the Creative Commons Attribution 4.0 License.
the Creative Commons Attribution 4.0 License.
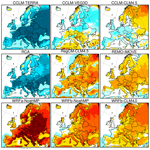
Biogeophysical impacts of forestation in Europe: first results from the LUCAS (Land Use and Climate Across Scales) regional climate model intercomparison
Edouard L. Davin
Diana Rechid
Marcus Breil
Rita M. Cardoso
Erika Coppola
Peter Hoffmann
Lisa L. Jach
Eleni Katragkou
Nathalie de Noblet-Ducoudré
Kai Radtke
Mario Raffa
Pedro M. M. Soares
Giannis Sofiadis
Susanna Strada
Gustav Strandberg
Merja H. Tölle
Kirsten Warrach-Sagi
Volker Wulfmeyer
The Land Use and Climate Across Scales Flagship Pilot Study (LUCAS FPS) is a coordinated community effort to improve the integration of land use change (LUC) in regional climate models (RCMs) and to quantify the biogeophysical effects of LUC on local to regional climate in Europe. In the first phase of LUCAS, nine RCMs are used to explore the biogeophysical impacts of re-/afforestation over Europe: two idealized experiments representing respectively a non-forested and a maximally forested Europe are compared in order to quantify spatial and temporal variations in the regional climate sensitivity to forestation. We find some robust features in the simulated response to forestation. In particular, all models indicate a year-round decrease in surface albedo, which is most pronounced in winter and spring at high latitudes. This results in a winter warming effect, with values ranging from +0.2 to +1 K on average over Scandinavia depending on models. However, there are also a number of strongly diverging responses. For instance, there is no agreement on the sign of temperature changes in summer with some RCMs predicting a widespread cooling from forestation (well below −2 K in most regions), a widespread warming (around +2 K or above in most regions) or a mixed response. A large part of the inter-model spread is attributed to the representation of land processes. In particular, differences in the partitioning of sensible and latent heat are identified as a key source of uncertainty in summer. Atmospheric processes, such as changes in incoming radiation due to cloud cover feedbacks, also influence the simulated response in most seasons. In conclusion, the multi-model approach we use here has the potential to deliver more robust and reliable information to stakeholders involved in land use planning, as compared to results based on single models. However, given the contradictory responses identified, our results also show that there are still fundamental uncertainties that need to be tackled to better anticipate the possible intended or unintended consequences of LUC on regional climates.
- Article
(20261 KB) - Full-text XML
-
Supplement
(36301 KB) - BibTeX
- EndNote
Land use change (LUC) affects climate through biogeophysical processes influencing surface albedo, evapotranspiration and surface roughness (Bonan, 2008; Davin and de Noblet-Ducoudré, 2010). The quantification of these effects is still subject to particularly large uncertainties, but there is growing evidence that LUC is an important driver of climate change at local to regional scales. For instance, the Land-Use and Climate, IDentification of robust impacts (LUCID) model intercomparison indicated that while LUC likely had a modest biogeophysical impact on global temperature since the pre-industrial era, it may have affected temperature in a similar proportion to greenhouse gas forcing in some regions (de Noblet-Ducoudré et al., 2012). Results from the Coupled Model Intercomparison Project Phase 5 (CMIP5) confirmed the importance of LUC for regional climate trends and for temperature extremes (Kumar et al., 2013; Lejeune et al., 2017, 2018).
In this light, it is particularly important to represent LUC forcings not only in global climate models but also in regional climate simulations. Yet, LUC forcings were not included in previous regional climate model (RCM) intercomparisons (Christensen and Christensen, 2007; Jacob et al., 2014; Mearns et al., 2012; Solman et al., 2013), which are the basis for numerous regional climate change assessments providing information for impact studies and the design of adaptation plans (Gutowski Jr. et al., 2016). RCMs have been applied individually to explore different aspects of land use impacts on regional climates (Davin et al., 2014; Gálos et al., 2013; Lejeune et al., 2015; Tölle et al., 2018; Wulfmeyer et al., 2014), but the robustness of such results is difficult to assess due to their reliance on single RCMs and due to the lack of a common protocol. There is therefore a need for a coordinated effort to better integrate LUC effects in RCM projections. The Land Use and Climate Across Scales (LUCAS) initiative (https://www.hzg.de/ms/cordex_fps_lucas/, last access: 10 February 2020) has been designed with this goal in mind. LUCAS is endorsed as a Flagship Pilot Study (FPS) by the World Climate Research Program-Coordinated Regional Climate Downscaling Experiment (WCRP-CORDEX) and was initiated by the European branch of CORDEX (EURO-CORDEX) (Rechid et al., 2017). The objectives of the LUCAS FPS are to promote the inclusion of the missing LUC forcing in RCM multi-model experiments and to identify the associated impacts with a focus on regional to local scales and considering timescales from extreme events to seasonal and multi-decadal trends and variability. LUCAS is designed in successive phases that will go from idealized to realistic high-resolution scenarios and intends to cover both land cover changes and land management impacts.
In the first phase of LUCAS, which is the focus of this study, idealized experiments over Europe are performed in order to benchmark the RCM sensitivity to extreme LUC. Two experiments (FOREST and GRASS) are performed using a set of nine RCMs. The FOREST experiment represents a maximally forested Europe, while in the GRASS experiment trees are replaced by grassland. Comparing FOREST to GRASS therefore indicates the theoretical potential of a maximum-forestation (encompassing both reforestation and afforestation) scenario over Europe. Given that forestation is one of the most prominent land-based mitigation strategies put forward in scenarios compatible with the Paris Agreement goals (Grassi et al., 2017; Griscom et al., 2017; Harper et al., 2018), it is essential to understand its full consequences beyond CO2 mitigation. These experiments are not meant to represent realistic scenarios, but they enable a systematic assessment and mapping of the biogeophysical impact of forestation across regions and seasons. Experiments of this type have already been performed using single regional or global climate models (Cherubini et al., 2018; Claussen et al., 2001; Davin and de Noblet-Ducoudré, 2010; Strandberg et al., 2018), but here they are performed for the first time using a multi-model ensemble approach, thus providing an unprecedented opportunity to assess uncertainties in the climate response to vegetation perturbations. In the following, we focus on the analysis of the surface energy balance and temperature response at the seasonal timescale, while future studies within LUCAS will explore further aspects (sub-daily timescale and extreme events, land–atmosphere coupling, etc.). We aim to quantify the potential effect of forestation over Europe, identify robust model responses, and investigate the possible sources of uncertainty in the simulated impacts.
2.1 RCM ensemble
Two experiments (GRASS and FOREST) were performed with an ensemble of nine RCMs, whose names and characteristics are presented in Table 1. All experiments were performed at 0.44∘ (∼50 km) horizontal resolution on the EURO-CORDEX domain (Jacob et al., 2014) with lateral boundary conditions and sea surface temperatures prescribed based on 6-hourly ERA-Interim reanalysis (Dee et al., 2011). The simulations are analysed over the period 1986–2015, and the earlier years (1979–1985 or a subset of these years depending on models; see Table 1) were used as spin-up period. The model outputs were aggregated to monthly values for use in this study. When showing results averaged across all nine RCMs, we refer to it as the multi-model mean (MMM).
A notable characteristic of the multi-model ensemble is that some RCMs share the same atmospheric scheme (i.e. same version and configuration) but are coupled to different land surface models (LSMs) or share the same LSM in combination with different atmospheric schemes (see Table 1). This allows us to evaluate the respective influence of atmospheric versus land process representation. For instance, the same version of COSMO-CLM (CCLM) is used in combination with three different LSMs (TERRA_ML, VEG3D and CLM4.5). Comparing results from these three CCLM-based configurations enables us to isolate the role of land process representation in this particular model. Conversely, CLM4.5 is used in combination with two different RCMs (CCLM and RegCM), which allows us to diagnose the influence of atmospheric processes on the results. Different configurations of WRF (Weather Research and Forecasting) are also used: WRFa-NoahMP and WRFb-NoahMP differ only in their atmospheric set-up, while WRFb-NoahMP and WRFb-CLM4.0 share the same atmospheric set-up but with different LSMs.
