the Creative Commons Attribution 4.0 License.
the Creative Commons Attribution 4.0 License.
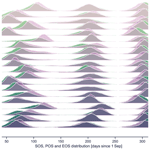
Widespread greening suggests increased dry-season plant water availability in the Rio Santa valley, Peruvian Andes
Lorenz Hänchen
Cornelia Klein
Fabien Maussion
Wolfgang Gurgiser
Pierluigi Calanca
Georg Wohlfahrt
In the semi-arid Peruvian Andes, the growing season is mostly determined by the timing of the onset and retreat of the wet season, to which annual crop yields are highly sensitive. Recently, local farmers in the Rio Santa basin (RSB) reported more erratic rainy season onsets and further challenges related to changes in rainfall characteristics. Previous studies based on local rain gauges, however, did not find any significant long-term rainfall changes, potentially linked to the scarce data basis and inherent difficulties in capturing the highly variable rainfall distribution typical for complex mountain terrain. To date, there remains considerable uncertainty in the RSB regarding changes in plant-available water over the last decades. In this study, we exploit satellite-derived information of high-resolution vegetation greenness as an integrated proxy to derive variability and trends of plant water availability. By combining MODIS Aqua and Terra vegetation indices (VIs), datasets of precipitation (both for 2000–2020) and soil moisture (since 2015), we explore recent spatio-temporal changes in the vegetation growing season. We find the Normalized Difference Vegetation Index (NDVI) to be coupled to soil moisture on a sub-seasonal basis, while NDVI and rainfall only coincide on interannual timescales. Over 20 years, we find significant greening in the RSB, particularly pronounced during the dry season (austral winter), indicating an overall increase in plant-available water over the past 2 decades. The start of the growing season (SOS) exhibits high interannual variability of up to 2 months compared to the end of the growing season (EOS), which varies by up to 1 month, therefore dominating the variability of the growing season length (LOS). The EOS becomes significantly delayed over the analysis period, matching the observed dry-season greening. While both in situ and gridded rainfall datasets show incoherent changes in annual rainfall for the region, Climate Hazards InfraRed Precipitation with Station data (CHIRPS) rainfall suggests significant positive dry-season trends for 2 months coinciding with the most pronounced greening. As the greening signal is strongly seasonal and reaches high altitudes on unglaciated valley slopes, we cannot link this signal to water storage changes on timescales beyond one rainy season, making interannual rainfall variability the most likely driver. Exploring El Niño–Southern Oscillation (ENSO) control on greening, we find an overall increased LOS linked to an earlier SOS in El Niño years, which however cannot explain the observed greening and delayed EOS. While our study could not corroborate anecdotal evidence of recent changes, we confirm that the SOS is highly variable and conclude that rainfed farming in the RSB would profit from future efforts being directed towards improving medium-range forecasts of the rainy season onset.
- Article
(12881 KB) - Full-text XML
- BibTeX
- EndNote
The Rio Santa valley in the tropical Peruvian Andes is characterized by high seasonal variability of precipitation with a rainy season lasting from approximately September to April, when 70 %–80 % of annual precipitation occurs, followed by a dry season with little to no rainfall (e.g., Schauwecker et al., 2014). In this region, rainfall seasonality is strongly controlled by tropical easterlies related to the South American monsoon system (Garreaud, 2009). Interannual differences in rainfall totals may reach up to 100 %, linked to high variability in the driving atmospheric circulation patterns. This variability is partly driven by the El Niño–Southern Oscillation (ENSO) phenomenon, but ENSO influences on rainfall patterns in the tropical Andes are complex and not coherent in space and time. For the Cordillera Blanca (the mountain range on the eastern side of the Rio Santa valley), studies suggest a general dry (wet) signal following El Niño (La Niña) events (Vuille et al., 2008; Maussion et al., 2015). But this linear relationship does not hold true for all events and is dependent on individual, localized anomalies in the upper tropospheric flows. Furthermore, the primary focus of most studies has been ENSO effects on anomalies in glacier mass balances in the highest altitudes of the Cordillera Blanca, which might not reflect the effects at lower altitudes in the Rio Santa basin (RSB). At the same time, studies focusing on the Pacific watershed of the Peruvian coast suggest a complex pattern where both dry and wet anomalies might occur in the adjacent mountain ranges (where the RSB is located) following El Niño events (Sanabria et al., 2018, 2019; Rau et al., 2017).
Small-scale farmers living along the slopes of the RSB are cultivating their crops closely linked to the water availability imposed by this seasonality. These subsistence-based cultivation practices are increasingly threatened by rural exodus, expansion of mining activities, industrialization of agriculture, and overall economic growth and modernization (Carey et al., 2014; Crabtree, 2002). Apart from these challenges, local farmers recently reported perceived changes in rainfall patterns, which additionally threaten their livelihoods (Mark et al., 2010; Perez et al., 2010; Gurgiser et al., 2016). Specifically, they reported (a) a higher variability in the onset of the rainy season, which complicates the planning for an ideal sowing date, (b) a higher occurrence of dry spells during the growing season leading to crop loss, and (c) more frequent occurrences of crop damaging events such as intense rainfalls, hail and ground frost. In contrast to these reports, the same authors (Gurgiser et al., 2016) could not find evidence for the reported patterns by analyzing two local rain gauge time series.
The complex terrain of the Andes is an important factor hampering the robustness of information on rainfall patterns and changes with large-scale rainfall drivers like ENSO being modulated by topography over short distances, creating microclimates. Hence, available data from rain gauges, often of questionable quality, additionally suffer from insufficient spatial coverage. Therefore, spatio-temporal distributions of rainfall across the valley and potential recent changes in patterns or seasonality still remain uncertain and have been reported neither for the spatial domain of the RSB (Schauwecker et al., 2014; Gurgiser et al., 2016) nor for larger scales (i.e., the tropical Andes region) (Vuille et al., 2003). But together with other climate variables, fine-scale precipitation patterns are a dominant driver for changes in ecosystem productivity (Nemani et al., 2003; Huxman et al., 2004; Knapp and Smith, 2001; Bonan, 2008; Beer et al., 2010; de Jong et al., 2013) and are of importance for downstream water shortages which to date are only assessed by quantifying the glacier mass-balance-runoff relation (e.g., Baraer et al., 2012; Bury et al., 2013; Mark et al., 2010; Condom et al., 2012; Kaser et al., 2003) or by future projections with locally highly uncertain results (Urrutia and Vuille, 2009; Buytaert and De Bièvre, 2012).
In the particular climatic setting of the semi-arid Peruvian Andes, the growing season of vegetation is mostly determined by seasonal water availability. Given most agricultural land is rainfed, crops and managed grasslands similarly rely on the seasonal rains (Rodriguez-Iturbe et al., 1999; Svoray and Karnieli, 2011; Schwinning et al., 2004; Forzieri et al., 2014). Other potentially limiting variables (i.e., radiation and temperature) are of minor importance for ecosystem productivity or successful rain-fed farming at the transitions between dry and wet season (Camberlin et al., 2007). Therefore, a strong relationship of remotely sensed vegetation indices (VIs), such as the Normalized Difference Vegetation Index (NDVI) (Rouse et al., 1974) can be expected to show a clear, albeit lagged response to available water, including rainfall and temporally delayed storage components of the hydrological cycle (i.e., soil moisture and snow/ice storages) (Richard and Poccard, 1998; Potter and Brooks, 1998; Wu et al., 2015). VIs have been successfully used for detecting climate anomalies (Karnieli et al., 2010), revealing long-term changes in climate (Richardson et al., 2013, 2018; Zhang, 2005) and understanding local effects of large-scale patterns such as ENSO (Kogan, 2000). VIs can also be exploited to calculate metrics of land surface phenology (LSP). Widely used metrics are related to phenophases, greening or senescence of plants, usually named start, peak and end of the season (SOS, POS, EOS) and can be used to deduce interannual variability or spatio-temporal changes in ecosystem status in response to weather events and climate change (e.g., Vrieling et al., 2013; Xu et al., 2016).
As the semi-arid climate causes water availability to be the key limiting factor for plant growth in the RSB, vegetation greenness as represented by the NDVI can serve as a bulk indicator for variability and changes in plant-available water. The main advantage of exploiting NDVI for understanding recent changes in water availability in the context of agriculture is the unprecedented spatio-temporal resolution on the one hand and the integrative nature of plant greenness on the other hand, meaning plant greenness is dynamically correlated with the environmental parameter which is limiting plant growth the most. As plant water availability is directly related to soil moisture (SM), rainfall data (i.e from weather stations), although representing the input variable in the terrestrial hydrological cycle, cannot fully represent the spatial redistribution and storage of water in the system, which is crucial to plant water availability. Furthermore, local rainfall observations have limited spatial representativity in complex mountainous terrain. Satellite-based retrievals of soil moisture alone on the other hand do not capture the vegetation response to changes in water availability and suffer from lower resolutions and/or short retrieval periods as well as from higher estimate uncertainties due to high sensitivity to vegetation cover. For this reason, the overarching goal of the study is to shed light on the interannual variability and decadal changes in water availability in the RSB in the context of perceived changes by local farmers by utilizing NDVI as an integrated proxy of plant water availability. For that, we
-
examine the seasonal and interannual relationship between NDVI, SM and different rainfall datasets;
-
quantify decadal changes (long-term trends) in NDVI at the annual and monthly level in the particular setting of the RSB, thus inferring changes in plant-available water;
-
analyze the temporal characteristics of the vegetation growing season based on LSP metrics to detect shifts in plant growth seasonality;
-
explore the relationship between NDVI behavior and ENSO, a major driver of the large-scale rainfall-controlling circulation patterns in the region.
