the Creative Commons Attribution 4.0 License.
the Creative Commons Attribution 4.0 License.
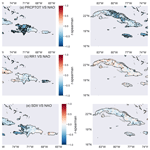
Changes in extreme precipitation patterns over the Greater Antilles and teleconnection with large-scale sea surface temperature
Carlo Destouches
Arona Diedhiou
Sandrine Anquetin
Benoit Hingray
Armand Pierre
Dominique Boisson
Adermus Joseph
This study examines changes in extreme precipitation over the Greater Antilles and their correlation with large-scale sea surface temperature (SST) for the period 1985 to 2015. The data used for this study were derived from two satellite products, Climate Hazards Group Infrared Precipitation with Stations (CHIRPS) and NOAA Daily Optimum Interpolation Sea Surface Temperature (DOISST) version 2.1, with resolutions of 5 and 25 km, respectively. Then, changes in the characteristics of six extreme precipitation indices defined by the World Meteorological Organization's Expert Team on Climate Change Detection and Indices (ETCCDI) are analyzed, and Spearman's correlation coefficient is used and evaluated by t test to investigate the influence of a few large-scale SST indices: (i) Caribbean Sea Surface Temperature (SST-CAR), (ii) Tropical South Atlantic (TSA), (iii) Southern Oscillation Index (SOI), and (iv) North Atlantic Oscillation (NAO). The results show that, at the regional scale, +NAO contributes significantly to a decrease in heavy precipitation (R95p), daily precipitation intensity (SDII), and total precipitation (PRCPTOT), whereas +TSA is associated with a significant increase in daily precipitation intensity (SDII). On an island scale, in Puerto Rico and southern Cuba, the positive phases of +TSA, +SOI, and +SST-CAR are associated with an increase in daily precipitation intensity (SDII) and heavy precipitation (R95p). However, in Jamaica and northern Haiti, the positive phases of +SST-CAR and +TSA are also associated with increased indices (SDII, R95p). In addition, the SST warming of the Caribbean Sea and the positive phase of the Southern Oscillation (+SOI) are associated with a significant increase in the number of rainy days (RR1) and the maximum duration of consecutive wet days (CWD) over the Dominican Republic and in southern Haiti.
- Article
(5394 KB) - Full-text XML
-
Supplement
(5215 KB) - BibTeX
- EndNote
Over the past 3 decades, the climatic hazards to which the Caribbean Basin has been exposed include recurrent cyclonic and hydrometeorological hazards characterized by increasing intensities (Joseph, 2006). The economic cost of 250 storms and floods over 40 years (1970 to 2009) for 12 Caribbean countries amounted to USD 19.7 billion in 2010, representing an annual average of 1 % of gross domestic product (GDP) (Burgess et al., 2018). The worst damage caused by these hydroclimatic events includes George in 1998, with 1000 victims in the Dominican Republic and losses estimated at 14 % of GDP, equivalent to approximately half the exports made that year (Naciones Unidades, Comision Economica para America Latina y el Caribe, 1988), and Matthew in Haiti (October 2016), with over 500 dead, 128 missing, 439 injured, and 2.1 million people affected, including 895 000 children (De Giorgi et al., 2021). Also, Hurricane Dorian caused property damage estimated at USD 2.5 billion when it came to rest over the Bahamas as a Category 5 storm in September 2019, rendering nearly 3000 homes uninhabitable and causing extensive damage to hospitals, schools, and fisheries (Deopersad et al., 2020). A severe drought episode affected the islands of the Caribbean from October 2019 to mid-2020, causing water shortages, bushfires, and agricultural losses. In Saint Vincent and the Grenadines, the 2020 drought was considered the worst in 50 years (Nurse, 2020). The Inter-American Development Bank predicts that the Caribbean could face climate-related losses of over USD 22 billion per year by 2050 (Marto et al., 2014).
In response to the climate extremes that are further weakening the island states of the Caribbean region, already in a situation of extreme socio-economic precariousness, several studies have been carried out in parallel to understand the associated physical processes and anticipate the evolution of these extreme climatic events. Research into the Caribbean climate goes back to the second half of the 20th century and has focused mainly on rainfall patterns (Curtis and Gamble, 2008) and the overall description of rainy seasons (Griffiths and Driscoll, 1982).
A more detailed study of the climate of the Caribbean was performed in 2001 and 2002 using indices derived from daily data to detect climate change (Peterson et al., 2001; Frich et al., 2002). This approach, which uses indices defined by the World Meteorological Organization's group of experts to characterize precipitation and temperature extremes, has enabled several studies to examine the state of climate extremes over the Caribbean (Stephenson et al., 2014; McLean et al., 2015). The results of these previous assessments agree that the frequency and intensity of climate extremes (heavy rainfall, drought spell, wet spell) over the Caribbean have increased over the last 30 years (Stephenson et al., 2014; Peterson et al., 2002; Beharry et al., 2015; Dookie et al., 2019) and will continue to do so until the end of the century (Taylor et al., 2018; Vichot‐Llano et al., 2021; Hall et al., 2013; Almazroui et al., 2021; McLean et al., 2015).
Climate teleconnections, the remote forcing of a region far from the source of disturbance, whether simultaneous or time-lagged (Mariani et al., 2018; Rodrigues et al., 2021), are generally derived from variations in sea surface temperature (SST) or atmospheric pressure at seasonal to interdecadal scales. Several of these have been shown to play a major role in modifying global weather patterns (Hurrell, 1995; Martens et al., 2018).
Previous studies have also shown the effect of east–west gradients in SST anomalies in the tropical Pacific and Atlantic on precipitation in the Caribbean, with a tendency for a warm Atlantic and a cold Pacific to favor precipitation in the Caribbean (Taylor and Enfield, 2002; Gimeno et al., 2011). Studies (Enfield et al., 2001; IPCC, 2007) also found that the monthly AMO (Atlantic Multidecadal Oscillation) index is an SST signal in the North Atlantic that influences the decadal-scale variability in precipitation. In addition, Peterson et al. (2002) analyzed the link between SST, temperature, and precipitation extremes over the Caribbean using ground-based observations. They showed that the extreme precipitation index averaged over the Caribbean has a strong correlation with SST over the Caribbean and the entire tropical North Atlantic Ocean. The work of Stephenson et al. (2014) examined the influence of the Atlantic Multidecadal Oscillation (AMO) on extreme precipitation from a ground-based observation network in the Caribbean. These results show that the AMO influences the variability in extreme temperature and precipitation events. However, considering that the effects of teleconnections caused by large-scale SSTs on weather are expected to become more extreme in the future due to climate change (Mariani et al., 2018), further research is needed on other SST indices such as NAO, SOI, TSA, SST-CAR, for which no in-depth studies have been carried out.
In this context, this study aimed to examine the remote impact of the tropical Pacific Ocean, the Atlantic Ocean, and the Caribbean Sea on observed changes in the tropical islands of the Greater Antilles, particularly the links between extreme precipitation indices and large-scale sea surface temperature indices. This paper is organized into five sections: Section 1 presents the study area and the associated climatology. Sections 2 and 3 describe the spatiotemporal variability in extreme precipitation indices at regional and local scales and the influence of SST indices on extreme precipitation. The last sections (Sects. 4–5) present the discussion and conclusion.
2.1 Study area
The Greater Antilles is a region between North and South America made up of islands bordered by the Caribbean Sea to the south and the Atlantic Ocean to the east (Fig. 1). These islands include Cuba, Hispaniola, Jamaica, and Puerto Rico. They have a monthly rainfall cycle characterized by two peaks: the first in May and the second between September and October (Giannini et al., 2000). The climatology of monthly rainfall in the Greater Antilles is strongly influenced by the subtropical North Atlantic anticyclone (Davis et al., 1997), the Caribbean Low-Level Jet (CLLJ), characterized by two peaks: the first in January and the second in July (Cook and Vizy, 2010). This jet plays a key role in transporting moisture to the Caribbean (Mo et al., 2005). The rainfall is also influenced by the Intertropical Convergence Zone (ITCZ) (Hastenrath, 2002), with maximum precipitation in May (Fig. S1b). Heavy autumn rainfall in the Greater Antilles (Fig. S1d) is generally associated with North Atlantic tropical cyclones, 85 % of which are of high intensity and originate from African easterlies (Agudelo et al., 2011; Thorncroft and Hodges, 2001) under warm Atlantic basin conditions. The spatial distribution of the total annual precipitation in the Greater Antilles, particularly on the islands, is not homogeneous due to the complexity of topography (Moron et al., 2015; Cantet, 2017). Precipitation is relatively high (2000–24 000 mm yr−1) at higher altitudes and in wind-exposed areas (Fig. S1b). In contrast, annual precipitation only reaches 500 mm yr−1 in leeward areas (Daly et al., 2003).