While the simulations we present are not suitable for model evaluation because of the idealized land cover characteristics, it is worthwhile to note that the RCMs included here have been part of previous evaluation studies over Europe (e.g. Kotlarski et al., 2014; Davin et al., 2016). Although for a given RCM the model version and configuration may differ from previously evaluated configurations, the systematic biases highlighted in these previous studies are likely still relevant here. In particular, a majority of RCMs suffer from predominantly cold and wet biases in most European regions, while the opposite is true in summer in Mediterranean regions (Kotlarski et al., 2014). The conditions that are too dry over southern Europe have been related in particular to land surface process representation including evapotranspiration (Davin et al., 2016).
2.2 FOREST and GRASS vegetation maps
Two vegetation maps have been created for use in the Phase 1 LUCAS experiments (Fig. S1 in the Supplement). The vegetation map used in the experiment FOREST is meant to represent a theoretical maximum of tree coverage, while in the vegetation map used in the experiment GRASS, trees are entirely replaced by grassland.
The starting point for both maps is a MODIS-based present-day land cover map at 0.5∘ resolution (Lawrence and Chase, 2007) providing the global distribution of 17 plant functional types (PFTs). Crops and shrubs which are present in the original map are not considered in the FOREST and GRASS experiments and are set to zero. To create the FOREST map, the fractional coverage of trees is expanded until trees occupy 100 % of the non-bare soil area. The proportion of various tree types (i.e. broadleaf to needleleaf and deciduous to evergreen) is conserved as in the original map as well as the fractional coverage of bare soil, which prevents expanding vegetation on land areas where it could not realistically grow (e.g. in deserts). If no trees are present in a given grid cell with less than 100 % bare soil, the zonal mean forest composition is taken as a representative value. This results in a map with only tree PFTs (PFT names) and bare soil, all other vegetation types being shrunk to zero. It is important to note that this FOREST map does not represent a potential vegetation map, which would imply a more conservative assumption in terms of forest expansion potential. Indeed, trees can grow even in regions where they would not naturally occur because of various human interventions (assisted afforestation, forest management, fire suppression, etc.). This FOREST map is therefore in line with the idea of considering both reforestation and afforestation potential, while still excluding forest expansion over dryland regions where irrigation measures would likely be necessary.
The GRASS map is then derived from the FOREST map by converting all tree PFTs into grassland PFTs, the C3-to-C4 ratio being conserved as in the original MODIS-based map as well as the bare soil fraction.
Since the various RCMs use different land use classification schemes (see Table 1), the PFT-based FOREST and GRASS maps were converted into model-specific land use classes for implementation into the respective RCMs. The specific conversion rules used in each RCM are summarized in Table 1 (note that for three out of the nine RCMs, no conversion was required). Urban areas, inland water and glacier, if included in a given RCM, were conserved as in the standard dataset of the respective RCM.
Table 1Names and characteristics of the RCMs used. NET-Temperate: needleleaf evergreen tree – temperate; NET-Boreal: needleleaf evergreen tree – boreal; NDT-Boreal: needleleaf deciduous tree – boreal; BET-Tropical: broadleaf evergreen tree – tropical; BET-Temperate: broadleaf evergreen tree – temperate; BDT-Tropical: broadleaf deciduous tree – tropical; BDT-Temperate: broadleaf deciduous tree – temperate; BDT-Boreal: broadleaf deciduous tree – boreal; BES-Temperate: broadleaf evergreen shrub – temperate; BDS-Temperate: broadleaf deciduous shrub – temperate; BDS-Boreal: broadleaf deciduous shrub – boreal. Institution IDs are as follows: JLU – Justus-Liebig-Universität Gießen; BTU: Brandenburgische Technische Universität; KIT – Karlsruhe Institute of Technology; ETH – Eidgenössische Technische Hochschule Zürich; SMHI – Swedish Meteorological and Hydrological Institute; ICTP – International Centre for Theoretical Physics; GERICS – Climate Service Center Germany; IDL – Instituto Amaro Da Costa; UHOH – University of Hohenheim; AUTH – Aristotle University of Thessaloniki.
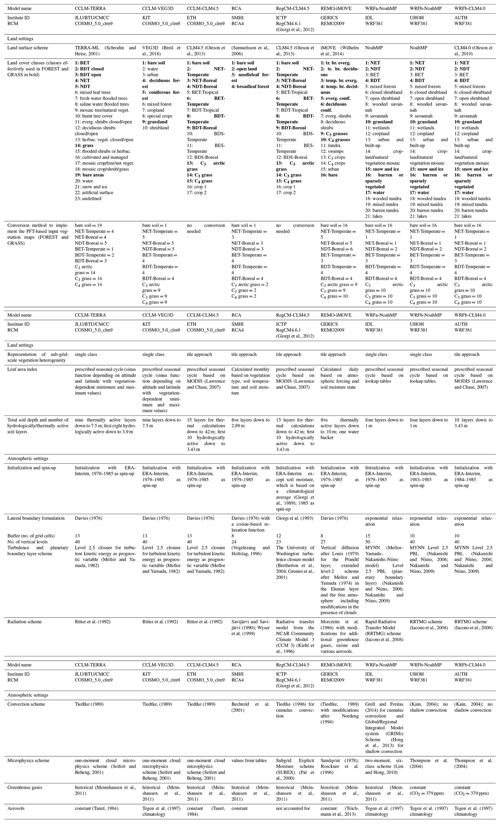
3.1 Temperature response
The effect of forestation (FOREST minus GRASS) on seasonal mean winter 2 m temperature is shown in Fig. 1. All RCMs simulate a warming pattern which is strongest in the northeast of Europe. This warming effect weakens toward the southwest of the domain even changing sign for instance in the Iberian Peninsula (except for REMO-iMOVE). In summer (Fig. 2), there is a very large spread of model responses with some RCMs predicting a widespread cooling from forestation (CCLM-TERRA and RCA), a widespread warming (RegCM-CLM4.5, REMO-iMOVE and the WRF models) or a mixed response (CCLM-VEG3D and CCLM-CLM4.5). Overall this highlights the strong seasonal contrasts in the temperature effect of forestation and the larger uncertainties associated with the summer response.
Looking separately at the response for daytime and nighttime 2 m temperatures also indicates important diurnal contrasts. The winter warming effect is stronger and more widespread for daily maximum temperature (Fig. 3), while daily minimum temperature shows a more contrasted cooling–warming dipole across the domain (Fig. 5). In summer, diurnal contrasts are even more pronounced with a majority of models showing an opposite sign of change for daily maximum and minimum temperatures over most of Europe (Figs. 4 and 6), namely a daytime warming effect and a nighttime cooling effect. Exceptions are RCA and CCLM-TERRA, which indicate a cooling for both daily maximum and minimum temperatures and REMO-iMOVE exhibiting a warming for both daytime and nighttime.
In terms of magnitude, the temperature signal is substantial. In all RCMs, there is at least one season with absolute temperature changes above 2∘ in some regions, for instance in winter and spring over northern Europe (Fig. S2). The magnitude of changes is even more pronounced for daily maximum temperature.