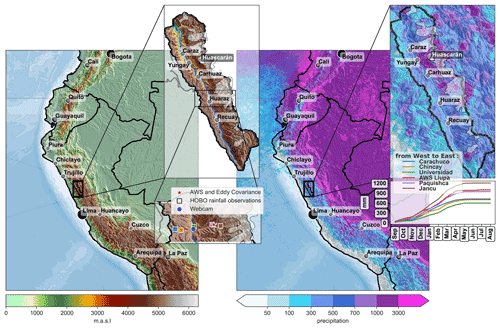
Figure 1Left: Overview of the topography (based on SRTM data, USGS EROS Archive, 2021). Important towns are shown (relative population by marker size). Black box marks RSB location and inset shows upper RSB including most important towns. Blue line indicates the Santa river; the range west (east) of the river is the Cordillera Negra (Blanca). Approximate glacier outlines are shown in white polygons. Small inset panel shows locations of rainfall observation transect by the AgroClimHuaraz (https://agroclim-huaraz.info/, last access: 15 March 2022) project. Right: TRMM rainfall climatology (Bookhagen and Strecker, 2008) shows rainfall gradient over central-west South America and the RSB (inset). Lower right-hand inset roughly illustrates the east–west precipitation gradient in the RSB of rain gauge observations (cumulative rainfall, averaged from 2016 to 2019).
2.1 Study area and local climate
The Rio Santa basin (also known as Callejón de Huaylas) is located in northwest central Peru, approximately 400 km northwest from the capital Lima (see Fig. 1). In several sections, the valley is densely populated while the majority of the land surface is used either for agriculture in the lower and extensive grazing in the higher altitudes. The complex interactions between the Andes' topography, the position of the Intertropical Convergence Zone (ITCZ), ENSO and the South American monsoon system shape the precipitation gradient between the Amazon Basin, which is among the rainiest places worldwide (Killeen et al., 2007; Espinoza et al., 2015), and the dry deserts along the Pacific coast with close to zero precipitation (Rau et al., 2017). The RSB is located between these two extremes, and consequently there is a precipitation gradient between the Cordillera Blanca range on the east slope of the valley and the Cordillera Negra range on the west slope within a few kilometers' distance as seen by rain gauge measurements and satellite precipitation retrievals in Fig. 1.
2.2 Vegetation indices data
For this analysis we acquired complete time series of NDVI and PR (pixel reliability) layers of the MODIS Terra and Aqua satellites (i.e., products MOD13Q1 (Didan, 2015a) and MYD13Q1 (Didan, 2015b), respectively) for a subset covering the RSB in NetCDF format. Both products contain images with a spatial resolution of 250 m and are composited from the best radiometric and geometric quality pixels (i.e., low clouds, low viewing zenith angle and highest values of NDVI) in a 16 d observation period. The composites in MOD13Q1 and MYD13Q1 are purposely phased 8 d apart and use the same spatial grid, which allows combining them. Both MOD13Q1 and MYD13Q1 were filtered to only retain pixels with the MODLAND quality assurance (QA) criteria “VI produced with good quality” and “VI produced but check other QA” and in a second step by removing low-quality VIs (“Lowest quality”, “Quality so low that it is not useful”, “L1B data faulty” and “Not useful for any other reason/not processed”). In a third step, only pixels with a “low” and an “average” aerosol quantity were included and pixels where adjacent clouds, mixed clouds and/or possible shadows were detected were removed from the dataset. Finally, the two filtered datasets were combined to cover 19 growing seasons (starting from 1 September) from 2000 to 2020 in 8 d temporal and 250 m spatial resolution. The consistent dataset was exported into a set of GeoTiff files for processing with the Decomposition and Analysis of Time Series software (DATimeS) software (Belda et al., 2020).
2.3 Precipitation and soil moisture data
We used gridded precipitation data from the Climate Hazards InfraRed Precipitation with Station data (CHIRPS) dataset (Funk et al., 2015) in 0.05∘ × 0.05∘ spatial and 1 d temporal resolution and subset the data for the evaluated MODIS NDVI period 2000–2020 and the spatial extent of the RSB. CHIRPS rainfall is derived by a combination of satellite and rain gauge data. In particular, precipitation is derived from thermal infrared cold cloud duration observations and blended with rain gauge data by weighted interpolation. Due to its comparably high spatial and high temporal resolution it is regularly used for regional studies in complex terrain as found in the RSB (e.g., Rivera et al., 2018; Torres-Batlló and Martí-Cardona, 2020; Segura et al., 2019). In addition, monthly L3 GPM–IMERG (Global Precipitation Measurement – Integrated MultisatellitE Retrievals) precipitation data were used for comparison (Huffman et al., 2019). IMERG provides global estimations of precipitation based on microwave satellite observations in combination with surface precipitation rain gauges. In contrast to CHIRPS, IMERG rainfall incorporates direct satellite radar measurements but suffers from coarser spatio-temporal resolution (0.1∘ × 0.1∘). We also compared our results with data from local weather stations operated by the National Meteorological and Hydrological Service of Peru (SENAHMI). Stations that suffered from larger data gaps over the NDVI time period were excluded from further analysis. This resulted in three suitable stations, all located along the valley floor (see Fig. 3d for approximate locations). Finally, we acquired Soil Moisture Active Passive (SMAP) Enhanced Level 3 radiometer global daily 9 km soil moisture retrieval beginning from March 2015 (O'Neill et al., 2021). We used the morning overpass times (denoted in the data layer names by the tag “AM”) of the Dual Channel Algorithm (DCA) and included both observations with recommended and uncertain quality as the data availability would have been low if the data with uncertain quality had been excluded. Using the MODIS NDVI data we constructed a mask to only include SMAP data which correspond to NDVI observations.
2.4 NDVI time series pre-processing
As our study area covers a variety of land-cover types, we used two state-of-the-art methods to derive LSP metrics from the NDVI time series: (1) Whittaker smoother (wt) (Atzberger and Eilers, 2011) and (2) Gaussian process regression (GPR) (Rasmussen, 2004), both implemented in DATimeS software (Belda et al., 2020). The Whittaker smoother (Whittaker, 1922) calculates least squares with a penalty based on how noisy the input time series is. The smoothness is controlled by a single parameter (λ). GPR is a non-parametric Bayesian approach where (hyper-)parameters are determined in a probabilistic way in the calculation. Recent studies showed advantages of GPR over standard models for gap filling and fusion of various biophysical parameters (Belda et al., 2020; Pipia et al., 2019; Mateo-Sanchis et al., 2018). Besides being promising in terms of yielding accurate estimates, GPR is different from other models since it determines uncertainty estimates for each pixel in addition to the fitted data. However, as differences between the results of the two methods turned out to be negligible, all analyses shown are based on GPR. The DATimeS software was set up by using a daily time step. To account for possible greening or browning trends in the NDVI time series, we used a seasonal amplitude to determine SOS and EOS. POS is defined as the day where NDVI reaches its seasonal maximum. All other settings were DATimeS default settings (i.e., SOS and EOS detection at 30 % amplitude; min prominence of 20 %; min separation of SOS and EOS of 100 d and no further smoothing method applied). Additionally we filtered the LSP results by masking the data on certain conditions: (i) pixels where the length of the growing season (LOS) was longer than 365 d; (ii) pixels where the amplitude or the maximum NDVI was 1 or greater; (iii) pixels where the order of SOS, POS and EOS was not given (e.g., POS after EOS); (iv) pixels where any SOS, POS or EOS were more than 365 d after the first September of the corresponding season were removed. Finally, we removed outliers by restricting the analysis to the 1st and the 99th percentile of SOS, POS and EOS. Parts of our study area also contain areas with irrigated agriculture, where two (or more) maxima in the NDVI signal per season are expected. To exclude such pixels where the NDVI seasonality is evidently decoupled from seasonal water availability, we used an approach based on autocorrelation analysis (Verstraete et al., 2008). The time series was split into 14 months (growing season ± 1 month) for each season (e.g., 1 July 2000 to 31 August 2001) and a 3-week rolling window was applied to the calculation of autocorrelation for each pixel and season independently. By detecting the local maxima of the autocorrelation, the number of peaks in each pixel was detected, and finally all pixels with more than one local autocorrelation maximum were excluded from further analysis (on average 7.23 % pixels per season removed with σ = 1.42 %). These pixels are exclusively located at the highest altitudes and close to the Rio Santa river. Additionally, we masked the whole time series with the global Land Cover product of the Copernicus Climate Change Service (C3S) at 300 m resolution (https://cds.climate.copernicus.eu/cdsapp#!/dataset/satellite-land-cover?tab=overview, last access: June 2020). Specifically, we removed all pixels which intersected with nine specific land-cover classes corresponding to flooded vegetation, urban areas, bare areas, water, snow and ice. We did not account for land-cover changes during the 20-year time period and masked the whole time series with the land-cover data from 2018.