2.2 Satellite data
This study was conducted using two satellite datasets: NOAA Daily Optimum Interpolation Sea Surface Temperature (DOISST) and Climate Hazards Group Infrared Precipitation with Stations version 2 (CHIRPSv2). The CHIRPSv2 data are quasi-global daily precipitation data (50° S–50° N) with a resolution of 0.05, available over the period of 1981 to 2022 (Funk et al., 2015a). Based on the techniques used by NOAA for estimating precipitation in the thermal infrared (Love et al., 2004), the CHIRPSv2 database was built from precipitation estimates based on cold cloud duration observations and a fusion incorporating monthly Climate Hazards Group Precipitation Climatology (CHPclim) (Funk et al., 2015b) precipitation data and in situ data from ground observation networks. Tropical Rainfall Measuring Mission Multi-Satellite Precipitation Analysis (TRMM 3B42v7) satellite products were also used to calibrate and reduce the bias in the estimates. The results of global and regional validation studies showed that CHIRPSv2 can be used to quantify the hydrological impacts of decreasing rainfall and increasing air temperatures in the Greater Horn of Africa (Funk et al., 2015a). In addition, the performance of CHIRPSv2, evaluated over certain regions of the Americas, has demonstrated its ability to reproduce the mean climate and its capacity to estimate extreme precipitation events (Rivera et al., 2019). Furthermore, in Colombia, the best results were obtained on a daily and monthly scale over the Magdalena River basin (Baez-Villanueva et al., 2018). CHIRPSv2 data are suitable for our study, as they perform well in the Caribbean (Centella-Artola et al., 2020), and, in the study by Bathelemy et al. (2022), it was shown that CHIRPSv2 performed well in estimating heavy precipitation based on the 90th percentile.
Sea surface temperatures (SSTs) are very important for monitoring and assessing climate change (IPCC, 2013). They can be derived either from observations from floating or moored buoys (Smith et al., 1996), from satellite observations (Merchant et al., 2014), or from a mixture (in situ + satellite) (HadSST, Rayner et al., 2003; DOSST, Reynolds et al., 2007). In this study, NOAA DOISST version 2.1 data were used; these are also daily sea surface temperature data derived from a combination of in situ sea surface temperature (SST) data obtained from ships and buoys and sea surface temperatures obtained from the Advanced Very-High-Resolution Radiometer (AVHRR) (Reynolds et al., 2007; Huang et al., 2021a). This satellite product is the result of a global file of 0.25° grid points, available over the period 1981–2020. In addition, it has been widely used for climate assessment and monitoring, notably as part of the reanalysis of the NOAA/NCEP climate prediction system (Saha et al., 2010). Work by Huang et al. (2021b) revealed that NOAA DOISST performs well in terms of bias compared with buoy and Argo observations and with the seven SST products.
The World Meteorological Organization's Expert Team on Climate Change Detection and Indices (ETCCDI) has defined 27 indices to characterize extreme precipitation and temperature events in terms of frequency, amplitude, and duration (Peterson et al., 2001). Although the proposed method includes numerous indices based on percentiles, with thresholds set to assess extremes that generally occur a few times a year and not necessarily high-impact events, it has paved the way for numerous research projects in the Caribbean (Stephenson et al., 2014; McLean et al., 2015). Six extreme precipitation indices (see Table 1 for details) were calculated: total annual precipitation (PRCPTOT), number of rainy days (RR1), intensity of rain events (SDII), and heavy precipitation (R95p), calculated with a threshold corresponding to the 95th percentile of the daily precipitation distribution, maximum number of consecutive wet days (CWD), and maximum number of consecutive dry days (CDD).
The spatiotemporal evolution of extreme precipitation in the Greater Antilles was investigated by analyzing the interannual variability in extreme precipitation index anomalies over a long period (1985–2015) and the change in percentage variations in extreme precipitation indices at decadal timescales. To characterize the percentage variations, we chose the Pij index, which is already used in the study by An et al. (2023), whose equation is presented hereafter.
where Pij is the average extreme precipitation index for the jth decade at the ith location and Pi(j+1) is the average extreme precipitation index for the (j+1)th decade at the ith location.
Previous studies have shown that precipitation in the Caribbean, particularly in the Greater Antilles, is influenced by the surface temperature of the Atlantic Ocean and tropical Pacific (Gimeno et al., 2011; Enfield et al., 2001). Thus, to investigate the impact of these basins on extreme precipitation over indices, we selected four large-scale SST indices, namely the Southern Oscillation Index (SOI), the North Atlantic Oscillation (NAO) (Jones et al., 1997), the Tropical South Atlantic (TSA), and the Caribbean Sea Surface Temperature (SST-CAR). Details of these indices can be consulted online: https://psl.noaa.gov/data/climateindices (last access: October 2023).
Analysis of the relationship between two variables is often of great interest for data analysis in research. It generally consists of characterizing the form and intensity of the link (relationship) between variables using a correlation coefficient. For two variables, X and Y, this coefficient is interpreted as (i) linear linkage, where the correlation coefficient is positive when X and Y values change in the same direction (that is, an increase in X leads to an increase in Y); (ii) linear linkage, where the correlation coefficient is negative when X and Y values change in the opposite direction (that is, an increase in X leads to a decrease in Y or vice versa); and (iii) non-linear monotonic linkage, where the correlation coefficient is positive when X and Y change in the same direction as in item (i), but with a small slope (Lewis-Beck, 1995; Sheskin, 2007). In the literature, Pearson's and Spearman's correlation coefficients are often the most widely used to measure the strength or degree of linkage between two variables. In this study, we used Spearman's non-parametric rank correlation coefficient (ρ) to assess the interannual link between extreme precipitation over indices and global SST indices. This non-parametric method was chosen because it does not require a normal distribution for the variables. Spearman also outperforms Pearson's linear coefficient in the case of outliers. On the other hand, linear trends can be detected using Pearson or Spearman tests, but the latter is preferable for monotonic non-linear relationships (Gauthier, 2001; Von Storch and Zwiers, 1999). Spearman's correlation has already been used in several studies to assess the links between teleconnection patterns and precipitation (Ríos-Cornejo et al., 2015; Khadgarai et al., 2021).
Spearman's correlation coefficient is calculated using the following equation:
where rspearman is the correlation coefficient and Ri = rang (Xi) and Si = rang (Yi) are, respectively, the data ranks of variables X and Y (X: extreme precipitation index; Y: large-scale SST index).
To test the significance of the relationship, whether the two variables are correlated or not, we use the t test for a threshold of 0.05 or less. This involves testing the two hypotheses (H0 and H1) based on the value of t to deduce the probability of observing a result that deviates as much as expected from the correlation. The formula for calculating the value of t using Spearman's correlation is as follows:
where n−2 is degrees of freedom and n is sample size.
Changes in precipitation extreme indices
Interannual changes in extreme precipitation indices over the Greater Antilles are shown in Fig. 2. In Fig. 2a, the period from 1985 to 1994 was generally marked by a decline in total annual precipitation. This decline was associated with a decrease in average rainfall intensity (Fig. 2c) and a reduction in the lengths of wet and dry spells (Fig. 2e, f). On the other hand, the period from 1995 to 2004 was mainly characterized by a decrease in the number of rainy days (Fig. 2b), associated with an increase in the average intensity of precipitation (Fig. 2c) and an increase in the contribution of heavy precipitation (Fig. 2d). Furthermore, during the period 2005–2015, an increase in the contribution of heavy precipitation was observed until 2012 (Fig. 2d). This was associated with an increase in the number of rainy days (Fig. 2b), an increase in the average intensity of precipitation (Fig. 2c), and an increase in the length of wet episodes (Fig. 2e).
