatol., 137, 1117–1134, 2019.
Kjellström, E., Bärring, L., Gollvik, S., Hansson, U., Jones, C., Samuelsson, P., Ullerstig, A., Willén, U., and Wyser, K.: A 140-year simulation of European climate with the new version of the Rossby Centre regional atmospheric climate model (RCA3), available at: http://www.diva-portal.org/smash/record.jsf?pid=diva2:947602 (last access: 19 November 2018), 2005.
Kjellström, E., Bärring, L., Nikulin, G., Nilsson, C., Persson, G., and Strandberg, G.: Production and use of regional climate model projections – A Swedish perspective on building climate services, Clim. Serv., 2–3, 15–29, 2016.
Kjellström, E., Nikulin, G., Strandberg, G., Christensen, O. B., Jacob, D., Keuler, K., Lenderink, G., van Meijgaard, E., Schär, C., Somot, S., Sørland, S. L., Teichmann, C., and Vautard, R.: European climate change at global mean temperature increases of 1.5 and 2 °C above pre-industrial conditions as simulated by the EURO-CORDEX regional climate models, Earth Syst. Dynam., 9, 459–478, https://doi.org/10.5194/esd-9-459-2018, 2018.
Klutse, N. A. B., Sylla, M. B., Diallo, I., Sarr, A., Dosio, A., Diedhiou, A., Kamga, A., Lamptey, B., Ali, A., Gbobaniyi, E. O., Owusu, K., Lennard, C., Hewitson, B., Nikulin, G., Panitz, H.-J., and Büchner, M.: Daily characteristics of West African summer monsoon precipitation in CORDEX simulations, Theor. Appl. Climatol., 123, 369–386, 2016.
Koenigk, T., Berg, P., and Döscher, R.: Arctic climate change in an ensemble of regional CORDEX simulations, Polar Res., 34, 24603, https://doi.org/10.3402/polar.v34.24603, 2015.
Kotlarski, S., Lüthi, D., and Schär, C.: The elevation dependency of 21st century European climate change: an RCM ensemble perspective, Int. J. Climatol., 35, 3902–3920, 2015.
Laprise, R.: Regional climate modelling, J. Comput. Phys., 227, 3641–3666, 2008.
Legates, D. R. and Willmott, C. J.: Mean seasonal and spatial variability in global surface air temperature, Theor. Appl. Climatol., 41, 11–21, 1990.
Lenderink, G. and Holtslag, A. A. M.: An updated length-scale formulation for turbulent mixing in clear and cloudy boundary layers, Q. J. Roy. Meteor. Soc., 130, 3405–3427, 2004.
Liang, X.-Z.: Regional climate model simulation of summer precipitation diurnal cycle over the United States, Geophys. Res. Lett., 31, 2033, https://doi.org/10.1029/2004GL021054, 2004.
Lindstedt, D., Lind, P., Kjellström, E., and Jones, C.: A new regional climate model operating at the meso-gamma scale: Performance over Europe, Tellus A, 67, 24138, https://doi.org/10.3402/tellusa.v67.24138, 2015.
Lucas-Picher, P., Caya, D., de Elía, R., and Laprise, R.: Investigation of regional climate models' internal variability with a ten-member ensemble of 10-year simulations over a large domain, Clim. Dynam., 31, 927–940, 2008.
Lucas-Picher, P., Laprise, R., and Winger, K.: Evidence of added value in North American regional climate model hindcast simulations using ever-increasing horizontal resolutions, Clim. Dynam., 48, 2611–2633, 2017.