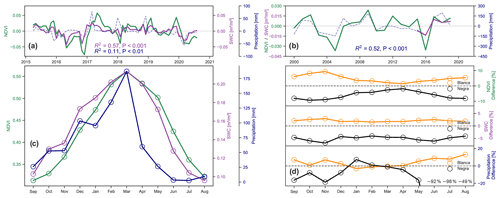
Figure 2(a) Domain mean monthly anomalies of detrended NDVI, SMAP soil moisture and CHIRPS rainfall time series between 2015 and 2020 (for the calculation of the coefficient of determination (R2) NDVI was shifted back 1 month for CHIRPS but not for SMAP data). (b) Domain mean annual anomalies of detrended NDVI, SMAP and CHIRPS time series between 2000 and 2019 (as SMAP data are only available for 4 complete years (2015–2019), it is shown but without R2 statistics). (c) Seasonal cycle of the whole RSB from 2015 to 2020 for NDVI, SMAP and CHIRPS data. (d) Relative differences of Cordillera Negra and Cordillera Blanca against the domain mean seasonal cycle for NDVI, SMAP and CHIRPS from 2015 to 2020.
3.1 Seasonal relationship between NDVI, soil moisture and rainfall
We first evaluate how the average regional NDVI relates to soil moisture and rainfall information from CHIRPS on a seasonal basis. Conceptually, rainfall is the primary input into the hydrological cycle, but since part of that rainfall is redistributed, soil moisture is more directly related to plant growth. This relation is illustrated in Fig. 2a where the domain-average detrended monthly anomalies of NDVI show significant co-variability with the soil moisture anomalies, including a response to drier and wetter years. This suggests that SMAP soil moisture data are suitable to detect short-term variability in plant water availability on a regional scale. However, its spatial resolution remains too coarse for evaluation of sub-valley soil water distribution, and its availability from 2015 onward does not allow trend analyses. For the CHIRPS data the relation of monthly anomalies is less clear with an explained variance through rainfall of 11 % for 2015–2020 (shown in Fig. 2a) and 14 % for 2000–2020 (not shown). For annual anomalies however, the explained variance reaches 52 % (Fig. 2b). These findings highlight strong NDVI sensitivity to interannual rainfall variability, while on shorter timescales there is little sensitivity of NDVI to rainfall anomalies. Given the described restrictions in soil moisture data, we rely on NDVI in combination with regional rainfall information for subsequent analyses.
In situ measurements indicate that seasonal rainfall shows a west–east gradient across the valley (see Fig. 1). This is confirmed by the gridded datasets in Fig. 2d, where all three datasets represent this difference in seasonal water availability between the two ranges. Monthly rainfall differences show less rainfall for the Cordillera Negra, particularly during the early rainy season with approximately 12 % for September, October and November compared to the average for the entire valley. (Fig. 2d). While CHIRPS suggests only minor differences between the ranges during the peak rainy season (January–March), corresponding lagged NDVI values (approximately February–April) remain lower (higher) on the Cordillera Negra (Blanca) with a minimum in April (Fig. 2d). This illustrates that NDVI is a useful metric to capture the response of vegetation to cumulative water availability in this region, which may better reflect vegetation and crop sensitivities than rainfall metrics alone. Soil moisture shows a smaller difference of +2 % and −5 % on average for the Cordillera Blanca and Negra compared to the complete RSB, which is most likely related to the coarser resolution of the SMAP dataset.
3.2 Decadal changes in NDVI and their consistencies with rainfall datasets
Next, we investigate potential changes in plant water availability in the RSB since 2000. In addition to vegetation greenness as represented by the NDVI, we consider three different rainfall datasets for detection of changes that may explain NDVI trends. The NDVI data themselves (Fig. 3a) reveal a greening tendency across the RSB, amounting to +10 % over the considered period, suggesting an increase in plant water availability given the strong NDVI sensitivity to SM illustrated in Fig. 2. In contrast, the rain gauge dataset and CHIRPS (Fig. 3b and d, respectively) do not show any significant changes during the observation period, while the IMERG dataset (Fig. 3c) indicates a significant reduction in rainfall. Stable annual rainfall in CHIRPS and station data in combination with the greening signal render considerable rainfall decrease as shown by IMERG unlikely. On a sub-seasonal basis, monthly NDVI trends as shown in Fig. 4 reveal widespread greening that is particularly pronounced during the dry season (approximately May to September). In May and August, this greening is in line with the CHIRPS data, while the other dry-season months show no clear rainfall signal. As illustrated in Fig. 2a, the NDVI signal is lagging behind the rainfall signal, and therefore correspondence between changes in rainfall and NDVI might also be affected by a few months' lag (Tote et al., 2011). Significant browning occurs in only a relatively small fraction of the area, consistently localized in urban areas or where mines are operating.
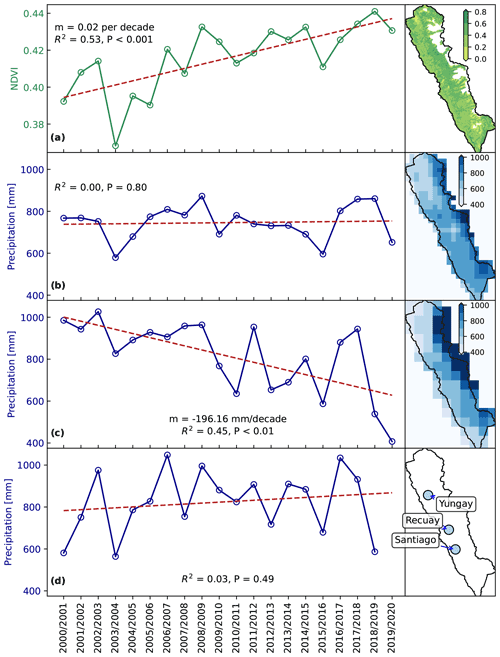
Figure 3Seasonal domain mean time series and linear regressions for NDVI and three different rainfall products: (a) MODIS NDVI, (b) CHIRPS, (c) IMERG and (d) local weather station data (SENAHMI). Small maps show mean NDVI over the time series, mean annual precipitation sums and weather station locations.
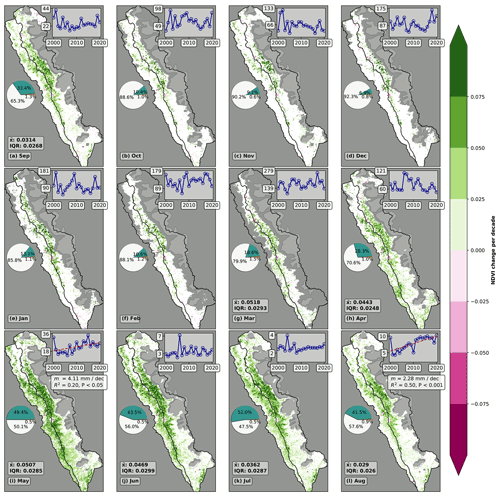
Figure 4Monthly greening and browning of NDVI. For months with at least 15 % significant pixels, median slopes values () and interquartile ranges (25 %–75 %) are shown. Only significant pixels (P < 0.05) are shown; white color indicates non-significant pixels, while gray areas correspond to no data due to frequent cloud cover or excluded land cover. Pie charts show relative frequencies of greening, browning and non-significant pixels. Small panels (blue line plots) show domain mean CHIRPS rainfall data for the respective month and additionally decadal slope (m) and linear regression statistics for significant (P < 0.05) relationships.
3.3 Characteristics of the vegetation growing season
To further explore if and how the vegetation growing seasonality may have changed, we extracted spatio-temporal information on LSP. As Fig. 5 indicates, SOS shows a large interannual variability, with a median of 79 d since 1 September and a maximum range of 60 d. In contrast, POS and EOS show much smaller fluctuations over time (median of 203 and 304 d since 1 September and maximum ranges of 30 and 34, respectively). Consequently, the growing season length (LOS) is mostly governed by the high variability of SOS. The Cordillera Negra shows both later SOS and earlier EOS in comparison to the Cordillera Blanca, while POS is similarly distributed for both ranges, which corresponds to the monthly differences shown in Fig. 2b–d. These differences between Cordillera Blanca and Negra remain clearly visible even on the pixel scale (Fig. 6a–c with an SOS 9 d later, an EOS 7 d earlier and an LOS 15 d shorter; median values of all pixels). Neither SOS nor LOS show larger-scale changes over the 20-year time series across the valley as seen in Fig. 6. EOS on the other hand, is shifted towards later dates on the valley scale, without dominant localized patterns that would suggest land-use change driving this shift (Fig. 6f). Although we identify a robust trend in EOS, it remains small compared to the variability of SOS and hence has little effect on the overall LOS.
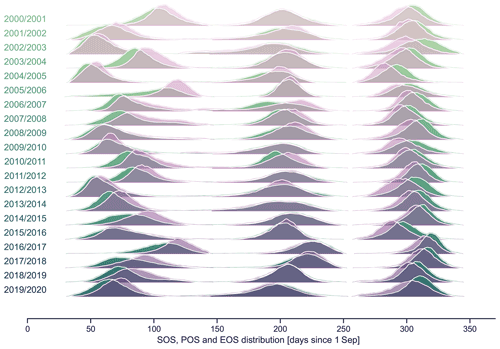
Figure 5Kernel density estimations (KDEs) for SOS, POS and EOS for each growing season of all valid pixels in the RSB. Green (Purple) color refers to pixels located east (west) of the Rio Santa (Cordillera Negra and Blanca, as in indicated in Fig. 1).