3.2 Surface energy balance
Changes in surface energy fluxes over land are summarized for eight European regions (the Alps, the British Isles, eastern Europe, France, the Iberian Peninsula, the Mediterranean, mid-Europe and Scandinavia) as defined in the PRUDENCE project (Christensen et al., 2007). Here we discuss results for two selected regions representative of northern Europe (Scandinavia; Fig. 9) and southern Europe (the Mediterranean; Fig. 10), while results for the full set of regions are provided in the Supplement (Figs. S11 to S18). One of the most robust features across models and seasons is an increase in surface net shortwave radiation. This increase is a direct consequence of the impact of forestation on surface albedo. Indeed all RCMs consistently simulate a year-round decrease in surface albedo due to the lower albedo of forest compared to grassland (Fig. S7). This decrease is strongest in winter and at high latitudes owing to the snow-masking effect of forest. However, the strongest increase in net shortwave radiation occurs in spring and summer in both regions because incoming radiation is higher in these seasons, thus implying a larger surface radiation gain despite the smaller absolute change in albedo. Notable outliers are REMO-iMOVE, exhibiting a smaller albedo decrease across all seasons and thus a less pronounced increase in net shortwave radiation, and CCLM-TERRA and RCA, which despite the albedo increase simulate a net shortwave radiation decrease in summer (only over Scandinavia in the case of RCA). In the latter two models, an increase in evapotranspiration triggers an increase in cloud cover and a subsequent decrease in incoming shortwave radiation (not shown) offsetting the change in surface albedo. The spatial pattern of surface net shortwave radiation change is relatively consistent across RCMs in winter with maximum net shortwave radiation increases well above 10 W m−2 in high-elevation regions and the northeast of Europe (Fig. 7). In summer, the magnitude of net shortwave radiation changes is overall larger as is the inter-model spread (Fig. 8). CCLM-TERRA is the only RCM to simulate a widespread decrease in net shortwave radiation, while RCA and CCLM-VEG3 also simulate net shortwave radiation decreases in some areas in particular in northern Europe. All other RCMs simulate a widespread increase in net shortwave radiation over land, with WRFa-NoahMP and WRFb-NoahMP exhibiting the strongest increase with values well above 20 W m−2 in most regions.
To a large extent, sensible heat flux follows shortwave radiation changes (i.e. a majority of models suggest an increase in sensible heat). This is also largely the case for ground heat flux (calculated here indirectly as the residual of the surface energy balance), which increases in autumn, winter and spring in most models due to the overall increase in absorbed radiation. Changes in the latent heat flux exhibit a higher degree of disagreement across models and seasons. For instance in spring, latent heat flux increases together with sensible heat over Scandinavia (Fig. 9), while it decreases in most models over the Mediterranean (Fig. 10). In summer, the agreement is low over Scandinavia, and there is a tendency for decreasing latent heat in the Mediterranean. At the European scale, there is a clear tendency of increasing latent heat flux in spring particularly over northern Europe, whereas in summer most RCMs (with the exception of CCLM-TERRA) indicate both increasing and decreasing latent heat depending on regions (Fig. S10).
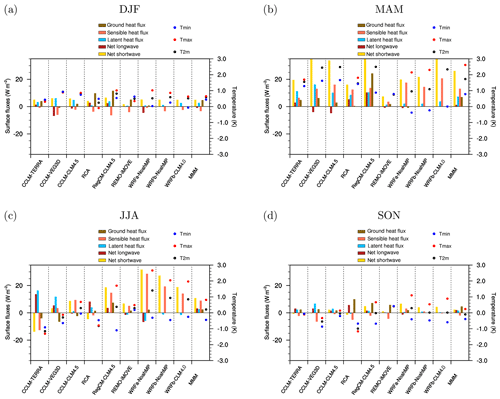
Figure 9Changes in temperature and in surface energy balance components (FOREST minus GRASS) averaged over Scandinavia for DJF, MAM, JJA and SON. Results for other regions are shown in the Supplement.
3.3 Origin of the inter-model spread
Changes in albedo and in the partitioning of turbulent heat fluxes are essential in determining the temperature effect of forestation. The dominant influence of albedo decrease is evident in winter and spring over northern Europe as illustrated for instance by the quasilinear inter-model relationship between the magnitude of changes in albedo and in 2 m temperature over Scandinavia in spring (Fig. 11a). The role of turbulent heat fluxes partitioning can be illustrated by examining changes in evaporative fraction (EF), calculated as the ratio between latent heat and the sum of latent and sensible heat. The advantage of using EF instead of latent heat flux is that the former provides a metric relatively independent of albedo change (since albedo change does influence the magnitude of turbulent heat fluxes through changes in available energy). Taking the example of Scandinavia in summer (Fig. 11b), it appears that there is a relatively linear relationship between changes in temperature and in EF. In other words, models showing a decrease in EF following forestation tend to simulate a warming and models showing an increase in EF simulate a cooling.
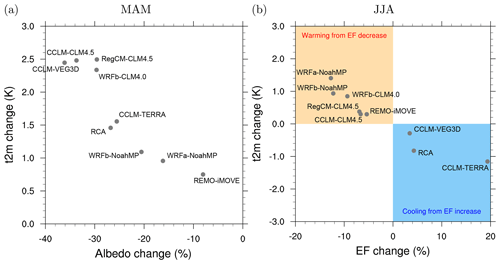
Figure 11Illustrative relationships between changes (FOREST minus GRASS) in 2 m temperature and albedo in spring (a) and between changes in 2 m temperature and EF (evaporative fraction) in summer (b) for Scandinavia.
In order to assess more systematically the role of individual drivers across regions and seasons, we perform a regression analysis using changes in albedo, EF and incoming surface shortwave radiation as explanatory variables and 2 m temperature as the variable to be explained. The rationale for using albedo, EF and incoming surface shortwave radiation as explaining factors is that the first two capture the intrinsic LUC-induced changes in land surface characteristics representing respectively the radiative and non-radiative impacts of LUC, whereas incoming surface shortwave radiation captures some of the potential subsequent atmospheric feedbacks (e.g. through cloud cover changes). Here we discuss the results of the regression analysis for Scandinavia and the Mediterranean (Fig. 12), while results for the full set of regions are provided in the Supplement (Figs. S19 and S20). Combining albedo, EF and incoming surface shortwave radiation into a multiple linear regression effectively explains a large fraction of the inter-model variance of the simulated temperature response (around 80 % of variance explained for both regions and all seasons except winter where the explained variance is much lower). Albedo change alone explains the largest part of the inter-model variance in spring over Scandinavia and in winter over the Mediterranean, indicating a dominance of radiative processes during these seasons. EF change alone explains the largest part of the inter-model variance in summer over Scandinavia and in spring, summer and autumn over the Mediterranean. Finally, incoming surface shortwave radiation explains a substantial part of the inter-model variance across most seasons although it is not a dominating factor. It is important to note the two main caveats of this simplified approach: (1) the explanatory variables are likely not fully independent due to the tightly coupled processes in the models; (2) other factors not included as explanatory variables may contribute to the temperature response (e.g. changes in surface roughness, other atmospheric feedbacks). Nevertheless, the fact that a large part of the variance can be explained by this simple linear model is an indication of the essential role of these selected processes. An exception is the winter season during which a very limited part of the inter-model spread can be explained, suggesting that other processes may play a dominant role. One potential process that could explain differences across RCMs is the occurrence of precipitation feedbacks. We note however that precipitation changes are small in all RCMs with no clear consensus among models (Fig. S5). One possible exception is the summer precipitation decrease in WRFa-NoahMP, which could be related to the use of the Grell–Freitas convection scheme (Table 1), while precipitation is less affected in WRFb-NoahMP and WRFb-CLM4.0, which use the Kain–Fritsch scheme. The stronger summer temperature increase in WRFa-NoahMP compared to WRFb-NoahMP and WRFb-CLM4.0 may therefore be linked to this precipitation feedback.