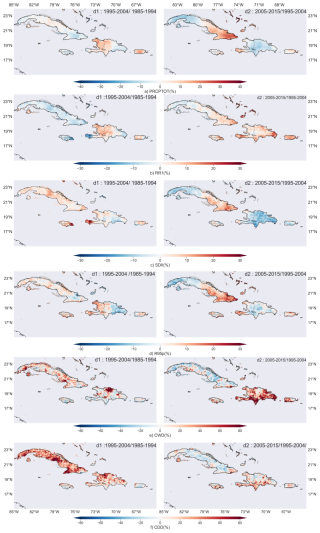
Figure 3Annual change (%) between 2 consecutive decades of precipitation indices over the Greater Antilles (left: 1995–2004 compared to 1985–1994; right: 2005–2015 compared to 1995–2004): (a) PRCPTOT, (b) RR1, (c) SDII, (d) R95p, (e) CWD, and (f) CDD.
Figure 3 shows the annual change in percentage between 2 consecutive decades of precipitation indices over the Greater Antilles (1985–2015). As shown in Fig. 3a, d2, there was an increase in total annual precipitation (PRCPTOT) in southeastern Cuba. This was associated with an increase in the number of rainy days (RR1) (Fig. 3b, d2) and the average intensity of precipitation per rainy day (SDII) (Fig. 3c, d2). These results were also observed in Puerto Rico, with an increase in total annual precipitation associated with an increase in RR1 (Fig. 3a, d2 and b, d2). In addition, a decrease in total annual precipitation (PRCPTOT) was observed on the island of Hispaniola (Dominican Republic and Haiti, except for the southern part) (Fig. 3a, d2). This was associated with a decrease in the average rainfall intensity per wet day (SDII) (Fig. 3c, d1). This decrease in the SDII was also recorded in Puerto Rico (Fig. 3c, d2). For heavy precipitation (R95p), as shown in Fig. 3d, d2, an increase was observed in the southeastern part of Cuba, whereas the whole island (Cuba) was affected in general by a decrease in wet sequences (CWD) (Fig. 3e, d2) and dry sequences (CDD) (Fig. 3f, d2). A decrease in heavy precipitation (R95p) was observed in the central and western regions of Haiti (Fig. 3d, d2). This was accompanied by an increase in wet sequences (CWD) over Haiti (Fig. 3e, d2). The Dominican Republic was also affected by this increase in wet sequences (CWD) (Fig. 3e, d2).
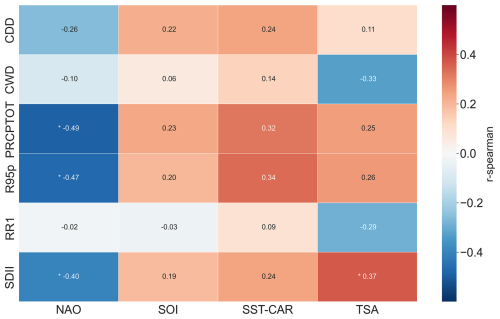
Figure 4Correlation between precipitation indices and large-scale SST in the Greater Antilles (1985–2015). The values in the table are the correlation coefficients of large-scale SST with extremes. The indices on the abscissa are the precipitation extremes, and those on the ordinate are the SST indices. The symbol (*) represents a statistically significant correlation at a threshold less than or equal to 0.05.
Variations in extreme precipitation indices under the influence of variables such as NAO, SOI, TSA, and SST-CAR were analyzed over the Greater Antilles. The influences of large-scale variables were classified as positive, negative, positive significant, or negative significant, as shown in Fig. 4. The results obtained by taking the intersections of the table in Fig. 4 show the values of the correlation coefficient (with its significance *) between the extreme precipitation indices (PRCPTOT, RR1, SDII, R95p, CWD, and CDD) and the influencing variables (NAO, SOI, TSA, and SST-CAR). Thus, the table in Fig. 4 shows that NAO has a negative effect on all extremes, while the other SST-CAR values are positive, except for the number of rainy days (RR1) and the number of consecutive rainy days (CWD). However, the positive phase of the +TSA index had a positive and significant effect on the average rainfall intensity per wet day (SDII), for which a correlation coefficient of 0.37 was obtained. Similarly, with the NAO index, a negative and significant effect (P<0.05) was observed on total annual precipitation (PRCPTOT), average precipitation intensity (SDII), and heavy precipitation (R95p), for which correlation coefficients of 0.49, 0.40, and 0.47, respectively, were obtained.
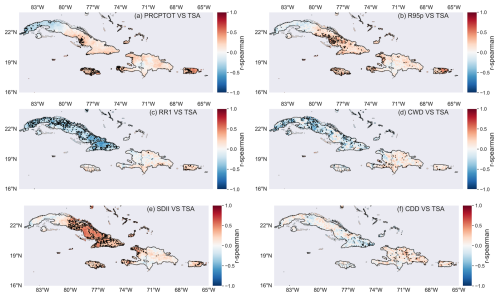
Figure 5Correlation between precipitation indices and the Tropical South Atlantic (TSA) index. The spatial correlation of extremes with TSA (SST average over 0–20° S, 10° E–30° W) for this figure is presented in two columns: the first column contains the indices (a) PRCPTOT vs. TSA, (b) RR1 vs. TSA, and (c) SDII vs. TSA, and the second column contains the indices (b) R95p vs. TSA, (d) CWD vs. TSA, and (f) CDD vs. TSA. Black dots represent areas where correlations are statistically significant at p≤0.05.
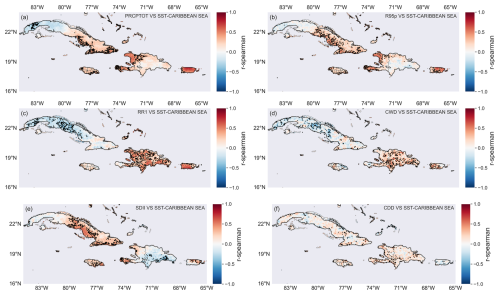
Figure 6Correlation between precipitation indices and Caribbean Sea Surface Temperature (SST-CAR). The spatial correlation of extremes with SST-CAR (SST average 14–16° N, 65–82° W) for this figure is presented in two columns: the first column contains the indices (a) PRCPTOT vs. SST-CAR, (b) RR1 vs. SST-CAR, and (c) SDII vs. SST-CAR, and the second column contains the indices (b) R95p vs. SST-CAR, (d) CWD vs. SST-CAR, and (f) CDD vs. SST-CAR. Black dots represent areas where correlations are statistically significant at p≤0.05.
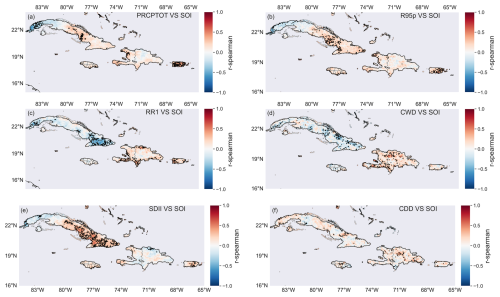
Figure 7Correlation between precipitation indices and the Southern Oscillation Index (SOI). The spatial correlation of extremes with SOI for this figure is presented in two columns: the first column contains the indices (a) PRCPTOT vs. SOI, (b) RR1 vs. SOI, and (c) SDII vs. SOI, and the second column contains the indices (b) R95p vs. SOI, (d) CWD vs. SOI, and (f) CDD vs. SOI. Black dots represent areas where correlations are statistically significant at p≤0.05.