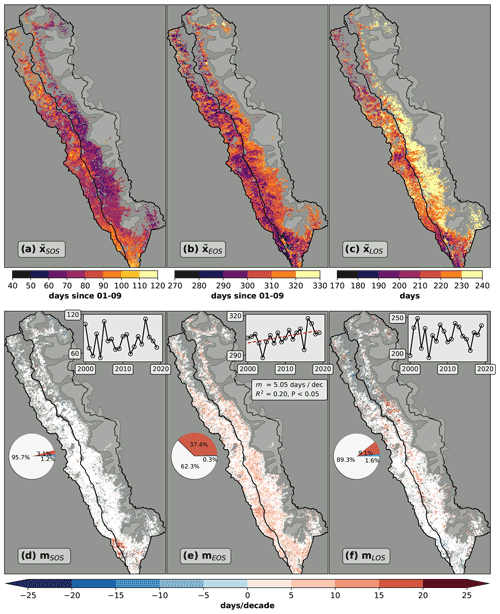
Figure 6Maps of LSP characteristics and trends. First row (a–c): median values of (a) SOS, (b) EOS and (c) LOS for the RSB. Only pixels where the full time series is available (20 seasons) are shown. Second row (d–f): linear regression for the same parameters; maps show decadal slope of the same parameters; inset scatterplots show time series of the domain median values with regression statistics if the regression is significant (P < 0.05). Only significant pixels (P < 0.05) are shown, white color indicates non-significant pixels, while gray areas correspond to no data. Pie charts indicate relative percentages of significant pixels, where red color indicates a forward shift and blue color a backward shift of the LSP metrics.
3.4 Influence of ENSO on interannual NDVI variability
Our results on pronounced vegetation greening at the end of the rainy season paired with a significant May rainfall trend suggested by CHIRPS point towards a later retreat of the rainy season. We now want to investigate whether the succession of ENSO phases over the last 2 decades may have produced this delay in the EOS signal. Therefore, we categorized NDVI and rainfall mean seasonal cycles by partitioning them into El Niño, La Niña and neutral phases. As Fig. 7b–d indicates, we find that early season (October to December) precipitation tends to be enhanced under El Niño conditions, although not significantly except in the local observations for November (Fig. 7d). This might be favoring early greening after the dry season (Fig. 7a, significant for November). At the same time, we find tendencies of lower (higher) mean seasonal precipitation (September to August) under El Niño (La Niña) conditions (not significant). For the three investigated rainfall products these are −3.6 %, −7.9 % and −1.5 % during El Niño phases (+7.1 %, +8.3 %, +4.4 % during La Niña) for CHIRPS, IMERG and local weather station observations compared to the mean seasonal precipitation of the complete time series (mean of all available years, independent of ENSO phases). Regarding vegetation, the increased early season plant-available water seems to trigger a contrasting greening response over the seasonal cycle in the RSB: early rainfalls induce favorable conditions for early plant growth and may allow earlier sowing for farmers. Expected reduced greening linked to less plant-available water during El Niño is insignificant in our analysis but mostly affects peak monsoon rainfall (see Fig. 7) when plant water stress should be low. This may explain why the associated reduction in mean annual precipitation and peak monsoon precipitation has little effect on the NDVI signal later in the rainy season (Fig. 7a). Although the investigated time series features only one multi-year El Niño event (seasons 2014/15, 2015/16), we suspect that the accumulated lack of rain during such events (−8.5 %, −3.9 %, +7.4 % and −20.7 %, −29.3 %, −17.5 % for the seasons 2014/2015 and 2015/2016 for CHIRPS, IMERG and local weather station observations) may have a cumulative detrimental effect on plant growth and result in overall browning tendencies as annual intermediate storages feeding soil moisture and sub-surface flows may become depleted earlier in the season. For the growing season 2016/2017 after the 2015/2016 El Niño this might be the case, as the growing season is clearly delayed (for most pixels the latest SOS, POS and EOS of the whole time series, compare Fig. 5). Yet, for this particular growing season, November rainfall is extremely reduced (by −52 %, −64 %, −92 % for the three rainfall products), which might be unrelated to the previous El Niño event but a similar pattern occurs in the growing season 2005/2006, where SOS is severely delayed and November precipitation is reduced (by −48 %, −26 %, −85 % for the three rainfall products) following a phase of El Niño. As Fig. 8 shows, anomalies in NDVI show a non-linear response to anomalies of the Niño 3.4 index. From September to December though, there is a positive correlation between the two variables with 18 % to 28 % explained variance, significant only for November and December. In May, where the strongest greening occurs (compare Fig. 4), the correlation remains insignificant at approximately 7 % explained variance, similar to other months at the end of the rainy season. Therefore, we cannot explain the observed changes in NDVI and EOS by ENSO alone.
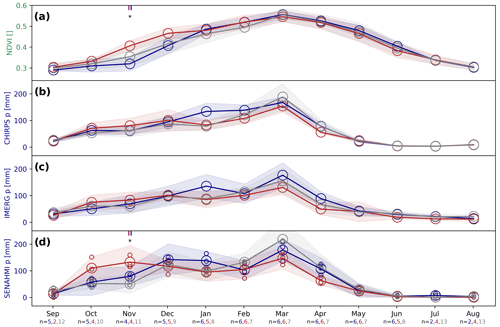
Figure 7Mean monthly seasonal time series for the 2000–2020 time series of NDVI and three rainfall products. Red (blue, gray) color indicates the month in El Niño (La Niña, neutral) classification after Trenberth (1997) of Niño 3.4. sea surface temperature anomalies (SSTa). We shifted the time series of SSTa by forward by 3 months to account for lagged responses of rainfall in the RSB (Maussion et al., 2015). Below the x axis, the number of months of each phase are displayed. Stars indicate significant results according to a Kruskal–Wallis and post hoc Conover's test (P < 0.05, corresponding phase marked by colored bars above the star). For panel (d), the average time series of three stations in the RSB were used, smaller circles indicating values of the three individual stations. Locations of these stations are shown in the bottom right panel of Fig. 3.
By combining metrics of LSP and statistical analyses of spatio-temporal data of MODIS NDVI in combination with SMAP soil moisture and different rainfall datasets, we aimed at creating a more robust picture of differences in water availability across the RSB and of temporal changes therein. The observed annual change towards vegetation greening, particularly widespread during the dry season, strongly suggests an increase in water availability. This is however not reflected in annual rainfall totals of any of the rainfall datasets, while soil moisture data, which shows reasonable agreement with intra-seasonal NDVI variability, are not available for the MODIS era. On the other hand, we found 52 % of regional interannual variability in NDVI to be explained by CHIRPS rainfall estimates suggesting strong rainfall control on NDVI variability in this region. We did not find strong near-immediate responses of NDVI to CHIRPS rainfall on a monthly basis (see Fig. 2). This may be a result of the fact that the NDVI–rainfall co-variability strongly varies throughout the season: during the wet season, plant growth is unlikely to be limited by water availability, but wet-season anomalies likely have an effect later in the season as water storages become depleted. In contrast, positive rainfall anomalies during the drier months can show an immediate increase in plant greenness, while negative anomalies may not result in any response. Accordingly, the time lag between rainfall and NDVI varies dynamically throughout each individual season.
Consistent with other studies which relied on CHIRPS data for trend analyses (e.g., Segura et al., 2019; Torres-Batlló and Martí-Cardona, 2020), our results suggest CHIRPS data to be suitable for regional studies in the Andes, but results should be interpreted with care and ideally compared with other independent data sources as conducted here, particularly for sub-catchment scales. We find the strongest vegetation greening during the drier months, which is at the same time the only period with significant CHIRPS trends in May and August. Because precipitation sums are small at that time, these changes might be below the precision of precipitation measurements, particularly in complex terrain, and trend magnitudes should be treated with caution. In fact, our analysis of different rainfall datasets on the domain scale gives inconclusive and inconsistent results regarding changes in annual precipitation totals, as previously reported by other authors (e.g., Gurgiser et al., 2016; Schauwecker et al., 2014; Vuille et al., 2003). This illustrates the feeble precipitation data basis and the uncertainty that comes with assessments that exclusively consider rainfall trends in the region, while highlighting the value of vegetation-focused analyses for assessing plant water availability in the context of rain-fed farming.
Overall, we found the mean seasonal cycle of NDVI across the RSB to be shifting towards higher values since 2000, with a reduction in amplitude linked to more pronounced late wet-season/dry-season greening. As many studies on changes in VIs in semi-arid areas suggest, greening patterns are not coherent and dominant drivers are diverse. Although currently greening appears to be the dominant signal across the Andes (and many other regions), one has to account for regional changes in climate and land use from case to case (Fensholt et al., 2012). The same applies for studies beyond regional scales (i.e., Peru), where the diversity of ecosystems and gradients in environmental variables may constrain transferable conclusions (Polk et al., 2020). Previously, a variety of potential drivers for greening in the tropical Andes were reported. Among these are the primary succession of recently deglaciated areas (Young et al., 2017), forestation activities (e.g., Aide et al., 2019) and agricultural land-use expansion (Bury et al., 2013). Although these mechanisms most likely also occurred in the Rio Santa basin during the observation period, they cannot explain how greening during the dry season occurs independent of altitude, aspect or land-cover type. By visually comparing pixels which show very intense values of greening with RGB imagery, we discovered some areas which were affected by land-cover change. These were mainly located at higher altitudes dominated by grassland and shrub (so-called puna) ecosystems. In some of these locations, the changes were related to afforestation of evergreen conifers in certain locations of the Cordillera Blanca (not shown). These pixels only occur in small numbers and therefore cannot be the dominant cause of the identified greening. Hence, we are confident that the widespread greening, particularly over the drier months, is linked to increased water availability indicating potential changes in the seasonality of rainfall and vegetation growth.