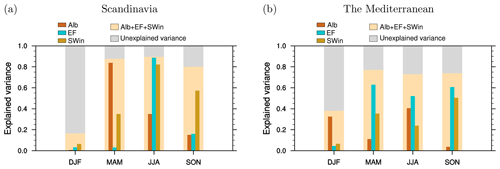
Figure 12Fraction of inter-model variance in 2 m temperature change (FOREST minus GRASS) explained by changes in albedo, evaporative fraction, incoming surface shortwave radiation or the three combined. Alb: inter-model correlation (Rsquared) between changes in albedo and 2 m temperature. EF: inter-model correlation (Rsquared) between changes in evaporative fraction and 2 m temperature. SWin: inter-model correlation (Rsquared) between changes in incoming surface shortwave radiation and 2 m temperature. Alb + EF + SWin: Rsquared of a multi-linear regression combining the three predictors. Results for other regions are shown in the Supplement.
Comparing results from different RCMs sharing either the same LSM or the same atmospheric model can help provide additional insights into the respective role of land versus atmospheric processes. By comparing for instance the temperature response across RCMs (Figs. 1 to 6), it appears, in summer particularly, that the three RCMs based on CCLM (i.e. same atmospheric model with three different LSMs) span almost the full range of RCM responses while CCLM-CLM4.5 and RegCM-CLM4.5 (i.e. same LSM and different atmospheric models) have generally similar patterns of change. This suggests that the summer temperature response to forestation is conditioned primarily by land process representation more than by atmospheric processes. To quantify objectively the level of similarity or dissimilarity between different RCMs, we compute the Euclidean distance across latitude and longitude between each RCM pairs for each season for differences in 2 m temperature and precipitation. This distance matrix is then used as a basis for a hierarchical clustering applying the Ward's clustering criterion (Ward, 1963). For the 2 m temperature response, the cluster analysis indicates a relatively high degree of similarity in winter between RCMs sharing the same atmospheric scheme, as illustrated in particular by the clustering of CCLM-TERRA and CCLM-CLM4.5 and of WRFb-NoahMP and WRFb-CLM4.0 (Fig. 13). In contrast, CCLM-TERRA and CCLM-CLM4.5 are relatively far apart in summer suggesting a stronger influence of land processes during this season. This tendency, however, does not arise in the WRF-based RCMs, with WRFb-NoahMP and WRFb-CLM4.0 showing a high degree of similarity even in summer. A possible explanation could be that NoahMP and CLM4.0 are structurally less different than TERRA and CLM4.5.
Results from nine RCMs show that, compared to grassland, forests imply warmer temperatures in winter and spring over northern Europe. This result is robust across RCMs and is a direct consequence of the lower albedo of forests, which is the dominating factor during these seasons. In summer and autumn, however, the RCMs disagree on the direction of changes, with responses ranging from a widespread cooling to a widespread warming above 2∘ in both cases. Although albedo change plays an important role in all seasons by increasing absorbed surface radiation, in summer inter-model differences in the temperature response are to a large extent induced by differences in EF. These conclusions are overall consistent with previous studies based on global climate models. Results from the LUCID and the CMIP5 model intercomparisons have indeed highlighted a robust, albedo-induced, winter cooling effect due to past deforestation at mid-latitudes (Lejeune et al., 2017), in other words implying a winter warming effect of forestation. On the other hand, no robust summer response has been identified in these intercomparisons, mainly attributed to a lack of agreement across models concerning evapotranspiration changes (Lejeune et al., 2017, 2018; de Noblet-Ducoudré et al., 2012).
Resolving this lack of consensus will require intensified efforts to confront models and observations and identify possible model deficiencies (Boisier et al., 2013, 2014; Duveiller et al., 2018a; Meier et al., 2018). For instance, a key feature emerging from observation-based studies is the fact that mid-latitude forests are colder during the day and warmer during the night compared to grassland (Duveiller et al., 2018b; Lee et al., 2011; Li et al., 2015). It is striking that none of the LUCID and CMIP5 models reflect this diurnal behaviour (Lejeune et al., 2017), nor do the RCMs analysed in this study (i.e. a majority of RCMs have a diurnal signal opposite to observations, two other RCMs indicate a cooling effect of forests for both day and night, and one exhibits a warming effect for both day and night). It is however important to note that this apparent contradiction may not be only attributable to model deficiencies and could be in part related to discrepancies on the scale of processes considered in models and observations. Indeed, observation-based estimates capture mainly local changes in surface energy balance and temperature due to land cover and are unlikely to reflect the type of large-scale atmospheric feedbacks triggered in coupled climate models (especially given the large-scale nature of the forest expansion considered in our experiments). Similarly, the fact that a majority of RCMs simulate a summer decrease in evapotranspiration over many regions following forestation is at odds with current observational evidence (Chen et al., 2018; Duveiller et al., 2018b; Meier et al., 2018) and might play a role in the simulated summer daytime warming in most RCMs. Although the reasons behind this behaviour may be model-specific, some recent work based on the CLM4.5 model, which is used in two of the RCMs here, sheds some light on the possible processes involved (Meier et al., 2018). It was found that while evapotranspiration is higher in spring under forested conditions in CLM4.5, trees become more water stressed than grassland in summer (even under equivalent soil moisture conditions) in particular due to unrealistic choices of root distribution, photosynthetic parameters and water uptake formulation. After improvement of these aspects in CLM4.5, evapotranspiration was found to be more realistically simulated, also resulting in an improved daytime temperature difference between grassland and forest (Meier et al., 2018). An important insight from this first phase of RCM experiments is therefore that particular attention should be given to model evaluation and benchmarking in future phases of the LUCAS initiative.
An additional insight from this study concerns the role of land versus atmospheric processes. Some of the participating RCMs share the same atmospheric scheme (i.e. the same version and configuration) but are coupled to different land surface models or share the same land surface model in combination with different atmospheric schemes. This represents a unique opportunity to objectively determine the origin of uncertainties in the simulated response. For instance, we find that land process representation is heavily involved in the large model spread in summer temperature response. The range of responses generated by using three different LSMs within the same atmospheric scheme (CCLM) is almost as large as the full model range in summer. Supporting this conclusion, a simple regression-based analysis shows that, except in winter, changes in albedo and EF can explain most of the inter-model spread in temperature sensitivity, in other words indicating that land processes primarily determine the simulated temperature response. Atmospheric processes can nevertheless also play a substantial or even dominant role for example in winter or for other variables such as precipitation.
In this first phase of LUCAS, we relied on idealized experiments at relatively low resolution (50 km) to gain insights into the biogeophysical role of forests across a range of European climates. Future phases of LUCAS will evolve toward increasing realism for instance by (1) investigating transient historical LUC forcing as well as RCP (representative concentration pathways)-based LUC scenarios, (2) considering a range of land use transitions beyond grassland to forest conversion and (3) assessing the added-value of higher (kilometre-scale) resolution when assessing local LUC impacts. Finally, the most societally relevant adverse effects or benefits from land management strategies may become apparent only when addressing changes in extreme events such as heatwaves or droughts (Davin et al., 2014; Lejeune et al., 2018), an aspect which will receive more attention in future analyses based on LUCAS simulations.
The data and scripts used are available upon request from the corresponding author.
The supplement related to this article is available online at: https://doi.org/10.5194/esd-11-183-2020-supplement.
ELD, DR, MB, RMC, EC, PH, LLJ, EK, KR, MR, PMMS, GS, SS, GS, MHT and KWS performed the RCM simulations, using vegetation maps produced by ELD. ELD designed the research, analysed the data and wrote the paper. All authors contributed to interpreting the results and revising the text.
The authors declare that they have no conflict of interest.