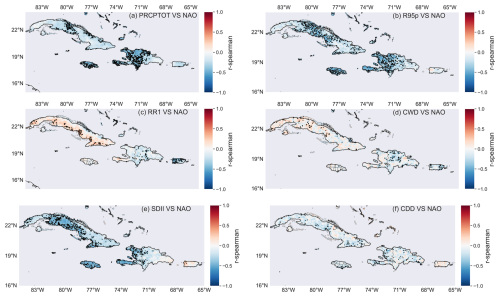
Figure 8Correlation between precipitation indices and the North Atlantic Oscillation (NAO). The spatial correlation of extremes with NAO for this figure is presented in two columns: the first column contains the indices (a) PRCPTOT vs. NAO, (b) RR1 vs. NAO, and (c) SDII vs. NAO, and the second column contains the indices (b) R95p vs. NAO, (d) CWD vs. NAO, and (f) CDD vs. NAO. Black dots represent areas where correlations are statistically significant at p≤0.05.
At a local scale, the results show that teleconnections have had positive and significant effects on extreme precipitation indices over the last 30 years in the countries of the Caribbean region, particularly in the Greater Antilles. The spatial extent of significance is indicated by the symbol (*). Thus, the double symbol (**) represents regions with a significant surface area greater than 50 % of the surface area, while the symbol (*) is used for a significant surface area less than 50 %. The figures (with a threshold at p≤0.05; Figs. 5–8) show the regions or countries over which positive and significant effects were observed for the Greater Antilles.
Figure 5 and Table S1 show the effect of the TSA index on extreme precipitation indices (PRCPTOT, RR1, SDII, R95p, CWD, and CDD) in the Greater Antilles. The results show that South Atlantic tropical warming, corresponding to the positive phase of the +TSA index, has a positive and significant effect (**) on the total annual precipitation (PRCPTOT) and heavy precipitation (R95p) in Puerto Rico (Fig. 5a, b; Table S1d). In Jamaica, it was also associated with an increase in total annual precipitation (PRPCPTOT) and heavy precipitation (R95p) (Fig. 5a, b; Table S1d). In Haiti, more specifically in the northern part, the increase in the average daily rainfall intensity (SDII) was also associated with +TSA warming (Fig. 5e; Table S1d). In northwestern Cuba, this positive phase of +TSA also had a positive and significant effect (**) on mean rainfall intensity per day (SDII) (Fig. 5e; Table S1d). Conversely, in southeastern Cuba, a positive effect was observed for heavy rainfall (R95p) (Fig. 5b; Table S1d).
Figure 6 and Table S1a show the effects of warming of the Caribbean Sea surface temperature (SST-CAR anomaly, averaged over 14–16° N, 65–82° W) on extreme precipitation in the Greater Antilles. In southern Haiti, an increase in total annual precipitation (PRCPTOT) and the number of rainy days (RR1) was observed as the SST-CAR warmed (Fig. 5a, c; Table S1a). In the same region, this increase was also observed for heavy rainfall (R95p) (Fig. 6b; Table S1a). In the Dominican Republic, the positive phase of +SST-CAR is associated with an increase in the duration of wet sequences (CWD) and the number of rainy days (RR1) (Fig. 6c, d; Table S1a). In southeastern Cuba, especially on the Caribbean coast, warming of SST-CAR is associated with an increase in total annual precipitation (PRCPTOT) and heavy precipitation (R95p) (Fig. 6a, b; Table S1a). An increase in heavy precipitation (R95p) during the positive phase of +SST-CAR was also observed in Puerto Rico (Fig. 6c; Table S1a).
Figure 7 and Table S1c show the results of the effect of SOI on extreme precipitation indices (PRCPTOT, RR1, SDII, R95p, CWD, and CDD) in the Caribbean region, particularly on the islands forming the Greater Antilles. In Puerto Rico, as shown (Fig. 7a, b; Table S1c), the positive phase of the +SOI was associated with an increase in total annual precipitation (PRCPTOT) and heavy precipitation (R95p). In Haiti, specifically in the south, this positive phase of the +SOI was associated with an increase in the number of rainy days (RR1), including the duration of wet sequences (CWD) (Fig. 7c, d; Table S1c). In the Dominican Republic, this is associated with an increase in the duration of wet sequences (CWD) (Fig. 7d; Table S1c). In southeastern Cuba, this is associated with an increase in the average intensity of precipitation per rainy day (SDII) and heavy precipitation (R95p) (Fig. 7e, b; Table S1c).
Figure 8 and Table S1b show the results of the effect of the NAO index on extreme precipitation indices (PRCPTOT, RR1, SDII, R95p, CWD, and CDD) in the Greater Antilles. The condensed results for different phases of the NAO index are presented in Table S1b. In contrast to the results for the other large-scale SST indices, the positive phase of the +NAO index was only associated with an increase in the number of rainy days (RR1) over Cuba. This increase was greater for coasts facing the Caribbean Sea (Fig. 8c; Table S1b).
The results of this study showed that extreme precipitation over the period 1985–2015 was influenced by four large-scale sea surface temperature indices (NAO, SOI, TSA, SST-CAR). On the other hand, on a local scale, notably over a few regions, the effects of this influence on certain precipitation indices were statistically significant, while, for other precipitation indices, non-significant effects were observed. To shed more light on these results, we discuss the following points: (i) the impact of sea surface pressure anomalies (NAO, SOI) and (ii) the impact of sea surface temperature anomalies (TSA, SST-CAR).
5.1 Impact of sea surface pressure anomalies (NAO, SOI)
In this study, the influence of the Atlantic Ocean over the Greater Antilles is assessed using two large-scale SST indices, NAO (Hurrell et al., 2003) and TSA. The phases (positive and negative) of the NAO index have been shown to affect circulation in the Northern Hemisphere (Thompson and Wallace, 2000). Also, this index is a measure of the meridional pressure gradient between the North Atlantic Subtropical High (NASH) and the Icelandic Low (Visbeck et al., 2001). Nevertheless, in this study, the results show that the NAO has a negative effect on all extreme precipitation over indices in the region (Fig. 4). In other words, the fluctuation of the NAO index and extreme precipitation over indices change in opposite phases; i.e., the negative (or positive) phase of the NAO is linked to the positive (or negative) phase of the precipitation indices. However, on a local scale (Fig. 8), notably in Cuba and southern Haiti, the positive effect of NAO+, corresponding to a weakening of the subtropical anticyclone (Wallace and Gutzler, 1981; North Atlantic Oscillation, 2023) and leading to very wet conditions in the Caribbean, particularly in the Greater Antilles (Giannini et al., 2000; Mo et al., 2005), is associated with an increase in the number of rainy days (RR1). These results are consistent with those of Jury et al. (2007), for whom the NAO exerts a certain influence on rainfall in the southeastern Caribbean.
In the case of the SOI (Fig. 7), studies have already shown that ENSO influences precipitation patterns in several regions, notably in South and North America (Ropelewski and Halpert, 1987). However, studies (Giannini et al., 2000, 2001b, a; Rodriguez-Vera et al., 2019) have shown that, on an interannual scale, ENSO is one of the most important factors influencing precipitation in the Caribbean. These results are consistent with those of our study. The influence of ENSO was assessed on extreme precipitation indices (Fig. 7) using the SOI. For example, the positive phase of the +SOI (sign of La Niña), characterized by abnormally cold ocean waters in the eastern tropical Pacific, led to an increase in total annual precipitation (PRCPTOT) and heavy precipitation (R95p) in Puerto Rico (Fig. 7a, b). It has also led to an increase in the number of rainy days (RR1), including the duration of wet sequences (CWD) in southern Haiti (Fig. 7c, d), while, in southeastern Cuba, it is associated with an increase in mean rainfall intensity per rainy day (SDII) and heavy precipitation (R95p) (Fig. 7b, e). This influence could be explained by the fact that La Niña brings wet conditions to the Caribbean (Klotzbach, 2011).