As known for the Amazon, ENSO-driven extreme events such as the drought during 2015/2016 can have complex effects such as having contrary anomalies in greening and photosynthesis for forests (Yang et al., 2018). For the (tropical) Andes region, little research was conducted regarding the effects of larger-scale circulation on vegetation. Related to farming, the highly variable SOS and consequently LOS in the RSB are probably the largest challenge for farmers as planning for sowing and crop choice can be difficult under these conditions. This is especially pronounced on the Cordillera Negra, where water availability is lower and LOS is shorter. The spatially widespread trend towards delayed EOS dates is in line with the identified dry-season greening and includes the relatively dry Cordillera Negra, where multi-year storages such as glaciers or wetlands are absent. This supports the hypothesis of a trend induced by changes in water availability, especially since magnitude, spatial distribution and seasonal timing are comparable with the rest of the valley, hence rendering other drivers of greening on temporal scales beyond one rainy season unlikely. Influences from anthropogenic activities can potentially cause a decoupling of naturally occurring water inputs and vegetation. This can be related to land-use practices such as irrigation, fertilizing or tilling. In spite of this, there are several arguments for the validity of our analysis. First, large parts of the RSB are characterized by small-scale, subsistence-based, rain-fed, non-industrial agriculture where a large-scale decoupling is not expected. Hence, we account for areas (i.e., at the valley floor) where a multi-modal growing season is realized by irrigation. Second, increasing glacial melt during the past decades might have increased (sub-surface) runoff and facilitated an extension of the agricultural growing season by irrigation. But as neither the magnitude nor the spatial pattern of greening is distinguishable between the glaciated Cordillera Blanca and non-glaciated Cordillera Negra slopes and hence farmers predominantly reported negative impacts (cf. Gurgiser et al., 2016) related to climate, this seems unlikely to be relevant. We want to point out that potential change in water availability during the late wet season remains not fully understood as (i) the vegetation decouples from the water availability signal later in the season as other factors becoming limiting and the explanatory power of NDVI data becomes limited and (ii) the availability of cloud-free scenes is poor during the wet season which causes uncertainties (see dry- and wet-season panels in Fig. 4). By contrast, the delay in EOS in a large proportion of the valley (see Fig. 6f) suggests a potential shift towards a slower retreat of the rainy season and/or slower decay of plant-available water accumulated during the rainy season.
In summary, we are mostly unable to confirm the local farmers' reports (see Sect. 1). Our results indicate that SOS in the RSB is featured with high interannual variability, but this variability has not significantly changed in the 20 years of observation. In spite of this, the farmers' perceptions seem comprehensible if experienced simultaneously with challenges of a different nature. Furthermore, the farmers reported increasing dry spells and more frequent occurrences of detrimental events (e.g., hail, frost). Regarding the dry spells, one methodological constraint is the focus on seasonal unimodal pixels, as severe drought events during the growing season might result in bi- or multi-modal seasonal cycles of NDVI. Nevertheless, an ongoing significant increase in severe dry spells is somewhat contrary to the observed greening pattern on a regional scale but cannot be precluded on the local scale or might be not noticeable in the NDVI signal as farmers might take measures if their crops are threatened by droughts. Regarding extreme meteorological events, our analysis does not allow clear statements as the information in NDVI is accumulative and, additionally, such events might occur locally only. But again, we do not expect detrimental effects on the seasonal cycle of the majority of the valley as we find widespread greening.
In recent years, our understanding of the hydroclimatological mechanisms has improved, particularly in the Andean regions. However, the discovered changes in water availability are spatially variable across the Andes as different interacting mechanisms modify the hydroclimatic system on different timescales (e.g., ENSO (Garreaud, 2009; Arias et al., 2021), Pacific Decadal Oscillation (PDO) (Campozano et al., 2020), seasonality of southern Pacific Anticyclone (al Fahad et al., 2020) and Bolivian High (Segura et al., 2019) circulation systems and consequently displacement of the ITCZ). No consistent pattern of rainfall in- or decrease for the period 2000–2020 is reported for either the tropical Andes (Rabatel et al., 2013) or the RSB (Schauwecker et al., 2014; Gurgiser et al., 2016), which could explain the increased plant water availability found in our study. Here, we find significantly increased early season NDVI and precipitation under El Niño conditions with only one significant month and small rainfall sums. Hence, we find non-significant tendencies of decreased mean seasonal precipitation values which are in line with glacier mass balance studies in the RSB (Kaser et al., 2003; Vuille et al., 2008; Maussion et al., 2015). The modulation of dry-season precipitation is rarely the focus of either glaciologists or climatologists and therefore remains poorly understood.
Understanding the drivers of the greening in the RSB remains challenging and raises several questions. We found that ENSO sequences for the observation period cannot explain the observed greening and delayed EOS. This is in line with a study on the impact of ENSO cycles on continental evaporation by Miralles et al. (2014). They suggest that El Niño is associated with negative evaporation anomalies in parts of the Andes and illustrate a recovery from El Niño-dominated evaporation conditions until approximately 2001 towards La Niña-dominated conditions starting in 2007. The early 2000s have a neutral El Niño tendency though, which again suggests that ENSO phases are unlikely to be the dominant driver for the later EOS. Globally, CO2 fertilization is thought to be the dominant driver for vegetation greening (e.g., Sitch et al., 2015; Zhu et al., 2016) as photosynthesis rates are accelerated and the water use efficiency of plants can be increased by stomatal closure with higher CO2 availability. But as water limitation can negate these benefits (e.g., Gray et al., 2016; Reich et al., 2014) and we find several indications of increased dry-season plant water availability, we suggest the greening to be governed by it, as previously observed for other, more thoroughly investigated semi-arid regions (e.g., Sahel (Dardel et al., 2014; Brandt et al., 2019; Huber et al., 2011; Hickler et al., 2005; Herrmann et al., 2005; Anyamba and Tucker, 2005; Eklundh and Olsson, 2003), southern Africa (Fensholt et al., 2012) or Australia (Donohue et al., 2009)). Additionally, greening induced by CO2 fertilization should be particularly pronounced during times when water availability is not the limiting factor (i.e., around POS), which contrasts with our findings. The observed greening trend might also induce a feedback of increased transpiration bringing more moisture from the soils into the atmosphere, which might be especially relevant during the dry season where this could lead to the beneficial recycling of moisture and promote feedbacks of rainfall and plant transpiration (Spracklen et al., 2012).
Changes in water availability are great concerns for local society as many inhabitants of the RSB are subsistence-based farmers who rely on rain-fed agriculture. To date, drivers of changes in water availability in the RSB remain unclear and the feeble climate data basis hinders an understanding of spatial patterns and temporal trends.
Our study illustrates that vegetation indices such as the NDVI can be exploited as an integrative proxy of water availability and to examine the plausibility of gridded datasets of coinciding parameters at a regional scale and in data-scarce environments. Specifically, we quantified the changes and variability of NDVI, derived land surface phenology metrics and analyzed several rainfall products. We find changes in annual rainfall between three products not to be coherent in space and time, while the NDVI data reveal a widespread greening trend, particularly pronounced during the dry season with low rainfall sums. Based on greening seasonality, we find the SOS to be strongly variable, while peak greening and the end of the growing season exhibit little variability in time. We find indications of increased early season but decreased peak monsoon precipitation during El Niño events, resulting in favorable conditions for early plant growth as water availability is crucial early in the season but less important during peak monsoon.
In consideration of the high variability in SOS and associated challenges for farmers, we suggest that future research should attempt to improve SOS forecasts derived from atmospheric circulation patterns. This could enable farmers to develop strategies to decrease risks of crop failure and optimize sowing dates. Although remote sensing nowadays provides information at unprecedented spatial resolution, we also emphasize the need for more and high-quality local measurements (e.g., automatic weather stations, flux measurements and Long Term Ecological Research (LTER) sites) to broaden the knowledge on the coupling between vegetation and hydroclimatic components in the Andes.
Code and datasets generated and/or analyzed during this study are available from the corresponding author on reasonable request.
LH performed the analysis and wrote the paper. CK, GW and FM advised and assisted LH in the analysis. CK prepared code for trend analysis; FM pre-processed the local weather station and rain gauge data. WG provided valuable local expertise on the RSB. All authors contributed to the interpretation of the results and to the writing and/or review of the paper.
The contact author has declared that neither they nor their co-authors have any competing interests.
Publisher's note: Copernicus Publications remains neutral with regard to jurisdictional claims in published maps and institutional affiliations.
We thank the handling editor and two anonymous reviewers for reading the paper critically and suggesting substantial improvements. We want to acknowledge our collaborators Rolando Cruz Encarnación and Alejo Cochachín Rapre for data collection and sensor maintenance, Mario Rohrer for providing access to the METEODAT platform where we acquired SENAHMI weather station data and Santiago Belda for his support with the DATimeS software. Furthermore, this research was supported by the Action CA17134 SENSECO (Optical synergies for spatiotemporal sensing of scalable ecophysiological traits) funded by COST (European Cooperation in Science and Technology).
This study was conducted in the frame of the AgroClim Huaraz project, funded by the Earth System Sciences call (“Water in Mountain Regions”, 2018) of the Austrian Academy of Sciences (OEAW).