Edouard L. Davin acknowledges support from the Swiss National Science Foundation (SNSF) through the CLIMPULSE project and thanks the Swiss National Supercomputing Centre (CSCS) for providing computing resources. Rita M. Cardoso and Pedro M. M. Soares acknowledge the projects LEADING (PTDC/CTA-MET/28914/2017) and FCT- UID/GEO/50019/2019 – Instituto Dom Luiz. Peter Hoffmann is funded by the Climate Service Center Germany (GERICS) of the Helmholtz-Zentrum Geesthacht in the frame of the HICSS (Helmholtz-Institut Climate Service Science) project LANDMATE. Lisa L. Jach, Kirsten Warrach-Sagi and Volker Wulfmeyer acknowledge support by the state of Baden-Württemberg through bwHPC and thank the Anton and Petra Ehrmann-Stiftung Research Training Group “Water-People-Agriculture” for financial support. The work of Eleni Katragkou and Giannis Sofiadis was supported by computational time granted from the Greek Research & Technology Network (GRNET) in the National HPC facility – ARIS – under project ID pr005025_thin. Nathalie de Noblet-Ducoudré thanks the “Investments d'Avenir” Programme overseen by the French National Research Agency (ANR) (LabEx BASC; ANR-11-LABX-0034). RCA simulations were performed on the Swedish climate computing resource Bi provided by the Swedish National Infrastructure for Computing (SNIC) at the Swedish National Supercomputing Centre (NSC) at Linköping University. G. Strandberg was partly funded by a research project financed by the Swedish Research Council VR (Vetenskapsrådet) on “Quantification of the biogeophysical and biogeochemical forcings from anthropogenic deforestation on regional Holocene climate in Europe, LandClim II”. Susanna Strada has been supported by the TALENTS3 Fellowship Programme (FP code 1718349004) funded by the autonomous region Friuli Venezia Giulia via the European Social Fund (Operative Regional Programme 2014–2020) and administered by the AREA Science Park (Padriciano, Italy). CCLM-TERRA simulations were performed at the German Climate Computing Center (DKRZ) through support from the Federal Ministry of Education and Research in Germany (BMBF). Merja H. Tölle acknowledges the funding of the German Research Foundation (DFG) through grant 401857120. We thank Richard Wartenburger for providing the R scripts that have been used to perform the cluster analysis. We acknowledge the support of LUCAS by WCRP-CORDEX as a Flagship Pilot Study.
This research has been supported by the Swiss National Science Foundation (grant no. 200021_172715).
This paper was edited by Somnath Baidya Roy and reviewed by two anonymous referees.
Bechtold, P., Bazile, E., Guichard, F., Mascart, P., and Richard, E.: A mass-flux convection scheme for regional and global models, Q. J. Roy. Meteor. Soc., 127, 869–886, https://doi.org/10.1002/qj.49712757309, 2001.
Boisier, J. P., de Noblet-Ducoudré, N., and Ciais, P.: Inferring past land use-induced changes in surface albedo from satellite observations: a useful tool to evaluate model simulations, Biogeosciences, 10, 1501–1516, https://doi.org/10.5194/bg-10-1501-2013, 2013.
Boisier, J. P., de Noblet-Ducoudré, N., and Ciais, P.: Historical land-use-induced evapotranspiration changes estimated from present-day observations and reconstructed land-cover maps, Hydrol. Earth Syst. Sci., 18, 3571–3590, https://doi.org/10.5194/hess-18-3571-2014, 2014.
Bonan, G. B.: Forests and climate change: Forcings, feedbacks, and the climate benefits of forests, Science, 320, 1444–1449, https://doi.org/10.1126/science.1155121, 2008.
Breil, M., Schädler, G. and Laube, N.: An Improved Soil Moisture Parametrization for Regional Climate Simulations in Europe, J. Geophys. Res.-Atmos., 123, 7331–7339, https://doi.org/10.1029/2018JD028704, 2018.
Bretherton, C. S., McCaa, J. R., Grenier, H., Bretherton, C. S., McCaa, J. R. and Grenier, H.: A New Parameterization for Shallow Cumulus Convection and Its Application to Marine Subtropical Cloud-Topped Boundary Layers. Part I: Description and 1D Results, Mon. Weather Rev., 132, 864–882, https://doi.org/10.1175/1520-0493(2004)132<0864:ANPFSC>2.0.CO;2, 2004.
Chen, L., Dirmeyer, P. A., Guo, Z., and Schultz, N. M.: Pairing FLUXNET sites to validate model representations of land-use/land-cover change, Hydrol. Earth Syst. Sci., 22, 111–125, https://doi.org/10.5194/hess-22-111-2018, 2018.
Cherubini, F., Huang, B., Hu, X., Tölle, M. H., and Strømman, A. H.: Quantifying the climate response to extreme land cover changes in Europe with a regional model, Environ. Res. Lett., 13, 074002, https://doi.org/10.1088/1748-9326/aac794, 2018.
Christensen, J. H. and Christensen, O. B.: A summary of the PRUDENCE model projections of changes in European climate by the end of this century, Climatic Change, 81, 7–30, https://doi.org/10.1007/s10584-006-9210-7, 2007.
Christensen, J. H., Carter, T. R., Rummukainen, M., and Amanatidis, G.: Evaluating the performance and utility of regional climate models: the PRUDENCE project, Climatic Change, 81, 1–6, https://doi.org/10.1007/s10584-006-9211-6, 2007.
Claussen, M., Brovkin, V., and Ganopolski, A.: Biogeophysical versus biogeochemical feedbacks of large-scale land cover change, Geophys. Res. Lett., 28, 1011–1014, 2001.
Davies, H. C.: A lateral boundary formulation for multi-level prediction models, Q. J. Roy. Meteor. Soc., 102, 405–418, https://doi.org/10.1002/qj.49710243210, 1976.
Davin, E. L. and de Noblet-Ducoudré, N.: Climatic impact of global-scale Deforestation: Radiative versus nonradiative processes, J. Climate, 23, 97–112, https://doi.org/10.1175/2009JCLI3102.1, 2010.
Davin, E. L., Seneviratne, S. I., Ciais, P., Olioso, A., and Wang, T.: Preferential cooling of hot extremes from cropland albedo management, P. Natl. Acad. Sci. USA, 111, 9757–9761, https://doi.org/10.1073/pnas.1317323111, 2014.
Davin, E. L., Maisonnave, E., and Seneviratne, S. I.: Is land surface processes representation a possible weak link in current Regional Climate Models?, Environ. Res. Lett., 11, 074027, https://doi.org/10.1088/1748-9326/11/7/074027, 2016.
Dee, D. P., Uppala, S. M., Simmons, A. J., Berrisford, P., Poli, P., Kobayashi, S., Andrae, U., Balmaseda, M. A., Balsamo, G., Bauer, P., Bechtold, P., Beljaars, A. C. M., van de Berg, L., Bidlot, J., Bormann, N., Delsol, C., Dragani, R., Fuentes, M., Geer, A. J., Haimberger, L., Healy, S. B., Hersbach, H., Holm, E. V, Isaksen, L., Kallberg, P., Koehler, M., Matricardi, M., McNally, A. P., Monge-Sanz, B. M., Morcrette, J.-J., Park, B.-K., Peubey, C., de Rosnay, P., Tavolato, C., Thepaut, J.-N., and Vitart, F.: The ERA-Interim reanalysis: configuration and performance of the data assimilation system, Q. J. Roy. Meteor. Soc., 137, 553–597, https://doi.org/10.1002/qj.828, 2011.
de Noblet-Ducoudré, N., Boisier, J.-P., Pitman, A., Bonan, G. B., Brovkin, V., Cruz, F., Delire, C., Gayler, V., van den Hurk, B. J. J. M., Lawrence, P. J., van der Molen, M. K., Mueller, C., Reick, C. H., Strengers, B. J., and Voldoire, A.: Determining Robust Impacts of Land-Use-Induced Land Cover Changes on Surface Climate over North America and Eurasia: Results from the First Set of LUCID Experiments, J. Climate, 25, 3261–3281, https://doi.org/10.1175/JCLI-D-11-00338.1, 2012.