5.2 Impact of sea surface temperature anomalies (TSA, SST-CAR)
The evolution of temperature anomalies in the South Atlantic (TSA average over 0–20° S, 10° E–30° W) and Caribbean Sea (SST-CAR average 14–16° N, 65–82° W) presented in Fig. S3 is marked by increasing warming in the Atlantic and Caribbean Sea over the period 1985–2015. In the Caribbean, warming has intensified over the past 3 decades in both seasons (DJF and MAM) (Fig. S2). Thus, the +SST-CAR phase in the Caribbean is associated with an increase in all extremes at the regional scale, apart from the mean rainfall intensity per wet day (SDII) (Fig. 4). Similarly, on a local scale, considering all islands, it leads to an increase in the number of consecutive rainy days (CWD) and in the number of consecutive rainy days on the island of Hispaniola (Haiti and the Dominican Republic) (Fig. 6c, d). The increase in heavy precipitation (R95p) in Puerto Rico, southeastern Cuba, and Haiti is also due to abnormally warm conditions and +SST-CAR in the Caribbean Sea (Fig. 6b). These results are in line with previous research on the influence of sea surface temperature on precipitation in the Caribbean, particularly in the Greater Antilles (Wang et al., 2006, 2007; Wu and Kirtman, 2011). Also, for the link between extreme precipitation and SSTs, it has been shown that the average rainfall intensity per wet day (SDII) and heavy precipitation (R95p) over the Caribbean have a strong correlation with the warm phase of the Caribbean Sea (Peterson et al., 2002). In contrast, the positive +TSA phase (TSA averaged over 0–20° S, 10° E–30° W), which corresponds to warmer sea surface temperatures (SSTs) in the Tropical South Atlantic (TSA), is associated with a southward shift in the ITCZ (Philander et al., 1996) and a weakening of the southeasterly (SE) trade winds (Nobre and Shukla, 1996). This phase, which is also associated with higher precipitation in northeastern Brazil (Utida et al., 2019), influenced precipitation indices over the Greater Antilles, particularly in Puerto Rico and central Cuba, where it led to an increase in heavy precipitation (R95p) (Fig. 5b). On the other hand, in southeastern Cuba and northwestern Haiti, this phase was associated with an increase in rainfall intensity per wet day (SDII) (Fig. 6e). These results are compared with those of the study by Utida et al. (2019), in which the influence of TSA on precipitation was assessed. The results of this study show that the warm phase of +TSA is associated with an increase in precipitation in southeastern Cuba. These results are consistent with this study's findings, namely that TSA influences southeastern Cuba.
This work provides a relevant analysis of the evolution of extreme precipitation and its link with global teleconnections over the Caribbean, particularly the Greater Antilles, over the period 1985–2015. Extreme precipitation indices (PRCPTOT, RR1, R95p, CWD, and CDD) defined by the World Meteorological Organization's Expert Team on Climate Change Detection and Indices (ETCCDI) were calculated. Next, the links between large-scale SST oscillation indices (NAO, SOI, TSA, and SST-CAR) and extreme precipitation indices (PRCPTOT, RR1, R95p, CWD, and CDD) were evaluated and tested using Spearman's correlation coefficient. The results show that warming in the Tropical South Atlantic (TSA) and the Caribbean Sea (mean SST 14–16° N, 65–82° W) and cooling in the eastern tropical Pacific (La Niña) have positive effects on all extreme precipitation indices. Except for the number of rainy days (RR1) and rainy episodes (CWD), negative correlations were observed. However, the significant effects on extremes were greatest at the island scale in the Greater Antilles. For example, in southeastern Cuba and Puerto Rico, there was an increase in heavy precipitation (R95p) and average rainfall intensity per wet day (SDII) associated with the positive phase of the indices (SOI, TSA, and SST-CAR), whereas, in Jamaica and northern Haiti, there were only two indices (TSA and SST-CAR). The number of rainy days (RR1) and the maximum duration of consecutive rainy days (CWD) showed a significant upward trend over southern Haiti and the Dominican Republic, in line with the positive phase of the Southern Oscillation (SOI) and warming east of the surface of the Caribbean Sea.
These results further improve our knowledge of the impact of certain global teleconnections on extreme precipitation in the Greater Antilles. They also highlight the most relevant teleconnection indices (SOI, SST-CAR (average SST-CAR 14–16° N, 65–82° W), and TSA) to be regarded as part of the impact study in the region to limit damage to key economic sectors such as agriculture, biodiversity, health, and energy.
The Climate Hazards Group Infrared Precipitation with Stations (CHIRPS) version 2 satellite data used in this study are available online at https://data.chc.ucsb.edu/products/CHIRPS-2.0/ (Climate Hazards Group, 2023). NOAA OISST v2.1 data can also be accessed online at (https://psl.noaa.gov/data/gridded/data.noaa.oisst.v2.highres.html; Physical Sciences Laboratory, 2023).
The supplement related to this article is available online at https://doi.org/10.5194/esd-16-497-2025-supplement.
Conceptualization: CD, AD, SA. Methodology: CD, AD, SA. Original draft preparation: CD. Review and editing: all authors.
The contact author has declared that none of the authors has any competing interests.
Publisher's note: Copernicus Publications remains neutral with regard to jurisdictional claims made in the text, published maps, institutional affiliations, or any other geographical representation in this paper. While Copernicus Publications makes every effort to include appropriate place names, the final responsibility lies with the authors.
This article is part of the special issue “Methodological innovations for the analysis and management of compound risk and multi-risk, including climate-related and geophysical hazards (NHESS/ESD/ESSD/GC/HESS inter-journal SI)”. It is not associated with a conference.
We would like to thank all those who contributed to the realization of this work. We cannot mention all the names here, but we are very grateful for all the support. We would like to thank Thomas Lagelouze and Abubakar Haruna from the IGE team; Eric Calais, Ralph Bathelemy, Elisée Villiard, and Kelly Guerrier from the URGeo and LMI CARIBACT team; and Didi Sacré Regis from the LMI-Nexus Abidjan team.
This research was carried out with the support of the following: (1) ARTS PhD grant from the Institut de Recherche pour le Développement (IRD), (2) Antenor Firmin PhD grant from the French Embassy in Haiti, (3) CARIBACT International Joint Laboratory, (4) CLIMEXHA Project (Anticipation of Extreme CLIMATE events in HAITI for sustainable development).
This paper was edited by Anais Couasnon and reviewed by two anonymous referees.