This paper was edited by Somnath Baidya Roy and reviewed by two anonymous referees.
Aide, T. M., Grau, H. R., Graesser, J., Andrade-Nuñez, M. J., Aráoz, E., Barros, A. P., Campos-Cerqueira, M., Chacon-Moreno, E., Cuesta, F., Espinoza, R., Peralvo, M., Polk, M. H., Rueda, X., Sanchez, A., Young, K. R., Zarbá, L., and Zimmerer, K. S.: Woody vegetation dynamics in the tropical and subtropical Andes from 2001 to 2014: Satellite image interpretation and expert validation, Glob. Change Biol., 25, 2112–2126, https://doi.org/10.1111/gcb.14618, 2019. a
al Fahad, A., Burls, N. J., and Strasberg, Z.: How will Southern Hemisphere subtropical anticyclones respond to global warming? Mechanisms and seasonality in CMIP5 and CMIP6 model projections, Clim. Dynam., 55, 703–718, https://doi.org/10.1007/s00382-020-05290-7, 2020. a
Anyamba, A. and Tucker, C. J.: Analysis of Sahelian vegetation dynamics using NOAA-AVHRR NDVI data from 1981–2003, J. Arid Environ., 63, 596–614, https://doi.org/10.1016/j.jaridenv.2005.03.007, 2005. a
Arias, P. A., Garreaud, R., Poveda, G., Espinoza, J. C., Molina-Carpio, J., Masiokas, M., Viale, M., Scaff, L., and van Oevelen, P. J.: Hydroclimate of the Andes Part II: Hydroclimate Variability and Sub-Continental Patterns, Front. Earth Sci., 8, p. 666, https://doi.org/10.3389/feart.2020.505467, 2021. a
Atzberger, C. and Eilers, P. H.: A time series for monitoring vegetation activity and phenology at 10-daily time steps covering large parts of South America, Int. J. Digit. Earth, 4, 365–386, https://doi.org/10.1080/17538947.2010.505664, 2011. a
Baraer, M., Mark, B. G., Mckenzie, J. M., Condom, T., Bury, J., Huh, K. I., Portocarrero, C., Gómez, J., and Rathay, S.: Glacier recession and water resources in Peru's Cordillera Blanca, J. Glaciol., 58, 134–150, https://doi.org/10.3189/2012JoG11J186, 2012. a
Beer, C., Reichstein, M., Tomelleri, E., Ciais, P., Jung, M., Carvalhais, N., Rödenbeck, C., Arain, M. A., Baldocchi, D., Bonan, G. B., Bondeau, A., Cescatti, A., Lasslop, G., Lindroth, A., Lomas, M., Luyssaert, S., Margolis, H., Oleson, K. W., Roupsard, O., Veenendaal, E., Viovy, N., Williams, C., Woodward, F. I., and Papale, D.: Terrestrial gross carbon dioxide uptake: Global distribution and covariation with climate, Science, 329, 834–838, https://doi.org/10.1126/science.1184984, 2010. a
Belda, S., Pipia, L., Morcillo-Pallarés, P., Rivera-Caicedo, J. P., Amin, E., De Grave, C., and Verrelst, J.: DATimeS: A machine learning time series GUI toolbox for gap-filling and vegetation phenology trends detection, Environ. Modell. Softw., 127, 104666, https://doi.org/10.1016/j.envsoft.2020.104666, 2020. a, b, c
Bonan, G. B. (2008). Forests and climate change: forcings, feedbacks, and the climate benefits of forests, Science, 320, 1444–1449, https://doi.org/10.1126/science.1155121, 2008. a
Bookhagen, B. and Strecker, M. R.: Orographic barriers, high-resolution TRMM rainfall, and relief variations along the eastern Andes, Geophys. Res. Lett., 35, 6403, https://doi.org/10.1029/2007GL032011, 2008. a
Brandt, M., Hiernaux, P., Rasmussen, K., Tucker, C. J., Wigneron, J. P., Diouf, A. A., Herrmann, S. M., Zhang, W., Kergoat, L., Mbow, C., Abel, C., Auda, Y., and Fensholt, R.: Changes in rainfall distribution promote woody foliage production in the Sahel, Communications Biology, 2, 1–10, https://doi.org/10.1038/s42003-019-0383-9, 2019. a
Bury, J., Mark, B. G., Carey, M., Young, K. R., McKenzie, J. M., Baraer, M., French, A., and Polk, M. H.: New Geographies of Water and Climate Change in Peru: Coupled Natural and Social Transformations in the Santa River Watershed, Ann. Assoc. Am. Geogr., 103, 363–374, https://doi.org/10.1080/00045608.2013.754665, 2013. a, b
Buytaert, W. and De Bièvre, B.: Water for cities: The impact of climate change and demographic growth in the tropical Andes, Water Resour. Res., 48, 8503, https://doi.org/10.1029/2011WR011755, 2012. a
Camberlin, P., Martiny, N., Philippon, N., and Richard, Y.: Determinants of the interannual relationships between remote sensed photosynthetic activity and rainfall in tropical Africa, Remote Sens. Environ., 106, 199–216, https://doi.org/10.1016/j.rse.2006.08.009, 2007. a
Campozano, L., Robaina, L., and Samaniego, E.: The Pacific decadal oscillation modulates the relation of ENSO with the rainfall variability in coast of Ecuador, Int. J. Climatol., 40, 5801–5812, https://doi.org/10.1002/joc.6525, 2020. a
Carey, M., Baraer, M., Mark, B. G., French, A., Bury, J., Young, K. R., and McKenzie, J. M.: Toward hydro-social modeling: Merging human variables and the social sciences with climate-glacier runoff models (Santa River, Peru), J. Hydrol., 518, 60–70, https://doi.org/10.1016/j.jhydrol.2013.11.006, 2014. a
Condom, T., Escobar, M., Purkey, D., Pouget, J. C., Suarez, W., Ramos, C., Apaestegui, J., Tacsi, A., and Gomez, J.: Simulating the implications of glaciers' retreat for water management: a case study in the Rio Santa basin, Peru, Water Int., 37, 442–459, https://doi.org/10.1080/02508060.2012.706773, 2012. a
Crabtree, J.: The impact of neo-liberal economics on Peruvian peasant agriculture in the 1990s, J. Peasant Stud., 29, 131–161, https://doi.org/10.1080/03066150412331311049, 2002. a
Dardel, C., Kergoat, L., Hiernaux, P., Mougin, E., Grippa, M., and Tucker, C. J.: Re-greening Sahel: 30 years of remote sensing data and field observations (Mali, Niger), Remote Sens. Environ., 140, 350–364, https://doi.org/10.1016/j.rse.2013.09.011, 2014. a
de Jong, R., Schaepman, M. E., Furrer, R., de Bruin, S., and Verburg, P. H.: Spatial relationship between climatologies and changes in global vegetation activity, Glob. Change Biol., 19, 1953–1964, https://doi.org/10.1111/gcb.12193, 2013. a
Didan, K.: MOD13Q1 MODIS/Terra vegetation indices 16-day L3 global 250 m SIN grid V006, NASA EOSDIS Land Processes DAAC [data set], https://doi.org/10.5067/MODIS/MOD13Q1.006, 2015a. a
Didan, K.: MYD13Q1 MODIS/Terra vegetation indices 16-day L3 global 250 m SIN grid V006, NASA EOSDIS Land Processes DAAC [data set], https://doi.org/10.5067/MODIS/MYD13Q1.006, 2015b. a
Donohue, R. J., Mcvicar, T. R., and Roderick, M. L.: Climate-related trends in Australian vegetation cover as inferred from satellite observations, 1981–2006, Glob. Change Biol., 15, 1025–1039, https://doi.org/10.1111/j.1365-2486.2008.01746.x, 2009. a
Eklundh, L. and Olsson, L.: Vegetation index trends for the African Sahel 1982–1999, Geophys. Res. Lett., 30, 1430-1433, https://doi.org/10.1029/2002GL016772, 2003. a
Espinoza, J. C., Chavez, S., Ronchail, J., Junquas, C., Takahashi, K., and Lavado, W.: Rainfall hotspots over the southern tropical Andes: Spatial distribution, rainfall intensity, and relations with large-scale atmospheric circulation, Water Resour. Res., 51, 3459–3475, https://doi.org/10.1002/2014WR016273, 2015. a
Fensholt, R., Langanke, T., Rasmussen, K., Reenberg, A., Prince, S. D., Tucker, C., Scholes, R. J., Le, Q. B., Bondeau, A., Eastman, R., Epstein, H., Gaughan, A. E., Hellden, U., Mbow, C., Olsson, L., Paruelo, J., Schweitzer, C., Seaquist, J., and Wessels, K.: Greenness in semi-arid areas across the globe 1981–2007 – an Earth Observing Satellite based analysis of trends and drivers, Remote Sens. Environ., 121, 144–158, https://doi.org/10.1016/j.rse.2012.01.017, 2012. a, b
Forzieri, G., Feyen, L., Cescatti, A., and Vivoni, E. R.: Spatial and temporal variations in ecosystem response to monsoon precipitation variability in southwestern North America, J. Geophys. Res.-Biogeo., 119, 1999–2017, https://doi.org/10.1002/2014JG002710, 2014. a