Duveiller, G., Forzieri, G., Robertson, E., Li, W., Georgievski, G., Lawrence, P., Wiltshire, A., Ciais, P., Pongratz, J., Sitch, S., Arneth, A., and Cescatti, A.: Biophysics and vegetation cover change: a process-based evaluation framework for confronting land surface models with satellite observations, Earth Syst. Sci. Data, 10, 1265–1279, https://doi.org/10.5194/essd-10-1265-2018, 2018a.
Duveiller, G., Hooker, J., and Cescatti, A.: The mark of vegetation change on Earth's surface energy balance, Nat. Commun., 9, 679, 3261–3281, https://doi.org/10.1038/s41467-017-02810-8, 2018.
Gálos, B., Hagemann, S., Hänsler, A., Kindermann, G., Rechid, D., Sieck, K., Teichmann, C., and Jacob, D.: Case study for the assessment of the biogeophysical effects of a potential afforestation in Europe, Carbon Balance Manag., 8, 3, https://doi.org/10.1186/1750-0680-8-3, 2013.
Giorgi, F., Bates, G. T., Giorgi, F., and Bates, G. T.: The Climatological Skill of a Regional Model over Complex Terrain, Mon. Weather Rev., 117, 2325–2347, https://doi.org/10.1175/1520-0493(1989)117<2325:TCSOAR>2.0.CO;2, 1989.
Giorgi, F., Marinucci, M. R., Bates, G. T., De Canio, G., Giorgi, F., Marinucci, M. R., Bates, G. T., and Canio, G. De: Development of a Second-Generation Regional Climate Model (RegCM2). Part II: Convective Processes and Assimilation of Lateral Boundary Conditions, Mon. Weather Rev., 121, 2814–2832, https://doi.org/10.1175/1520-0493(1993)121<2814:DOASGR>2.0.CO;2, 1993.
Giorgi, F., Coppola, E., Solmon, F., Mariotti, L., Sylla, M., Bi, X., Elguindi, N., Diro, G., Nair, V., Giuliani, G., Turuncoglu, U., Cozzini, S., Güttler, I., O'Brien, T., Tawfik, A., Shalaby, A., Zakey, A., Steiner, A., Stordal, F., Sloan, L., and Brankovic, C.: RegCM4: model description and preliminary tests over multiple CORDEX domains, Clim. Res., 52, 7–29, https://doi.org/10.3354/cr01018, 2012.
Grassi, G., House, J., Dentener, F., Federici, S., Den Elzen, M., and Penman, J.: The key role of forests in meeting climate targets requires science for credible mitigation, Nat. Clim. Change, 7, 220–228, https://doi.org/10.1038/nclimate3227, 2017.
Grell, G. A. and Freitas, S. R.: A scale and aerosol aware stochastic convective parameterization for weather and air quality modeling, Atmos. Chem. Phys., 14, 5233–5250, https://doi.org/10.5194/acp-14-5233-2014, 2014.
Grenier, H., Bretherton, C. S., Grenier, H., and Bretherton, C. S.: A Moist PBL Parameterization for Large-Scale Models and Its Application to Subtropical Cloud-Topped Marine Boundary Layers, Mon. Weather Rev., 129, 357–377, https://doi.org/10.1175/1520-0493(2001)129<0357:AMPPFL>2.0.CO;2, 2001.
Griscom, B. W., Adams, J., Ellis, P. W., Houghton, R. A., Lomax, G., Miteva, D. A., Schlesinger, W. H., Shoch, D., Siikamaki, J. V, Smith, P., Woodbury, P., Zganjar, C., Blackman, A., Campari, J., Conant, R. T., Delgado, C., Elias, P., Gopalakrishna, T., Hamsik, M. R., Herrero, M., Kiesecker, J., Landis, E., Laestadius, L., Leavitt, S. M., Minnemeyer, S., Polasky, S., Potapov, P., Putz, F. E., Sanderman, J., Silvius, M., Wollenberg, E., and Fargione, J.: Natural climate solutions, P. Natl. Acad. Sci. USA, 114, 11645–11650, https://doi.org/10.1073/pnas.1710465114, 2017.
Gutowski Jr., W. J., Giorgi, F., Timbal, B., Frigon, A., Jacob, D., Kang, H.-S., Raghavan, K., Lee, B., Lennard, C., Nikulin, G., O'Rourke, E., Rixen, M., Solman, S., Stephenson, T., and Tangang, F.: WCRP COordinated Regional Downscaling EXperiment (CORDEX): a diagnostic MIP for CMIP6, Geosci. Model Dev., 9, 4087–4095, https://doi.org/10.5194/gmd-9-4087-2016, 2016.
Harper, A. B., Powell, T., Cox, P. M., House, J., Huntingford, C., Lenton, T. M., Sitch, S., Burke, E., Chadburn, S. E., Collins, W. J., Comyn-Platt, E., Daioglou, V., Doelman, J. C., Hayman, G., Robertson, E., van Vuuren, D., Wiltshire, A., Webber, C. P., Bastos, A., Boysen, L., Ciais, P., Devaraju, N., Jain, A. K., Krause, A., Poulter, B., and Shu, S.: Land-use emissions play a critical role in land-based mitigation for Paris climate targets, Nat. Commun., 9, 2938, https://doi.org/10.1038/s41467-018-05340-z, 2018.
Hong, S.-Y., Koo, M.-S., Jang, J., Esther Kim, J.-E., Park, H., Joh, M.-S., Kang, J.-H., Oh, T.-J., Hong, S.-Y., Koo, M.-S., Jang, J., Kim, J.-E. E., Park, H., Joh, M.-S., Kang, J.-H., and Oh, T.-J.: An Evaluation of the Software System Dependency of a Global Atmospheric Model, Mon. Weather Rev., 141, 4165–4172, https://doi.org/10.1175/MWR-D-12-00352.1, 2013.
Iacono, M. J., Delamere, J. S., Mlawer, E. J., Shephard, M. W., Clough, S. A., and Collins, W. D.: Radiative forcing by long-lived greenhouse gases: Calculations with the AER radiative transfer models, J. Geophys. Res., 113, D13103, https://doi.org/10.1029/2008JD009944, 2008.
Jacob, D., Petersen, J., Eggert, B., Alias, A., Christensen, O. B., Bouwer, L. M., Braun, A., Colette, A., Déqué, M., Georgievski, G., Georgopoulou, E., Gobiet, A., Menut, L., Nikulin, G., Haensler, A., Hempelmann, N., Jones, C., Keuler, K., Kovats, S., Kröner, N., Kotlarski, S., Kriegsmann, A., Martin, E., van Meijgaard, E., Moseley, C., Pfeifer, S., Preuschmann, S., Radermacher, C., Radtke, K., Rechid, D., Rounsevell, M., Samuelsson, P., Somot, S., Soussana, J.-F., Teichmann, C., Valentini, R., Vautard, R., Weber, B., and Yiou, P.: EURO-CORDEX: new high-resolution climate change projections for European impact research, Reg. Environ. Change, 14, 563–578, https://doi.org/10.1007/s10113-013-0499-2, 2014.
Kain, J. S.: The Kain–Fritsch Convective Parameterization: An Update, J. Appl. Meteorol., 43, 170–181, https://doi.org/10.1175/1520-0450(2004)043<0170:TKCPAU>2.0.CO;2, 2004.