Masson, V., Le Moigne, P., Martin, E., Faroux, S., Alias, A., Alkama, R., Belamari, S., Barbu, A., Boone, A., Bouyssel, F., Brousseau, P., Brun, E., Calvet, J.-C., Carrer, D., Decharme, B., Delire, C., Donier, S., Essaouini, K., Gibelin, A.-L., Giordani, H., Habets, F., Jidane, M., Kerdraon, G., Kourzeneva, E., Lafaysse, M., Lafont, S., Lebeaupin Brossier, C., Lemonsu, A., Mahfouf, J.-F., Marguinaud, P., Mokhtari, M., Morin, S., Pigeon, G., Salgado, R., Seity, Y., Taillefer, F., Tanguy, G., Tulet, P., Vincendon, B., Vionnet, V., and Voldoire, A.: The SURFEXv7.2 land and ocean surface platform for coupled or offline simulation of earth surface variables and fluxes, Geosci. Model Dev., 6, 929–960, https://doi.org/10.5194/gmd-6-929-2013, 2013.
Moufouma-Okia, W. and Jones, R.: Resolution dependence in simulating the African hydroclimate with the HadGEM3-RA regional climate model, Clim. Dynam., 44, 609–632, 2015.
Munday, C. and Washington, R.: Systematic Climate Model Rainfall Biases over Southern Africa: Links to Moisture Circulation and Topography, J. Climate, 31, 7533–7548, 2018.
Nikulin, G., Jones, C., Giorgi, F., Asrar, G., Büchner, M., Cerezo-Mota, R., Christensen, O. B., Déqué, M., Fernandez, J., Hänsler, A., van Meijgaard, E., Samuelsson, P., Sylla, M. B., and Sushama, L.: Precipitation climatology in an ensemble of CORDEX-Africa regional climate simulations, J. Climate, 25, 6057–6078, 2012.
Nikulin, G., Lennard, C., Dosio, A., Kjellström, E., Chen, Y., Hänsler, A., Kupiainen, M., Laprise, R., Mariotti, L., Maule, C. F., van Meijgaard, E., Panitz, H.-J., Scinocca, J. F., and Somot, S.: The effects of 1.5 and 2 degrees of global warming on Africa in the CORDEX ensemble, Environ. Res. Lett., 13, 065003, https://doi.org/10.1088/1748-9326/aab1b1, 2018.
Olsson, J., Berg, P., and Kawamura, A.: Impact of RCM Spatial Resolution on the Reproduction of Local, Subdaily Precipitation, J. Hydrometeorol., 16, 534–547, 2015.
Panitz, H.-J., Dosio, A., Büchner, M., Lüthi, D., and Keuler, K.: COSMO-CLM (CCLM) climate simulations over CORDEX-Africa domain: analysis of the ERA-Interim driven simulations at 0.44∘ and 0.22∘ resolution, Clim. Dynam., 42, 3015–3038, 2014.
Prein, A. F., Gobiet, A., Truhetz, H., Keuler, K., Goergen, K., Teichmann, C., Maule, C. F., Van Meijgaard, E., Déqué, M., Nikulin, G., Vautard, R., Colette, A., Kjellström, E., and Jacob, D.: Precipitation in the EURO-CORDEX 0. 11∘ and 0. 44∘ simulations: high resolution, high benefits?, Clim. Dynam., 46, 383–412, 2016.
Räisänen, J., Hansson, U., Ullerstig, A., Döscher, R., Graham, L. P., Jones, C., Meier, H. E. M., Samuelsson, P., and Willén, U.: European climate in the late twenty-first century: regional simulations with two driving global models and two forcing scenarios, Clim. Dynam., 22, 13–31, 2004.
Rana, A., Nikulin, G., Kjellström, E., Strandberg, G., Kupiainen, M., Hansson, U., and Kolax, M.: Contrasting regional and global climate simulations over South Asia, Clim. Dynam., 54, 2883–2901, https://doi.org/10.1007/s00382-020-05146-0, 2020.
Rummukainen, M.: State-of-the-art with regional climate models, WIREs Clim. Change, 1, 82–96, 2010.
Rummukainen, M.: Added value in regional climate modeling, WIREs Clim. Change, 7, 145–159, 2016.
Rummukainen, M., Räisänen, J., Bringfelt, B., Ullerstig, A., Omstedt, A., Willén, U., Hansson, U., and Jones, C.: A regional climate model for northern Europe: model description and results from the downscaling of two GCM control simulations, Clim. Dynam., 17, 339–359, 2001.