Agudelo, P. A., Hoyos, C. D., Curry, J. A., and Webster, P. J.: Probabilistic discrimination between large-scale environments of intensifying and decaying African easterly waves, Clim. Dynam., 36, 1379–1401, https://doi.org/10.1007/s00382-010-0851-x, 2011. a
Almazroui, M., Islam, M. N., Saeed, F., Saeed, S., Ismail, M., Ehsan, M. A., Diallo, I., O'Brien, E., Ashfaq, M., Martínez-Castro, D., Cavazos, T., Cerezo-Mota, R., Tippett, M. K., Gutowski, W. J., Alfaro, E. J., Hidalgo, H. G., Vichot-Llano, A., Campbell, J. D., Kamil, S., Rashid, I. U., Sylla, M. B., Stephenson, T., Taylor, M., and Barlow, M.: Projected Changes in Temperature and Precipitation Over the United States, Central America, and the Caribbean in CMIP6 GCMs, Earth Syst. Environ., 5, 1–24, https://doi.org/10.1007/s41748-021-00199-5, 2021. a
An, D., Eggeling, J., Zhang, L., He, H., Sapkota, A., Wang, Y.-C., and Gao, C.: Extreme precipitation patterns in the Asia–Pacific region and its correlation with El Niño-Southern Oscillation (ENSO), Sci. Rep., 13, 11068, https://doi.org/10.1038/s41598-023-38317-0, 2023. a
Baez-Villanueva, O. M., Zambrano-Bigiarini, M., Ribbe, L., Nauditt, A., Giraldo-Osorio, J. D., and Thinh, N. X.: Temporal and spatial evaluation of satellite rainfall estimates over different regions in Latin-America, Atmos. Res., 213, 34–50, https://doi.org/10.1016/j.atmosres.2018.05.011, 2018. a
Bathelemy R., Brigode P., Boisson D., and Tric, E.: Rainfall in the Greater and Lesser Antilles: Performance of five gridded datasets on a daily timescale, J. Hydrol.: Regional Studies, 43, 101203, https://doi.org/10.1016/j.ejrh.2022.101203, 2022. a
Beharry, S. L., Clarke, R. M., and Kumarsingh, K.: Variations in extreme temperature and precipitation for a Caribbean island: Trinidad, Theor. Appl. Climatol., 122, 783–797, https://doi.org/10.1007/s00704-014-1330-9, 2015. a
Burgess, C. P., Taylor, M. A., Spencer, N., Jones, J., and Stephenson, T. S.: Estimating damages from climate-related natural disasters for the Caribbean at 1.5 °C and 2 °C global warming above preindustrial levels, Reg. Environ. Change, 18, 2297–2312, https://doi.org/10.1007/s10113-018-1423-6, 2018. a
Cantet, P.: Mapping the mean monthly precipitation of a small island using kriging with external drifts, Theor. Appl. Climatol., 127, 31–44, https://doi.org/10.1007/s00704-015-1610-z, 2017. a
Centella-Artola, A., Bezanilla-Morlot, A., Taylor, M. A., Herrera, D. A., Martinez-Castro, D., Gouirand, I., Sierra-Lorenzo, M., Vichot-Llano, A., Stephenson, T., Fonseca, C., Campbell, J., and Alpizar, M.: Evaluation of Sixteen Gridded Precipitation Datasets over the Caribbean Region Using Gauge Observations, Atmosphere, 11, 1334, https://doi.org/10.3390/atmos11121334, 2020. a
Climate Hazards Group: Index of /products/CHIRPS-2.0, Climate Hazards Group [data set], https://data.chc.ucsb.edu/products/CHIRPS-2.0/, last access: February 2023. a
Cook, K. H. and Vizy, E. K.: Hydrodynamics of the Caribbean low-level jet and its relationship to precipitation, J. Climate, 23, 1477–1494, https://doi.org/10.1175/2009JCLI3210.1, 2010. a
Curtis, S. and Gamble, D. W.: Regional Variations of the Caribbean Mid-Summer Drought, Theor. Appl. Climatol., 94, 25–34, https://doi.org/10.1007/s00704-007-0342-0, 2008. a
Daly, C., Helmer, E. H., and Quiñones, M.: Mapping the climate of Puerto Rico. Vieques Culebra, Int. J. Climate, 23, 1359–1381, https://doi.org/10.1002/joc.937, 2003. a
Davis, R. E., Hayden, B. P., Gay, D. A., Phillips, W. L., and Jones, G. V.: The North Atlantic subtropical anticyclone, J. Climate, 10, 728–744, https://doi.org/10.1175/1520-0442(1997)010<0728:TNASA>2.0.CO;2, 1997. a
De Giorgi, A., Solarna, D., Moser, G., Tapete, D., Cigna, F., Boni, G., Rudari, R., Serpico, S. B., Pisani, A. R., Montuori, A., and Zoffoli, S.: Monitoring the Recovery after 2016 Hurricane Matthew in Haiti via Markovian Multitemporal Region-Based Modeling, Remote Sens., 13, 3509, https://doi.org/10.3390/rs13173509, 2021. a
Deopersad, C., Persaud, C., Chakalall, Y., Bello, O., Masson, M., Perroni, A., Carrera-Marquis, D., Fontes de Meira, L., Gonzales, C., Peralta, L., Skerette, N., Marcano, B., Pantin, M., Vivas, G., Espiga, C., Allen, E., Ruiz, E., Ibarra, F., Espiga, F., and Nelson, M.: Assessment of the Effects and Impacts of Hurricane Dorian in the Bahamas, IDB Publications, https://doi.org/10.18235/0002582, 2020. a
Dookie, N., Chadee, X. T., and Clarke, R. M.: Trends in extreme temperature and precipitation indices for the Caribbean small islands: Trinidad and Tobago, Theor. Appl. Climatol., 136, 31–44, https://doi.org/10.1007/s00704-018-2463-z, 2019. a
Enfield, D. B., Mestas-Nunez, A. M., and Trimble, P. J.: The Atlantic Multidecadal Oscillation and Its Relation to Rainfall and River Flows in the Continental U.S., Geophys. Res. Lett., 28, 2077–2080, https://doi.org/10.1029/2000GL012745, 2001. a, b
Frich P., Alexander, L. V., Della-Marta, P., Gleason, B., Haylock, M., Klein Tank, A. M. G., and Peterson, T.: Observed Coherent Changes in Climatic Extremes during the Second Half of the Twentieth Century, Clim. Res., 19, 193–212, https://doi.org/10.3354/cr019193, 2002. a
Funk, C., Peterson, P., Landsfeld, M., Pedreros, D., Verdin, J., Shukla, S., Husak, G., Rowland, J., Harrison, L., Hoell, A., and Michaelsen, J.: The climate hazards infrared precipitation with stations – a new environmental record for monitoring extremes, Sci. Data, 2, 150066, https://doi.org/10.1038/sdata.2015.66, 2015a. a, b
Funk, C., Verdin, A., Michaelsen, J., Peterson, P., Pedreros, D., and Husak, G.: A global satellite-assisted precipitation climatology, Earth Syst. Sci. Data, 7, 275–287, https://doi.org/10.5194/essd-7-275-2015, 2015b. a
Gauthier, T. D.: Detecting trends using Spearman's rank correlation coefficient, Environ. Forensic, 2, 359–362, https://doi.org/10.1006/enfo.2001.0061, 2001. a
Giannini, A., Kushnir, Y., and Cane, M. A.: Interannual Variability of Caribbean Rainfall, ENSO, and the Atlantic Ocean, J. Climate, 13, 297–311, https://doi.org/10.1175/1520-0442(2000)013<0297:IVOCRE>2.0.CO;2, 2000. a, b, c
Giannini, A., Cane, M. A., and Kushnir, Y.: Interdecadal Changes in the ENSO Teleconnection to the Caribbean Region and the North Atlantic Oscillation, J. Climate, 14, 2867–2879, https://doi.org/10.1175/1520-0442(2001)014<2867:ICITET>2.0.CO;2, 2001a. a
Giannini, A., Kushnir, Y., and Cane, M. A.: Seasonality in the impact of ENSO and the North Atlantic High on Caribbean Rainfall, Phys. Chem. Earth B, 28, 143–147, https://doi.org/10.1016/S1464-1909(00)00231-8, 2001b. a
Gimeno, L., Magaña, V., and Enfield, D. B.: Introduction to special section on the role of the Atlantic warm pool in the climate of the Western Hemisphere, J. Geophys. Res., 116, D00Q01, https://doi.org/10.1029/2011JD016699, 2011. a, b
Griffiths, J. F. and Driscoll, J. M.: Survey of climatology, Charles Merril, Columbus, OH, ISBN 9780675099943, 1982. a
Hall, T. C., Sealy, A. M., Stephenson, T. S., Kusunoki, S., Taylor, M. A., Chen, A. A., and Kitoh, A.: Future climate of the Caribbean from a super-high-resolution atmospheric general circulation model, Theor. Appl. Climatol., 113, 271–287, https://doi.org/10.1007/s00704-012-0779-7, 2013. a
Harris, I., Jones, P. D., Osborn, T. J., and Lister, D. H.: Mise à jour des grilles à haute résolution des observations climatiques mensuelles – l'ensemble de données CRU TS3.10, J. Climatol., 34, 623–642, https://doi.org/10.1002/joc.3711, 2014.