Kiehl, J. T., Hack, J. J., Bonan, G. B., Boville, B. A., Breigleb, B. P., Williamson, D., and Rasch, P.: Description of the NCAR Community Climate Model (CCM3), Tech. Rep. NCAR/TN-420+STR, National Center for Atmospheric Research, 1996.
Kotlarski, S., Keuler, K., Christensen, O. B., Colette, A., Déqué, M., Gobiet, A., Goergen, K., Jacob, D., Lüthi, D., van Meijgaard, E., Nikulin, G., Schär, C., Teichmann, C., Vautard, R., Warrach-Sagi, K., and Wulfmeyer, V.: Regional climate modeling on European scales: a joint standard evaluation of the EURO-CORDEX RCM ensemble, Geosci. Model Dev., 7, 1297–1333, https://doi.org/10.5194/gmd-7-1297-2014, 2014.
Kumar, S., Dirmeyer, P. A., Merwade, V., DelSole, T., Adams, J. M., and Niyogi, D.: Land use/cover change impacts in CMIP5 climate simulations: A new methodology and 21st century challenges, J. Geophys. Res.-Atmos., 118, 6337–6353, https://doi.org/10.1002/jgrd.50463, 2013.
Lawrence, P. J. and Chase, T. N.: Representing a new MODIS consistent land surface in the Community Land Model (CLM 3.0), J. Geophys. Res., 112, G01023, https://doi.org/10.1029/2006JG000168, 2007.
Lee, X., Goulden, M. L., Hollinger, D. Y., Barr, A., Black, T. A., Bohrer, G., Bracho, R., Drake, B., Goldstein, A., Gu, L., Katul, G., Kolb, T., Law, B. E., Margolis, H., Meyers, T., Monson, R., Munger, W., Oren, R., U, K. T. P., Richardson, A. D., Schmid, H. P., Staebler, R., Wofsy, S., and Zhao, L.: Observed increase in local cooling effect of deforestation at higher latitudes, Nature, 479, 384–387, https://doi.org/10.1038/nature10588, 2011.
Lejeune, Q., Davin, E. L., Guillod, B. P., and Seneviratne, S. I.: Influence of Amazonian deforestation on the future evolution of regional surface fluxes, circulation, surface temperature and precipitation, Clim. Dynam., 44, 2769–2786, https://doi.org/10.1007/s00382-014-2203-8, 2015.
Lejeune, Q., Seneviratne, S. I., and Davin, E. L.: Historical land-cover change impacts on climate: Comparative assessment of LUCID and CMIP5 multimodel experiments, J. Climate, 30, 386–390, https://doi.org/10.1175/JCLI-D-16-0213.1, 2017.
Lejeune, Q., Davin, E. L., Gudmundsson, L., Winckler, J., and Seneviratne, S. I.: Historical deforestation locally increased the intensity of hot days in northern mid-latitudes, Nat. Clim. Change, 8, 386–390, https://doi.org/10.1038/s41558-018-0131-z, 2018.
Li, Y., Zhao, M., Motesharrei, S., Mu, Q., Kalnay, E., and Li, S.: Local cooling and warming effects of forests based on satellite observations, Nat. Commun., 6, 6603, https://doi.org/10.1038/ncomms7603, 2015.
Lim, K.-S. S. and Hong, S.-Y.: Development of an Effective Double-Moment Cloud Microphysics Scheme with Prognostic Cloud Condensation Nuclei (CCN) for Weather and Climate Models, Mon. Weather Rev., 138, 1587–1612, https://doi.org/10.1175/2009MWR2968.1, 2010.
Louis, J.-F.: A parametric model of vertical eddy fluxes in the atmosphere, Bound.-Lay. Meteorol., 17, 187–202, https://doi.org/10.1007/BF00117978, 1979.
Mearns, L. O., Arritt, R., Biner, S., Bukovsky, M. S., McGinnis, S., Sain, S., Caya, D., Correia, J., Flory, D., Gutowski, W., Takle, E. S., Jones, R., Leung, R., Moufouma-Okia, W., McDaniel, L., Nunes, A. M. B., Qian, Y., Roads, J., Sloan, L., Snyder, M., Mearns, L. O., Arritt, R., Biner, S., Bukovsky, M. S., McGinnis, S., Sain, S., Caya, D., Jr., J. C., Flory, D., Gutowski, W., Takle, E. S., Jones, R., Leung, R., Moufouma-Okia, W., McDaniel, L., Nunes, A. M. B., Qian, Y., Roads, J., Sloan, L., and Snyder, M.: The North American Regional Climate Change Assessment Program: Overview of Phase I Results, B. Am. Meteorol. Soc., 93, 1337–1362, https://doi.org/10.1175/BAMS-D-11-00223.1, 2012.
Meier, R., Davin, E. L., Lejeune, Q., Hauser, M., Li, Y., Martens, B., Schultz, N. M., Sterling, S., and Thiery, W.: Evaluating and improving the Community Land Model's sensitivity to land cover, Biogeosciences, 15, 4731–4757, https://doi.org/10.5194/bg-15-4731-2018, 2018.
Meinshausen, M., Smith, S. J., Calvin, K., Daniel, J. S., Kainuma, M. L. T., Lamarque, J.-F., Matsumoto, K., Montzka, S. A., Raper, S. C. B., Riahi, K., Thomson, A., Velders, G. J. M., and van Vuuren, D. P. P.: The RCP greenhouse gas concentrations and their extensions from 1765 to 2300, Climatic Change, 109, 213–241, https://doi.org/10.1007/s10584-011-0156-z, 2011.
Mellor, G. L. and Yamada, T.: Hierarchy of turbulence closure models for planetary boundary-layers, J. Atmos. Sci., 31, 1791–1806, 1974.
Mellor, G. L. and Yamada, T.: Development of a turbulence closure-model for geophysical fluid problems, Rev. Geophys., 20, 851–875, 1982.
Morcrette, J. J., Smith, L. and Fouquart, Y.: Pressure and temperature dependence of the absorption in longwave radiation parametrizations, Contrib. Atmos. Phys., 59, 455–469, 1986.
Nakanishi, M. and Niino, H.: An Improved Mellor–Yamada Level-3 Model: Its Numerical Stability and Application to a Regional Prediction of Advection Fog, Bound.-Lay. Meteorol., 119, 397–407, https://doi.org/10.1007/s10546-005-9030-8, 2006.
Nakanishi, M. and Niino, H.: Development of an Improved Turbulence Closure Model for the Atmospheric Boundary Layer, J. Meteorol. Soc. Jpn., 87, 895–912, https://doi.org/10.2151/jmsj.87.895, 2009.
Nordeng, T. E.: Extended versions of the convective parametrization scheme at ECMWF and their impact on the mean and transient activity of the model in the tropics. ECMWF Research Department, Technical Momorandum No. 206, October 1994, European Centre for Medium Range Weather Forecasts, Reading, UK, 41 pp., 1994.
Oleson, K. W., Lawrence, D. M., Gordon, B., Flanner, M. G., Kluzek, E., Peter, L. J., Levis, S., Swenson, S. C., and Thornton, P. E.: Technical description of version 4.0 of the Community Land Model (CLM) NCAR Technical Note NCAR/TN-478+STR, National Center for Atmospheric Research, Boulder, CO, p. 257, https://doi.org/10.5065/D6FB50WZ, 2010.
Oleson, K. W., Lawrence, D. M., Bonan, G. B., Drewniak, B., Huang, M., Koven, C. D., Levis, S., Li, F., Riley, W. J., Subin, Z. M., Swenson, S. C., Thornton, P. E., Bozbiyik, A., Fisher, R., Kluzek, E., Lamarque, J.-F., Lawrence, P. J., Leung, L. R., Lipscomb, W., Muszala, S., Ricciuto, D. M., Sacks, W., Sun, Y., Tang, J., and Yang, Z.-L.: Technical description of version 4.5 of the Community Land Model (CLM), Boulder, CO, 2013.