Samuelsson, P., Jones, C. G., Willén, U., Ullerstig, A., Gollvik, S., Hansson, U., Jansson, E., Kjellström, C., Nikulin, G., and Wyser, K.: The Rossby Centre Regional Climate model RCA3: model description and performance, Tellus A, 63, 4–23, 2011.
Sanchez-Gomez, E. and Somot, S.: Impact of the internal variability on the cyclone tracks simulated by a regional climate model over the Med-CORDEX domain, Clim. Dynam., 51, 1005–1021, 2018.
Sato, T., Miura, H., Satoh, M., Takayabu, Y. N., and Wang, Y.: Diurnal Cycle of Precipitation in the Tropics Simulated in a Global Cloud-Resolving Model, J. Climate, 22, 4809–4826, 2009.
Schneider, U., Becker, A., Finger, P., Meyer-Christoffer, A., Ziese, M., and Rudolf, B.: GPCC's new land surface precipitation climatology based on quality-controlled in situ data and its role in quantifying the global water cycle, Theor. Appl. Climatol., 115, 15–40, 2014.
Scinocca, J. F., Kharin, V. V., Jiao, Y., Qian, M. W., Lazare, M., Solheim, L., Flato, G. M., Biner, S., Desgagne, M., and Dugas, B.: Coordinated Global and Regional Climate Modeling, J. Climate, 29, 17–35, 2016.
Shongwe, M. E., Lennard, C., Liebmann, B., Kalognomou, E.-A., Ntsangwane, L., and Pinto, I.: An evaluation of CORDEX regional climate models in simulating precipitation over Southern Africa: CORDEX simulation of rainfall over Southern Africa, Atmos. Sci. Lett., 16, 199–207, 2015.
Sørland, S. L., Schär, C., Lüthi, D., and Kjellström, E.: Bias patterns and climate change signals in GCM-RCM model chains, Environ. Res. Lett., 13, 074017, https://doi.org/10.1088/1748-9326/aacc77, 2018.
Sylla, M. B., Giorgi, F., Coppola, E., and Mariotti, L.: Uncertainties in daily rainfall over Africa: assessment of gridded observation products and evaluation of a regional climate model simulation, Int. J. Climatol., 33, 1805–1817, 2013a.
Sylla, M. B., Diallo, I., and Pal, J. S.: West African Monsoon in State-of-the-Science Regional Climate Models, in Climate Variability – Regional and Thematic Patterns, edited by: Tarhule, A., InTech., London, UK, 2013b.
Tamoffo, A. T., Moufouma-Okia, W., Dosio, A., James, R., Pokam, W. M., Vondou, D. A., Fotso-Nguemo, T. C., Guenang, G. M., Kamsu-Tamo, P. H., Nikulin, G., Longandjo, G.-N., Lennard, C. J., Bell, J.-P., Takong, R. R., Haensler, A., Tchotchou, L. A. D., and Nouayou, R.: Process-oriented assessment of RCA4 regional climate model projections over the Congo Basin under 1.5 ∘C and 2 ∘C global warming levels: influence of regional moisture fluxes, Clim. Dynam., 53, 1911–1935, https://doi.org/10.1007/s00382-019-04751-y, 2019.
Tangang, F., Supari, S., Chung, J. X., Cruz, F., Salimun, E., Ngai, S. T., Juneng, L., Santisirisomboon, J., Santisirisomboon, J., Ngo-Duc, T., Phan-Van, T., Narisma, G., Singhruck, P., Gunawan, D., Aldrian, E., Sopaheluwakan, A., Nikulin, G., Yang, H., Remedio, A. R. C., Sein, D., and Hein-Griggs, D.: Future changes in annual precipitation extremes over Southeast Asia under global warming of 2 ∘C, APN Science Bulletin, 8, 3–8,, https://doi.org/10.30852/sb.2018.436, 2018.
Temperton, C., Hortal, M., and Simmons, A.: A two-time-level semi-Lagrangian global spectral model, Q. J. Roy. Meteor. Soc., 127, 111–127, 2001.