Hastenrath, S.: The intertropical convergence zone of the eastern Pacific revisited. Int. J. Climatol. 22, 347–356, https://doi.org/10.1002/joc.739, 2002. a
Huang, B., Liu, C., Banzon, V., Freeman, E., Graham, G., Hankins, B., Smith, T., and Zhang, H.: Improvements of the Daily Optimum Interpolation Sea Surface Temperature (DOISST) Version 2.1, J. Climate, 34, 2923–2939, https://doi.org/10.1175/JCLI-D-20-0166.1, 2021a. a
Huang, B., Liu, C., Freeman, E., Graham, G., Smith, T., and Zhang, H.: Assessment and Intercomparison of NOAA Daily Optimum Interpolation Sea Surface Temperature (DOISST) Version 2.1, J. Climate, 34, 7421–7441, https://doi.org/10.1175/JCLI-D-21-0001.1, 2021b. a
Hurrell, J. W.: Decadal trends in the north Atlantic oscillation: regional temperatures and precipitation, Science, 269, 676–679, https://doi.org/10.1126/science.269.5224.676, 1995. a
Hurrell, J. W., Kushnir, Y., Ottersen, G., and Visbeck, M.: An Overview of the North Atlantic Oscillation, in: The North Atlantic Oscillation: climatic significance and environmental impact, edited by: Hurrell, J. W., Kushnir, Y., Ottersen, G., and Visbeck, M., Geophysical Monograph Series, 134, AGU (American Geophysical Union), Washington, DC, 1–36, https://doi.org/10.1029/134GM01, 2003. a
IPCC: Climate Change 2007, Synthesis Report, Working Group, I, II and III contributions to the Fourth Assessment Report, http://www.ipcc.ch (last access: June 2023), 2007. a
IPCC: Climate Change: The Physical Science Basis, in: Contribution of Working Group I to the Fifth Assessment Report of the Intergovernmental Panel on Climate Change, edited by: Stocker, T. F., Qin, D., Plattner, G.-K., Tignor, M., Allen, S. K., Boschung, J., Nauels, A., Xia, Y., Bex, V., and Midgley, P. M., Cambridge University Press, Cambridge, 1535 pp., ISBN 978-92-9169-138-8, 2013. a
Jones, P. D., Jónsson, T., and Wheeler, D.: Extension to the North Atlantic Oscillation using early instrumental pressure observations from Gibraltar and South-West Iceland, Int. J. Climatol., 17, 1433–1450, https://doi.org/10.1002/(SICI)1097-0088(19971115)17:13<1433::AID-JOC203>3.0.CO;2-P, 1997. a
Joseph, P.: La Caraïbe, données environnementales. Coordonné par Joseph P. Karthala, Paris, et Géode (Groupe de recherche Géographie, développement, environnement de la Caraïbe), ÉÉditions KARTHALA, 458 pp., ISBN 978-2845867567, 2006. a
Jury, M., Malmgren, B. A., and Winter, A.: Subregional precipitation climate of the Caribbean and relationships with ENSO and NAO, J. Geophys. Res., 112, D16107, https://doi.org/10.1029/2006JD007541, 2007. a
Khadgarai, S., Kumar, V., and Pradhan, P. K.: The Connection between Extreme Precipitation Variability over Monsoon Asia and Large-Scale Circulation Patterns, Atmosphere, 12, 1492, https://doi.org/10.3390/atmos12111492, 2021. a
Klotzbach, P. J.: The influence of El Niño–Southern Oscillation and the Atlantic multidecadal oscillation on Caribbean tropical cyclone activity, J. Climate, 24, 721–731, https://doi.org/10.1175/2010JCLI3705.1, 2011. a
Lewis-Beck, M. S.: Data Analysis: An Introduction, Sage Publications, Thousand Oaks, CA, ISBN 9780803957725, 1995. a
Love, T. B., Kumar, V., Xie, P., and Thiaw, W.: A 20-year daily Africa precipitation climatology using satellite and gauge data, 14th Conf. on Applied Meteorology, Seattle, WA, Amer. Meteor. Soc., P5.4, 2004, http://ams.confex.com/ams/pdfpapers/67484.pdf (last access: December 2023), 2004. a
Mariani, M., Holz, A., Veblen, T. T., Williamson, G., Fletcher, M.-S., and Bowman, D. M. J. S.: Climate change amplifications of climate-fire teleconnections in the Southern Hemisphere, Geophys. Res. Lett., 45, 5071–5081, https://doi.org/10.1029/2018GL078294, 2018. a, b
Martens, B., Waegeman, W., Dorigo, W. A., Verhoest, N. E. C., and Miralles, D. G.: Terrestrial evaporation response to modes of climate variability, npj Climate and Atmospheric Science, 1, 43, https://doi.org/10.1038/s41612-018-0053-5, 2018. a
Marto, R., Alvarez, L., and Suarez, D.: Background Paper: LAC Small Island Development States, IDB Publications, https://doi.org/10.18235/0009228, 2014. a
McLean, N. M., Stephenson, T. S., Taylor, M. A., and Campbell, J. D.: Characterization of Future Caribbean Rainfall and Temperature Extremes across Rainfall Zones, Adv. Meteorol., 2015, 1–18, https://doi.org/10.1155/2015/425987, 2015. a, b, c
Merchant, C. J., Embury, O., Roberts-Jones, J., Fiedler, E., Bulgin, C. E., Corlett, G. K., Good, S., McLaren, A., Rayner, N., Morak-Bozzo, S., and Donlon, C.: Sea surface temperature datasets for climate applications from Phase 1 of the European Space Agency Climate Change Initiative (SST CCI), Geosci. Data J., 1, 179–191, https://doi.org/10.1002/gdj3.20, 2014. a
Mo, K. C., Chelliah, M., Carrera, M. L., Higgins, R. W., and Ebisuzaki, W.: Atmospheric Moisture Transport over the United States and Mexico as Evaluated in the NCEP Regional Reanalysis, J. Hydrometeor., 6, 710–728, https://doi.org/10.1175/JHM452.1, 2005. a, b
Moron, V., Frelat, R., Jean-Jeune, P. K., and Gaucherel, C.: Interannual and intra-annual variability of rainfall in Haiti (1905–2005), Clim. Dynam., 45, 915–932, https://doi.org/10.1007/s00382-014-2326-y, 2015. a
Naciones Unidades, Comision economica para America Latima y el Caribe: Repuplica Dominica: evaluacion de los danos ocasionados por el huracan Georges, sus implicaciones para el desarrollo del pais, 91 pp., https://hdl.handle.net/11362/25344 (last access: June 2023), 1988. a
Nobre, P. and Shukla, J.: Variation of Sea Surface Temperature, Wind Stress and Rainfall over the Tropical Atlantic and South America, J. Climate, 9, 2464–2479, https://doi.org/10.1175/1520-0442(1996)009<2464:VOSSTW>2.0.CO;2, 1996. a
North Atlantic Oscillation: NOAA's Climate Prediction Center, https://www.climate.gov/news-features/understanding-climate/climate-variability-north-atlantic-oscillation, last access: 8 December 2023. a
Nurse, M.: Droughts, hurricanes, pandemic, and hope: Current realities im- pacting Caribbean agriculture. CARICOM Today, 5 June, https://www.caricomstats.org/E-CISTAR/2020/06/03/droughts-hurricanes-pandemic-and-hope-current-realities-impacting-caribbean-agriculture/ (last access: 19 March 2023), 2023. a
Peterson, T. C., Folland, C., Gruza, G., Hogg, W., Mokssit, A., and Plummer, N.: Report of the activities of the Working Group on Climate Change Detection and related rapporteurs, World Meteorl. Organ. Tech. Doc. 1071, 143 pp., Comm. for Climatol., World Meteorol. Organ., Geneva, 2001. a, b
Peterson, T. C., Taylor, M. A., Demeritte, R., Duncombe, D. L., Burton, S., Thompson, F., Porter, A., Mercedes, M., Villegas, E., Semexant Fils, R., Klein Tank, A., Martis, A., Warner, R., Joyette, A., Mills, W., Alexander, L., and Gleason, B.: Recent changes in climate extremes in the Caribbean region: RECENT CHANGES IN CLIMATE EXTREMES IN THE CARIBBEAN REGION, J. Geophys. Res., 107, ACL 16-1–ACL 16-9, https://doi.org/10.1029/2002JD002251, 2002. a, b, c