Funk, C., Peterson, P., Landsfeld, M., Pedreros, D., Verdin, J., Shukla, S., Husak, G., Rowland, J., Harrison, L., Hoell, A., and Michaelsen, J.: The climate hazards infrared precipitation with stations – A new environmental record for monitoring extremes, Scientific Data, 2, 1–21, https://doi.org/10.1038/sdata.2015.66, 2015. a
Garreaud, R. D.: The Andes climate and weather, Adv. Geosci., 22, 3–11, https://doi.org/10.5194/adgeo-22-3-2009, 2009. a, b
Gray, S. B., Dermody, O., Klein, S. P., Locke, A. M., McGrath, J. M., Paul, R. E., Rosenthal, D. M., Ruiz-Vera, U. M., Siebers, M. H., Strellner, R., Ainsworth, E. A., Bernacchi, C. J., Long, S. P., Ort, D. R., and Leakey, A. D.: Intensifying drought eliminates the expected benefits of elevated carbon dioxide for soybean, Nature Plants, 2, 1–8, https://doi.org/10.1038/nplants.2016.132, 2016. a
Gurgiser, W., Juen, I., Singer, K., Neuburger, M., Schauwecker, S., Hofer, M., and Kaser, G.: Comparing peasants' perceptions of precipitation change with precipitation records in the tropical Callejón de Huaylas, Peru, Earth Syst. Dynam., 7, 499–515, https://doi.org/10.5194/esd-7-499-2016, 2016. a, b, c, d, e
Herrmann, S. M., Anyamba, A., and Tucker, C. J.: Recent trends in vegetation dynamics in the African Sahel and their relationship to climate, Global Environ. Chang., 15, 394–404, https://doi.org/10.1016/j.gloenvcha.2005.08.004, 2005. a
Hickler, T., Eklundh, L., Seaquist, J. W., Smith, B., Ardö, J., Olsson, L., Sykes, M. T., and Sjöström, M.: Precipitation controls Sahel greening trend, Geophys. Res. Lett., 32, L21415, https://doi.org/10.1029/2005GL024370, 2005. a
Huber, S., Fensholt, R., and Rasmussen, K.: Water availability as the driver of vegetation dynamics in the African Sahel from 1982 to 2007, Global Planet. Change, 76, 186–195, https://doi.org/10.1016/j.gloplacha.2011.01.006, 2011. a
Huffman, G. J., Stocker, E. F., Bolvin, D. T., Nelkin, E. J., and Tan, J.: GPM IMERG Final Precipitation L3 1 month 0.1 degree × 0.1 degree V06, Greenbelt, MD, Goddard Earth Sciences Data and Information Services Center (GES DISC) [data set], last access: 8 March 2021, https://doi.org/10.5067/GPM/IMERG/3B-MONTH/06, 2019. a
Huxman, T. E., Smith, M. D., Fay, P. A., Knapp, A. K., Shaw, M. R., Lolk, M. E., Smith, S. D., Tissue, D. T., Zak, J. C., Weltzin, J. F., Pockman, W. T., Sala, O. E., Haddad, B. M., Harte, J., Koch, G. W., Schwinning, S., Small, E. E., and Williams, D. G.: Convergence across biomes to a common rain-use efficiency, Nature, 429, 651–654, https://doi.org/10.1038/nature02561, 2004. a
Karnieli, A., Agam, N., Pinker, R. T., Anderson, M., Imhoff, M. L., Gutman, G. G., Panov, N., and Goldberg, A.: Use of NDVI and land surface temperature for drought assessment: Merits and limitations, J. Climate, 23, 618–633, https://doi.org/10.1175/2009JCLI2900.1, 2010. a
Kaser, G., Juen, I., Georges, C., Gómez, J., and Tamayo, W.: The impact of glaciers on the runoff and the reconstruction of mass balance history from hydrological data in the tropical Cordillera Bianca, Perú, J. Hydrol., 282, 130–144, https://doi.org/10.1016/S0022-1694(03)00259-2, 2003. a, b
Killeen, T. J., Douglas, M., Consiglio, T., Jørgensen, P. M., and Mejia, J.: Dry spots and wet spots in the Andean hotspot, J. Biogeogr., 34, 1357–1373, https://doi.org/10.1111/j.1365-2699.2006.01682.x, 2007. a
Knapp, A. K. and Smith, M. D.: Variation among biomes in temporal dynamics of aboveground primary production, Science, 291, 481–484, https://doi.org/10.1126/science.291.5503.481, 2001. a
Kogan, F. N.: Satellite-observed sensitivity of world land ecosystems to El Niño/La Niña, Remote Sens. Environ., 74, 445–462, https://doi.org/10.1016/S0034-4257(00)00137-1, 2000. a
Mark, B. G., Bury, J., McKenzie, J. M., French, A., and Baraer, M.: Climate Change and Tropical Andean Glacier Recession: Evaluating Hydrologic Changes and Livelihood Vulnerability in the Cordillera Blanca, Peru, Ann. Assoc. Am. Geogr., 100, 794–805, https://doi.org/10.1080/00045608.2010.497369, 2010. a
Mateo-Sanchis, A., Muñoz-Marí, J., Campos-Taberner, M., García-Haro, J., and Camps-Valls, G.: Gap filling of biophysical parameter time series with multi-output Gaussian Processes, in: Proceedings of the IGARSS 2018–2018 IEEE International Geoscience and Remote Sensing Symposium, Valencia, Spain, 22–27 July 2018, 4039–4042, https://doi.org/10.1109/IGARSS.2018.8519254, 2018. a
Maussion, F., Gurgiser, W., Großhauser, M., Kaser, G., and Marzeion, B.: ENSO influence on surface energy and mass balance at Shallap Glacier, Cordillera Blanca, Peru, The Cryosphere, 9, 1663–1683, https://doi.org/10.5194/tc-9-1663-2015, 2015. a, b, c
Miralles, D. G., Van Den Berg, M. J., Gash, J. H., Parinussa, R. M., De Jeu, R. A., Beck, H. E., Holmes, T. R., Jiménez, C., Verhoest, N. E., Dorigo, W. A., Teuling, A. J., and Johannes Dolman, A.: El Niño-La Niña cycle and recent trends in continental evaporation, Nat. Clim. Change, 4, 122–126, https://doi.org/10.1038/nclimate2068, 2014. a
Nemani, R. R., Keeling, C. D., Hashimoto, H., Jolly, W. M., Piper, S. C., Tucker, C. J., Myneni, R. B., and Running, S. W.: Climate-driven increases in global terrestrial net primary production from 1982 to 1999, Science, 300, 1560–1563, https://doi.org/10.1126/science.1082750, 2003. a
O'Neill, P., Chan, S., Njoku, E., Jackson, T., Bindlish, R., Chaubell, J., and Colliander, A.: SMAP Enhanced L3 Radiometer Global and Polar Grid Daily 9 km EASE-Grid Soil Moisture, Version 5, National Snow and Ice Data Center (subset of Rio Santa basin), https://doi.org/10.5067/4DQ54OUIJ9DL, 2021. a
Pipia, L., Muñoz-Marí, J., Amin, E., Belda, S., Camps-Valls, G., and Verrelst, J.: Fusing optical and SAR time series for LAI gap fillingwith multioutput Gaussian processes, Remote Sens. Environ., 235, 111452, https://doi.org/10.1016/j.rse.2019.111452, 2019. a
Polk, M. H., Mishra, N. B., Young, K. R., and Mainali, K.: Greening and browning trends across Peru's diverse environments, Remote Sens.-Basel, 12, 2418, https://doi.org/10.3390/rs12152418, 2020. a
Potter, C. S. and Brooks, V.: Global analysis of empirical relations between annual climate and seasonality of NDVI, Int. J. Remote Sens., 19, 2921–2948, https://doi.org/10.1080/014311698214352, 1998. a
Rabatel, A., Francou, B., Soruco, A., Gomez, J., Cáceres, B., Ceballos, J. L., Basantes, R., Vuille, M., Sicart, J.-E., Huggel, C., Scheel, M., Lejeune, Y., Arnaud, Y., Collet, M., Condom, T., Consoli, G., Favier, V., Jomelli, V., Galarraga, R., Ginot, P., Maisincho, L., Mendoza, J., Ménégoz, M., Ramirez, E., Ribstein, P., Suarez, W., Villacis, M., and Wagnon, P.: Current state of glaciers in the tropical Andes: a multi-century perspective on glacier evolution and climate change, The Cryosphere, 7, 81–102, https://doi.org/10.5194/tc-7-81-2013, 2013. a
Rasmussen, C. E.: Gaussian Processes in machine learning, Lect. Note. Comput. Sc., 3176, 63–71, https://doi.org/10.1007/978-3-540-28650-9_4, 2004. a
Rau, P., Bourrel, L., Labat, D., Melo, P., Dewitte, B., Frappart, F., Lavado, W., and Felipe, O.: Regionalization of rainfall over the Peruvian Pacific slope and coast, Int. J. Climatol., 37, 143–158, https://doi.org/10.1002/joc.4693, 2017. a, b
Reich, P. B., Hobbie, S. E., and Lee, T. D.: Plant growth enhancement by elevated CO2 eliminated by joint water and nitrogen limitation, Nat. Geosci., 7, 920–924, https://doi.org/10.1038/ngeo2284, 2014. a
Richard, Y. and Poccard, I.: A statistical study of NDVI sensitivity to seasonal and interannual rainfall variations in Southern Africa, Int. J. Remote Sens., 19, 2907–2920, https://doi.org/10.1080/014311698214343, 1998. a