Termonia, P., Fischer, C., Bazile, E., Bouyssel, F., Brožková, R., Bénard, P., Bochenek, B., Degrauwe, D., Derková, M., El Khatib, R., Hamdi, R., Mašek, J., Pottier, P., Pristov, N., Seity, Y., Smolíková, P., Španiel, O., Tudor, M., Wang, Y., Wittmann, C., and Joly, A.: The ALADIN System and its canonical model configurations AROME CY41T1 and ALARO CY40T1, Geosci. Model Dev., 11, 257–281, https://doi.org/10.5194/gmd-11-257-2018, 2018.
Tiedtke, M.: A Comprehensive Mass Flux Scheme for Cumulus Parameterization in Large-Scale Models, Mon. Weather Rev., 117, 1779–1800, 1989.
Torma, C., Giorgi, F., and Coppola, E.: Added value of regional climate modeling over areas characterized by complex terrain-Precipitation over the Alps, J. Geophys. Res.-Atmos., 120, 3957–3972, 2015.
Undén, P., Rontu, L., Jäarvinen, H., Lynch, P., and Calvo, J.: HIRLAM-5 scientific documentation, SMHI, SMHI, SE-601 76 Norrköping, Sweden, 2002.
University of Delaware: Terrestrial Air Temperature and Precipitation: Monthly Climatologies (V 4.01), available at: http://climate.geog.udel.edu/~climate/html_pages/download.html, last access: 20 September 2019.
University of East Anglia (UEA): Climatic Research Unit (CRU) Time-Series (TS) Version 3.23 of High Resolution Gridded Data of Month-by-month Variation in Climate, available at: https://catalogue.ceda.ac.uk/uuid/5dca9487dc614711a3a933e44a933ad3, last access: 20 September 2019.
Van der Linden, P. and Mitchell, J. F.: ENSEMBLES: Climate change and its impacts-Summary of research and results from the ENSEMBLES project, Met Office Hadley Centre, Exeter, UK, 2009.
Walther, A., Jeong, J.-H., Nikulin, G., Jones, C., and Chen, D.: Evaluation of the warm season diurnal cycle of precipitation over Sweden simulated by the Rossby Centre regional climate model RCA3, Atmos. Res., 119, 131–139, 2013.
Wang, J. and Kotamarthi, V. R.: Downscaling with a nested regional climate model in near-surface fields over the contiguous United States: WRF dynamical downscaling, J. Geophys. Res.-Atmos., 119, 8778–8797, 2014.
Washington, R., James, R., Pearce, H., Pokam, W. M., and Moufouma-Okia, W.: Congo Basin rainfall climatology: can we believe the climate models?, Philos. T. Roy. Soc. B, 368, 1625, https://doi.org/10.1098/rstb.2012.0296, 2013.
Wu, M., Schurgers, G., Rummukainen, M., Smith, B., Samuelsson, P., Jansson, C., Siltberg, J., and May, W.: Vegetation–climate feedbacks modulate rainfall patterns in Africa under future climate change, Earth Syst. Dynam., 7, 627–647, https://doi.org/10.5194/esd-7-627-2016, 2016.
Wu, M., Schurgers, G., Ahlström, A., Rummukainen, M., Miller, P. A., Smith, B., and May, W.: Impacts of land use on climate and ecosystem productivity over the Amazon and the South American continent, Environ. Res. Lett., 12, 054016, https://doi.org/10.1088/1748-9326/aa6fd6, 2017.
Xue, Y., Janjic, Z., Dudhia, J., Vasic, R., and De Sales, F.: A review on regional dynamical downscaling in intraseasonal to seasonal simulation/prediction and major factors that affect downscaling ability, Atmos. Res., 147–148, 68–85, 2014.
Zhang, W., Jansson, C., Miller, P. A., Smith, B., and Samuelsson, P.: Biogeophysical feedbacks enhance the Arctic terrestrial carbon sink in regional Earth system dynamics, Biogeosciences, 11, 5503–5519, https://doi.org/10.5194/bg-11-5503-2014, 2014.