Philander, S. G. H., Gu, D. D., Lambert, G., Li, T., Halpern, D., Lau, N., and Pacanowski, R. C.: Why the ITCZ Is Mostly North of the Equator, J. Climate, 9, 2958–2972, https://doi.org/10.1175/1520-0442(1996)009<2958:WTIIMN>2.0.CO;2, 1996. a
Physical Sciences Laboratory: NOAA OI SST V2 High Resolution Dataset, Physical Sciences Laboratory [data set], https://psl.noaa.gov/data/gridded/data.noaa.oisst.v2.highres.html, last access: July 2023. a
Rayner, N. A., Parker, D. E., Horton, E. B., Folland, C. K., Alexander, L. V., Rowell, D. P., Kent, E. C., and Kaplan, A.: Global analyses of sea surface temperature, sea ice, and night marine air temperature since the late nineteenth century, J. Geophys. Res., 108, 4407, https://doi.org/10.1029/2002JD002670, 2003. a
Reynolds, R. W., Smith, T. M., Liu, C., Chelton, D. B., Casey, K. S., and Schlax, M. G.: Daily high-resolution blended analyses for sea surface temperature, J. Climate, 20, 5473–5496, https://doi.org/10.1175/2007JCLI1824.1, 2007. a, b
Ríos-Cornejo, D., Penas, A., Álvarez-Esteban, R., and del Río, S.: Links between teleconnection patterns and precipitation in Spain, Atmos. Res., 156, 14–28, https://doi.org/10.1016/j.atmosres.2014.12.012, 2015. a
Rivera, J. A., Hinrichs, S., and Marianetti, G.: Using CHIRPS dataset to assess wet and dry conditions along the semiarid central-western Argentina, Adv. Meteor., 18, 8413964, https://doi.org/10.1155/2019/8413964, 2019. a
Rodrigues, M., Peña-Angulo, D., Russo, A., Zúñiga-Antón, M., and Cardil, A.: Do climate teleconnections modulate wildfire-prone conditions over the Iberian Peninsula?, Environ. Res. Lett., 16, 044050, https://doi.org/10.1088/1748-9326/abe25d, 2021. a
Rodriguez-Vera, G., Romero-Centeno, R., Castro, C. L., and Castro, V. M.: Coupled Interannual Variability of Wind and Sea Surface Temperature in the Caribbean Sea and the Gulf of Mexico, J. Climate, 32, 4263–4280, https://doi.org/10.1175/JCLI-D-18-0573.1, 2019. a
Ropelewski C. F. and Halpert, M. S.: Global and regional scale precipitation and temperature patterns associated with El Niño–Southern Oscillation, Mon. Weather Rev., 115, 1606–1626, https://doi.org/10.1175/1520-0493(1987)115<1606:GARSPP>2.0.CO;2, 1987. a
Saha, S., Moorthi, S., Pan, H.-L., Wu, X., Wang, Jiande, Nadiga, S., Tripp, P., Kistler, R., Woollen, J., Behringer, D., Liu, H., Stokes, D., Grumbine, R., Gayno, G., Wang, Jun, Hou, Y.-T., Chuang, H., Juang, H.-M. H., Sela, J., Iredell, M., Treadon, R., Kleist, D., Van Delst, P., Keyser, D., Derber, J., Ek, M., Meng, J., Wei, H., Yang, R., Lord, S., van den Dool, H., Kumar, A., Wang, W., Long, C., Chelliah, M., Xue, Y., Huang, B., Schemm, J.-K., Ebisuzaki, W., Lin, R., Xie, P., Chen, M., Zhou, S., Higgins, W., Zou, C.-Z., Liu, Q., Chen, Y., Han, Y., Cucurull, L., Reynolds, R. W., Rutledge, G., and Goldberg, M.: The NCEP Climate Forecast System Reanalysis, B. Am. Meteorol. Soc., 91, 1015–1058, https://doi.org/10.1175/2010BAMS3001.1, 2010. a
Sheskin, D. J.: Handbook of Parametric and Nonparametric Statistical Procedures, 4th edn., Boca Raton, FL, Chapman & Hall/CRC, ISBN 978-1439858011, 2007. a
Smith, T. M., Reynolds, R. W., Livezey, R. E., and Stokes, D. C.: Reconstruction of Historical Sea Surface Temperatures Using Empirical Orthogonal Functions, J. Climate, 9, 1403–1420, https://doi.org/10.1175/1520-0442(1996)009<1403:ROHSST>2.0.CO;2, 1996. a
Stephenson, T. S., Vincent, L. A., Allen, T., Van Meerbeeck, C. J., McLean, N., Peterson, T. C., Taylor, M. A., Aaron-Morrison, A. P., Auguste, T., Bernard, D., Boekhoudt, J. R. I., Blenman, R. C., Braithwaite, G. C., Brown, G., Butler, M., Cumberbatch, C. J. M., Etienne-Leblanc, S., Lake, D. E., Martin, D. E., McDonald, J. L., Ozoria Zaruela, M., Porter, A. O., Santana Ramirez, M., Tamar, G. A., Roberts, B. A., Sallons Mitro, S., Shaw, A., Spence, J. M., Winter, A., and Trotman, A. R.: Changes in extreme temperature and precipitation in the Caribbean region, 1961–2010, Int. J. Climatol., 34, 2957–2971, https://doi.org/10.1002/joc.3889, 2014. a, b, c, d
Taylor, M. A. and Enfield, D. B.: Chen, Influence of the tropical Atlantic versus the tropical Pacific on Caribbean rainfall, J. Geophys. Res., 107, 3127, https://doi.org/10.1029/2001JC001097, 2002. a
Taylor, M. A., Clarke, L. A., Cantella, A., Bezanilla, A., Stephenson, T. S., Jones, J., Campbell, J. D., Vichot, A., and Charlery, J.: Future Caribbean Climates in a World of Rising Temperatures: The 1.5 vs 2.0 Dilemma, J. Climate, 31, 2907–2926, https://doi.org/10.1175/JCLI-D-17-0074.1, 2018. a
Thompson, D. W. J. and Wallace, J. M.: Annular modes in the extratropical circulation Part I: Month-to-month variability, J. Climate, 13, 1000–1016, https://doi.org/10.1175/1520-0442(2000)013<1000:AMITEC>2.0.CO;2, 2000. a
Thorncroft, C. and Hodges, K.: African easterly wave variability and its relationship to Atlantic tropical cyclone activity, J. Climate, 14, 1166–1179, https://doi.org/10.1175/1520-0442(2001)014<1166:AEWVAI>2.0.CO;2, 2001. a
Utida, G., Cruz, F. W., Etourneau, J., Bouloubassi, I., Schefuß, E., Vuille, M., Novello, V. F., Prado, L. F., Sifeddine, A., Klein, V., Zular, A., Viana, J. C. C., and Turcq, B.: Tropical South Atlantic influence on Northeastern Brazil precipitation and ITCZ displacement during the past 2300 years, Sci. Rep., 9, 1698, https://doi.org/10.1038/s41598-018-38003-6, 2019. a, b
Vichot‐Llano, A., Martinez‐Castro, D., Bezanilla‐Morlot, A., Centella‐Artola, A., and Giorgi, F.: Projected changes in precipitation and temperature regimes and extremes over the Caribbean and Central America using a multiparameter ensemble of RegCM4, Int. J. Climatol., 41, 1328–1350, https://doi.org/10.1002/joc.6811, 2021. a
Visbeck, M. H., Hurrell, J. W., Polvani, L., and Cullen, H. M.: The North Atlantic Oscillation: Past, present, and future, P. Natl. Acad. Sci. USA, 98, 12876–12877, https://doi.org/10.1073/pnas.231391598, 2001. a
Von Storch, H. and Zwiers, F. C.: Statistical Analysis in Climate Research. Cambridge University Press, Cambridge, 0 521 45071 3, 1999. a
Wallace, J. M. and Gutzler, D. S.: Teleconnections in the Geopotential Height Field during the Northern Hemisphere Winter, Mon. Weather Rev., 109, 784–812, https://doi.org/10.1175/1520-0493(1981)109<0784:TITGHF>2.0.CO;2, 1981. a
Wang, C., Enfield, D. B., Lee, S., and Landsea, C. W.: Influences of the Atlantic Warm Pool on Western Hemisphere Summer Rainfall and Atlantic Hurricanes, J. Climate, 19, 3011–3028, https://doi.org/10.1175/JCLI3770.1, 2006. a
Wang, C., Lee, S., and Enfield, D. B.: Impact of the Atlantic Warm Pool on the Summer Climate of the Western Hemisphere, J. Climate, 20, 5021–5040, https://doi.org/10.1175/JCLI4304.1, 2007. a
Wu, R. and Kirtman, B. P.: Caribbean Sea rainfall variability during the rainy season and relationship to the equatorial Pacific and tropical Atlantic SST, Clim. Dynam., 37, 1533–1550, https://doi.org/10.1007/s00382-010-0927-7, 2011. a