Richardson, A. D., Keenan, T. F., Migliavacca, M., Ryu, Y., Sonnentag, O., and Toomey, M.: Climate change, phenology, and phenological control of vegetation feedbacks to the climate system, Agr. Forest Meteorol., 169, 156–173, https://doi.org/10.1016/j.agrformet.2012.09.012, 2013. a
Richardson, A. D., Hufkens, K., Milliman, T., Aubrecht, D. M., Furze, M. E., Seyednasrollah, B., Krassovski, M. B., Latimer, J. M., Nettles, W. R., Heiderman, R. R., Warren, J. M., and Hanson, P. J.: Ecosystem warming extends vegetation activity but heightens vulnerability to cold temperatures, Nature, 560, 368–371, https://doi.org/10.1038/s41586-018-0399-1, 2018. a
Rivera, J. A., Marianetti, G., and Hinrichs, S.: Validation of CHIRPS precipitation dataset along the Central Andes of Argentina, Atmos. Res., 213, 437–449, https://doi.org/10.1016/j.atmosres.2018.06.023, 2018. a
Rodriguez-Iturbe, I., D'Odorico, P., Porporato, A., and Ridolfi, L.: On the spatial and temporal links between vegetation, climate, and soil moisture, Water Resour. Res., 35, 3709–3722, https://doi.org/10.1029/1999WR900255, 1999. a
Rouse, J. W. J., Haas, R. H., Schell, J. A., and Deering, D. W.: Monitoring vegetation systems in the Great Plains with ERTS, in: Third ERTS Symposium, NASA SP-351, Washington DC, 10 December 1973, 309–317, 1974. a
Sanabria, J., Bourrel, L., Dewitte, B., Frappart, F., Rau, P., Solis, O., and Labat, D.: Rainfall along the coast of Peru during strong El Niño events, Int. J. Climatol., 38, 1737–1747, https://doi.org/10.1002/joc.5292, 2018. a
Sanabria, J., Carrillo, C. M., and Labat, D.: Unprecedented Rainfall and Moisture Patterns during El Niño 2016 in the Eastern Pacific and Tropical Andes: Northern Perú and Ecuador, Atmosphere, 10, 768, https://doi.org/10.3390/atmos10120768, 2019. a
Schauwecker, S., Rohrer, M., Acuña, D., Cochachin, A., Dávila, L., Frey, H., Giráldez, C., Gómez, J., Huggel, C., Jacques-Coper, M., Loarte, E., Salzmann, N., and Vuille, M.: Climate trends and glacier retreat in the Cordillera Blanca, Peru, revisited, Global Planet. Change, 119, 85–97, https://doi.org/10.1016/j.gloplacha.2014.05.005, 2014. a, b, c, d
Schwinning, S., Sala, O. E., Loik, M. E., and Ehleringer, J. R.: Thresholds, memory, and seasonality: understanding pulse dynamics in arid/semi-arid ecosystems, Oecologia, 141, 191–193, https://doi.org/10.1007/s00442-004-1683-3, 2004. a
Segura, H., Junquas, C., Espinoza, J. C., Vuille, M., Jauregui, Y. R., Rabatel, A., Condom, T., and Lebel, T.: New insights into the rainfall variability in the tropical Andes on seasonal and interannual time scales, Clim. Dynam., 53, 405–426, https://doi.org/10.1007/s00382-018-4590-8, 2019. a, b, c
Sitch, S., Friedlingstein, P., Gruber, N., Jones, S. D., Murray-Tortarolo, G., Ahlström, A., Doney, S. C., Graven, H., Heinze, C., Huntingford, C., Levis, S., Levy, P. E., Lomas, M., Poulter, B., Viovy, N., Zaehle, S., Zeng, N., Arneth, A., Bonan, G., Bopp, L., Canadell, J. G., Chevallier, F., Ciais, P., Ellis, R., Gloor, M., Peylin, P., Piao, S. L., Le Quéré, C., Smith, B., Zhu, Z., and Myneni, R.: Recent trends and drivers of regional sources and sinks of carbon dioxide, Biogeosciences, 12, 653–679, https://doi.org/10.5194/bg-12-653-2015, 2015. a
Spracklen, D. V., Arnold, S. R., and Taylor, C. M.: Observations of increased tropical rainfall preceded by air passage over forests, Nature, 489, 282–285, https://doi.org/10.1038/nature11390, 2012. a
Svoray, T. and Karnieli, A.: Rainfall, topography and primary production relationships in a semiarid ecosystem, Ecohydrology, 4, 56–66, https://doi.org/10.1002/eco.123, 2011. a
Torres-Batlló, J. and Martí-Cardona, B.: Precipitation trends over the southern Andean Altiplano from 1981 to 2018, J. Hydrol., 590, 125485, https://doi.org/10.1016/j.jhydrol.2020.125485, 2020. a, b
Tote, C., Beringhs, K., Swinnen, E., and Govers, G.: Monitoring environmental change in the Andes based on SPOT-VGT and NOAA-AVHRR time series analysis, in: 2011 6th International Workshop on the Analysis of Multi-Temporal Remote Sensing Images, Multi-Temp 2011 – Proceedings, Trento, Italy, 12–14 July 2011, 268–272, 2011. a
Trenberth, K. E.: The definition of El Nino, B. Am. Meteorol. Soc., 78, 2771–2778, https://doi.org/10.1175/1520-0477(1997)078<2771:TDOENO>2.0.CO;2, 1997. a, b
Urrutia, R. and Vuille, M.: Climate change projections for the tropical Andes using a regional climate model: Temperature and precipitation simulations for the end of the 21st century, J. Geophys. Res., 114, D02108, https://doi.org/10.1029/2008JD011021, 2009. a
USGS EROS Archive: Digital Elevation – Shuttle Radar Topography Mission (SRTM) 1 Arc-second Global, USGS EROS Archive [data set], https://www.usgs.gov/centers/eros, last access: January 2021. a
Verstraete, M. M., Gobron, N., Aussedat, O., Robustelli, M., Pinty, B., Widlowski, J. L., and Taberner, M.: An automatic procedure to identify key vegetation phenology events using the JRC-FAPAR products, Adv. Space Res., 41, 1773–1783, https://doi.org/10.1016/j.asr.2007.05.066, 2008. a
Vrieling, A., de Leeuw, J., and Said, M.: Length of Growing Period over Africa: Variability and Trends from 30 Years of NDVI Time Series, Remote Sens.-Basel, 5, 982–1000, https://doi.org/10.3390/rs5020982, 2013. a
Vuille, M., Bradley, R. S., Werner, M., and Keimig, F.: 20th century climate change in the tropical Andes: observations and model results, in: Climate variability and change in high elevation regions: Past, present & future, edited by: Diaz, H. F., 75–99, Springer, Dordrecht, 2003. a, b
Vuille, M., Kaser, G., and Juen, I.: Glacier mass balance variability in the Cordillera Blanca, Peru and its relationship with climate and the large-scale circulation, Global Planet. Change, 62, 14–28, https://doi.org/10.1016/j.gloplacha.2007.11.003, 2008. a, b
Whittaker, E. T.: On a new method of graduation, P. Edinburgh Math. Soc., 41, 63–75, https://doi.org/10.1017/s0013091500077853, 1922. a
Wu, D., Zhao, X., Liang, S., Zhou, T., Huang, K., Tang, B., and Zhao, W.: Time-lag effects of global vegetation responses to climate change, Glob. Change Biol., 21, 3520–3531, https://doi.org/10.1111/gcb.12945, 2015. a
Xu, C., Liu, H., Williams, A. P., Yin, Y., and Wu, X.: Trends toward an earlier peak of the growing season in Northern Hemisphere mid-latitudes, Glob. Change Biol., 22, 2852–2860, https://doi.org/10.1111/gcb.13224, 2016. a
Yang, J., Tian, H., Pan, S., Chen, G., Zhang, B., and Dangal, S.: Amazon drought and forest response: Largely reduced forest photosynthesis but slightly increased canopy greenness during the extreme drought of 2015/2016, Glob. Change Biol., 24, 1919–1934, https://doi.org/10.1111/gcb.14056, 2018. a
Young, K. R., Ponette-González, A. G., Polk, M. H., and Lipton, J. K.: Snowlines and Treelines in the Tropical Andes, Ann. Am. Assoc. Geogr., 107, 429–440, https://doi.org/10.1080/24694452.2016.1235479, 2017. a
Zhang, X.: Monitoring the response of vegetation phenology to precipitation in Africa by coupling MODIS and TRMM instruments, J. Geophys. Res., 110, D12103, https://doi.org/10.1029/2004JD005263, 2005. a
Zhu, Z., Piao, S., Myneni, R. B., Huang, M., Zeng, Z., Canadell, J. G., Ciais, P., Sitch, S., Friedlingstein, P., Arneth, A., Cao, C., Cheng, L., Kato, E., Koven, C., Li, Y., Lian, X., Liu, Y., Liu, R., Mao, J., Pan, Y., Peng, S., Peuelas, J., Poulter, B., Pugh, T. A., Stocker, B. D., Viovy, N., Wang, X., Wang, Y., Xiao, Z., Yang, H., Zaehle, S., and Zeng, N.: Greening of the Earth and its drivers, Nat. Clim. Change, 6, 791–795, https://doi.org/10.1038/nclimate3004, 2016. a