Pal, J. S., Small, E. E., and Eltahir, E. A. B.: Simulation of regional-scale water and energy budgets: Representation of subgrid cloud and precipitation processes within RegCM, J. Geophys. Res.-Atmos., 105, 29579–29594, https://doi.org/10.1029/2000JD900415, 2000.
Rechid, D., Davin, E., de Noblet-Ducoudré, N., and Katragkou, E.: CORDEX Flagship Pilot Study LUCAS – Land Use & Climate Across Scales – a new initiative on coordinated regional land use change and climate experiments for Europe, in 19th EGU General Assembly, EGU2017, proceedings from the conference held 23–28 April, 2017 in Vienna, Austria, 19, p. 13172, 2017.
Ritter, B., Geleyn, J.-F., Ritter, B., and Geleyn, J.-F.: A Comprehensive Radiation Scheme for Numerical Weather Prediction Models with Potential Applications in Climate Simulations, Mon. Weather Rev., 120, 303–325, https://doi.org/10.1175/1520-0493(1992)120<0303:ACRSFN>2.0.CO;2, 1992.
Roeckner, E., Arpe, K., Bentsson, L., Christoph, M., Claussen, M., Dümenil, L., Esch, M., Giorgetta, M., Schlese, U., and Schulzweida, U.: The atmospheric genaral circulation model ECHAM-4: Model description and simulation of present day climate. Max-Planck Institut für Meteorologie Report No. 218, 90 pp., 1996.
Samuelsson, P., Gollvik, S., and Ullerstig, A.: The land-surface scheme of the Rossby Centre regional atmospheric model (RCA3), Reports Meteorology, 122, SMHI, SE-60176 Norrköping, Sweden, 2006.
Savijärvi, H. and Savijärvi, H.: Fast Radiation Parameterization Schemes for Mesoscale and Short-Range Forecast Models, J. Appl. Meteorol., 29, 437–447, https://doi.org/10.1175/1520-0450(1990)029<0437:FRPSFM>2.0.CO;2, 1990.
Schrodin, R. and Heise, E.: The multi-layer version of the DWD soil model TERRA-LM, COSMO Tech. Rep. 2, 2001, Deutscher Wetterdienst, available at: http://www.cosmo-model.org/content/model/documentation/techReports/docs/techReport02.pdf (last access: 10 February 2020), 2001.
Seifert, A. and Beheng, K. D.: A double-moment parameterization for simulating autoconversion, accretion and selfcollection, Atmos. Res., 59–60, 265–281, https://doi.org/10.1016/S0169-8095(01)00126-0, 2001.
Solman, S. A., Sanchez, E., Samuelsson, P., da Rocha, R. P., Li, L., Marengo, J., Pessacg, N. L., Remedio, A. R. C., Chou, S. C., Berbery, H., Le Treut, H., de Castro, M., and Jacob, D.: Evaluation of an ensemble of regional climate model simulations over South America driven by the ERA-Interim reanalysis: model performance and uncertainties, Clim. Dynam., 41, 1139–1157, https://doi.org/10.1007/s00382-013-1667-2, 2013.
Strandberg, G., Kjellström, E., Strandberg, G., and Kjellström, E.: Climate impacts from afforestation and deforestation in Europe, Earth Interact, 23, 1–27, https://doi.org/10.1175/EI-D-17-0033.1, 2018.
Sundqvist, H.: A parameterization scheme for non-convective condensation including prediction of cloud water content, Q. J. Roy. Meteor. Soc., 104, 677–690, https://doi.org/10.1002/qj.49710444110, 1978.
Tanré, D., Geleyn, J., and Slingo, J.: First results of the introduction of an advanced aerosol‐radiation interaction in ECMWF low resolution global model, in: Aerosols and Their Climatic Effects, edited by: Gerber, H. and Deepak, A., 133–177, A. Deepak, Hampton, Va, 1984.
Tegen, I., Hollrig, P., Chin, M., Fung, I., Jacob, D., and Penner, J.: Contribution of different aerosol species to the global aerosol extinction optical thickness: Estimates from model results, J. Geophys. Res.-Atmos., 102, 23895–23915, https://doi.org/10.1029/97JD01864, 1997.
Teichmann, C., Eggert, B., Elizalde, A., Haensler, A., Jacob, D., Kumar, P., Moseley, C., Pfeifer, S., Rechid, D., Remedio, A., Ries, H., Petersen, J., Preuschmann, S., Raub, T., Saeed, F., Sieck, K., Weber, T., Teichmann, C., Eggert, B., Elizalde, A., Haensler, A., Jacob, D., Kumar, P., Moseley, C., Pfeifer, S., Rechid, D., Remedio, A. R., Ries, H., Petersen, J., Preuschmann, S., Raub, T., Saeed, F., Sieck, K., and Weber, T.: How Does a Regional Climate Model Modify the Projected Climate Change Signal of the Driving GCM: A Study over Different CORDEX Regions Using REMO, Atmosphere-Basel, 4, 214–236, https://doi.org/10.3390/atmos4020214, 2013.
Thompson, G., Rasmussen, R. M., and Manning, K.: Explicit Forecasts of Winter Precipitation Using an Improved Bulk Microphysics Scheme. Part I: Description and Sensitivity Analysis, Mon. Weather Rev., 132, 519–542, https://doi.org/10.1175/1520-0493(2004)132<0519:EFOWPU>2.0.CO;2, 2004.
Tiedtke, M.: A Comprehensive Mass Flux Scheme for Cumulus Parameterization in Large-Scale Models, Mon. Weather Rev., 117, 1779–1800, 1989.
Tiedtke, M.: An Extension of Cloud-Radiation Parameterization in the ECMWF Model: The Representation of Subgrid-Scale Variations of Optical Depth, Mon. Weather Rev., 124, 745–750, https://doi.org/10.1175/1520-0493(1996)124<0745:AEOCRP>2.0.CO;2, 1996.
Tölle, M. H., Breil, M., Radtke, K., and Panitz, H.-J.: Sensitivity of European Temperature to Albedo Parameterization in the Regional Climate Model COSMO-CLM Linked to Extreme Land Use Changes, Front. Environ. Sci., 6, 123, https://doi.org/10.3389/fenvs.2018.00123, 2018.
Vogelezang, D. H. P. and Holtslag, A. A. M.: Evaluation and model impacts of alternative boundary-layer height formulations, Bound.-Lay. Meteorol., 81, 245–269, https://doi.org/10.1007/BF02430331, 1996.
Ward, J. H.: Hierarchical Grouping to Optimize an Objective Function, J. Am. Stat. Assoc., 58, 236–244, https://doi.org/10.1080/01621459.1963.10500845, 1963.
Wilhelm, C., Rechid, D., and Jacob, D.: Interactive coupling of regional atmosphere with biosphere in the new generation regional climate system model REMO-iMOVE, Geosci. Model Dev., 7, 1093–1114, https://doi.org/10.5194/gmd-7-1093-2014, 2014.
Wulfmeyer, V., Branch, O., Warrach-Sagi, K., Bauer, H.-S., Schwitalla, T., Becker, K., Wulfmeyer, V., Branch, O., Warrach-Sagi, K., Bauer, H.-S., Schwitalla, T., and Becker, K.: The Impact of Plantations on Weather and Climate in Coastal Desert Regions, J. Appl. Meteorol. Clim., 53, 1143–1169, https://doi.org/10.1175/JAMC-D-13-0208.1, 2014.
Wyser, K., Rontu, L., and Savijärvi, H.: Introducing the Effective Radius into a Fast Radiation Scheme of a Mesoscale Model, Contr. Atmos. Phys., 72, 205–218, 1999.